Advanced Techniques for Emotional Tone Analysis in Surveys
-
Bella Williams
- 10 min read
Emotion Tone Analytics has emerged as a powerful tool in the realm of survey research, revolutionizing how we interpret respondent sentiments. Traditional survey analysis often focuses merely on quantitative data, leaving behind a wealth of emotional insights. By incorporating emotional tone analytics, researchers can gain a clearer understanding of not only what respondents think, but also how they feel about their experiences and opinions.
As we delve deeper into emotion tone analytics, it becomes clear that this approach enhances decision-making in various fields such as marketing and human resources. Understanding emotional responses can lead to more effective strategies, catering to the nuanced perspectives of different audiences. This section lays the groundwork for exploring advanced techniques that harness these insights, paving the way for more targeted and impactful survey outcomes.
Analyze & Evaluate Calls. At Scale.
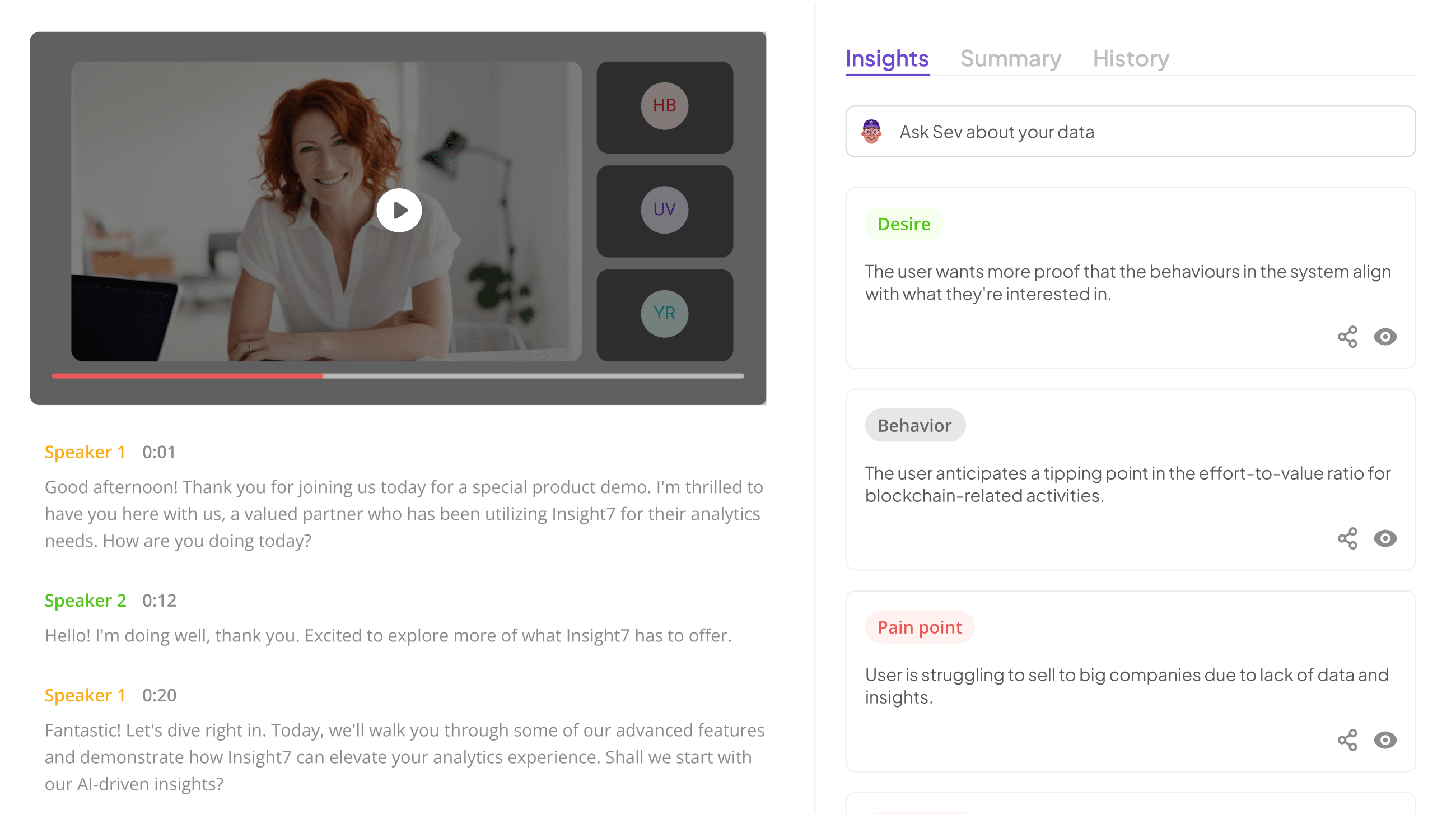
Emotion Tone Analytics: Understanding Its Importance in Surveys
Emotion Tone Analytics plays a pivotal role in modern survey techniques, enhancing the understanding of respondents' feelings and attitudes. By analyzing emotional tone, researchers can uncover insights that go beyond mere numerical data. This deeper understanding facilitates more effective decision-making, allowing organizations to gauge customer sentiment and engagement accurately.
Various industries, especially marketing and human resources, have begun to harness these insights. For example, businesses can tailor their strategies based on emotional tone data, leading to targeted campaigns or improved employee relations. Recognizing emotional cues helps organizations connect meaningfully with their audience, ultimately influencing key strategic decisions. As Emotion Tone Analytics continues to evolve, its implementation will refine how feedback is collected and interpreted, ensuring more nuanced and effective insights.
The Role of Emotion Tone Analytics in Modern Survey Techniques
Emotion Tone Analytics plays a pivotal role in modern survey techniques by enriching the data collection process. Traditional survey methods often focus solely on quantitative metrics, leaving richer qualitative insights untapped. With Emotion Tone Analytics, researchers can interpret how respondents truly feel about a subject, going beyond mere numbers to capture the essence of their sentiments. This deeper understanding can enhance decision-making and strategy formulation.
Incorporating Emotion Tone Analytics into surveys requires careful consideration of elements such as context, wording, and the emotional framework of questions. By analyzing vocal tone and language used in responses, organizations can gain insights into customer satisfaction, employee engagement, and market trends. Ultimately, the integration of this analytical approach not only improves respondent experience but also enables businesses to respond more effectively to customer needs and market dynamics. Adopting these advanced techniques signals a commitment to leveraging emotional data for strategic growth.
- Explanation of why emotional tone analysis is crucial in understanding survey responses.
Understanding the emotional tone behind survey responses is essential for gaining a deeper insight into participant feelings and sentiments. Emotion tone analysis reveals the subtleties of emotions that plain numerical data fails to capture. This technique allows researchers to contextualize feedback, enhancing the overall understanding of customer attitudes and satisfaction levels.
Beyond merely analyzing quantitative data, emotional tone analytics enables organizations to identify patterns in respondentsโ emotions. By discerning whether feedback leans towards positivity or negativity, businesses can better tailor their strategies, leading to improved customer experiences. Additionally, this approach allows for more nuanced interpretations, providing valuable context that can drive decision-making. Ultimately, incorporating emotional tone analysis fosters a more empathetic connection with respondents, ensuring that their voices are genuinely heard and understood. This is crucial in a landscape where emotional intelligence can set a brand apart from its competitors.
- Overview of how this technique offers deeper insights beyond numerical data.
Emotion Tone Analytics takes survey data analysis beyond mere numbers, revealing the underlying sentiments that shape consumer opinions. By focusing on emotional nuances, this technique can unearth feelings such as frustration, satisfaction, or enthusiasm that simple numerical ratings might overlook. Instead of just calculating averages or percentages, understanding emotional tones creates a more comprehensive perspective on what respondents truly feel.
Two primary advantages illustrate how Emotion Tone Analytics provides deeper insights:
Contextual Understanding: This technique offers context for the data, allowing researchers to discern why certain ratings were given. For instance, positive feedback might accompany strong emotional expressions that indicate not just satisfaction, but genuine delight or relief.
Pattern Recognition: It enables the identification of emotional trends within diverse demographic segments. By analyzing how different groups respond emotionally, organizations gain actionable insights that can drive more effective strategies and enhance product offerings.
Such in-depth analyses can transform how organizations engage with respondents and develop solutions tailored to their emotional journeys.
Real-Life Applications of Emotion Tone Analytics
Emotion Tone Analytics plays a significant role across various industries, enhancing the way organizations interpret survey data. For instance, in marketing, brands utilize emotional tone insights to improve their messaging strategies. By assessing customer responses, companies can tailor their campaigns to resonate emotionally with their audience. This approach not only fosters stronger customer relationships but also drives engagement and conversion rates.
Similarly, human resources departments leverage Emotion Tone Analytics for employee feedback and engagement surveys. Understanding the emotional tone of responses allows HR professionals to identify underlying issues, creating a more supportive workplace environment. This analytical technique aids in shaping policies and strategies that enhance employee satisfaction and retention. Consequently, organizations that effectively implement emotion tone analysis gain a competitive advantage, making data-driven decisions that reflect both quantitative and qualitative insights from their surveys. Ultimately, applying this technology bridges the gap between numbers and human experience.
- Discussion of industries leveraging emotional tone insights, such as marketing and human resources.
In various industries, understanding emotional tone provides a powerful advantage, especially in marketing and human resources. These fields utilize emotional tone analytics to gain insights that directly impact strategies and decision-making processes. In marketing, for instance, brands can tailor their messaging to resonate more deeply with their target audience by analyzing customer feedback and responses. This understanding helps create campaigns that evoke the desired emotions, ultimately driving engagement and conversions.
Similarly, human resources departments benefit significantly from emotional tone insights. By analyzing employee feedback, HR can identify underlying sentiments, address concerns, and improve workplace culture. This, in turn, leads to higher employee satisfaction and retention rates. Both industries illustrate how critical it is to interpret emotional nuances in communication. Ultimately, leveraging these insights fosters better relationships and enhances overall performance, making emotion tone analytics an essential tool for organizational success.
- Examples of how emotional tone data can influence strategic decisions.
Emotion Tone Analytics provides valuable insights that shape strategic decision-making. For example, companies can analyze customer feedback to identify trends in emotional responses. Understanding whether the sentiment in survey responses is positive, negative, or neutral can guide marketing strategies. A sudden surge in negative feedback might prompt a review of customer service practices or product quality, ensuring that companies remain responsive to their audience's needs.
Furthermore, emotional tone data can prioritize areas for innovation. If survey responses indicate frustration over a specific feature, product development teams can focus on enhancements to improve user experience. This approach not only addresses customer pain points but also builds stronger brand loyalty. Ultimately, organizations that harness Emotion Tone Analytics effectively can make informed, proactive decisions that drive success. By staying attuned to the emotional climate surrounding their products or services, businesses can cultivate a competitive edge in the marketplace.
Extract insights from interviews, calls, surveys and reviews for insights in minutes
Advanced Techniques in Emotion Tone Analytics
Advanced Techniques in Emotion Tone Analytics focus on enhancing our understanding of emotional responses using sophisticated methodologies. In the realm of surveys, it is essential to move beyond the surface-level data, digging deeper into the emotional nuances that shape responses. This deep dive not only provides clearer insights but also equips stakeholders with precise information needed for strategic enhancements.
One notable advancement involves the use of machine learning algorithms to detect emotional tones within responses. Training these models requires a systematic approach that includes gathering diverse datasets and continuously refining algorithms based on feedback. Additionally, natural language processing (NLP) plays a crucial role; it helps in parsing language intricacies, allowing for more accurate recognition of emotional cues. This combination of machine learning and NLP not only improves the quality of emotion tone analytics but also empowers organizations to make informed decisions based on profound emotional insights.
Machine Learning in Emotion Tone Analytics
Machine learning plays a pivotal role in emotion tone analytics, enhancing our understanding of emotional responses in survey data. By utilizing advanced algorithms, machine learning models can be trained to identify subtle emotional cues in text. This capability enables organizations to gain deeper insights into customer sentiment, which traditional methods may overlook.
There are several key steps involved in employing machine learning for emotion tone analysis. First, data collection is essential; gathering a diverse array of survey responses forms the foundation of the analysis. Next, data pre-processing helps clean and structure the input, ensuring the model receives optimal information. Following this, training the model involves using labeled datasets to teach it how to recognize specific emotional tones. Lastly, evaluation and refinement are crucial for improving accuracy over time. Ultimately, these techniques empower organizations to tailor their strategies based on nuanced emotional insights, leading to more effective engagement with their audiences.
- Introduction to the application of machine learning algorithms in emotional tone detection.
Emotion Tone Analytics offers a revolutionary approach to understanding human responses in surveys. As organizations seek to capture not just data, but the emotions driving that data, machine learning algorithms have become essential tools. These algorithms can sift through vast amounts of textual data, identifying emotional cues that traditional methods might overlook, thereby enriching the interpretation of survey results.
The application of machine learning in emotional tone detection empowers businesses to extract nuanced insights. By training models on diverse datasets, these algorithms learn to recognize patterns associated with different emotional states. This innovative technique transforms raw survey responses into a wealth of information, enabling organizations to make informed decisions based on the true sentiments of their respondents.
- Step-by-step outline of how machine learning models are trained to recognize emotional cues.
To train machine learning models for recognizing emotional cues, we follow distinct steps that ensure accuracy and reliability. The process begins with gathering and preprocessing data, which often includes cleaning text input and removing irrelevant information. This ensures that the models learn from quality data, enhancing emotion tone analytics.
Next, we utilize labeled datasets that contain examples of various emotional expressions. By employing supervised learning algorithms, the models learn to identify patterns associated with specific emotions. These algorithms are continuously refined through the validation process, where model performance is evaluated against a set of unseen data. Hyperparameter tuning is also crucial, as it optimizes the model's ability to generalize emotional cues effectively.
Finally, once trained, the machine learning models undergo testing to confirm their accuracy in recognizing emotions. By following these steps, businesses can better leverage emotion tone analytics, providing deeper insights from survey data to inform decision-making strategies.
Natural Language Processing (NLP) for Enhanced Emotion Tone Analysis
Natural Language Processing (NLP) has emerged as a powerful tool for enhancing emotion tone analytics in surveys. By utilizing NLP techniques, organizations can effectively process and analyze large volumes of text data, thereby extracting meaningful insights regarding emotional tones in customer feedback. This technique helps transform raw data into understandable patterns that highlight customer sentiments, moving beyond simple quantitative analysis.
The implementation of NLP for emotion tone analytics involves several key steps. Firstly, data preprocessing ensures that the text is clean and structured, making it easier to analyze. Next, sentiment analysis algorithms are applied to detect and classify emotions expressed in the text. Finally, insights are interpreted, allowing businesses to make informed decisions based on emotional cues. By integrating NLP into their analysis, organizations can gain a competitive edge by responding promptly to customer needs and adjusting strategies accordingly.
- Exploration of how NLP tools can be used to refine emotion detection.
Natural Language Processing (NLP) tools offer robust solutions for refining emotion detection in survey responses. By analyzing text, these tools can extract emotional nuances that traditional analysis might miss. This ability enhances Emotion Tone Analytics, allowing businesses to better understand customer sentiments and expectations.
To implement NLP for refining emotion detection, several key steps should be considered. First, data preprocessing is essential, where text is cleaned and prepared for analysis. Next, sentiment analysis algorithms can classify emotions within the responses. Utilizing machine learning models can further improve accuracy by training on diverse datasets that represent various emotional expressions. Additionally, developing custom emotion lexicons specific to industry jargon can enhance the relevance of insights gained.
Through the strategic application of NLP tools, organizations can transform raw survey data into actionable insights, thus driving informed decisions that resonate more effectively with target audiences.
- Steps involved in implementing NLP techniques for analyzing survey data.
To implement NLP techniques for analyzing survey data, itโs essential to follow structured steps to ensure effective Emotion Tone Analytics. First, data collection must be organized, ideally sourcing open-ended responses that provide rich emotional context. Next, preprocessing is key; this involves cleaning the text by removing extraneous characters and normalizing the language. By transforming the data into a suitable format, we enhance the accuracy of our analytical models.
After preprocessing, the extraction of emotional features takes place. Utilizing NLP libraries, such as NLTK or SpaCy, enables the identification of keywords and phrases that signal emotional tone. Subsequently, deploying sentiment analysis models becomes vital, typically involving training supervised learning algorithms to recognize specific emotional nuances. Finally, visualizing the results in a coherent manner not only aids in understanding but also allows stakeholders to derive actionable insights swiftly. By following these steps, organizations can harness the power of NLP to significantly enhance their survey data analysis efforts.
Top Tools for Emotion Tone Analytics in Surveys
Emotion Tone Analytics plays a pivotal role in modern survey techniques, allowing researchers to glean deeper insights from participant responses. One effective way to implement this analysis is through specialized tools designed to dissect and interpret emotional undertones in textual data. Comprehending emotional tones can elevate survey analysis by providing context beyond mere numerical responses, enhancing overall data comprehension.
Among the top tools available, several stand out in facilitating this process. First, MonkeyLearn harnesses machine learning capabilities to automate emotion detection, streamlining the analysis of large datasets. Next is IBM Watson Tone Analyzer, which categorizes emotional tones, enabling nuanced interpretations of participant sentiments. Lexalytics offers comprehensive analytics by examining language to uncover emotional insights, while Google Cloud Natural Language utilizes robust natural language processing techniques to dissect sentiments effectively. Each of these tools contributes uniquely to Emotion Tone Analytics, enhancing the quality of survey insights and informing strategic decisions.
insight7
To unlock the full potential of Emotion Tone Analytics, it is vital to grasp its multifaceted nature and practical applications. This advanced technique allows organizations to gain deeper insights into user sentiments, transforming raw data into actionable intelligence. The ability to analyze emotional tones can enhance understanding of customer feedback, leading to improved service strategies and marketing approaches.
There are several key factors to consider when implementing Emotion Tone Analytics effectively. First, the integration of machine learning algorithms plays a significant role in refining data analysis. These algorithms are designed to recognize emotional cues within textual data, enabling precise identification of sentiments expressed by respondents. Second, the application of Natural Language Processing (NLP) enhances the clarity and depth of emotion detection, allowing analysts to extract nuanced insights from complex survey responses. By understanding and applying these advanced techniques, organizations can foster stronger connections with their customers and stay ahead of emerging market trends.
- Overview of insight7 and its capabilities in emotional tone analysis.
The platform offers comprehensive capabilities in emotional tone analysis, greatly enhancing the ability to sift through vast amounts of qualitative data. By providing intuitive tools, it allows users to easily analyze customer interactions at scale. Traditional methods of analysis often leave companies with scattered insights that can be difficult to interpret. However, this platform not only streamlines the analysis but also highlights the emotional tones that underlie customer feedback.
Understanding emotional tones can be incredibly beneficial for organizations. It enables companies to identify connection points and friction in customer experiences, informing strategic decisions. By tapping into these insights, businesses can transform raw data into actionable strategies, leading to improved customer engagement and satisfaction. Ultimately, emotional tone analytics empowers organizations to stay ahead in a competitive landscape by effectively managing and interpreting customer sentiment.
Other Leading Tools
In the evolving field of Emotion Tone Analytics, various leading tools are available to enhance the effectiveness of emotional tone analysis in surveys. Each tool offers unique features that cater to different analytics needs, allowing businesses to assess customer sentiments more accurately.
MonkeyLearn stands out with its machine learning capabilities, enabling users to customize sentiment analysis models based on their specific requirements. Meanwhile, IBM Watson Tone Analyzer excels in differentiating and assessing tones in written text, providing comprehensive insights into emotional nuances. Lexalytics also offers advanced analytics that combine machine learning and natural language processing, allowing for in-depth emotion detection. Lastly, Google Cloud Natural Language leverages robust NLP services to provide a seamless integration for evaluating emotional tones in survey data.
By exploring these tools, organizations can better translate customer insights into actionable strategies, ultimately fostering stronger connections with their audience. These options empower companies to stay ahead of the competition in understanding emotional responses, which can lead to more informed decision-making.
- MonkeyLearn: A guide on using MonkeyLearns machine learning features for emotion analysis.
Understanding Emotion Tone Analytics is essential when utilizing advanced machine learning features for effective emotion analysis. This platform enables users to effortlessly extract and evaluate emotional cues from survey data, making the process accessible for teams across various industries. By implementing these tools, organizations can unveil significant insights that go beyond conventional data metrics. This transformation offers a clearer understanding of customer sentiments, ultimately empowering decision-makers.
To leverage these features successfully, follow these steps. First, upload your survey data or conversation transcripts directly onto the platform. Next, utilize the built-in capabilities to analyze this text for emotional tone indicators, like sentiment or mood variations. Then, interpret the output through visual summaries and detailed analytics. Lastly, apply these insights to address customer needs, refine marketing strategies, or enhance employee engagement. This systematic approach ensures a comprehensive understanding of emotional tone, enabling organizations to make informed and impactful decisions.
- IBM Watson Tone Analyzer: How this tool differentiates and assesses tones in survey responses.
The IBM Watson Tone Analyzer stands as a powerful tool in the domain of Emotion Tone Analytics, particularly in the analysis of survey responses. It excels in parsing through textual data to identify emotional tones, such as joy, sadness, anger, and others. By utilizing advanced algorithms, this tool can differentiate among various emotional expressions, providing a nuanced understanding of participant sentiments.
This analysis is essential for organizations looking to enhance their survey data insights. For instance, the tool can reveal how respondents truly feel about a product or service beyond mere satisfaction ratings. As a result, businesses can tailor their strategies based on actual emotional reactions rather than relying solely on quantitative feedback. Understanding these emotional tones enables companies to make informed, empathetic adjustments in their offerings, leading to better customer engagement and satisfaction.
Through careful assessment of emotional signals, organizations gain an in-depth perspective that can influence their decision-making processes and improve overall effectiveness in communicating with their audiences.
- Lexalytics: Insight into its advanced analytics and emotion detection techniques.
Advanced analytics in emotion detection involves sophisticated techniques that enhance understanding of emotional tone in survey responses. Tools today can seamlessly analyze large data sets, extracting nuanced insights that go beyond basic sentiment. Utilizing machine learning, these platforms recognize emotional cues, allowing businesses to gain a deeper comprehension of customer experiences.
Incorporating natural language processing further refines this analysis. This technology dissects language patterns, making it possible to identify subtle emotional nuances. By utilizing these advanced analytics, organizations can effectively respond to customersโ needs, tailoring their strategies based on genuine emotional feedback. Such approaches are essential in fostering meaningful relationships with consumers, ultimately leading to improved satisfaction and loyalty.
Understanding these advanced techniques is crucial for any business looking to harness the power of emotional tone analytics. By doing so, they can make informed decisions that resonate with their audience on a deeper emotional level.
- Google Cloud Natural Language: Discussion on leveraging Googles NLP services for emotional tone analysis.
Utilizing advanced technology for emotion tone analysis can significantly enhance survey insights. One effective method involves applying natural language processing (NLP) tools to scrutinize textual data for emotional cues. By tapping into sophisticated algorithms, organizations can effectively gauge the emotional tone of respondents, leading to a deeper understanding of their sentiments. This goes beyond mere metrics, enabling businesses to connect with their audience on a more profound level.
The key advantages of using NLP services for emotional tone analysis include improved accuracy in detecting emotions, the ability to analyze large datasets, and streamlined processing times. First, these tools can interpret language nuances and context to accurately identify feelings such as joy, anger, sadness, or surprise. Second, processing vast amounts of survey data becomes feasible, allowing organizations to uncover widespread sentiment patterns. Lastly, this rapid analysis facilitates timely decision-making, ensuring businesses remain agile and responsive to customer needs. By embracing these techniques, organizations can truly elevate their understanding of consumer emotions.
Conclusion: The Future of Emotion Tone Analytics in Surveys
The future of Emotion Tone Analytics in surveys is poised for transformative growth, reflecting advancements in artificial intelligence and data processing techniques. As organizations increasingly recognize the significance of understanding emotional nuances, these analytics provide a pathway to richer insights that drive better decision-making. By integrating machine learning and natural language processing, survey responses can deliver more than just data; they can reveal deeper human experiences and sentiments.
In the coming years, we can expect enhanced capabilities that will refine how Emotion Tone Analytics is applied. Emerging trends, such as real-time sentiment analysis and multi-dimensional emotional mapping, will allow businesses to adapt quickly to customer feedback. This evolution will empower organizations to create personalized experiences and strategies grounded in genuine emotional understanding, shaping a more empathetic approach to engagement.
- Recap of the advancements and importance of emotion tone analytics.
Advancements in emotion tone analytics have transformed how we interpret survey data, making it a critical tool for organizations seeking deeper insights. By analyzing the emotional tone behind responses, businesses can better understand customer sentiments and motives that numbers alone may not reveal. This added layer offers a richer context, enhancing decision-making processes across various sectors.
The importance of emotion tone analytics extends beyond mere data interpretation. It empowers organizations to tailor their approaches in marketing, customer service, and human resources by providing actionable insights. For instance, an understanding of emotional tone can significantly improve customer engagement strategies and foster better relationships with clients. As technology evolves, it will be essential for organizations to harness these advancements to remain competitive and responsive to their audiences' needs.
- Predictions for future developments in the field and emerging trends.
As Emotion Tone Analytics continues to evolve, several predictions emerge regarding its future development and emerging trends. Firstly, a significant rise in the integration of artificial intelligence technologies is anticipated. This will enhance the precision of emotional tone detection, enabling systems to analyze complex emotional cues more effectively. Secondly, advancements in natural language processing are expected to refine the granularity of insights obtained from survey responses. This will empower organizations to better understand the nuances of consumer sentiment.
Moreover, the use of Emotion Tone Analytics will likely expand across diverse sectors, including healthcare, education, and finance. Such expansion will enhance the ability to gauge stakeholder attitudes and improve engagement strategies.
Lastly, the increasing demand for real-time analytics suggests that surveys will evolve, allowing organizations to capture emotional responses instantly. This trend will ultimately lead to more responsive and adaptive decision-making processes across various industries.