How do AI platforms detect real-time escalation signals?
-
Bella Williams
- 10 min read
Escalation Detection Algorithms serve as vital components in modern AI platforms, enabling them to discern potential crises in real time. These algorithms continuously analyze vast streams of data, aiming to identify patterns and anomalies that signify escalating issues, be it in customer service settings or security monitoring.
By employing sophisticated methods, they help organizations respond swiftly to emerging challenges. Understanding how these algorithms function can enhance their effectiveness, ultimately leading to more proactive and informed decision-making strategies. In essence, mastering these algorithms equips users with the tools needed to navigate complex landscapes efficiently.
Extract insights from Customer & Employee Interviews. At Scale.
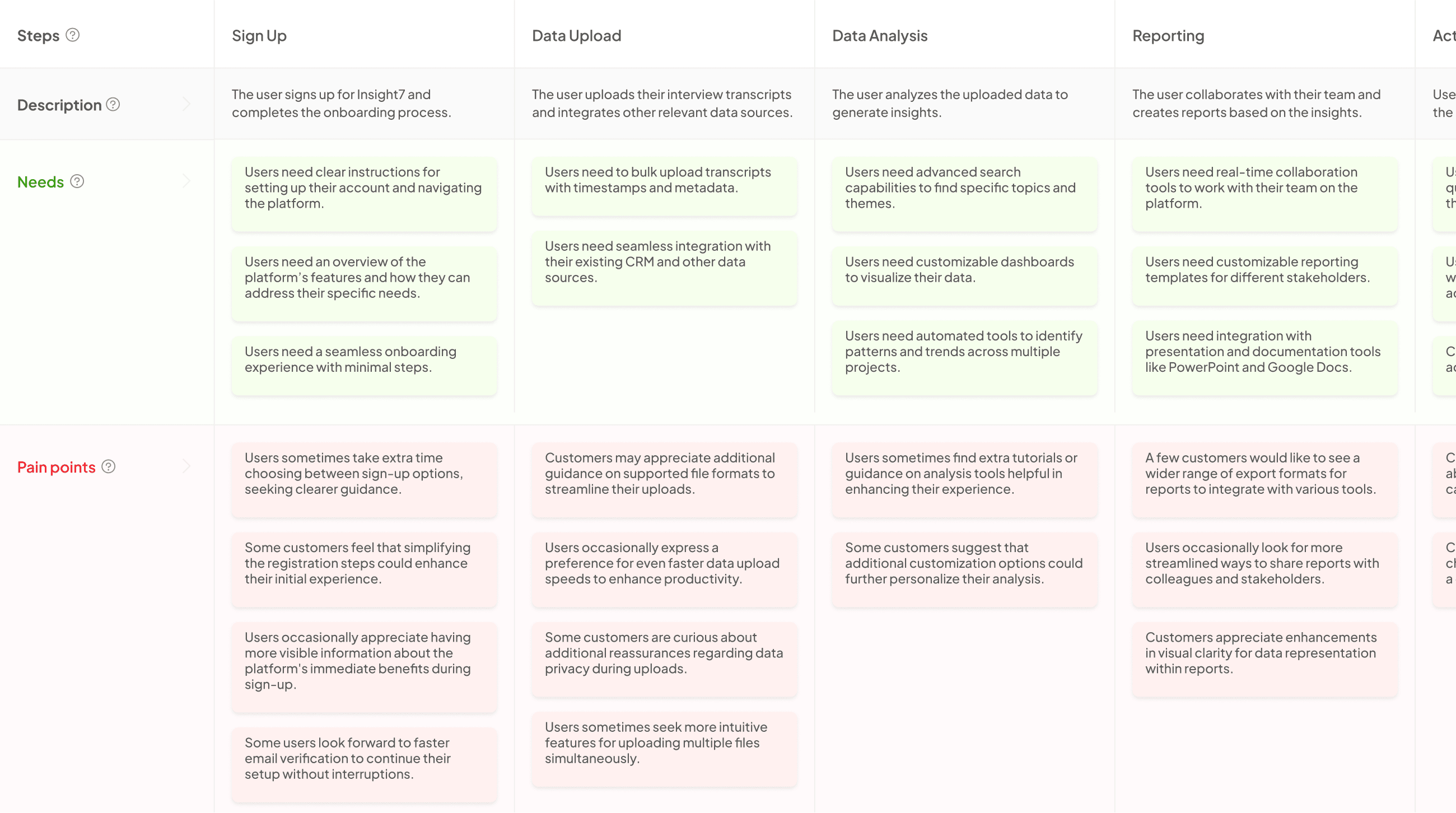
Understanding Escalation Detection Algorithms in AI Platforms
Escalation Detection Algorithms are essential tools in AI platforms, designed to identify signals that may indicate rising issues or potential crises. These algorithms analyze conversations and interactions in real-time, seeking patterns and anomalies that deviate from the norm. For instance, they may recognize sudden changes in sentiment or tone that suggest a customer’s frustration is escalating.
To enhance effectiveness, an array of techniques can be employed. First, recognizing anomalies within data allows the algorithms to flag unusual behaviors or phrases. Second, classifying these signals based on threat levels helps prioritize responses. By employing machine learning models and real-time monitoring systems, AI platforms can continuously adapt and refine their detection capabilities. This proactive approach ensures that potential escalations are managed promptly, minimizing negative impacts and improving overall customer satisfaction. Understanding these algorithms empowers organizations to maintain a responsive environment.
The Basics of Escalation Detection
Escalation detection algorithms serve as the backbone of real-time monitoring systems, identifying critical shifts in data that may indicate underlying issues. By continuously analyzing interactions, these algorithms help in recognizing unexpected behaviors or signals that could escalate into significant problems. This process begins with gathering vast amounts of data across multiple channels to create a baseline of normal operational behavior, laying the groundwork for effective detection.
The effectiveness of escalation detection lies in algorithmic precision. It focuses on flagging anomalies that diverge from established patterns, such as sudden changes in customer sentiment or abnormal transaction spikes. Once these signals are identified, the algorithms classify them by severity, providing organizations with timely insights that enable rapid response. This proactive approach helps mitigate risks before they escalate further, ensuring a more stable operational environment. Understanding these foundational elements is essential for leveraging AI platforms effectively.
Common Signals and Patterns in Escalation Detection
Escalation Detection Algorithms utilize various signals and patterns to effectively identify potential threats in real-time. Understanding these common indicators is crucial for organizations aiming to enhance their responsiveness. One primary signal is the recognition of unusual patterns in customer interactions. This may involve changes in tone, language complexity, or frequency of communication, serving as precursors to possible escalation events.
Another significant pattern lies in the correlation between customer behavior and specific triggers. For instance, abrupt increases in complaint frequency or negative feedback can suggest underlying issues, prompting the need for immediate attention. By honing in on these signals, AI platforms can help businesses mitigate risks effectively. Ultimately, understanding these signals not only ensures timely escalation detection but also guides teams in developing proactive communication strategies. This approach fosters a more robust customer experience and supports overall business growth.
[optional step-by-step guide might involve]- Step 1: Recognizing anomalies within data
Recognizing anomalies within data forms the foundation of effective escalation detection algorithms. Anomalies can be defined as patterns or data points that deviate significantly from the norm. To identify these anomalies, AI platforms analyze large datasets in real time, allowing for swift detection of unusual activity. This process often begins with the establishment of a baseline, where normal behavior and trends are defined, enabling the system to spot deviations quickly.
As anomalies are detected, they can be classified by severity and context, guiding further actions. For instance, a sudden spike in negative customer feedback might indicate an emerging crisis or product issue. Therefore, understanding the context surrounding these anomalies is crucial for appropriate response mechanisms. By leveraging accurate data analysis, organizations can not only react swiftly but also take proactive measures to prevent potential escalation.
- Step 2: Classifying signals based on threat levels
Classifying signals is a critical step that follows the identification of potential escalation indicators. In this process, different signals are evaluated and categorized based on their threat levels. AI platforms employ advanced algorithms to analyze these signals, assessing various factors including urgency, historical patterns, and context. By quantifying these aspects, the algorithms can effectively filter out benign activities from those requiring immediate attention.
The classification typically involves determining which signals signify minimal risk and which indicate a severe threat that necessitates further investigation. By establishing a structured hierarchy of threats, organizations can prioritize their response efforts appropriately. For instance, a mild warning might trigger routine monitoring, while a high-risk alert could mobilize an immediate action plan. This precision in classification ensures that resources are optimally deployed, enhancing the system’s responsiveness to genuine threats.
[optional tools]Techniques and Tools for Escalation Detection Algorithms
In the realm of escalation detection algorithms, innovative techniques and tools are essential for effective monitoring and analysis. By employing machine learning models, organizations can identify and interpret signals that indicate potential escalations. These models utilize historical data to recognize patterns, enabling systems to differentiate between normal interactions and those that may signify risks. Real-time monitoring is another critical technique, allowing for immediate feedback and timely responses to emerging threats.
To implement these algorithms successfully, various tools are available. First, predictive analytics tools can forecast escalation risks based on customer interactions. Next, sentiment analysis tools assess text and voice communications to understand emotional states. Additionally, workflow automation can streamline processes, ensuring that critical alerts are prioritized for quick action. By combining these methods, AI platforms enhance their ability to detect escalation signals effectively, thus facilitating informed decision-making and proactive management.
- Step 1: Utilizing machine learning models
Machine learning models play a pivotal role in the effective detection of escalation signals in AI platforms. By employing advanced algorithms, these models can sift through vast datasets to recognize patterns and anomalies that might indicate an impending escalation. This process involves training the models on historical data, allowing them to learn from past incidents and refine their predictive capabilities. Over time, the models become adept at distinguishing between normal fluctuations and genuine escalation signals, crucial for timely interventions.
Additionally, the integration of real-time data feeds significantly enhances the performance of these models. AI platforms can analyze ongoing interactions and customer sentiments as they are generated, improving the accuracy of escalation detection. By utilizing techniques like natural language processing and sentiment analysis, machine learning models ensure that organizations can respond promptly to customer concerns, thereby mitigating potential issues before they escalate. Thus, leveraging escalation detection algorithms effectively transforms how businesses manage crises and maintain customer relations.
- Step 2: Implementing real-time monitoring systems
Implementing real-time monitoring systems involves several crucial steps that effectively enhance the capabilities of escalation detection algorithms. To begin, it's essential to establish a robust framework that captures data from various sources in real time. This data should include both structured inputs, such as transaction records, and unstructured inputs like customer feedback. By utilizing escalation detection algorithms, organizations can identify patterns and anomalies that signal potential issues before they escalate.
Additionally, integrating advanced analytics allows teams to classify these escalation signals according to urgency and impact. Regularly analyzing this data ensures continuous improvement in detection rates, allowing organizations to respond swiftly and effectively. Furthermore, involving end-users in the design process can optimize how data is presented, making insights more actionable. As systems evolve, automating report generation can provide timely updates, ultimately transforming the approach to issue management and enhancing overall responsiveness.
(Optional Tools Section) Top Tools for Real-Time Escalation Detection
Effective escalation detection relies on specific tools designed to identify signals in real-time. Various options are available that employ sophisticated algorithms to analyze customer interactions rapidly. These tools transform vast amounts of data into actionable insights, allowing organizations to respond promptly to potential issues.
💬 Questions about How do AI platforms detect real-time escalation signals??
Our team typically responds within minutes
For optimal performance in real-time escalation detection, consider these key tools:
Natural Language Processing (NLP) Platforms: Such tools analyze textual data from customer communications, effectively identifying sentiment and urgency in requests.
Machine Learning Frameworks: These frameworks continuously improve through data input, optimizing the accuracy of escalation detection algorithms over time.
Real-Time Analytics Software: This software monitors customer interactions live, enabling immediate detection of significant changes in behavior or sentiment.
Automated Notification Systems: They alert relevant teams about escalations, ensuring timely and coordinated responses.
Leveraging these tools enhances your organization's ability to stay proactive, fostering improved customer relations through prompt action.
insight7
Effective escalation detection relies on advanced algorithms that can process vast amounts of data in real-time. These algorithms continuously monitor various customer interactions, identifying patterns and signals indicative of escalating issues. By analyzing customer conversations and behavioral data, AI platforms can accurately detect when a situation requires immediate attention, enhancing responsiveness and mitigating potential problems.
Key factors contributing to the success of escalation detection algorithms include anomaly recognition, signal classification, and context analysis. First, recognizing anomalies within the data helps pinpoint deviations from normal behavior. Second, classifying signals based on their urgency allows for prioritization and focused responses. Lastly, understanding the context of customer interactions can further inform the nature of the escalation, equipping teams with the insights needed to address issues proactively. By employing these techniques, organizations can improve customer satisfaction and operational efficiency, ultimately leading to better business outcomes.
Tool 2
Escalation Detection Algorithms play a vital role in identifying critical changes and anomalies within data streams. These algorithms leverage advanced machine learning techniques to classify escalations based on specific patterns and signals. By continuously analyzing incoming data, they can detect real-time escalation signals that indicate potential issues needing immediate attention.
One effective strategy involves recognizing anomalies within the data. Algorithms can be trained to identify deviations from established norms, flagging these as potential escalation signals. Following this, it is crucial to classify these signals based on their severity. Implementing a tiered classification system allows teams to prioritize responses effectively, ensuring that urgent matters receive the necessary focus and resources. With these tools and techniques in place, organizations can enhance their ability to respond promptly and efficiently to emerging challenges. This proactive approach not only mitigates risks but also fosters a culture of continuous improvement and operational resilience.
Tool 3
In the realm of escalation detection algorithms, Tool 3 serves as a pivotal component in real-time monitoring systems. This tool utilizes sophisticated patterns to identify potential threats by analyzing significant variables in incoming data. The algorithms assess various signals that may indicate heightened risk or escalation, which is crucial for timely interventions. Such proactive measures ensure that potential issues are addressed before they escalate into serious problems.
Moreover, Tool 3 integrates seamlessly with existing frameworks, allowing organizations to adapt quickly to changing conditions. It emphasizes the importance of machine learning techniques, enhancing the accuracy of predictions and reducing false positives. By continuously learning from new inputs, this tool refines its detection capabilities. Users can confidently rely on this technology to streamline their response strategies, facilitating a more effective approach to real-time escalation detection. Understanding and implementing these algorithms significantly contributes to improved decision-making processes and overall operational efficiency.
Tool 4
When exploring Tool 4 within the context of escalation detection algorithms, it is essential to understand the underlying mechanics. Escalation Detection Algorithms are designed to capture and analyze behavioral patterns indicative of potential issues. These tools implement sophisticated data analysis techniques to pinpoint irregularities and early signals that may lead to escalation.
The process typically involves several key components. First, these algorithms monitor various data streams in real time, identifying anomalies related to user behavior, communication patterns, or operational issues. Next, they classify these signals based on urgency and potential impact, allowing organizations to respond effectively. This systematic approach ensures that potential risks are addressed promptly, keeping operations smooth and reducing the likelihood of significant disruptions. Investing in robust escalation detection capabilities enhances an organization’s readiness to tackle emerging challenges efficiently.
Tool 5
Escalation Detection Algorithms play a crucial role in identifying potential issues before they escalate. When integrated into AI platforms, these algorithms continuously monitor interactions and data flows to pinpoint anomalies. The process begins with recognizing irregular patterns, which serve as red flags for potential escalation. Systems utilize advanced techniques to analyze vast amounts of data, making it possible to act swiftly in response to any indication of rising risks.
To effectively implement escalation detection, certain key steps are vital. First, machine learning models are employed to learn from historical data, enabling the identification of trends and anomalies that signal possible escalations. Next, real-time monitoring systems are established to ensure that alerts are instantaneous, allowing teams to respond proactively rather than reactively. This dual approach helps organizations stay ahead of potential crises, securing both their reputation and operational integrity.
Generate Journey maps, Mind maps, Bar charts and more from your data in Minutes
Conclusion: Mastering Escalation Detection Algorithms for Real-Time Response
Mastering escalation detection algorithms is crucial for organizations striving for prompt and effective responses to potential issues. These algorithms identify signals that indicate when situations may arise, allowing timely action before challenges escalate. By utilizing machine learning and real-time monitoring systems, businesses can effectively analyze vast amounts of data, identifying anomalies and patterns that may not be immediately apparent.
In summary, a proficient understanding of escalation detection algorithms supports organizations in staying ahead of potential crises. This proactive approach enables teams to develop strategies based on actionable insights, ultimately fostering improved decision-making and customer satisfaction. Being prepared to respond effectively can significantly enhance an organization's resilience in a fast-paced environment.
💬 Questions about How do AI platforms detect real-time escalation signals??
Our team typically responds within minutes