The rise of artificial intelligence in quality assurance has reshaped how we address challenges in various industries. AI QA superiority surfaces as a pivotal theme, illustrating how sophisticated algorithms can effectively analyze vast amounts of data without the constraints of human error. This transition drives efficiency, consistency, and productivity, marking a transformational moment in QA practices, especially in software testing and customer support.
In the following sections, we will delve into seven compelling real-world use cases that highlight AI's advantages over traditional human approaches. From significantly enhancing bug detection accuracy to streamlining customer interactions, these examples will provide insight into the effectiveness of AI-driven quality assurance. By understanding these applications, businesses can harness AI's potential to improve their QA processes and deliver exceptional results.
Analyze qualitative data. At Scale.
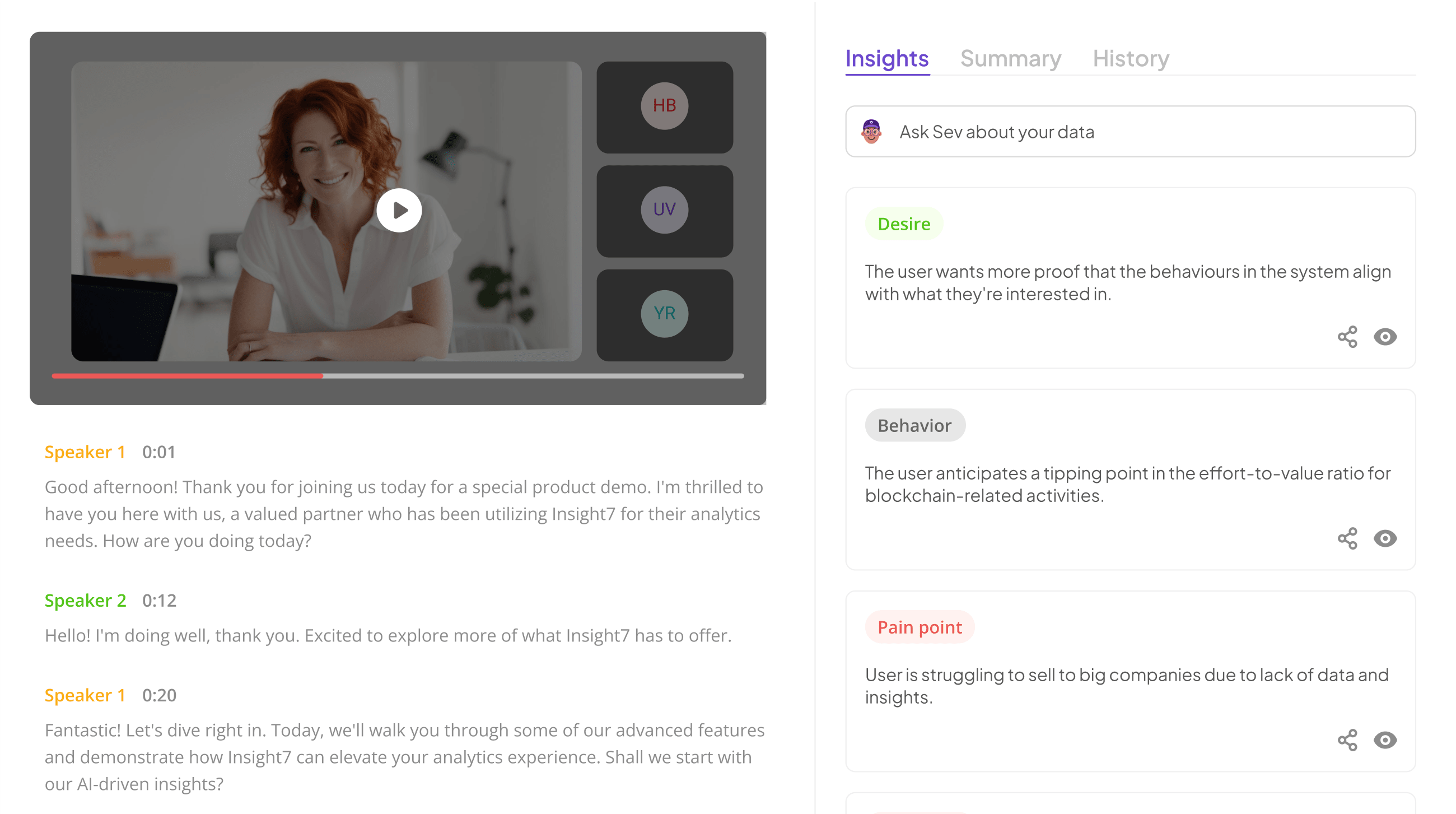
AI QA Superiority In Software Testing
AI QA Superiority in Software Testing ensures enhanced accuracy and efficiency in identifying software bugs. Traditional testing methods often rely heavily on human intuition, which can lead to missed errors. In contrast, AI can analyze vast amounts of code and test scenarios much faster, minimizing the risk of human error. Its ability to learn from previous test outcomes allows AI to continuously improve detection rates, making it a vital asset in the software development lifecycle.
Moreover, AI simplifies regression testing by automatically running comparative tests on new software versions against established standards. This drastically reduces testing time and costs, enabling teams to focus on more strategic tasks. By implementing AI in QA processes, organizations experience improved product quality and reduced time to market, highlighting the undeniable AI QA superiority in software testing. Ultimately, the integration of AI not only enhances quality assurance but also drives innovation in software development.
Enhancing Bug Detection Accuracy
AI QA Superiority significantly enhances bug detection accuracy, which is critical for effective software quality assurance. This advancement transforms how QA teams identify and rectify issues during the development process. Traditional bug detection methods often rely on human testers, who may overlook subtle errors due to cognitive biases or fatigue. In contrast, AI algorithms apply meticulous precision, analyzing vast datasets to uncover bugs that human testers might miss.
AI-driven tools utilize machine learning techniques to continuously improve their detection capabilities. By recognizing patterns in historical data, these tools can predict where bugs are likely to occur, enabling proactive resolutions. Moreover, the speed at which AI can process information drastically reduces the time to identify and fix bugs. Consequently, organizations can achieve higher software quality, resulting in better user experiences and decreased costs associated with post-launch fixes. This transformation illustrates how embracing AI QA Superiority will reshape the future of quality assurance procedures.
Reducing Testing Time and Costs
In the realm of software testing, reducing testing time and costs emerges as a vital concern for organizations. With the sheer volume of applications requiring thorough QA processes, the need for efficiency has never been more pressing. AI QA superiority excels in automating repetitive tasks, significantly cutting down the time needed for quality assurance. By utilizing machine learning algorithms, AI systems can quickly analyze vast datasets, identifying bugs and anomalies faster than human testers. This rapid analysis not only accelerates the overall testing process but also helps in allocating resources more effectively.
Moreover, the cost-effectiveness of implementing AI in QA is evident. Human testers can be expensive and limited in the number of tests they can conduct in a given timeframe. In contrast, AI tools can perform hundreds of tests simultaneously at a fraction of the cost. This shift toward automation allows companies to reallocate their budget towards innovation and development, ultimately improving product quality and user satisfaction. As AI continues to dominate the QA landscape, its advantages in time and cost savings become increasingly undeniable.
Extract insights from interviews, calls, surveys and reviews for insights in minutes
Transforming Customer Support: AI QA Superiority
In the pursuit of transforming customer support, AI QA superiority stands out as a game-changer. Traditional methods of handling customer inquiries often fall short, leading to inefficiencies and slower response times. However, by integrating AI-driven solutions like advanced chatbots, businesses can significantly enhance their support operations. These chatbots are designed to efficiently manage and respond to inquiries, thus ensuring quicker resolution times and improved customer satisfaction.
Additionally, AI-powered sentiment analysis plays a crucial role in maintaining quality assurance within customer support. By accurately interpreting customer emotions and feedback, organizations can pinpoint areas for improvement and make informed decisions. This capability allows for a proactive approach to customer service, rather than a reactive response. Overall, embracing AI QA solutions not only streamlines processes but also fosters a more engaging and responsive customer support experience.
Chatbot Efficiency in Handling Inquiries
Chatbots have revolutionized the way inquiries are handled, demonstrating clear AI QA superiority in various scenarios. These intelligent systems can respond to customer queries instantly, eliminating long wait times that often frustrate users. By analyzing extensive data sets, chatbots deliver accurate responses tailored to individual needs, significantly enhancing user experience. They operate 24/7, ensuring that customers receive assistance whenever they require it.
Moreover, chatbots excel at managing multiple interactions simultaneously, streamlining service delivery and boosting efficiency. This capacity allows businesses to focus on more complex inquiries that require human intervention. As organizations increasingly adopt chatbot technology, they find that these systems not only improve response times but also enhance overall customer satisfaction. The implementation of chatbots offers a strategic advantage, positioning businesses to respond swiftly to customer demands while maintaining high-quality service.
AI-Driven Sentiment Analysis for Quality Assurance
AI-Driven Sentiment Analysis for Quality Assurance enhances the evaluation process by accurately gauging customer emotions and feedback. This allows organizations to understand user sentiment regarding products and services in real-time. While human analysis can be subjective and time-consuming, AI systems process vast amounts of feedback swiftly, delivering insights that are not easily attainable through manual methods.
Additionally, AI-driven sentiment analysis identifies patterns and trends that may go unnoticed by human reviewers. These insights can markedly improve quality assurance practices by pinpointing areas for improvement. By integrating AI's capabilities in sentiment analysis, organizations can create targeted strategies to enhance customer satisfaction and product quality, showcasing the AI QA superiority in transforming traditional quality assurance frameworks. Ultimately, this leads to greater efficiency in responding to customer needs and increasing overall service quality.
Advanced Data Analytics: Harnessing AI QA Superiority
Advanced data analytics plays a pivotal role in realizing AI QA superiority. Through predictive analytics, systems can anticipate potential quality issues before they arise, allowing for timely interventions. This foresight reduces the risk of defects, ensuring a smoother development process and higher-quality outputs. Moreover, the ability to analyze vast datasets enables organizations to identify patterns that human teams might overlook.
Automated data validation further enhances quality assurance efforts. AI systems can efficiently check for errors across numerous data entries, significantly minimizing the time and effort required. Unlike human inspection, which can be prone to fatigue and biases, AI algorithms consistently deliver high accuracy, ensuring that quality benchmarks are consistently met. By harnessing these advanced analytics techniques, organizations can significantly improve their QA processes, solidifying their competitive edge in an increasingly data-driven landscape.
Predictive Analytics in QA Processes
Predictive analytics plays a vital role in enhancing quality assurance (QA) processes. By utilizing historical data, AI can forecast potential defects or issues before they arise, allowing teams to proactively address concerns. This approach not only minimizes errors but significantly improves the efficiency of the QA process by anticipating problem areas.
AIโs capability to analyze vast datasets empowers organizations to identify patterns and trends that would be difficult for human analysts to discern. This predictive measure ensures that teams can focus on high-risk areas, leading to more effective testing protocols and resource allocation. Moreover, when combined with automated data validation techniques, the superiority of AI in QA processes becomes even more evident. By adopting these methodologies, businesses consistently achieve higher quality standards and customer satisfaction.
Automated Data Validation and Error Detection
Automated data validation and error detection significantly enhance quality assurance processes, showcasing AI QA superiority in todayโs digital landscape. Traditional methods often rely on human judgment, which can be slow and prone to oversight. In contrast, automated systems efficiently check data against pre-defined rules, identifying discrepancies far quicker than a human can.
This automation can operate continuously, scanning incoming data streams for validation and errors in real-time. The application of advanced algorithms enables identification of patterns and anomalies that might elude manual inspection. Additionally, the scalability of AI systems allows them to handle vast amounts of data simultaneously, ensuring consistency and accuracy levels that human experts struggle to match. As organizations look to improve their quality assurance frameworks, leveraging automated data validation and error detection is proving to be an essential strategy, solidifying AI's position at the forefront of QA methodologies.
Conclusion: The Future of AI QA Superiority
As we look toward the future, AI QA superiority promises to redefine the quality assurance landscape across industries. The evolving capabilities of artificial intelligence allow for unprecedented accuracy and efficiency, far surpassing human limitations. AI can rapidly analyze vast amounts of data, quickly identifying trends and issues that would take humans much longer to uncover.
Furthermore, as AI continues to advance, its integration into QA processes will lead to better resource allocation, allowing humans to focus on more complex tasks. The collaboration between human expertise and AI technology will enhance overall performance, ultimately driving innovations that elevate quality assurance to new heights. Embracing AI QA superiority not only improves outcomes but also paves the way for a more efficient and effective future in quality assurance practices.