In today’s fast-paced digital environment, the need for precise quality assurance (QA) metrics has never been more critical. Traditional methods of manual monitoring can often fall short, leading to inaccuracies and slower responses to quality issues. This is where AI-driven QA metrics step in, transforming the landscape by providing unparalleled insights through advanced data analytics. By incorporating AI technology, businesses can enhance their understanding of performance and user experience, leading to data-backed decision-making.
AI-driven QA metrics not only streamline the monitoring process but also offer deeper, real-time insights that manual methods cannot match. For organizations striving for excellence in their software development, adopting these innovative metrics can significantly reduce errors and improve overall quality. As we delve into the six key metrics captured more effectively by AI, we will uncover the benefits that drive the shift from manual approaches to AI-powered solutions.
Analyze qualitative data. At Scale.
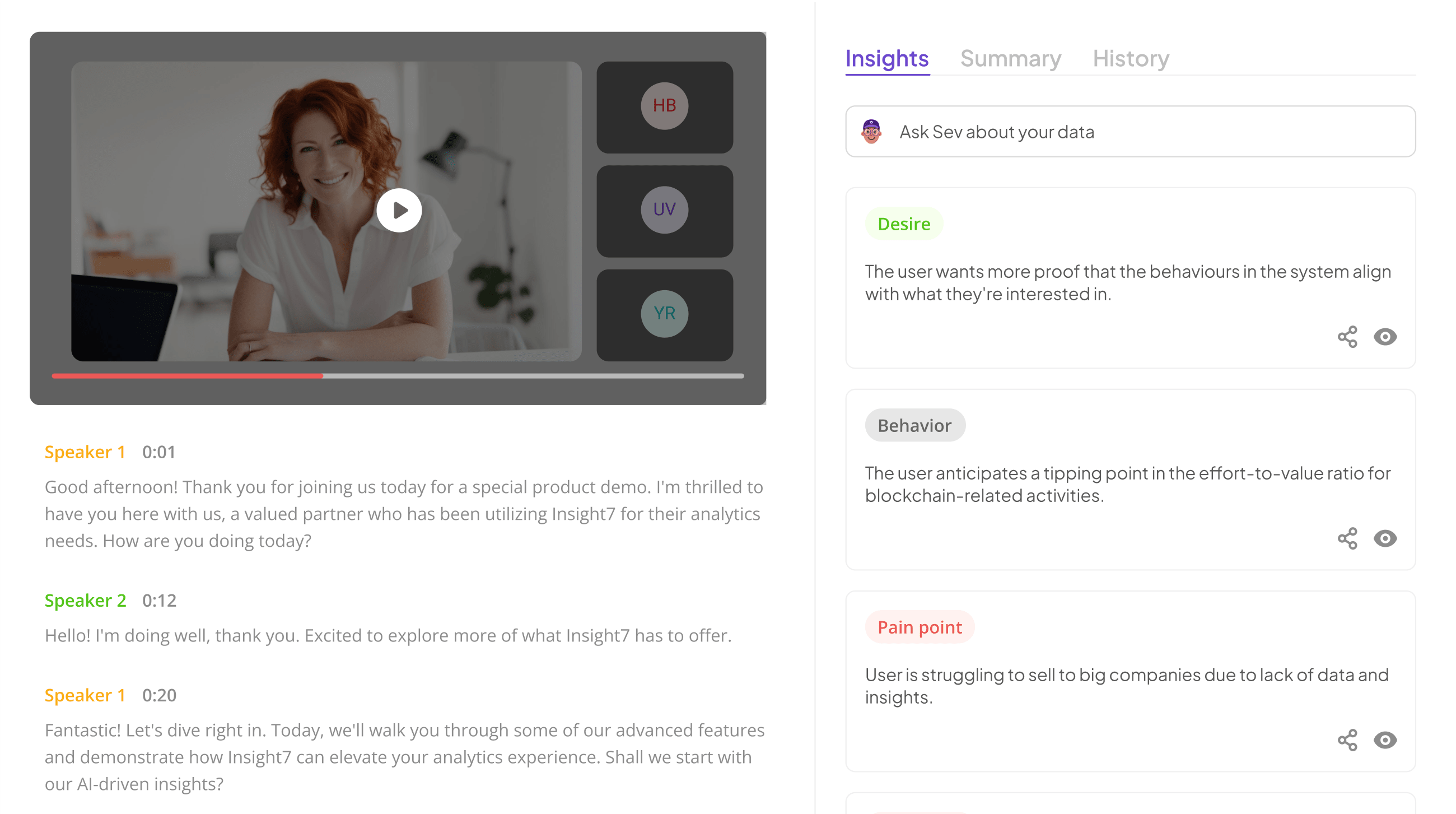
The Shift from Manual to AI-driven QA Metrics
The transition from manual to AI-driven QA metrics represents a pivotal moment in quality assurance practices. Traditional manual monitoring often involves labor-intensive processes that not only consume significant time but also increase the risk of human errors. With a reliance on human judgment, the potential for oversight can result in critical quality issues being missed, leading to costly repercussions in software development.
On the other hand, AI-driven QA metrics offer a robust solution that enhances efficiency and effectiveness. They provide real-time data analysis, enabling organizations to respond more swiftly to emerging problems. This shift to AI-driven metrics ensures increased accuracy and consistency in monitoring, ultimately leading to improved software quality. Organizations can now unlock deeper insights and make informed decisions based on comprehensive data analysis, fostering a culture of continuous improvement in their QA practices.
The Limitations of Manual Monitoring
Manual monitoring of quality assurance processes presents several limitations that can hinder efficiency and accuracy. First, these processes tend to be time-consuming, as teams must dedicate significant resources to collect and analyze data. For example, assessing a limited sample of calls necessitates picking and choosing which calls to monitor, ultimately resulting in incomplete insights. This method can restrict the ability to gain a comprehensive understanding of overall quality metrics.
Moreover, human error is an unavoidable aspect of manual monitoring. Analysts may overlook critical details or misinterpret data, which can lead to inaccuracies in results. These inconsistencies can undermine the reliability of gathered insights, making it difficult to trust the findings. In contrast, AI-driven QA metrics offer a more streamlined approach, allowing for comprehensive and precise quality evaluations with minimal risk of error. Ultimately, the shift towards AI-driven solutions can transform how organizations monitor and enhance their quality assurance efforts, enabling a more efficient and data-driven approach.
- Time-consuming Processes
Time-consuming processes often derail the efficiency of quality assurance workflows, making them less effective. Manual monitoring of QA metrics can involve extensive hours spent on data collection and analysis, ultimately slowing down decision-making. This method not only consumes precious resources but also increases the likelihood of human error. Manual processes force teams to sift through mountains of data and reports, which can hinder timely interventions in performance issues.
AI-driven QA Metrics revolutionize this landscape by automating labor-intensive tasks. With AI tools, organizations can rapidly gather and analyze data, freeing up personnel for strategic initiatives. This intelligent automation streamlines workflows, ensuring that potential issues are flagged for review before escalating. By mitigating time-consuming processes, teams become more agile and responsive without sacrificing quality, establishing a continuous improvement cycle beneficial for overall operational productivity.
- Human Error Factors
Human errors can significantly affect the outcomes of quality assurance (QA) processes. These mistakes often stem from fatigue, lack of training, or misunderstanding of complex systems. In a traditional monitoring environment, human oversight can lead to inconsistencies and errors that may go unnoticed until they impact the end user. Such oversights can ultimately undermine product quality and customer satisfaction.
AI-driven QA Metrics systematically reduce the risk of human error by leveraging advanced algorithms for data analysis. With real-time performance tracking, AI tools automatically detect deviations from expected outcomes, allowing for quicker responses. By minimizing reliance on manual input, organizations can significantly enhance accuracy and consistency in their quality assurance efforts. In an age where precision is paramount, transitioning to automated metrics not only increases efficiency but also fortifies the overall quality of output, ensuring a more reliable product for consumers.
The Advantages of AI-Driven Monitoring
AI-driven QA Metrics offer significant advantages over traditional manual monitoring, making them an essential tool for quality assurance. One of the primary benefits is real-time data analysis, which allows teams to identify issues as they occur. This immediate insight enables organizations to respond swiftly, reducing delays and enhancing overall efficiency.
Additionally, AI-driven monitoring increases accuracy and consistency. Human error is an inherent risk in manual processes, often leading to oversight or misinterpretation. In contrast, AI systems reliably process vast amounts of data, ensuring that metrics are consistently captured and analyzed. This not only boosts the quality of insights gathered but also fosters a more data-driven decision-making environment. Embracing these advantages can transform QA processes, ultimately leading to improved product quality and better customer satisfaction.
- Real-time Data Analysis
AI-driven QA Metrics significantly enhance real-time data analysis capabilities, allowing organizations to make informed decisions faster than ever. By employing sophisticated algorithms, AI tools can continuously monitor user interactions and system performance. This process generates a wealth of data that is instantly analyzed, providing immediate insights into application behavior and user satisfaction.
With traditional manual monitoring, data collection and interpretation can take significant time. In contrast, AI-driven monitoring swiftly identifies patterns and anomalies, enabling teams to respond promptly. For example, AI can detect spikes in user complaints or performance issues in real-time, allowing developers to address problems proactively. This agility leads to improved software quality and user experience, ultimately resulting in higher customer satisfaction and retention. By integrating real-time data analysis into QA processes, organizations can stay ahead, making adjustments that elevate their products based on real data rather than assumptions.
- Increased Accuracy and Consistency
AI-driven QA Metrics significantly enhance accuracy and consistency in monitoring quality assurance processes. By harnessing the capabilities of artificial intelligence, organizations can reduce human error and ensure that data is analyzed with exceptional precision. This shift from traditional methods enables real-time insights, allowing teams to make data-driven decisions faster than ever before.
Moreover, the consistency provided by AI ensures that the monitoring process adheres to established benchmarks and metrics. Automated systems can identify anomalies and trends with great reliability, eliminating the variability introduced by manual assessments. As a result, organizations witness improved performance outcomes and higher-quality product deliveries. Ultimately, adopting AI-driven QA Metrics fosters a more agile and responsive quality assurance environment, enhancing overall operational efficiency and effectiveness.
Extract insights from interviews, calls, surveys and reviews for insights in minutes
6 Key AI-driven QA Metrics Captured Better with AI
AI-driven QA metrics represent a transformative approach in quality assurance, significantly improving how organizations capture and analyze data. These metrics not only automate labor-intensive processes but also enhance the accuracy and speed of quality evaluations. One of the key advantages lies in defect detection, where AI systems efficiently identify and classify issues that might take considerable time when addressed manually. This automation allows teams to focus on high-priority tasks rather than being bogged down by extensive error checks.
Moreover, performance monitoring becomes seamless through real-time data analysis, enabling organizations to react swiftly to any emerging issues. This proactive stance fosters a culture of continuous improvement, vital for software development. Another notable metric is usability feedback analysis. Utilizing AI for sentiment analysis allows for nuanced understanding of user experiences, which manual methods often overlook. Together, these AI-driven QA metrics facilitate a comprehensive, insightful view of quality assurance processes, helping organizations ensure superior software performance.
1. Defect Detection and Classification
AI-driven QA metrics play a crucial role in defect detection and classification, enhancing software quality. Automation technologies enable swift identification of bugs, reducing the time spent on manual checks. As systems continuously analyze data, they flag issues in real time, facilitating quicker resolutions.
Moreover, predictive analysis offers insights into potential future defects by recognizing trends from historical data. By assessing patterns, AI tools can predict where defects are likely to occur next, allowing teams to proactively address these areas. This proactive approach contrasts with traditional methods, which often react to problems after they emerge. Overall, AI-driven QA metrics streamline defect detection and classification, significantly improving both efficiency and accuracy in quality assurance processes.
- Automation of Bug Identification
Automation of Bug Identification significantly enhances the quality assurance process by streamlining how defects are detected in software. With AI-driven QA metrics, organizations can move beyond manual methods that are often slow and prone to human error. Automated systems can tirelessly scan codebases in real-time, rapidly identifying bugs before they escalate into user-facing issues. This shift ensures that potential problems are resolved earlier in the development cycle, ultimately saving time and resources.
One of the most pronounced benefits of this approach is the predictive analysis capabilities that AI brings to the table. By analyzing historical data trends, AI systems can anticipate where bugs are most likely to occur, allowing teams to preemptively address vulnerabilities. This holistic understanding not only optimizes the bug identification process but also enhances overall software quality, giving organizations a competitive edge in their respective markets. Embracing AI-driven QA metrics in bug identification is essential for teams aiming for excellence in software development.
- Predictive Analysis of Trends
Predictive analysis of trends is revolutionizing how we approach quality assurance metrics. AI-driven QA metrics empower teams to uncover patterns in data that often go unnoticed in manual reviews. By analyzing historical data, AI systems can accurately forecast potential defects or performance issues before they affect user experience. This proactive approach shifts the QA process from reactive measures to anticipatory actions, ensuring a higher standard of product quality.
Moreover, the integration of AI in monitoring allows for continuous improvement in QA practices. Machine learning algorithms enhance the ability to identify correlations within vast datasets, highlighting trends over time. This insight facilitates informed decision-making, enabling teams to address issues before they escalate. As businesses increasingly rely on AI-driven metrics, the predictive analysis of trends becomes a cornerstone of effective quality assurance, leading towards improved software performance and customer satisfaction.
2. Performance Monitoring and Optimization
Performance monitoring and optimization are essential components of an effective quality assurance strategy. In today's fast-paced digital environment, businesses must leverage AI-driven QA metrics to gain insights and enhance their performance monitoring efforts. This approach provides real-time performance data, ensuring that potential issues are identified before they escalate into significant problems.
Additionally, AI-powered systems enable an automated alert system for anomalies in performance. This level of responsiveness surpasses traditional manual monitoring methods, allowing teams to focus on strategic improvement rather than reactive measures. Optimizing performance through continuous monitoring not only boosts product reliability but also enhances user satisfaction by delivering a consistently high-quality experience. With AI-driven QA metrics, organizations can anticipate challenges, streamline operations, and foster a culture of continuous improvement.
- Real-time Performance Data
Real-time performance data plays a crucial role in optimizing software quality, especially when utilizing AI-driven QA metrics. With AI technology at the helm, performance data can be captured and analyzed instantly, providing teams with immediate insights into system functionality. This agility enables organizations to swiftly address potential issues before they escalate, maintaining the integrity of applications.
Additionally, real-time performance data allows for continuous monitoring that far surpasses traditional methods. AI systems review vast quantities of data, detecting anomalies and performance dips that may go unnoticed in manual evaluations. Such immediate feedback not only streamlines processes but also enhances decision-making, allowing teams to make informed adjustments on-the-fly. This dynamic approach empowers businesses to stay competitive in an ever-evolving digital landscape, ensuring optimal performance at all times.
- Automated Alert System for Anomalies
An automated alert system for anomalies plays a vital role in enhancing AI-driven QA metrics. This system continuously monitors various aspects of software performance, allowing for immediate identification of any irregularities. Instead of relying on human discretion, which can be error-prone, this AI solution delivers consistent oversight. Thus, it reduces the time spent on manual checks, streamlining the quality assurance process significantly.
When the system detects an anomaly, it triggers alerts in real time. This instant feedback allows teams to act swiftly, mitigating potential risks before they become significant problems. Additionally, the system's ability to analyze historical data ensures that alerts are not only reactive but also preventive. By utilizing advanced algorithms, it anticipates trends and issues, ensuring a proactive approach to quality assurance. In today’s fast-paced digital environment, adopting such automated systems is essential for maintaining software integrity and user satisfaction.
3. Usability Feedback Analysis
Usability feedback analysis plays a vital role in understanding user experiences and preferences. With AI-driven QA metrics, organizations can efficiently collect, process, and analyze user feedback to enhance product quality. AI tools excel in performing sentiment analysis on feedback, allowing for real-time insights into customer opinions. This process minimizes manual effort, ensuring faster access to critical insights that help shape product improvements.
AI-driven methodologies categorize user experiences based on the feedback received. By leveraging AI technology, companies can identify trends and patterns that may go unnoticed in traditional methods. This not only facilitates a better understanding of user needs but also aligns product features with user expectations. Overall, utilizing AI-driven QA metrics for usability feedback analysis empowers businesses to make informed decisions and adapt swiftly to changing customer requirements.
- Sentiment Analysis and User Feedback
In the realm of QA metrics, sentiment analysis and user feedback offer profound insights that drive product improvement. By employing AI-driven QA metrics, organizations can effectively categorize user sentiments into positive, negative, and neutral feedback. This automatic classification enables faster responses to customer concerns and fosters enhanced user experiences, allowing businesses to adapt more swiftly to user needs.
Furthermore, AI tools can analyze trends in user feedback, revealing common pain points and areas for enhancement. These insights form a valuable foundation for product development strategies. The ability to correlate sentiment data with specific product features adds another layer of understanding, guiding teams toward evidence-based decisions. AI-driven sentiment analysis not only saves time compared to manual methods but significantly improves the accuracy of the interpretations. Consequently, businesses are empowered to refine their offerings and evolve based on direct user feedback.
- AI-driven Experience Categorization
AI-driven Experience Categorization focuses on refining user interactions by employing advanced algorithms to analyze data patterns. This innovative approach evaluates user feedback and interactions in real-time, allowing for swift adaptations that enhance overall service quality. By leveraging these capabilities, organizations can categorize customer experiences more effectively and react proactively to emerging trends.
Moreover, AI-driven QA metrics facilitate the identification of critical areas needing improvement. It streamlines the feedback analysis process, differentiating between positive and negative sentiments with remarkable accuracy. Consequently, businesses can prioritize enhancements based on user needs, leading to better customer satisfaction. The transition from traditional methods to AI-driven analysis not only boosts operational efficiency but ensures a more intuitive understanding of the user experience. This combination serves to elevate software quality and foster long-term user loyalty.
Tools Enhancing AI-driven QA Metrics
Tools that enhance AI-driven QA metrics play a pivotal role in modern software development. These tools are specifically designed to address the challenges posed by traditional manual monitoring methods. With their ability to collect, analyze, and report on quality assurance data in real-time, they provide insights that manual methods often overlook. By automating the process of defect detection and performance monitoring, these tools significantly reduce human error and inefficiencies.
For instance, leading AI-driven QA tools like Applitools and Testim offer unique features that streamline testing processes. Applitools utilizes visual AI to ensure interface consistency across devices, while Testim employs machine learning for test automation. Additionally, platforms such as Mabl and Functionize deliver intelligent overview and seamless integration for continuous testing. Together, these tools not only elevate the accuracy of AI-driven QA metrics but also facilitate a more agile and responsive development environment.
insight7
AI-driven QA metrics revolutionize the way companies assess quality assurance processes. These metrics excel in capturing vital data that manual monitoring simply cannot match. For instance, AI can analyze vast amounts of data in real-time, identifying patterns and trends that would take human analysts considerable time to discern. This capability not only enhances accuracy but also allows teams to respond to issues promptly, minimizing downtime and enhancing overall efficiency.
Moreover, machine learning algorithms continuously improve their analysis based on historical data, further refining their ability to diagnose problems. In contrast, manual processes often rely on human intuition, which may introduce errors and inconsistencies. As organizations strive for continuous improvement, adopting AI-driven QA metrics is essential for staying competitive in today's fast-paced environment. By integrating these advanced technologies into QA practices, businesses can unlock deeper insights and achieve higher standards in software quality assurance.
- Features and Benefits Overview
The transition to AI-driven QA metrics represents a significant advancement in monitoring software quality. Unlike traditional manual methods, which are often slow and prone to human error, AI technology delivers immediate insights that improve decision-making processes. With capabilities such as real-time data analysis and predictive analytics, organizations can identify potential defects before they escalate. This proactive approach not only enhances product quality but also streamlines operational efficiency.
AI-driven QA metrics empower teams to focus on strategic improvements rather than mundane tasks. By automating the identification of anomalies and offering detailed reports, teams gain a comprehensive understanding of user experiences and system performance. Features like sentiment analysis enable organizations to capture nuanced feedback, while robust data categorization tools assist in prioritizing essential usability insights. Embracing these innovative technologies streamlines processes and significantly boosts overall software quality.
Additional Top Tools
In exploring AI-driven QA metrics, it's essential to consider the additional top tools that can further enhance your testing capabilities. Solutions like Applitools offer visual AI testing, which ensures that your software interfaces are both functional and visually appealing. Their ability to detect visual discrepancies makes it an indispensable tool for maintaining quality consistency across various resolutions and devices.
Similarly, Testim provides an AI-powered automation framework that simplifies the test creation process. By utilizing machine learning, it adapts to various application states, significantly reducing maintenance efforts. Mabl stands out for its intelligent test automation; it continuously learns from your development processes, enhancing the accuracy of automated tests. Finally, Functionize presents a unified AI testing platform that combines various testing methodologies. Leveraging these tools will not only streamline your QA processes but also empower your team to capture critical insights efficiently, positioning you ahead in today’s competitive landscape.
- Applitools: Visual AI Testing Efficiency
Visual AI testing efficiency transforms the realm of quality assurance by leveraging AI-driven QA metrics. This methodology streamlines the testing process, allowing teams to streamline their workflows while ensuring impeccable software quality. Visual testing employs advanced algorithms to identify discrepancies between the expected and actual visual representations of an application, minimizing the potential for human error often seen in manual testing methods.
The adoption of visual AI testing not only enhances accuracy but also accelerates the detection of visual bugs, enhancing overall testing efficiency. By utilizing these AI-driven metrics, QA teams can gain insights into usability and layout issues more swiftly than traditional approaches. Consequently, this reduces the testing cycle time, allowing teams to focus on core development objectives. In an era where user experience is paramount, incorporating visual AI testing becomes a vital strategy, ensuring that software products consistently meet high standards of quality and visual integrity.
- Testim: AI-Powered Automation
AI-driven QA Metrics revolutionize how quality assurance is conducted, offering streamlined automation that enhances efficiency. With the rise of advanced algorithms, AI-powered automation systems efficiently analyze large sets of data, identifying inconsistencies and defects that might be overlooked in a manual review. This system not only saves time but also provides a level of precision that human monitoring can rarely match, establishing a benchmark in automated quality assurance.
Furthermore, AI-driven QA Metrics deliver insights in real-time, ensuring that any discrepancies are addressed proactively. By continuously learning from existing data patterns, these systems predict and adapt to new challenges, further refining the testing process. Through automation, teams can focus on strategic initiatives rather than getting bogged down by repetitive tasks, leading to improved product quality and user satisfaction. Embracing AI-powered automation signifies a shift toward more robust quality assurance practices, making the software development process more efficient and reliable.
- Mabl: Intelligent Test Automation
Intelligent test automation plays a vital role in revolutionizing the software quality assurance landscape. Integrating AI-driven QA metrics into the testing process allows teams to analyze vast amounts of data effortlessly and with heightened accuracy. Detailed insights generated through automation empower organizations to identify defects earlier and prioritize them effectively. This streamlined process minimizes the burdens of manual testing, ultimately enhancing productivity.
Moreover, intelligent test automation transforms the way metrics are captured and analyzed. By leveraging continuous testing, teams can maintain consistent performance checks, ensuring that each release meets stringent quality standards. The automated nature of processes like regression testing and usability feedback analysis not only expedites work but also guarantees a depth of data that manual monitoring often overlooks. Thus, intelligent test automation decisively positions teams to deliver high-quality software products while minimizing operational inefficiencies.
- Functionize: Unified AI Testing Platform
The Unified AI Testing Platform serves as a powerful solution for enhancing quality assurance (QA) processes. This platform simplifies the way teams access and utilize AI-driven QA metrics, enabling effortless interaction without the need for extensive technical knowledge. As a result, insights can be democratized across the organization, allowing every team member to leverage quality metrics efficiently.
Within the platform, users can automate the analysis of large data sets, uncovering critical insights into customer experiences, pain points, and overall software performance. This swift identification is crucial for timely decision-making, helping teams stay ahead of issues before they escalate. By capturing QA metrics through an AI framework, the platform transforms complex data into actionable insights, ultimately supporting continuous improvement without the drawbacks of manual monitoring. As organizations transition to AI-driven methods, they become better equipped to ensure superior software quality and user satisfaction.
Conclusion: Embracing AI-driven QA Metrics for Superior Software Quality
AI-driven QA Metrics are transforming the software quality assurance landscape by surpassing traditional manual methods. By incorporating advanced algorithms and machine learning, businesses can achieve unprecedented accuracy and consistency in monitoring their software systems. The shift to AI allows for real-time data analysis, enabling teams to detect and classify defects much faster than human oversight can provide.
Embracing these innovative metrics facilitates a proactive approach to quality assurance. Companies can now identify usability issues through sentiment analysis and automate the performance monitoring process. As organizations adapt to these AI-driven metrics, the overall quality of software products improves significantly, leading to enhanced user satisfaction and a stronger competitive edge in the market.