In recent years, the landscape of quality assurance has undergone a significant transformation, thanks to the advent of cutting-edge QA AI evaluation systems. These advanced tools empower QA teams to streamline their processes, ensuring higher efficiency and accuracy in assessments. Imagine a world where repetitive tasks are automated, freeing up resources to focus on strategic decision-making and innovative problem-solving.
Cutting-edge QA AI systems utilize complex algorithms and real-time data analysis to deliver valuable insights into performance metrics. By automating reporting and providing actionable feedback, these systems not only enhance the evaluation process but also contribute to continuous improvement within teams. As organizations seek to maintain a competitive edge, embracing these technologies is essential for modern QA practices.
Analyze qualitative data. At Scale.
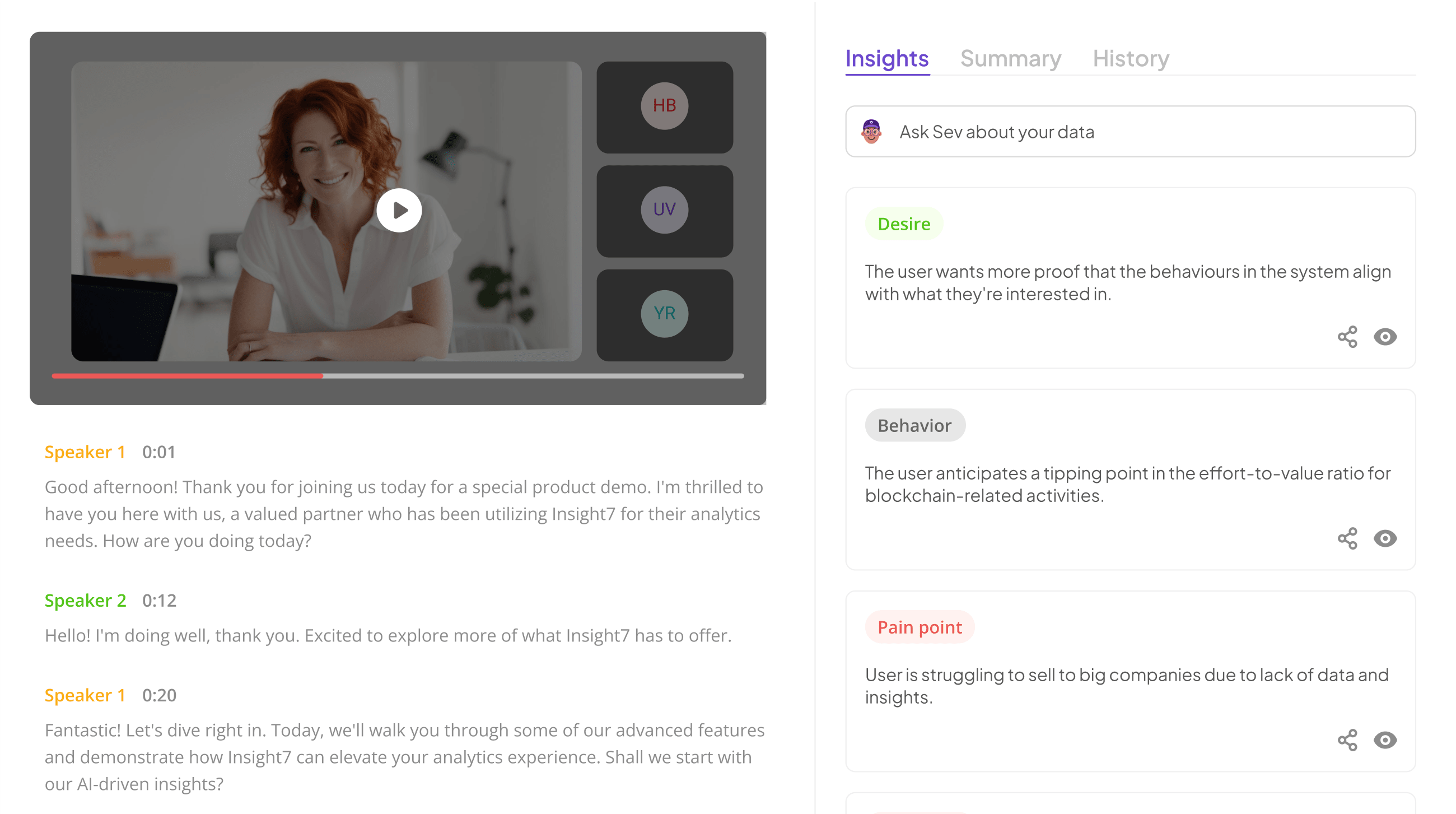
Understanding the Role of Cutting-edge QA AI in Evaluation Systems
Cutting-edge QA AI technologies are transforming the landscapes of evaluation systems within quality assurance teams. As organizations strive for higher efficiency and accuracy, these advanced tools offer a systematic approach to evaluating performance metrics. By leveraging real-time data analysis, these systems provide immediate feedback, empowering QA teams to refine their processes and enhance product quality steadily.
The role of cutting-edge QA AI extends beyond mere data processing. It includes creating customizable evaluation criteria that align with specific organizational goals. By defining key performance indicators and weighting them appropriately, teams can assess calls or interactions based on tailored benchmarks. This structured approach not only saves time but also fosters accountability and consistency in evaluations. With performance insights becoming more accessible, organizations can address compliance challenges more effectively, ensuring they meet industry standards and continuously improve their services.
The Evolution of QA AI Tools
The journey of QA AI tools began with simple rule-based systems that offered a limited perspective on quality assurance. As technology advanced, these tools evolved, harnessing machine learning and natural language processing. This transition shifted the focus from manual checks to more sophisticated, AI-driven evaluations, paving the way for cutting-edge QA AI tools that provide deeper insights.
Today, these tools can analyze vast amounts of data in real-time, offering immediate feedback and scores tailored to individual team members. The evolution has not just optimized efficiency but has also enhanced the accuracy of evaluations. With capabilities like automated reporting, QA teams can now devote more time to strategic initiatives and less on repetitive tasks. This transformative journey highlights the growing importance of integrating advanced technologies in quality assurance, empowering teams to enhance performance and deliver exceptional results.
- Historical overview of AI in quality assurance.
The historical landscape of AI in quality assurance has evolved dramatically over the past few decades. Initially, QA processes relied on manual evaluations, which were often time-consuming and prone to human error. However, as technology advanced, businesses recognized the potential of artificial intelligence to enhance their QA efforts. This led to the development of cutting-edge QA AI systems that streamline processes, improve accuracy, and provide actionable insights.
In the early days, AI applications in QA were rudimentary, focusing on basic data pattern recognition. Over time, algorithms became more sophisticated, enabling complex real-time data analysis and automated reporting. Organizations now leverage these innovations to enhance performance evaluations, ensuring compliance and driving continuous improvement. This transition from manual to AI-driven evaluations marks a pivotal moment in the QA journey, leading to more efficient and effective quality assurance practices.
- The shift towards AI-driven evaluations.
The shift towards AI-driven evaluations marks a transformative period in quality assurance. Traditional methods often relied on manual processes, which were time-consuming and prone to human error. Now, with the emergence of cutting-edge QA AI, evaluation systems can analyze data in real-time, providing insights that significantly enhance decision-making. These advanced tools automate reporting, allowing QA teams to focus on strategic actions rather than data gathering.
The transition to AI-driven evaluations offers several advantages. First, these systems increase accuracy by minimizing biases that can occur in manual assessments. Second, they provide consistent metrics across different evaluations, ensuring uniformity in quality checks. Lastly, AI can identify trends and anomalies that may go unnoticed through conventional methods, highlighting areas for improvement. Embracing this technological shift positions QA teams to achieve greater efficiency and uphold high standards, fostering an adaptive and responsive quality assurance process.
Key Features of AI Evaluation Systems
Cutting-edge QA AI evaluation systems are designed to streamline and enhance quality assurance processes, ensuring that teams operate efficiently. One of the most significant features of these systems is real-time data analysis, which allows teams to evaluate performance metrics as they occur. This capability ensures that any deviations from expected standards can be addressed immediately, promoting timely interventions.
Another key feature of these AI evaluation systems is automated reporting and insights generation. By eliminating the need for manual report compilation, QA teams can focus their efforts on interpreting the data and implementing improvements. These systems also typically offer customization options for reports, allowing teams to tailor outputs according to their specific needs. This flexibility not only enhances usability but also ensures the outputs align closely with decision-making processes. Ultimately, these features position cutting-edge QA AI as indispensable tools for modern quality assurance teams seeking optimal performance.
- Real-time data analysis.
Real-time data analysis transforms how QA teams approach their projects, allowing them to track and interpret data as it surfaces. With cutting-edge QA AI, teams can quickly respond to emerging trends and anomalies, ensuring a proactive rather than reactive stance. This immediate availability of insights enables QA analysts to make data-driven decisions swiftly, which is crucial in fast-paced environments.
There are several key aspects to consider regarding real-time data analysis. First, it provides continuous monitoring, allowing teams to pinpoint problems as they develop. Second, AI-driven tools can automate the identification of significant patterns in data, enhancing the efficiency of the analysis process. Lastly, integrating real-time feedback loops creates a dynamic environment for ongoing improvement, ultimately leading to higher-quality outcomes for products and services. By utilizing cutting-edge QA AI, teams can stay ahead of issues and refine their quality assurance processes effectively.
- Automated reporting and insights.
Automated reporting and insights are crucial components of cutting-edge QA AI systems. They provide real-time analysis and visualization of data, enabling teams to make informed decisions swiftly. With automated reporting, quality assurance processes become streamlined, allowing teams to focus on more strategic tasks instead of time-consuming manual report generation. This shift not only improves efficiency but also enhances the accuracy of insights gathered, thus driving overall project success.
Moreover, these insights offer a comprehensive understanding of customer experiences by highlighting significant pain points and desires. By utilizing advanced algorithms, QA teams can easily access meaningful data and evidence from customer interactions. This capability enables direct responses to customer feedback, ultimately fostering a more positive user experience. In essence, automated reporting and insights serve as foundational elements that empower teams to achieve excellence through innovative QA practices.
Top Cutting-edge QA AI Evaluation Tools for Teams
In the realm of quality assurance, cutting-edge QA AI tools have revolutionized how teams approach evaluation processes. These systems streamline the evaluation of calls, transcripts, and customer interactions, ensuring a comprehensive quality assurance experience. By automating data analysis, these tools help teams identify critical themes and provide actionable insights without requiring extensive training. For teams navigating vast amounts of customer interaction data, the adoption of sophisticated QA AI solutions can significantly enhance efficiency and effectiveness.
Several top-tier tools exemplify the capabilities of cutting-edge QA AI. First, consider Tool A, which offers advanced functionalities including customizable evaluation templates and comprehensive reporting. These features empower QA teams to align their evaluations with specific criteria tailored to their organizational needs. Another notable example is Tool B, known for its seamless integration capabilities with existing workflows, ensuring that teams can implement improvements without disrupting established processes. Embracing these tools transforms the landscape of quality assurance, fostering an environment of continuous improvement and customer satisfaction.
Insight7
Cutting-edge QA AI has revolutionized the way quality assurance teams evaluate and enhance their processes. Insight7 highlights the importance of advanced AI systems in analyzing vast amounts of customer data. With traditional methods struggling under the weight of increasing complexity, teams need solutions that streamline analysis and deliver actionable insights more effectively.
This innovative platform offers self-service capabilities, making it simple for QA teams to harness customer interviews and conversations. By transforming raw data into structured insights, teams can better understand customer needs and streamline collaboration. Real-time analysis allows for timely decision-making, ensuring that companies can remain competitive in an evolving market. Ultimately, adopting cutting-edge QA AI empowers teams to act swiftly on insights, creating a data-driven culture that fosters continuous improvement and innovation.
- Overview and capabilities.
Cutting-edge QA AI systems offer a transformative approach for quality assurance teams aiming for efficiency and accuracy. These advanced tools enable real-time data analysis, making it possible to monitor interactions and extract valuable insights effectively. Additionally, automated reporting simplifies the process of tracking metrics and performance standards, allowing teams to focus on actionable strategy rather than manual data collection.
The capabilities of these systems extend beyond mere data processing. They provide benchmarks to assess service quality, allowing for continuous improvement opportunities based on customer feedback. For instance, by evaluating conversation tone and content, teams can calibrate their training programs to better align with customer expectations. In essence, cutting-edge QA AI not only enhances operational efficiency but also empowers teams to make informed decisions that drive customer satisfaction.
- Use cases in QA teams.
Cutting-edge QA AI systems present transformative use cases that streamline quality assurance processes for teams. These advanced technologies help in automating evaluations of calls, assessing adherence to compliance templates, and identifying areas for improvement in customer interactions. For QA teams, the ability to analyze large volumes of data efficiently is crucial, allowing them to focus on delivering high-quality customer experiences.
One prominent use case involves customizing evaluation criteria to align with specific organizational needs. Teams can set various templates, such as quality assurance or sales call evaluations, to assess performance against predetermined standards. By leveraging AI to transcribe and analyze interactions, QA professionals can uncover insights regarding compliance, customer education, and problem resolution. This not only enhances the learning experience but also drives continuous improvements within teams, making cutting-edge QA AI indispensable in todayโs competitive environment.
Other Leading Tools
As organizations increasingly adopt advanced technologies, several prominent tools complement cutting-edge QA AI systems. Tool A offers robust functionalities that empower QA teams to conduct more efficient evaluations. Its automated testing capabilities enable teams to save time while enhancing accuracy in detecting potential issues. By streamlining processes, Tool A allows teams to focus on strategic decision-making rather than getting bogged down by repetitive tasks.
On the other hand, Tool B stands out with its unique integration capabilities, designed to seamlessly fit into existing workflows. By offering compatible solutions that enhance communication and collaboration, Tool B helps teams engage in collective problem-solving. As organizations strive to optimize their quality assurance processes, these prominent tools testify to the growing trend of utilizing cutting-edge QA AI for improved outcomes. Embracing these systems can significantly enhance efficiency and foster continuous improvement within QA teams.
Tool A
Tool A stands out as a vital resource for modern QA teams seeking to enhance their evaluation processes. This cutting-edge QA AI harnesses advanced algorithms to analyze incoming data efficiently and effectively. With a user-friendly interface, teams can seamlessly upload call recordings and convert them into actionable insights, allowing for comprehensive evaluations without needing extensive training or research expertise.
One of the main functionalities of Tool A is its ability to create customizable evaluation templates. With this feature, teams can assess calls against specific criteria such as compliance, customer engagement, and problem resolution. By structuring evaluations around these core components, the tool enables QA professionals to pinpoint areas for improvement and deliver targeted feedback. This not only enhances the quality of evaluations but also fosters a culture of continuous learning within teams, ultimately empowering them to achieve excellence in customer service.
- Key functionalities.
The key functionalities of cutting-edge QA AI evaluation systems play a crucial role in enhancing the efficiency and effectiveness of quality assurance teams. Firstly, these systems excel in real-time data analysis, allowing teams to monitor interactions and performance continuously. This immediacy helps identify trends or issues, facilitating proactive measures rather than reactive fixes.
Additionally, automated reporting capabilities significantly reduce the time spent on manual evaluations. AI systems can generate insightful reports that highlight key performance indicators and trends effectively. These reports guide decision-making processes, enabling teams to focus on areas needing improvement and thus enhance overall service quality.
Moreover, the integration of AI-driven insights allows for benchmarking against standards or targets. This ensures that quality assurance efforts align closely with organizational goals. By harnessing these key functionalities, QA teams can achieve a level of precision and responsiveness that was previously unattainable.
- Benefits for QA teams.
The adoption of cutting-edge QA AI has immense benefits for QA teams. By streamlining processes, these advanced systems enhance accuracy and productivity. They minimize human error through automated evaluations, leading to more consistent testing outcomes. This reliability fosters stronger team confidence, enabling them to focus on higher-level insights rather than repetitive tasks.
Additionally, cutting-edge QA AI tools provide real-time data analysis, offering immediate feedback that allows teams to adapt swiftly. The automated reporting features not only save time but also present insights in user-friendly formats. The integration of these tools enhances collaboration among team members, ensuring everyone is aligned on project goals. Ultimately, these benefits result in shorter development cycles and improved product quality, contributing to the overall success of the organization. By embracing these technologies, QA teams can significantly elevate their performance and strategic agility.
Tool B
Tool B stands out as a pivotal component in the realm of cutting-edge QA AI. It offers unique features designed to streamline quality assurance processes effectively. The integration capabilities of Tool B are particularly noteworthy; it seamlessly interfaces with existing systems, enabling effortless data flow and communication among diverse platforms. This integration fosters collaboration within QA teams, enhancing productivity and efficiency.
Moreover, Tool B prioritizes user experience, providing an intuitive interface that simplifies the navigation of its advanced functionalities. With features such as automated reporting and real-time data insights, QA specialists can make informed decisions quickly. As organizations aim to enhance their quality assurance efforts, adopting Tool B can significantly contribute to achieving a more cohesive and efficient QA operation. Embracing these cutting-edge QA AI innovations empowers teams to address challenges while maintaining high standards in their products or services.
- Unique features.
The unique features of cutting-edge QA AI systems set them apart in a landscape increasingly crowded with automation solutions. First and foremost, these systems offer real-time data analysis, enabling teams to receive immediate insights. This immediacy allows QA teams to identify issues as they arise, rather than relying on post-analysis, which can delay necessary actions. Moreover, advanced AI systems enhance the collaboration process within QA teams by consolidating data scattered across numerous files and providing a centralized platform for insights.
Additionally, automated reporting capabilities greatly reduce the time spent on manual documentation, allowing teams to focus more on actionable strategies. The integration of machine learning algorithms allows these systems to learn over time, improving accuracy and efficiency with every evaluation. These features not only streamline workflows but also empower QA teams to make data-driven decisions swiftly, ultimately enhancing product quality and customer satisfaction.
- Integration capabilities.
Integration capabilities play a pivotal role in maximizing the potential of cutting-edge QA AI tools. By seamlessly connecting with existing systems, these advanced AI solutions enhance data flow, ensuring comprehensive insight generation. Effective integration facilitates the synchronization of various quality assurance processes, such as monitoring customer service interactions and analyzing performance metrics. This allows QA teams to deliver consistent and timely feedback, directly influencing improvements in service quality.
Moreover, the adaptability of these AI systems to integrate with databases, CRM platforms, and communication tools expands their functionality. The resultant ecosystem enhances the team's ability to assess call quality and align customer inquiries with organizational offerings. By harnessing these integration capabilities, QA teams can foster a more efficient workflow, leverage actionable insights, and drive continuous improvement. This interconnected approach ultimately transforms how organizations assess and elevate their quality assurance standards, empowering them to stay ahead in a competitive landscape.
Extract insights from interviews, calls, surveys and reviews for insights in minutes
Implementing Cutting-edge QA AI Systems
Implementing cutting-edge QA AI systems requires a strategic approach to ensure seamless integration into existing workflows. The first step involves identifying the specific needs of your QA team. By assessing pain points and desired outcomes, you can select the right AI tool that aligns with your objectives. Itโs crucial to choose a platform that offers features such as real-time data analysis and automated reporting, which can significantly enhance efficiency.
Additionally, training team members on how to effectively use these AI systems is essential. This training fosters familiarity with the tools and encourages collaboration. Once implemented, measuring the impact of these systems is vital. Setting clear KPIs will allow you to analyze the data collected and drive continuous improvements, ensuring that your QA processes evolve alongside advancements in technology. By actively managing these aspects, your team can fully realize the potential of cutting-edge QA AI.
Steps to Integrate Advanced AI in QA Workflows
Integrating cutting-edge QA AI into workflows requires a structured approach to ensure maximum efficacy. Begin by clearly identifying your team's needs, which can vary based on the complexity and scope of your quality assurance tasks. Gather input from all stakeholders to understand their challenges and aspirations, aligning them with the capabilities of AI solutions.
Next, select the right tool that matches these needs, considering factors such as scalability, user-friendliness, and specific functionalities. Once you've chosen a tool, invest time in training your team; familiarity with the technology will enhance their ability to leverage AI effectively. An ongoing support structure for staff, including resources and guidance, will further ease this transition and foster a culture of continuous improvement. By taking these measured steps, your team can successfully integrate advanced AI into QA workflows and reap significant benefits.
- Identifying team needs.
Identifying team needs is essential in the journey toward implementing cutting-edge QA AI systems. It begins with understanding the unique challenges your team faces, whether they stem from workflow inefficiencies, communication gaps, or the volume of data to be managed. Involving team members in discussions helps pinpoint specific areas where advanced AI can streamline processes and improve outcomes.
Next, consider the capabilities your team lacks. Analyzing existing tools and workflows enables you to identify opportunities for automation and insights generation. By defining measurable goals, such as reducing manual reporting time or increasing customer satisfaction, you can clearly articulate what AI solutions would benefit your setup the most. This comprehensive evaluation ensures that any new system integrates smoothly, addressing your team's specific pain points and enhancing performance in the long run.
- Selecting the right tool.
Selecting the right tool for your quality assurance team is a pivotal step towards achieving excellence. With various cutting-edge QA AI systems available, teams must first identify their specific needs. Start by evaluating the scale of the operations, the volume of data you handle, and the nature of the compliance requirements. Understanding these parameters allows for a more targeted approach in selecting the right tool.
Next, consider the integration capabilities of each tool. Ensure that the chosen system can seamlessly interact with your existing processes and frameworks. It should also provide user-friendly interfaces to enhance team collaboration. Additionally, review the support provided, including training resources to help your team adapt swiftly. By carefully analyzing these factors, you can confidently select a cutting-edge QA AI solution that enhances your team's effectiveness and drives continuous improvement.
- Training team members.
Training team members is a critical aspect of successfully implementing cutting-edge QA AI systems. For teams to effectively utilize these advanced tools, training must be comprehensive and tailored to specific roles within the organization. This training should not only cover the functionalities of the AI tools but also the underlying principles of quality assurance and data analysis. Engaging team members through hands-on experiences can significantly enhance their understanding and confidence in using these systems.
To ensure effective training, consider these key strategies:
- Customized Learning Paths: Design distinct training modules for different roles, ensuring that each team member receives relevant instruction.
- Hands-on Workshops: Incorporate practical sessions where team members can experiment with the AI systems, helping them to grasp complex concepts.
- Continuous Support: Establish resources such as manuals and FAQs, and create a support system for ongoing questions and challenges.
- Feedback Mechanism: Regularly solicit feedback on training effectiveness and make iterative improvements.
By focusing on these strategies, you equip your team to harness the full potential of cutting-edge QA AI, thereby maximizing quality assurance outcomes.
Measuring the Impact of AI Implementation
To effectively assess the success of AI implementation in quality assurance environments, it's crucial to measure its impact continually. First, define key performance indicators (KPIs) tailored to your QA goals. These could include metrics such as error reduction rates, processing speed, and user satisfaction scores. By establishing these benchmarks upfront, you create a framework for evaluating improvements over time.
Next, analyze the data collected from your cutting-edge QA AI tools. This analysis should focus on identifying trends, gauging performance against the set KPIs, and recognizing areas for further enhancement. Gathering insights into how automated reporting and real-time data analysis influence your workflows will provide valuable feedback for continuous improvement. Ultimately, the success of AI implementation rests on the ability to adapt strategies based on empirical evidence gathered during this ongoing evaluation process.
- Setting KPIs.
In any QA team utilizing cutting-edge QA AI, setting KPIs is essential for evaluating progress and success. Start by defining specific, measurable, and relevant metrics that align with your organizationโs goals. Consider incorporating metrics such as response time, the accuracy of issue detection, and the efficiency of training programs. This ensures your KPIs address both quantitative and qualitative aspects of performance.
Next, continuously monitor these KPIs over time for actionable insights. Track trends in performance and adjust strategies as needed. For example, if the data indicates a need for improved training on certain problems, adjust your training focus accordingly. Using cutting-edge QA AI for this purpose not only enhances data tracking but also provides insights that traditional methods may overlook, leading to a truly informed approach to quality assurance.
- Analyzing the data for continuous improvement.
Analyzing the data for continuous improvement involves a systematic approach that empowers QA teams to refine their processes. Cutting-edge QA AI plays a crucial role in transforming raw data into actionable insights, allowing teams to identify patterns and trends. By employing advanced analytics, teams can discern what works effectively and what requires attention in their workflows.
To enhance continuous improvement, consider these key actions:
Identify Key Metrics: Focus on metrics that directly impact quality outcomes. Examples include defect rates and customer feedback scores.
Monitor Trends Over Time: Use AI tools to track changes in metrics over different time periods. This analysis helps in identifying recurring issues or improvements.
Implement Feedback Loops: Create systems for gathering ongoing feedback from team members and stakeholders. This input can guide the evolution of workflows and project strategies.
Test and Iterate: Regularly experiment with new approaches based on data insights. Iteration fosters innovation and aligns efforts with user needs.
By effectively analyzing data, QA teams can create a culture of continuous improvement, driving better quality assurance through informed decision-making.
Conclusion: Leveraging Cutting-edge QA AI for Enhanced Outcomes
Integrating cutting-edge QA AI into quality assurance workflows significantly enhances performance and outcome reliability. These sophisticated systems provide unparalleled insights through real-time data analysis and automated reporting, fostering a culture of continuous improvement within QA teams. The ability to assess individual performance metrics ensures that teams can quickly identify areas for growth, streamlining operations and driving overall efficiency.
Adopting these innovative tools empowers organizations to respond to challenges effectively and remain competitive in today's fast-paced environment. By embracing cutting-edge QA AI, teams not only achieve better compliance but also cultivate a proactive approach to quality assurance, ultimately leading to improved outcomes and higher customer satisfaction.