Forecasting accuracy benchmarking is a critical process in the world of AI reporting models. By assessing how well an AI system predicts outcomes, organizations can significantly enhance their decision-making processes. In today's data-driven environment, the importance of precise forecasting cannot be overstated, as accurate predictions directly influence strategic planning and resource allocation. Achieving high forecasting accuracy benchmarking involves understanding the specific criteria that define success in your context.
Employing AI in forecasting provides numerous advantages, including the ability to analyze large datasets efficiently. AI models, when properly calibrated, can deliver real-time insights, thus enabling organizations to react promptly to market changes. Understanding how to benchmark forecasting accuracy ensures that AI tools remain effective and aligned with business objectives, ultimately leading to enhanced performance and competitive advantage.
Analyze qualitative data. At Scale.
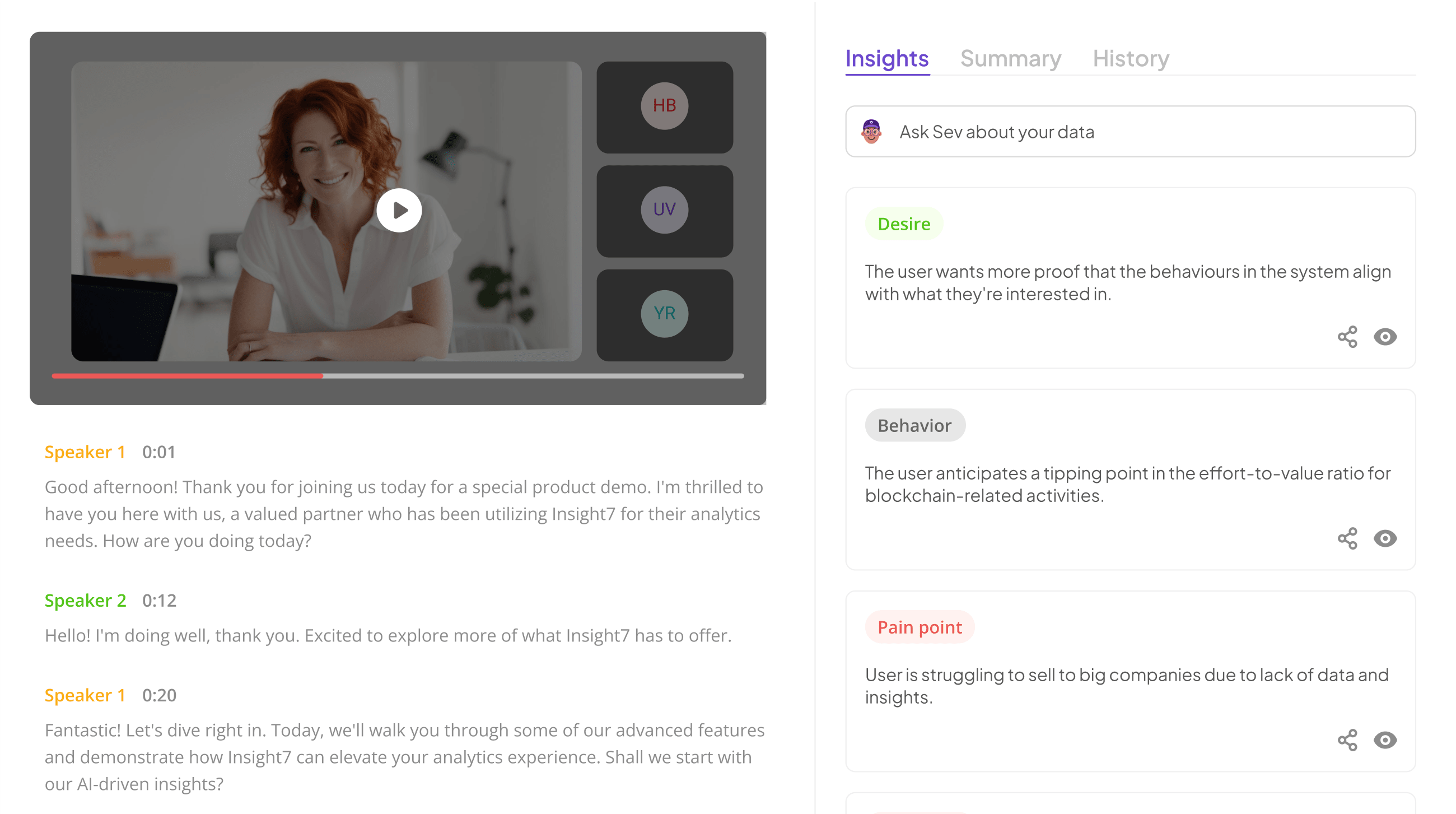
Understanding the Importance of Forecasting Accuracy Benchmarking
Forecasting Accuracy Benchmarking serves as a critical cornerstone for organizations aiming to enhance their predictive capabilities. By establishing benchmarks, businesses can evaluate their forecasting processes and identify areas needing improvement. This ongoing assessment is essential as it not only promotes accountability but also fosters data-driven decision-making that drives growth.
Understanding the nuances of Forecasting Accuracy Benchmarking allows teams to enhance their operational strategies significantly. Organizations using AI-powered tools can automate and refine this benchmarking process, making it faster and more accurate. Itโs vital to note that a robust benchmarking framework integrates historical performance data and current market conditions, paving the way for improved forecasting accuracy in future projections. Ultimately, prioritizing accurate forecasting can lead to better resource allocation, optimized inventory levels, and increased customer satisfaction.
What is Forecasting Accuracy Benchmarking?
Forecasting Accuracy Benchmarking refers to the process of evaluating a forecast's precision against established standards or metrics. Effective benchmarking allows organizations to understand their forecasting performance relative to past predictions, industry averages, or best practices. By systematically assessing accuracy, companies can identify gaps and areas for improvement, leading to more reliable forecasting models.
The integration of AI in this process enhances the benchmarking experience. AI algorithms can analyze vast amounts of historical data, revealing patterns and trends that manual methods may overlook. Moreover, AI allows for real-time adjustments, enabling forecasts to adapt based on incoming data. In essence, forecasting accuracy benchmarking empowers organizations to improve decision-making through a more refined and precise forecasting approach.
The Role of AI in Enhancing Forecasting Accuracy
Artificial intelligence plays a transformative role in enhancing forecasting accuracy. By utilizing advanced algorithms and data analysis techniques, AI can process vast amounts of historical data, revealing patterns that traditional methods may overlook. This capability allows organizations to create more reliable predictions, leading to smarter decision-making. As businesses continuously seek to improve their forecasting accuracy benchmarking, integrating AI into their models becomes essential.
AI not only improves prediction accuracy but also adapts to changing market conditions in real time. Machine learning models can learn from new data, constantly refining their forecasts and thus increasing their validity. Automated processes reduce human errors and biases, resulting in a more objective approach to forecasting. Ultimately, AIโs significant contributions to forecasting accuracy benchmarking position it as an indispensable tool for businesses aiming to stay competitive in an evolving marketplace.
Extract insights from interviews, calls, surveys and reviews for insights in minutes
Key Steps for Effective Forecasting Accuracy Benchmarking Using AI Models
To effectively conduct forecasting accuracy benchmarking using AI models, itโs essential to follow specific steps diligently. Start by selecting the right AI tools. Popular options such as Prophet, TensorFlow, and AutoTS offer varying functionalities that can enhance forecasting performance. Next, ensure you establish clear benchmarking criteria. These standards will guide your evaluation and allow for meaningful comparisons.
After setting the criteria, focus on data collection and preparation. Comprehensive datasets enhance the accuracy of your models. Once the models are trained, analyze and interpret the results meticulously. This involves understanding how different factors influence forecasting accuracy. By following these key steps, organizations can achieve reliable forecasting benchmarks that can drive informed decision-making and resource allocation.
Step 1: Selecting the Right AI Tools for Forecasting
When embarking on the journey of forecasting accuracy benchmarking, the first step involves selecting the right AI tools tailored for your unique needs. A thoughtful selection process enhances the forecasting capabilities significantly, allowing for more accurate predictions. It's crucial to evaluate tools like Prophet, TensorFlow, and AutoTS based on their features, scalability, and ease of use.
Begin by identifying the specific requirements of your forecasting project. Consider factors such as the volume of data and the complexity of models needed. Once you understand your goals, compare different AI tools against these criteria. Testing a few options through pilot projects can provide insightful feedback, helping you finalize the tool that best fits your forecasting accuracy benchmarking needs. This foundational step sets the stage for building robust forecasting models that contribute to informed decision-making and strategic planning.
- insight7
In the realm of Forecasting Accuracy Benchmarking, understanding how various AI models perform is crucial. By systematically evaluating different forecasting tools, organizations can identify which models best meet their unique needs. These benchmarks provide a framework for assessing the precision of predictions against actual outcomes, allowing enterprises to refine their approaches over time.
To effectively benchmark forecasting accuracy, there are several key considerations. First, standardize the metrics you'll use to evaluate model performance, such as mean absolute error or root mean square error. Next, identify a diverse set of historical data for testing purposes. This will ensure that the benchmarks are robust and account for variability in data. Lastly, continually revisit your benchmarks and adjust them as your understanding of the data and AI models evolves. Regular reviews will keep your forecasting methods aligned with organizational goals and market conditions.
- Prophet
Prophet
Prophet is an innovative tool designed for forecasting time series data, particularly effective in identifying trends and seasonal patterns. It excels at making accurate predictions, which is essential for effective forecasting accuracy benchmarking. Users can input historical data, and Prophet automatically generates a model that adapts to unique fluctuations in the data. This adaptability serves businesses well, particularly those with fluctuating demand or diverse seasonal effects.
To effectively utilize Prophet for forecasting accuracy benchmarking, consider the following key aspects:
Data Preparation: Ensure your time series data is clean and formatted correctly for optimal results. Cleaning the data enhances model accuracy.
Model Selection: While Prophet is a strong option, evaluating alternatives like TensorFlow or AutoTS may provide insights into different capabilities in various scenarios.
Validation of Results: After creating forecasts, validate the accuracy against actual outcomes to refine your benchmarking process. Regularly updating the models with new data will maintain their relevance and precision.
By integrating Prophet into your forecasting strategy, you gain a powerful ally in improving accuracy while making informed, data-driven decisions.
- TensorFlow
TensorFlow stands out as a powerful tool for conducting Forecasting Accuracy Benchmarking. Its flexibility allows users to build sophisticated machine learning models tailored for forecasting tasks. By leveraging TensorFlowโs capabilities, data scientists can capture complex patterns in historical data, which significantly enhances the accuracy of predictions. This approach facilitates a deeper analysis of performance metrics, enabling teams to refine their strategies over time.
When using TensorFlow, ensure that key components are considered for effective benchmarking. First, choose appropriate architectures like recurrent neural networks or long short-term memory networks, as they are well-suited for time-series data. Next, utilize TensorFlow's extensive libraries for optimizing models, allowing for fine-tuning that optimizes forecasting accuracy. Finally, visualize the results through TensorFlowโs built-in tools to compare predictions against actual outcomes, providing clarity on areas needing improvement. This structured approach fosters not only reliable forecasting models but also cultivates continuous learning within organizations.
- AutoTS
AutoTS is an innovative tool designed to automate time series forecasting processes, making it a vital asset in forecasting accuracy benchmarking. Users can benefit from its ability to effortlessly test multiple models and select the most accurate one suited for their data. With AutoTS, forecasting becomes simpler and more efficient, allowing businesses to focus more on analyzing results and less on tedious setup.
This tool operates by generating models from the data provided, enabling real-time analysis and comparison. AutoTS can evaluate different forecasting algorithms automatically, ensuring that users achieve the best predictions possible. Enhanced accuracy in results leads to better decision-making, ultimately positively impacting business performance. By streamlining the modeling process, AutoTS plays a critical role in the broader context of AI reporting models and drives effective forecasting accuracy benchmarking.
- TimeGAN
TimeGAN is a generative adversarial network specifically designed for time-series data. It presents a groundbreaking approach to generating synthetic time-series data while capturing temporal dependencies in datasets. This model is particularly beneficial for forecasting accuracy benchmarking because it enables users to create realistic, yet artificial datasets to test forecasting models.
The algorithm consists of a generator and a discriminator, which work against each other to improve data generation continuously. By leveraging TimeGAN, researchers and practitioners can simulate various scenarios and assess how different forecasting models perform against these generated datasets. This process not only enhances model evaluation but also provides insights into the robustness and reliability of forecasting techniques. Ultimately, utilizing TimeGAN for forecasting accuracy benchmarking can lead to improved predictions and greater confidence in decision-making based on these forecasts.
Step 2: Setting Up Benchmarking Criteria
Establishing effective benchmarking criteria is crucial in your journey toward achieving accurate forecasting. This step involves clearly defining the parameters and metrics that will serve as your standard for evaluating performance. Begin by selecting key performance indicators (KPIs) related to forecasting accuracy, such as mean absolute error or root mean square error, which will allow you to quantify your results effectively.
Next, ensure that these criteria are relevant to your specific business context and objectives. Tailoring your benchmarks to reflect the unique aspects of your market will provide a more realistic evaluation of your forecasting models. It is essential to document these criteria comprehensively, as this transparency will help maintain consistency throughout your benchmarking process. By establishing solid forecasting accuracy benchmarking practices, you'll be positioned to make informed adjustments that enhance your AI reporting models.
Step 3: Analyzing and Interpreting Benchmarking Results
Analyzing and interpreting benchmarking results is crucial for understanding your forecasting accuracy. Begin by examining key metrics derived from your model outputs, such as mean absolute error (MAE) and root mean square error (RMSE). These metrics offer quantitative insights into how well your model predicts outcomes compared to actual results. Tracking these figures over time helps you identify patterns in forecasting accuracy, which enables more informed decisions about model adjustments.
Furthermore, it is essential to contextualize these metrics within the benchmarks you established. Compare your results against industry standards or historical data to assess how well your models perform. Insights should be documented, allowing for a narrative that reveals strengths and weaknesses in your forecasting strategy. By synthesizing this information, you can refine your techniques and adapt future models for improved accuracy. Ultimately, this step empowers you to develop more reliable forecasting methods, thus enhancing decision-making processes.
Conclusion: Final Thoughts on Forecasting Accuracy Benchmarking
In conclusion, mastering forecasting accuracy benchmarking is crucial for organizations seeking to enhance their predictive capabilities through AI reporting models. By utilizing these benchmarks, teams can identify strengths and weaknesses in their forecasting processes, enabling data-driven decisions for improvement.
Furthermore, consistent evaluation through robust AI tools allows businesses to adapt to ever-changing market conditions. As forecasting models evolve, maintaining a commitment to accuracy ensures that organizations remain competitive and agile. Ultimately, effective forecasting accuracy benchmarking fosters innovation and empowers stakeholders to make informed choices based on reliable data insights.