Most Scalable Speech Analytics Engines With AI Enhancement
-
Bella Williams
- 10 min read
AI-Driven Speech Analytics has revolutionized how businesses understand their customer interactions. By leveraging advanced algorithms, companies can analyze vast amounts of audio data in real-time, extracting insights that enhance customer service and operational efficiency. This technology enables organizations to decode conversations, identify trends, and assess performance metrics that are vital for business growth.
In a landscape where effective communication is paramount, AI-Driven Speech Analytics offers a solution that not only automates the analysis process but also scales with organizational needs. As businesses expand, the ability to seamlessly process increasing volumes of data becomes essential. This section will delve into the benefits of adopting AI-enhanced speech analytics engines, focusing on their scalability features and overall impact on performance.
Analyze & Evaluate Calls. At Scale.
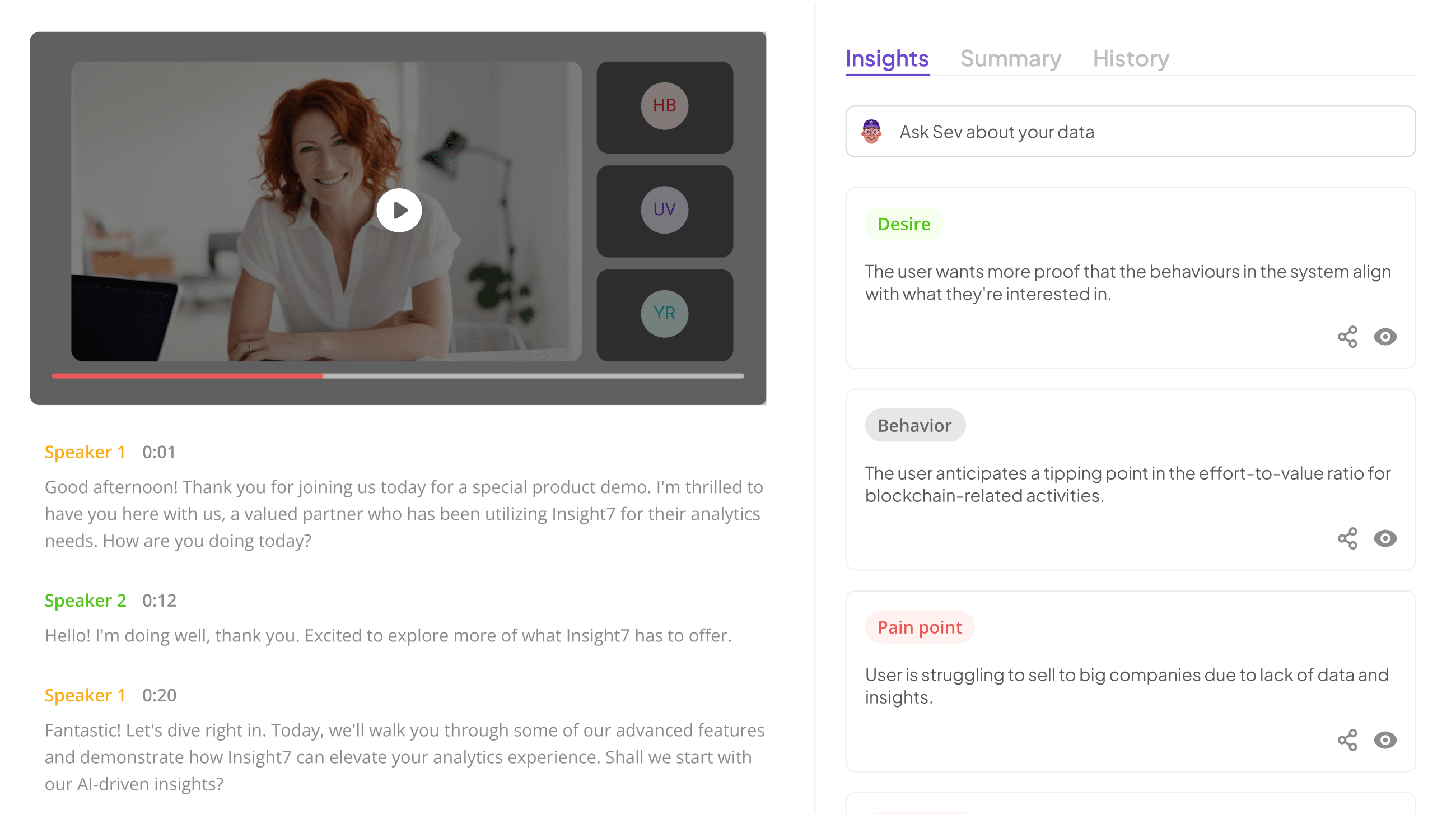
Understanding the Importance of Scalability in AI-Driven Speech Analytics
In the rapidly growing field of AI-Driven Speech Analytics, scalability is paramount. Organizations are inundated with data from customer interactions, necessitating robust systems that can adapt and grow alongside their needs. As the volume of conversations and interactions skyrockets, it becomes essential for analytics engines to provide insights efficiently without compromising performance.
Understanding why scalability matters allows companies to make informed decisions about the tools they adopt. Scalable systems enable businesses to handle increased workloads seamlessly while ensuring rapid response times. This agility allows companies to derive actionable insights quickly, giving them a competitive edge. Furthermore, a scalable analytics engine can facilitate collaboration and data sharing among teams, breaking down silos and enhancing overall productivity. By embracing scalable AI-Driven Speech Analytics, organizations position themselves to harness customer insights effectively, turning data into strategic advantages in a fast-paced environment.
Why Scalability Matters
Scalability is a critical factor in AI-Driven Speech Analytics. As businesses expand and the volume of customer interactions increases, traditional analysis methods can falter. This can lead to delayed insights, which in turn hampers decision-making capabilities. By ensuring scalability, organizations can efficiently manage large datasets and derive valuable insights without overwhelming their resources.
Effective scalability allows AI-Driven Speech Analytics engines to process multiple conversations simultaneously. This ensures timely feedback and actionable insights, which are essential in today’s fast-paced market. Furthermore, scalable systems support the integration of advanced features, enhancing analytical depth. With the ability to swiftly adapt to changing demands, organizations can capture emerging trends and customer sentiments, providing them with a competitive edge. Ultimately, prioritizing scalability in speech analytics is not just about capacity; it is also about enabling organizations to respond proactively to customer needs, ensuring long-term success.
Key Metrics for Assessing Scalability
To effectively assess scalability in AI-driven speech analytics, several critical metrics should be considered. First, throughput measures the volume of audio data processed within a specific timeframe, indicating how well the engine can handle increasing demands. Next, latency refers to the time taken from audio input to output generation. Low latency is vital for real-time applications, ensuring immediate responsiveness.
Additionally, the architecture's elasticity matters; scalable solutions can deploy additional resources as needed without significant downtime. Resource utilization should also be monitored to optimize efficiency and balance performance with cost. Finally, the adaptability of the engine to integrate new features or technologies is crucial for maintaining relevancy in a fast-evolving AI landscape. By analyzing these metrics, organizations can make informed decisions, ensuring that their AI-driven speech analytics solutions remain effective and responsive to future challenges.
AI-Driven Speech Analytics: Exploring the Top Engines
AI-Driven Speech Analytics has become a pivotal technology for organizations seeking to elevate their operational efficiency and customer interactions. By harnessing advanced algorithms and machine learning capabilities, these engines dissect spoken language to extract actionable insights from voice data. Companies can make informed decisions based on real-time analysis of conversations, enhancing overall engagement strategies.
When exploring the top engines, several key players stand out. Each engine offers unique features tailored for scalability, such as real-time processing, multi-language support, and integration capabilities with existing systems. Google Cloud Speech-to-Text, for example, excels in accuracy and adaptability, making it suitable for diverse applications. Similarly, the Microsoft Azure Speech Service provides extensive customization options, empowering businesses to fine-tune their analytics according to specific needs. Ultimately, the choice of engine should reflect a business's objectives, ensuring it aligns with their growth plans and analytical requirements.
Extract insights from interviews, calls, surveys and reviews for insights in minutes
List of Leading AI-Driven Speech Analytics Engines
The landscape of AI-Driven Speech Analytics is rapidly evolving, with numerous engines offering innovative features to enhance performance and scalability. Choosing the right analytics engine is critical for organizations seeking comprehensive insights into their customer interactions. The leading engines in this space provide capabilities such as real-time transcription, speaker identification, and sentiment analysis. These features empower businesses to improve decision-making, streamline operations, and enhance customer experiences.
A few notable engines include those that utilize advanced natural language processing to dissect and analyze conversations effectively. They leverage machine learning algorithms that continuously adapt and improve accuracy over time. Each engine excels in specific areas, such as multi-language support or integration with existing business tools, making them suitable for diverse industry needs. By focusing on scalability, these AI-Driven Speech Analytics engines ensure that they can handle increasing amounts of data without sacrificing performance quality.
- insight7
AI-driven speech analytics has transformed the way businesses extract meaningful insights from customer interactions. A robust platform such as insight7 enables organizations to analyze large volumes of audio data seamlessly. This technology allows companies to capture nuanced customer signals that traditional methods often overlook. In today’s fast-paced environment, timely access to these insights can significantly improve decision-making and competitive advantage.
However, adopting AI-driven speech analytics can pose challenges. For instance, businesses must ensure they have the infrastructure to process data at scale. Additionally, integration with existing systems is crucial to make the most of these insights. By aligning AI capabilities with operational strategies, companies can facilitate more effective collaboration among teams and enhance overall productivity. Ultimately, the goal is to leverage customer interactions to drive actionable business strategies, resulting in improved customer satisfaction and loyalty.
- Google Cloud Speech-to-Text
Google Cloud Speech-to-Text stands out as a pivotal resource for AI-driven speech analytics, catering to the burgeoning need for scalable solutions. This tool excels in transforming audio recordings into text, enabling businesses to tap into valuable insights. With its capability to transcribe large volumes of audio simultaneously, it offers a streamlined approach for analyzing conversations.
One of the core benefits lies in its intuitive interface, which simplifies the process of file uploads and analytics. After transcription, users can easily extract crucial insights from conversations, allowing for enhanced decision-making. Moreover, this service continuously evolves, integrating advanced features that further improve its efficiency. By utilizing this powerful engine, businesses can navigate their data effectively, drawing actionable conclusions with remarkable ease, ultimately boosting their operational efficiency. In the realm of AI-driven speech analytics, this offering is indispensable for those aiming to maximize scalability and insight generation.
- Microsoft Azure Speech Service
AI-Driven Speech Analytics has transformed how organizations analyze spoken data. Among the leading engines, one standout option provides exceptional capabilities for processing and interpreting audio insights. This service harnesses advanced machine learning algorithms to accurately transcribe speech and recognize individual voices, significantly improving the quality of analytics.
One key feature is its ability to deliver real-time insights, which enhances decision-making processes. Users can generate detailed reports that evaluate speaker performance, compliance, and overall engagement. Moreover, customization allows organizations to adapt the service for specific applications, such as compliance and quality assurance. By utilizing this service, companies can maximize their operational efficiency and improve customer interactions, ensuring they stay ahead in a competitive landscape.
- Amazon Transcribe
As organizations strive to extract meaningful insights from audio data, AI-Driven Speech Analytics becomes a vital asset. One prominent solution offers a highly efficient way to transcribe and analyze conversations at scale. Users can easily upload multiple audio files simultaneously, streamlining the transcription process. This capability allows businesses to handle a large volume of calls without compromising accuracy or speed, making it ideal for industries where call data is crucial.
Once the audio is transcribed, the platform provides tools for analyzing the data through intuitive templates and query options. Users can quickly identify pain points and extract key insights from conversations, transforming unstructured audio into actionable data. The ability to visualize this information enhances understanding and facilitates effective decision-making. By focusing on scalability and user experience, this AI-Driven Speech Analytics solution empowers businesses to harness the full potential of their conversation data.
- IBM Watson Speech to Text
IBM Watson Speech to Text offers a powerful solution for converting audio into text, which is essential for organizations looking to maximize insights from their conversations. The process begins with high-volume transcription capabilities, allowing users to upload numerous audio files simultaneously. This functionality streamlines the analysis of customer interactions, making it easier to access valuable information hidden in recorded dialogues.
Once transcribed, the platform enables efficient analysis of the text. Users can identify key themes, pain points, and trends effortlessly. Moreover, the generated transcripts facilitate the extraction of actionable insights through simple queries and summaries. This overall process exemplifies how AI-driven speech analytics can transform raw audio data into structured insights, delivering substantial value for businesses seeking to enhance their decision-making and customer experiences. By integrating such tools, organizations position themselves to stay ahead in competitive markets while effectively leveraging their conversational data.
Features that Enhance Scalability
Scalability is essential in AI-Driven Speech Analytics, as it ensures the ability to grow and adapt in response to increasing data demands. Effective features that enhance scalability include cloud infrastructure, which allows for seamless management of vast amounts of audio data. Additionally, advanced algorithms capable of processing and analyzing audio in real time significantly contribute to the overall efficiency of these engines.
Another crucial aspect is the flexibility of integration options, allowing businesses to merge multiple data sources without disruption. Furthermore, user-friendly dashboards enable easy access to insights, fostering collaboration among teams. When organizations can effectively utilize AI-Driven Speech Analytics, they become better equipped to capture customer signals, translate insights into actionable strategies, and ultimately maintain a competitive edge in a rapidly evolving market. These scalable features ensure that analytics can meet the growing needs of businesses and adapt to future challenges.
Conclusion: The Future of AI-Driven Speech Analytics
The future of AI-Driven Speech Analytics promises a transformative impact on industries reliant on customer interactions. As these technologies continue to evolve, they will offer unprecedented levels of accuracy, enabling organizations to decode customer sentiments and preferences with remarkable clarity. Enhanced scalability features will ensure that businesses can analyze vast amounts of data efficiently, resulting in improved decision-making processes and actionable insights.
Moreover, the integration of machine learning algorithms will refine performance over time. This progression will empower organizations to customize their analytics strategies in real-time, aligning their services with customer expectations more effectively. As AI continues to unfold, the potential applications of AI-Driven Speech Analytics will only expand, creating richer, more responsive communication channels.