6 Use Cases Where AI QA Outperforms Human Evaluation
-
Hello Insight
- 10 min read
In recent years, artificial intelligence (AI) has dramatically transformed various industries. One of its most compelling applications is in quality assurance (QA), where AI QA superiority takes center stage. Organizations increasingly rely on AI-driven evaluations to enhance productivity, streamline processes, and reduce human error. This shift highlights the potential of AI to provide consistent, accurate evaluations that often surpass traditional methods.
AI QA systems utilize advanced algorithms to assess compliance, analyze data, and deliver insights at unparalleled speed. This section will explore six specific use cases where AI QA not only outperforms human evaluation but also paves the way for innovative solutions. By understanding these cases, readers will grasp the vital role that AI QA superiority plays in modern evaluation and its transformative impact on various domains.
Analyze & Evaluate Calls. At Scale.
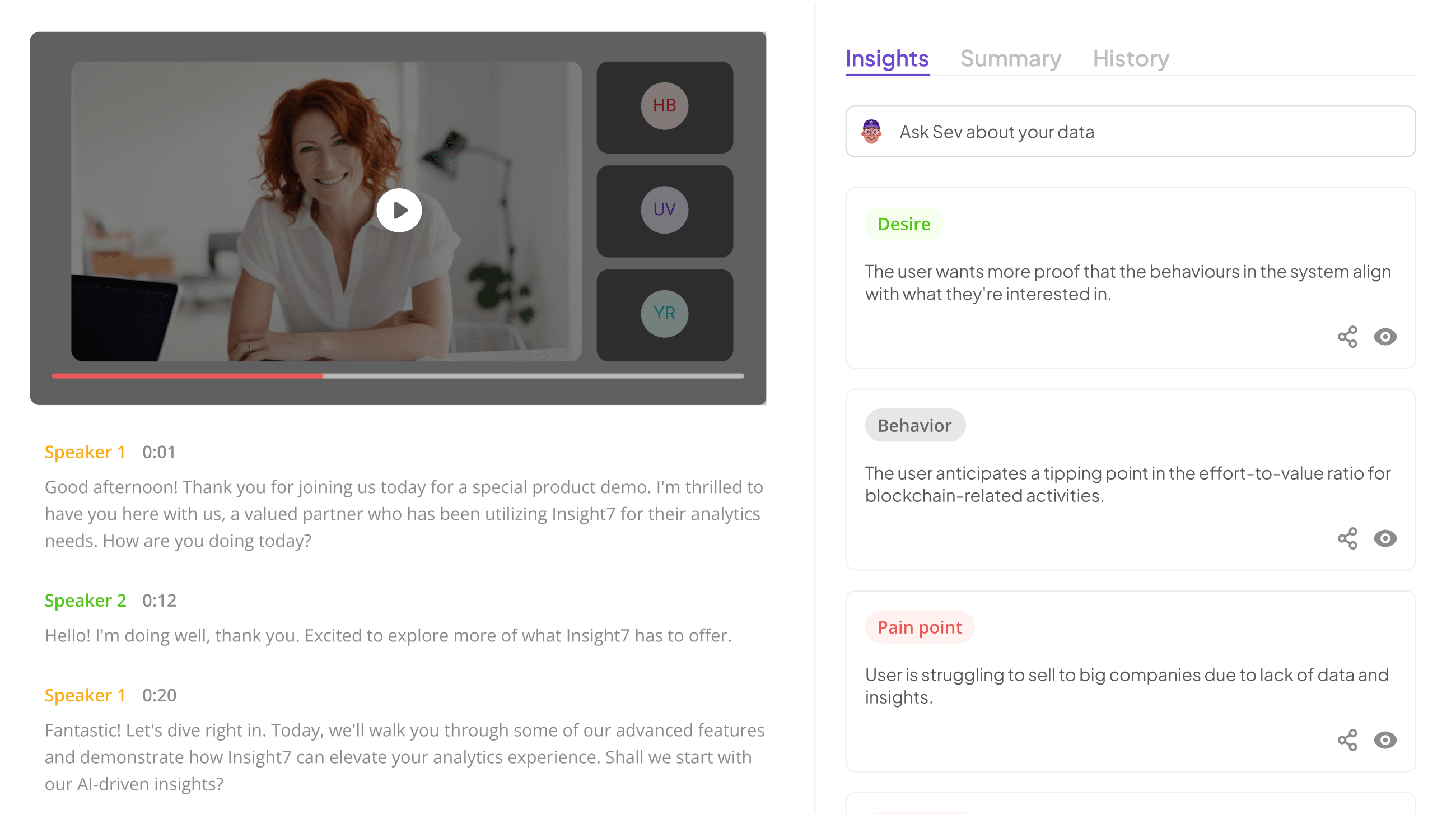
The Inherent Advantages of AI QA: Efficiency and Consistency
AI QA delivers distinct advantages in efficiency and consistency, marking its superiority over human evaluation. One major benefit is speed; AI systems can process vast amounts of data in a fraction of the time it takes for a human. This capability not only accelerates decision-making but also ensures that evaluations are delivered promptly, maintaining the momentum of projects and allowing for rapid iterations. For companies facing high volumes of data or repetitive tasks, this efficiency translates to significant time and cost savings.
Additionally, consistency is another hallmark of AI QA superiority. Unlike human evaluators, who may be influenced by subjective factors or fatigue, AI systems apply uniform criteria across all evaluations. This impartiality fosters trust in the results, making it easier to make informed decisions. As a result, organizations can leverage AI QA to standardize processes, minimize errors, and enhance overall quality in their outputs. Embracing these inherent advantages lays the foundation for a more reliable and effective quality assurance strategy.
AI QA Superiority in Speed and Timeliness
The speed and timeliness of AI QA represent significant advantages over human evaluation processes. With AI systems, quality assurance can occur in real time, instantly processing vast amounts of data and identifying issues more swiftly than any human team could manage. This rapid analysis minimizes delays in product launches, allowing organizations to remain competitive in fast-paced markets.
Moreover, AI QA systems continuously operate without the constraints of human fatigue or availability, ensuring that evaluations are consistently timely and effective. As organizations increasingly generate customer insights at scale, the ability to quickly synthesize this data into actionable strategies becomes vital. Embracing AI QA not only optimizes operational efficiency but also enhances decision-making speed, positioning businesses to outpace their competition. Ultimately, the AI QA superiority in speed and timeliness is reshaping how organizations approach quality assurance, paving the way for smarter, faster business practices.
Consistent Outcomes: Eliminating Human Bias with AI QA Superiority
AI QA Superiority hinges on its ability to provide consistent outcomes, effectively eliminating human bias from the evaluation process. Unlike human assessors who may be influenced by emotions or personal experiences, AI systems rely on data-driven methodologies. This objectivity ensures that evaluations remain consistent across various scenarios, minimizing variability and potential subjectivity.
Furthermore, AI QA excels in high-volume assessments, processing large datasets with remarkable accuracy. By leveraging predefined criteria, AI can identify discrepancies and maintain quality control better than human evaluators. This not only elevates the overall quality of evaluations but also optimizes the feedback loop, facilitating timely interventions. Ultimately, embracing AI QA Superiority fosters trust in evaluation processes, paving the way for more reliable and efficient outcomes in diverse applications.
Extract insights from interviews, calls, surveys and reviews for insights in minutes
Exploring Six Key Use Cases of AI QA Superiority
In exploring six key use cases of AI QA superiority, we delve into how artificial intelligence enhances quality assurance across various fields. The efficiency and accuracy provided by AI-driven solutions are reshaping traditional evaluation methods. For instance, in software testing, AI can significantly improve precision by building robust test cases that adapt as software evolves. This adaptability allows for quicker identification of bugs, thus accelerating the development cycle.
Another pivotal use case is in customer support, where AI chatbots provide 24/7 assistance. These systems can engage users instantly, resolving queries without the delays typical of human agents. Additionally, they gather feedback seamlessly, ensuring businesses continually improve their customer experience. By examining these distinct scenarios, we can appreciate the remarkable potential of AI QA to surpass human evaluation, offering organizations the reliability and consistency necessary for success.
Enhancing Software Testing: Precision and Scalability
Enhancing software testing through precision and scalability offers remarkable opportunities in quality assurance. AI QA superiority comes into play by building robust test cases that ensure comprehensive coverage of various scenarios. Advanced algorithms can evaluate vast amounts of past data, identifying patterns that human testers may overlook. This capability improves test case design and enhances the likelihood of uncovering elusive bugs before deployment.
Subsequently, analyzing test outcomes becomes a streamlined process with AI. It can dissect results meticulously and provide insightful feedback regarding software performance. This precision leads to quicker debugging and minimizes the risk of errors, ultimately elevating the overall quality of software products. By leveraging these AI-driven methodologies, organizations can achieve both enhanced accuracy and scalability in their testing processes, empowering teams to meet growing demands while maintaining excellence.
- Step 1: Building Robust Test Cases with AI QA
Building robust test cases is a critical first step in utilizing AI QA for effective evaluations. The process begins with a clear definition of evaluation criteria that aligns with your specific requirements. AI excels at systematically identifying these criteria, ensuring every aspect is covered without omission. This advantage in organizing test cases provides a structured approach to quality assurance, significantly enhancing the reliability of outcomes.
Once the criteria are established, AI can automate the test case generation based on intricate patterns observed in past data. This automation promotes thoroughness, as it can simulate a multitude of scenarios that human evaluators may overlook. By utilizing AI QA superiority, organizations can implement tests that are not only comprehensive but also efficient. This investment in robust test cases ultimately leads to higher quality results, empowering teams to focus on actionable insights rather than repetitive evaluation tasks.
- Step 2: Analyzing Test Outcomes for Accurate Debugging
Analyzing test outcomes is crucial for accurate debugging, enabling teams to identify and rectify issues efficiently. With AI QA superiority, this process becomes more precise and reliable. AI systems can analyze vast amounts of data quickly, isolating patterns and anomalies that may evade human evaluation. This not only reduces the time spent on inspections but also facilitates a thorough examination of each test result.
The first step in effective analysis involves categorizing outcomes into actionable segments. This allows for deeper insights into failing components or recurring problems. Following that, teams must assess whether the discrepancies are due to misunderstandings in the test criteria or inherent flaws in the software. Utilizing AI can streamline this process, as it applies sophisticated algorithms to identify root causes systematically. By leveraging AI for outcomes analysis, organizations can enhance their debugging accuracy significantly, minimizing error margins and boosting overall software quality.
Revolutionizing Customer Support: 24/7 Availability and Instant Solutions
In today's fast-paced digital environment, revolutionizing customer support hinges on delivering 24/7 availability and instant solutions. AI chatbots play a pivotal role in achieving this goal by handling customer inquiries at any hour. These intelligent systems are designed to respond to questions quickly and accurately, reducing wait times and enhancing customer satisfaction. When implemented effectively, businesses can ensure that customers receive immediate assistance, even during off-peak hours.
Additionally, AI's capabilities extend to gathering and analyzing feedback seamlessly, allowing organizations to understand customer preferences in real-time. This data-driven approach helps teams to adapt their strategies and improve service offerings incessantly. Ultimately, the AI QA superiority transforms customer interactions by increasing efficiency and responsiveness. This leads to a more engaged customer base and improved overall experiences, positioning businesses ahead of the competition.
- Step 1: Implementing AI Chatbots for Immediate Query Resolution
Implementing AI chatbots is crucial for addressing customer queries promptly. These digital assistants enhance service efficiency and improve customer satisfaction. With AI QA superiority, chatbots provide tailored responses, ensuring unique challenges are met swiftly. By processing inquiries instantly, they alleviate pressure from human agents, allowing for a more consultative approach where necessary.
There are three key strategies for successfully implementing AI chatbots for immediate query resolution. First, define specific goals for what the chatbot should achieve. Having clear objectives enables more focused design and functionality. Second, invest in robust training data to ensure the chatbot understands various user intents. Finally, continuously monitor performance metrics to refine interactions based on user feedback. This structured approach leads to a superior user experience, illustrating AI's potential to outperform traditional methods in query resolution.
- Step 2: Using AI for Seamless Customer Experience Feedback
AI QA Superiority in collecting and analyzing customer experience feedback provides a robust framework to enhance service quality. By employing AI, organizations can capture a wide array of customer sentiments across various channels in real time. This ability to process and funnel information makes it simpler to identify trends and sentiments that might influence product or service adjustments.
Utilizing AI in this domain involves a few key approaches. First, harnessing machine learning algorithms allows for the immediate interpretation of vast amounts of feedback data, pinpointing both positive and negative customer experiences. Next, AI-driven sentiment analysis can categorize feedback effectively, enabling businesses to respond proactively. Lastly, continuous learning capabilities of AI systems mean that the feedback mechanism becomes ever more refined over time. This holistic approach not only provides valuable insights but also ensures a more seamless experience for customers, emphasizing the significant advantages of AI QA superiority in modern customer feedback systems.
Complementary Tools for AI-Powered Quality Assurance
Complementary tools play a crucial role in enhancing AI-powered quality assurance by providing additional functionalities that support AI QA superiority. Various innovative platforms and applications integrate seamlessly with AI systems to strengthen quality assessment processes. These tools can augment analysis, automate repetitive tasks, and generate insightful reports, facilitating a more comprehensive evaluation framework.
Leading tools such as insight7 and Test.ai streamline the testing process by offering robust automation solutions, ensuring faster and more accurate results. Visual testing platforms like Applitools help in identifying visual inconsistencies, while Functionize enables intelligent automation tailored for web and mobile applications. Lastly, mabl supports agile teams with continuous testing capabilities, fostering an environment of rapid development and deployment. Together, these complementary tools empower teams to leverage AI QA superiority effectively, making quality assurance more efficient and reliable.
Top Tools to Elevate Your AI QA Strategy
To elevate your AI QA strategy, consider integrating cutting-edge tools that enhance evaluation processes. These technologies streamline workflows while ensuring that high standards of accuracy and efficiency are met. The right tools can significantly enhance your QA framework, allowing you to harness the AI QA superiority that emerges from automation and data-driven insights.
Some top tools to consider include Test.ai for robust automated testing that scales easily and Applitools, which focuses on advancing visual testing capabilities to improve user interfaces. Additionally, Functionize can facilitate intelligent automation for web and mobile applications. By incorporating these tools, you create a QA environment that not only meets but exceeds traditional evaluation benchmarks, showcasing the strength of AI in quality assurance practices. Embracing this technology enables organizations to improve their products continuously, accelerating innovation and enhancing customer satisfaction.
insight7: Leading the AI QA Transformation
As organizations embrace AI QA superiority, a transformative shift is occurring within quality assurance practices. The integration of artificial intelligence enhances the speed, consistency, and accuracy of evaluations beyond what human evaluators can achieve. With AI-driven tools, companies can analyze vast amounts of data and customer interactions in real time. This approach streamlines the evaluation process, allowing for immediate feedback and actionable insights, ultimately fostering more informed decision-making.
To lead this AI QA transformation, organizations must focus on three key areas. First, investing in advanced AI technologies ensures precise and scalable solutions tailored to their specific needs. Second, training teams to leverage these innovations effectively fosters a culture of continuous improvement. Lastly, aligning AI capabilities with overarching business strategies reinforces the importance of customer-centric evaluation processes. By embracing AI QA superiority, organizations position themselves to respond swiftly to market changes and enhance their competitive edge.
Test.ai: Innovating Automated Testing
Innovative automated testing solutions have transformed quality assurance in the tech industry. These advancements leverage AI capabilities, optimizing both accuracy and speed. The integration of AI in testing processes has demonstrated AI QA superiority over human evaluation by eliminating biases and streamlining workflows. As a result, companies can ensure higher software reliability with fewer resources.
One significant aspect of AI-driven testing is its ability to autonomously generate intricate test cases based on specific requirements. This adaptability allows rapid testing across various environments and scenarios, providing results far quicker than conventional methods. Additionally, the vast data analysis performed by AI can uncover patterns, leading to more effective debugging processes. In environments where efficiency is crucial, automated testing models consistently outperform human efforts, highlighting the potential for AI to redefine the future of quality assurance.
Applitools: Advancing Visual Testing Capabilities
Applitools has become a pivotal player in enhancing visual testing capabilities, driven by the advantages that AI QA superiority offers. Traditional visual testing often falls victim to human error, which can compromise the accuracy of results. In contrast, AI-driven visual assessments ensure high consistency in evaluating user interfaces across different devices and environments. By automating visual testing, teams can swiftly identify discrepancies, enabling them to deliver flawless user experiences.
The technology employs advanced algorithms to analyze visual changes, making it possible to detect even the smallest differences that a human tester might overlook. This not only accelerates the testing process but also enhances the quality of software products. Furthermore, integrating AI into visual testing can streamline workflows, ultimately allowing teams to focus on strategic decision-making rather than repetitive tasks. Thus, as organizations seek to evolve their quality assurance practices, embracing these advancements is essential for achieving superior testing outcomes.
Functionize: Intelligent Automation for Web and Mobile Applications
Intelligent automation for web and mobile applications represents a significant evolution in quality assurance. This technology streamlines testing processes, offering both precision and scalability that human evaluation often struggles to achieve. Using advanced AI algorithms, businesses can optimize their workflows and reduce error rates considerably. This not only speeds up the launch of applications but also enhances user satisfaction by minimizing inconsistencies.
The role of intelligent automation becomes particularly crucial in high-traffic scenarios where user expectations are at their peak. Automated systems efficiently analyze user interactions, identify friction points, and provide actionable insights. As a result, organizations can focus on innovation and improvement rather than getting bogged down by routine testing tasks. This illustrates the undeniable AI QA superiority in ensuring that both web and mobile applications deliver a seamless user experience.
mabl: Continuous Testing for Agile Teams
In agile environments, continuous testing is pivotal for maintaining software quality while adapting to rapid development cycles. mabl empowers agile teams by offering a solution that integrates AI-driven testing capabilities into their workflows. This AI QA superiority enables teams to automate test creation and execution, significantly reducing the time and resources typically required for quality assurance. By providing real-time insights into application performance, teams can easily identify and address issues as they arise.
Additionally, mabl's adaptability allows for ongoing testing across various stages of development. This continuous testing framework supports iterative improvements, giving teams the flexibility to make changes without sacrificing quality. Moreover, the intelligent analysis provided by AI helps in making data-driven decisions, ensuring that tests align with user expectations. Consequently, teams can confidently deliver high-quality software that meets the demands of their users and stakeholders. With mabl, agile teams thrive through enhanced efficiency and consistent outcomes in their testing endeavors.
Conclusion: Embracing AI QA Superiority for Future Innovations
As we conclude our exploration of AI QA superiority, it becomes clear that integrating AI into quality assurance processes is not merely an option but a necessity for future innovation. Embracing AI-powered quality assurance streamlines operations, enhances accuracy, and significantly reduces the time required to deliver results. Organizations can leverage these benefits to remain competitive and responsive in a fast-paced market.
Furthermore, the distinct advantages of AI QA, such as unbiased evaluations and consistent outcomes, highlight its superiority over traditional methods. By prioritizing AI in quality assurance, companies position themselves to harness new technologies and continually improve their products and services. This forward-thinking approach will ultimately pave the way for groundbreaking advancements and sustained success.