Launching an AI QA Pilot can be a transformative step for organizations looking to integrate artificial intelligence into their quality assurance processes. This pilot phase serves as an essential testing ground, allowing teams to assess the technology's practical applications and align it with their unique requirements. It provides a structured environment to identify potential benefits and pitfalls, ensuring that the final implementation is both effective and efficient.
Successful deployment starts with careful planning and collaboration among various stakeholders. Establishing clear objectives and key metrics during the pilot phase will enable teams to evaluate the AI QA tools' performance accurately. By focusing on well-defined criteria and maintaining open communication, organizations can derive meaningful insights that inform their approach to full deployment.
Analyze qualitative data. At Scale.
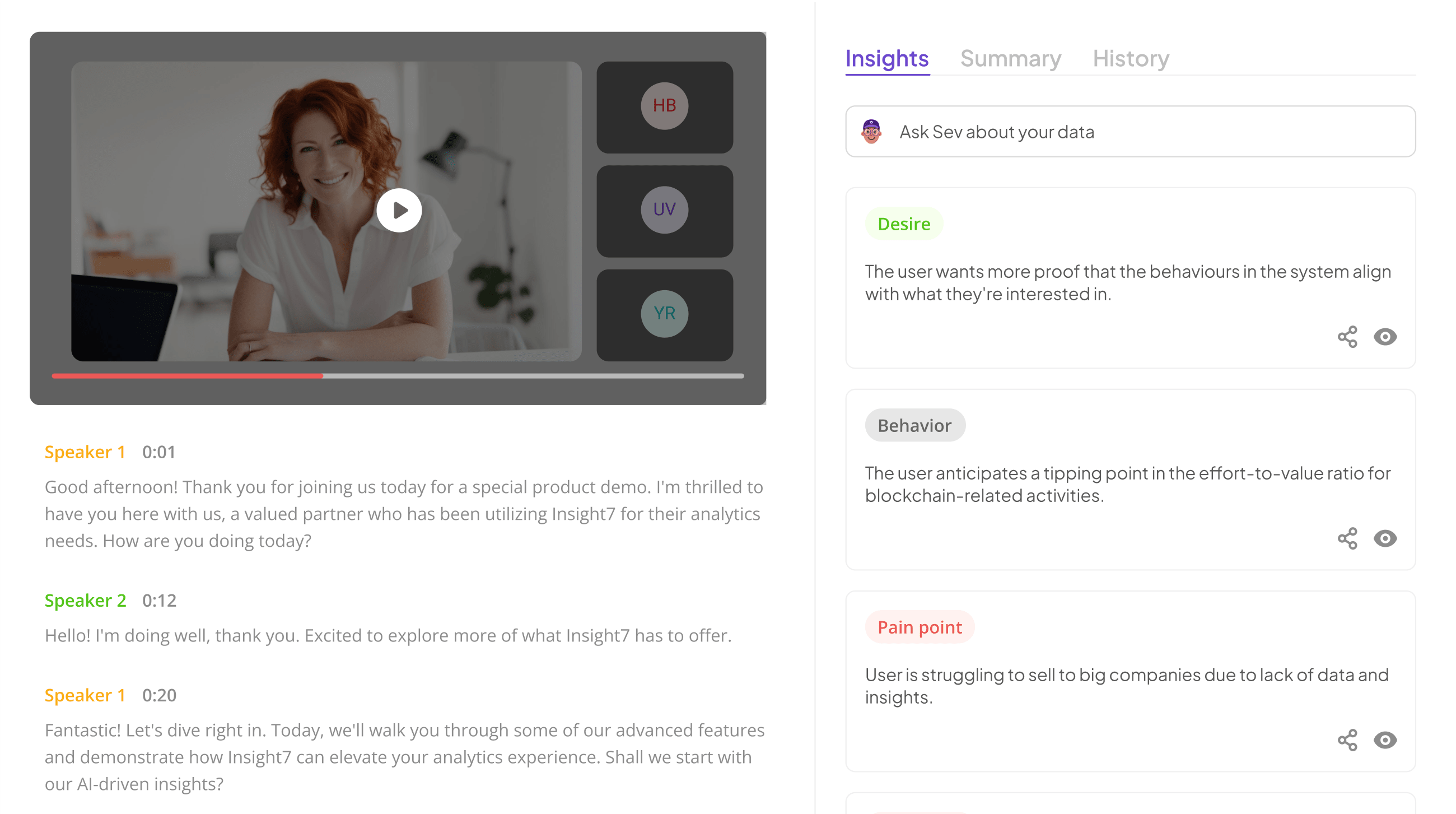
Planning Your AI QA Pilot: Initial Preparations
Planning your AI QA Pilot involves critical initial preparations that set the stage for success. Begin by identifying clear objectives and key performance metrics. This step ensures that everyone involved understands what success looks like and how it will be measured. Key metrics may include error rates, response times, and overall user satisfaction. Aligning on these facets early on will help focus your efforts and streamline decision-making throughout the pilot.
Next, assemble a cross-functional team that brings diverse perspectives to the table. Include individuals from IT, operations, and customer support to ensure that all relevant experiences shape the pilot. By collaborating with people who possess varied expertise, you can better address the unique requirements of your AI QA Pilot. Moreover, fostering open communication among team members will facilitate problem-solving and enhance the pilot’s effectiveness. Taking these steps will not only prepare you for the complexities ahead but also increase your chances for a successful deployment.
Identifying Objectives and Key Metrics
Identifying clear objectives and key metrics is essential for running an effective AI QA pilot. Establishing precise goals allows teams to direct their focus toward the most impactful areas. For instance, an objective might be to enhance response accuracy in customer interactions by a specific percentage within a defined period. When objectives are well-defined, it becomes easier to measure progress and understand the pilot's effectiveness throughout its duration.
Selecting key metrics is equally significant. Consider metrics such as the number of successful interactions, time taken to resolve issues, or improvement in user satisfaction. These data points provide a comprehensive view of how the AI-powered QA system is performing compared to the desired objectives. Regularly monitoring these metrics during the AI QA pilot ensures timely adjustments can be made, optimizing engagement and outcomes before the full deployment.
Assembling a Cross-Functional Team
Assembling a cross-functional team is crucial for the success of your AI QA pilot. A well-rounded team ensures that diverse perspectives and expertise are integrated into the project. Start by identifying members from various departments such as IT, QA, product management, and customer experience. Each team member brings unique skills, facilitating collaborative problem-solving.
Once you have your core team, establish clear roles and responsibilities. This will help maintain focus and efficiency during the pilot. Encourage open communication to foster an environment where ideas can flow freely. Regular meetings can help track progress and address challenges promptly. By assembling a cohesive cross-functional team, you lay a solid foundation for effectively executing your AI QA pilot, ultimately leading to a smoother full deployment.
Executing the AI QA Pilot: Steps for Implementation
To successfully execute your AI QA pilot, begin with a clear plan. First, select the right tools tailored to the specific needs of your AI QA pilot. Comprehensive research into available options will allow you to choose platforms that align with your business objectives. In addition to primary tools, consider any supplementary testing applications that can enhance your pilot experience.
Next, it's crucial to design and configure test cases that reflect real-world scenarios. This involves collaborating with your team to develop relevant scenarios that will generate actionable data. As the pilot progresses, carefully collect and analyze the corresponding data. This stage is vital for identifying patterns, strengths, and areas for improvement, which will ultimately guide adjustments before full deployment. Strong implementation will set a solid foundation for evaluating the AI QA pilot effectively.
Extract insights from interviews, calls, surveys and reviews for insights in minutes
Step 1: Choose the Right Tools for Your AI QA Pilot
Choosing the right tools for your AI QA pilot is essential for setting a strong foundation. Begin by evaluating your specific quality assurance needs and determining the features that are vital for your project. Consider tools that offer comprehensive data analysis capabilities and user-friendly interfaces, allowing your team to efficiently manage data without requiring extensive training. The ideal AI QA solution should easily support various evaluation criteria tailored to your organization's unique processes.
Next, assess the scalability and integration capabilities of the tools under consideration. A tool that can grow with your demands and seamlessly integrate with existing systems will enhance collaboration and reduce friction in your workflows. By selecting appropriate technology, your AI QA pilot can effectively provide insights and drive performance improvements. Remember, the right tools empower your team to focus on critical quality metrics, ultimately leading to better outcomes and smoother transitions to full deployment.
- insight7: Overview and Benefits
The AI QA Pilot offers a structured approach to evaluate the effectiveness of AI-driven quality assurance tools before full deployment. This pilot phase allows organizations to test the robustness of AI systems within existing workflows. By running a pilot, teams can identify potential challenges, assess system capabilities, and uncover user needs that might not have been addressed during initial planning.
Implementing the AI QA Pilot has several benefits. First, it fosters early identification of issues, enabling timely adjustments to improve system performance. Second, it helps teams gauge user acceptance, ensuring that the technology aligns with user expectations. Finally, the pilot enables companies to collect valuable data and insights that will inform future enhancements. Overall, conducting an AI QA Pilot lays a solid foundation for successful integration, ultimately leading to a more efficient quality assurance process in the long run.
- Other AI QA Testing Tools
When considering the deployment of AI-powered quality assurance (QA), various AI QA testing tools can enhance the pilot testing phase. These tools are fundamental in gauging the effectiveness and accuracy of your AI systems before scaling them up. Utilizing the right tools helps to identify areas that may need improvement and ensures the overall quality of the AI outputs.
Several notable tools come to mind, each offering unique capabilities. For instance, there are tools that excel at automated testing, allowing for fast and thorough evaluations of AI performance. Others specialize in natural language processing, which can be crucial when assessing conversational AI. Some tools focus on tracking user interactions, providing insights into how real-world users engage with your AI-powered solutions. Exploring these options is essential for optimizing your AI QA pilot and making informed decisions prior to full deployment.
Step 2: Design and Configure Test Cases
Creating effective test cases is crucial for the success of your AI-powered QA pilot. Start by identifying the specific goals you want to achieve with your pilot. This might involve evaluating customer interactions, compliance checks, or general usability. Once you have your objectives clear, develop detailed test cases that mirror real-world scenarios, ensuring they cover a variety of interactions. Each case should include specific criteria against which the AI will be tested, allowing for thorough evaluation.
Next, configure these test cases within your AI testing tool. This includes defining inputs, expected outcomes, and evaluation metrics. It’s important to collaborate with your cross-functional team to ensure all relevant aspects are considered. Each test case should not only assess the performance of the AI but also provide insights into user experiences and compliance standards. Establishing a solid foundation with well-designed test cases is essential for gathering meaningful data from your AI QA pilot.
Step 3: Collect and Analyze Pilot Data
Collecting and analyzing pilot data is a crucial step in executing your AI-powered QA pilot. It allows you to assess how effectively your AI tool performs in real-world scenarios. Begin by establishing a clear framework for collecting data, focusing on user feedback and performance metrics. This information will provide valuable insights into the AI’s functionality and highlight any areas needing improvement.
Once the data is collected, conduct a thorough analysis to identify patterns and trends. Look for specific metrics that align with your pilot objectives, such as accuracy rates and user satisfaction scores. It’s essential to understand not only what the data is showing but also why it matters for your overall deployment strategy. This step will inform necessary adjustments and help garner team support as you move toward full implementation.
AI QA Pilot Evaluation and Feedback
Evaluating the effectiveness of an AI QA Pilot is crucial for determining its readiness for broader application. First, a thorough review of pilot outcomes against established objectives should be conducted. This evaluation involves examining qualitative and quantitative metrics, such as call quality adherence and resolution rates. By aligning results with your initial goals, you can gauge whether the AI solution meets your requirements or needs adjustments.
Next, gathering feedback from all team members and stakeholders provides invaluable insights. Engaging those who interact with the AI QA Pilot ensures that various perspectives inform the final assessment. Their input will highlight areas for improvement, potential limitations, and unforeseen benefits. Synthesizing this feedback is essential in refining the AI-powered QA process before full deployment, ultimately contributing to a smoother transition and better end-user experiences.
Reviewing Results Against Objectives
Evaluating the AI QA Pilot entails a systematic approach to measuring outcomes against the pre-defined objectives. Start by collecting the data generated during the pilot phase, which will highlight how effectively the AI tools performed their tasks. It’s crucial to analyze this data against the objectives established before the pilot. For instance, look at how well the AI managed engagements, evaluated calls, and produced accurate assessments based on the selected criteria.
Next, synthesize your findings to create an actionable report that showcases both successful elements and areas requiring improvement. Discuss the results with your cross-functional team, focusing on what worked well and what didn’t. Regularly reviewing results against objectives not only validates the pilot's effectiveness but also ensures that your strategy aligns with overall goals. Utilizing feedback from team members and stakeholders will aid in refining your approach before full deployment, making the transition smoother and more efficient.
Seeking Team and Stakeholder Feedback
Gathering feedback from your team and stakeholders is a vital component of the AI QA pilot process. This feedback not only provides insights into the effectiveness of the pilot but also highlights areas for improvement. Engaging diverse perspectives fosters a collaborative environment where everyone feels included in the development process. As you implement the AI QA pilot, create structured opportunities for feedback, such as surveys or targeted discussions, to ensure that all voices are heard.
Encouraging open communication allows stakeholders to express their concerns and suggestions. Prioritize feedback that aligns with your objectives, focusing on how the AI QA tool meets quality assurance needs and enhances business operations. Analyze this input carefully, as it will guide necessary adjustments before full deployment. Ultimately, understanding the experiences and needs of both teams and stakeholders will help create a more robust and effective AI-powered solution.
Conclusion: Moving from AI QA Pilot to Full Deployment
Transitioning from an AI QA pilot to full deployment is a pivotal step for any organization. This phase requires a careful evaluation of the pilot results to ensure alignment with your objectives. Analyzing the data collected during the pilot can provide valuable insights into areas for improvement, helping you make informed decisions moving forward.
Moreover, gathering feedback from all stakeholders fosters a culture of collaboration and innovation. As you prepare for full deployment, ensure that the learnings from the AI QA pilot are integrated into your broader quality assurance strategy. This meticulous approach will set the foundation for success in leveraging AI technology across your operations effectively.