How to Prioritize QA Reviews Based on Predictive Sentiment Analysis
-
Bella Williams
- 10 min read
Sentiment-Based QA Prioritization transforms how organizations assess quality assurance through the lens of emotional responses. As customer interactions become increasingly complex, understanding sentiment can provide invaluable insights. By identifying positive, neutral, and negative sentiments in interactions, organizations can prioritize QA reviews effectively.
In this introduction, we'll explore the significance of integrating sentiment analysis into QA workflows. This methodology allows teams to focus their efforts where they matter most, ensuring that negative experiences are addressed promptly. Ultimately, Sentiment-Based QA Prioritization not only enhances operational efficiency but also improves overall customer satisfaction.
Analyze & Evaluate Calls. At Scale.
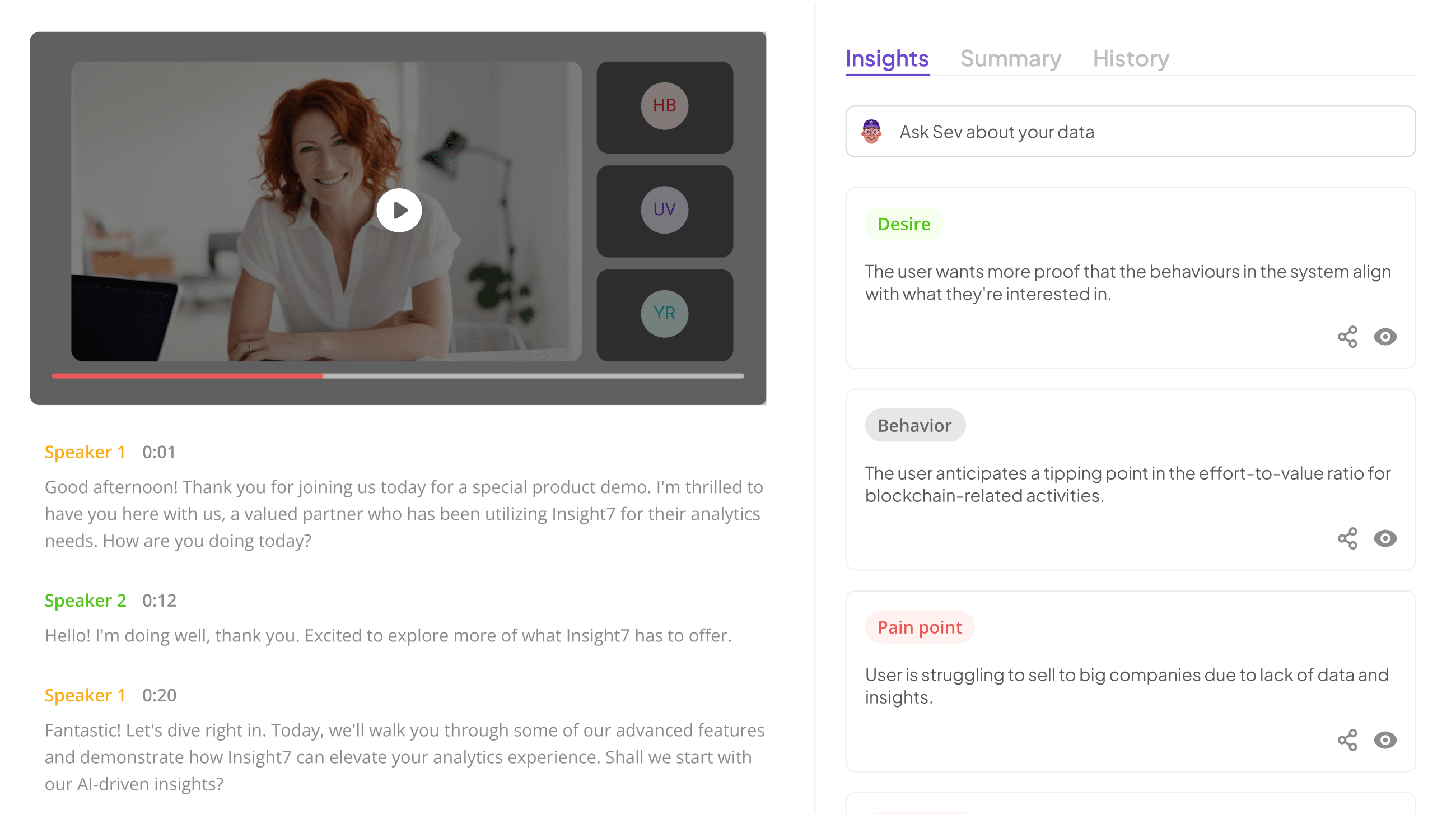
Understanding Sentiment-Based QA Prioritization
Understanding Sentiment-Based QA Prioritization involves recognizing how emotional responses impact quality assurance processes. This approach analyzes customer feedback and sentiments to prioritize QA reviews effectively. By assessing the emotions expressed in interactions, teams can identify areas requiring immediate attention based on the severity of issues raised.
In implementing Sentiment-Based QA Prioritization, itโs essential to define clear criteria for evaluation. Teams should begin by collecting sentiment data from customer interactions. This data can then inform which situations or calls need thorough reviews, ensuring the focus is on high-impact areas. Additionally, employing advanced analytics tools can enhance precision in identifying trends and patterns in customer sentiments that relate to product performance or service delivery. Embracing this data-driven methodology ultimately leads to improved QA outcomes and customer satisfaction.
How Predictive Sentiment Analysis Works
Predictive sentiment analysis operates by examining customer feedback, comments, and reviews to gauge the overall sentiment towards a product or service. By employing advanced algorithms, this method analyzes linguistic patterns within the text, categorizing sentiments as positive, negative, or neutral. This processing enables organizations to uncover hidden insights that might otherwise go unnoticed. With predictive modeling, historical sentiment data can be leveraged to forecast future customer behavior and sentiment trends.
In the context of sentiment-based QA prioritization, the insights gained from this analysis directly inform the quality assurance process. For instance, products receiving consistently negative feedback can be flagged for immediate review, ensuring that serious issues are addressed swiftly. This proactive approach not only enhances customer satisfaction but also streamlines the QA process, prioritizing resources towards areas most likely to impact user experience negatively. Thus, organizations can effectively react to customer sentiment, optimizing their QA efforts based on real-time insights.
Benefits of Implementing Sentiment-Based QA
Implementing sentiment-based QA prioritization brings significant benefits to the quality assurance process. First, it enables teams to identify issues that may negatively impact customer experiences. By analyzing sentiment data, organizations can prioritize reviews based on the emotional tone of customer interactions, helping to address critical pain points more effectively. This targeted approach leads to insights that specifically enhance customer satisfaction and loyalty.
Second, sentiment-based QA prioritization allows for efficient resource allocation. Instead of evaluating all interactions equally, teams can focus on those that exhibit strong negative sentiment, thereby maximizing quality improvements where they are needed most. Additionally, this strategic prioritization fosters a proactive culture within teams, encouraging continuous improvement based on real user feedback. By integrating sentiment analysis into the QA process, companies can significantly enhance their product quality and overall customer experience.
Extract insights from interviews, calls, surveys and reviews for insights in minutes
Implementing Sentiment-Based QA Prioritization
Implementing sentiment-based QA prioritization begins with collecting sentiments from various customer interactions. Utilizing techniques such as text analysis and sentiment scoring, organizations can evaluate customer emotions derived from calls, chats, and emails. By categorizing positive, negative, and neutral sentiments, teams can identify the areas needing immediate attention. This step is crucial as it allows QA evaluators to focus on specific instances that reflect customer dissatisfaction or confusion.
The next phase involves prioritizing the QA reviews based on these sentiment scores. By establishing a scoring system for calls, teams can determine which reviews should receive immediate focus. High-sentiment scores indicate successful interactions that can be further analyzed for best practices, while lower scores highlight calls that require corrective measures. This structured approach to sentiment-based QA prioritization not only streamlines the review process but also enhances overall customer satisfaction and product quality.
Step 1: Collecting and Analyzing Sentiment Data
Collecting and analyzing sentiment data is a critical first step in the process of sentiment-based QA prioritization. To effectively gather this data, one should begin by identifying various sources of customer feedback, such as surveys, reviews, social media, and call transcripts. Each of these sources can offer invaluable insights into customer perceptions and feelings about your product or service. Once you have acquired this data, the next step involves categorizing it based on emotional tone, distinguishing between positive, neutral, and negative sentiments.
Analyzing sentiment data is crucial for understanding customer experiences and anticipating potential QA issues. Advanced analytical tools can help visualize the data, highlighting trends and patterns that may indicate underlying problems. Focus on key metrics that reflect customer satisfaction or dissatisfaction, as these elements will inform your QA review priorities. By systematically collecting and analyzing sentiment data, you can establish a robust foundation for prioritizing reviews and improving overall product quality.
Step 2: Prioritizing QA Reviews Using Sentiment Scores
In Step 2: Prioritizing QA Reviews Using Sentiment Scores, the focus shifts to how sentiment scores can inform and enhance your Quality Assurance (QA) review process. By employing a sentiment-based QA prioritization approach, teams can effectively assess which reviews require immediate attention. This method helps in identifying trends in customer feedback, enabling team members to discern between positive and negative sentiments rapidly.
To implement this prioritization, begin by establishing clear criteria that align with your organization's goals. Consider key factors such as urgency, impact on customer experience, and frequency of specific feedback themes. Next, utilize sentiment scoring tools to analyze calls or reviews against these criteria. As scores are generated, focus your QA efforts on reviews that register higher negative sentiments or critical issues. By concentrating on areas that matter most, teams can improve customer satisfaction and drive substantial improvements in service quality.
Top Tools for Sentiment-Based QA Prioritization
When considering sentiment-based QA prioritization, itโs crucial to employ the right tools to streamline processes effectively. Various platforms specialize in gathering and analyzing sentiment data, leading to better prioritization of QA reviews. Key players like Insight7 and MonkeyLearn offer intuitive interfaces and robust features that cater to businesses of all sizes. These tools automate the analysis of customer feedback in real time, significantly reducing the time required for manual evaluation.
Additionally, Lexalytics, Aylien, and IBM Watson provide advanced sentiment analysis capabilities that can further enhance your QA prioritization strategy. By integrating these tools, you will be able to quantify sentiment scores that dictate which reviews need immediate attention. Ultimately, utilizing these technologies will not only improve QA efficiency but also ensure that you respond proactively to customer concerns, allowing your organization to stay competitive.
๐ฌ Questions about How to Prioritize QA Reviews Based on Predictive Sentiment Analysis?
Our team typically responds within minutes
insight7
To effectively implement Sentiment-Based QA Prioritization, organizations must first understand the underlying principles of predictive sentiment analysis. By gathering and analyzing sentiment data, teams can better gauge customer perceptions and feelings toward their products or services. This process is vital for pinpointing critical areas that require thorough QA reviews, ensuring that the most impactful content receives focused attention.
Next, QA teams should utilize sentiment scores to prioritize their reviews. Higher sentiment scores indicate areas where enhancing quality can lead to improved customer satisfaction and loyalty. By addressing the insights derived from sentiment analysis, teams can streamline their processes, minimize delays, and enhance the overall customer experience. Ultimately, using sentiment analysis for QA prioritization creates a more responsive and adaptive review system, positioning organizations to meet customer needs effectively and efficiently.
MonkeyLearn
In the quest for effective Sentiment-Based QA Prioritization, advanced tools play a crucial role. One such tool simplifies the process of gathering and interpreting customer feedback. Users can easily upload various files, including recorded calls, to analyze and derive meaningful insights. The platform's intuitive interface allows anyone in the organization to engage with the data without prior training. This pushes the boundaries of data accessibility and democratizes insights across teams.
The platform processes interactions to unveil key pain points and customer sentiments, creating a clearer picture of the user experience. Its versatility enables users to summarize large datasets and extract critical insights efficiently. This capability not only enhances the quality of QA reviews but also ensures that the most pressing concerns are prioritized based on actual customer sentiments. By leveraging these insights, teams can focus their efforts more effectively, thereby fostering improved customer satisfaction and operational excellence.
Lexalytics
The role of predictive sentiment analysis in QA processes cannot be overstated. Advanced tools can access and evaluate customer interactions, providing insights that inform QA priorities. This analysis facilitates an understanding of customer sentiment, revealing critical pain points, desires, and overall experiences. By utilizing these insights, organizations can better allocate their resources, focusing on areas with negative sentiment to enhance customer satisfaction.
Incorporating sentiment-based QA prioritization allows teams to act on real-time feedback. The system visually organizes calls and interactions, offering insights into customer experiences. This insight not only highlights problematic areas but also uncovers positive feedback, informing areas of improvement. Ultimately, enhancing product quality and customer satisfaction hinges on effectively prioritizing QA reviews based on these crucial sentiments. This strategic focus can significantly streamline QA efforts and facilitate meaningful improvements across product offerings.
Aylien
Aylien offers powerful tools that enable predictive sentiment analysis, transforming how organizations approach QA prioritization. By generating actionable insights from customer feedback, Aylien allows teams to focus their quality assurance efforts more effectively. Understanding sentiment signals provides clarity on which aspects of the product demand immediate review, enhancing the overall user experience.
Incorporating sentiment-based QA prioritization can significantly streamline processes. First, QA teams can identify high-risk areas based on negative sentiment indicators, facilitating quicker response times. Second, utilizing these insights promotes collaboration across departments, ensuring that essential feedback does not get lost in scattered documents. Lastly, predictive sentiment analysis empowers organizations to maintain competitiveness, adapting to customer needs in real time. Emphasizing these strategies can lead to better product quality and higher customer satisfaction.
IBM Watson
IBM Watson plays a significant role in sentiment-based QA prioritization by utilizing advanced predictive analytics. This AI-driven solution processes vast amounts of data, identifying patterns and sentiments in customer feedback and interactions. By carefully analyzing this information, organizations can gauge the emotional tone of customer communications and determine their impact on quality assurance reviews.
To effectively implement this technology in your QA processes, consider the following steps:
Data Integration: Connect various data sources, including customer feedback and interaction records, to enable a comprehensive analysis.
Sentiment Analysis: Use Watsonโs sentiment analysis capabilities to evaluate customer sentiments accurately, categorizing them as positive, neutral, or negative.
Prioritized Insights: Based on the sentiment scores derived, prioritize QA reviews, focusing on areas that require immediate attention.
By harnessing sentiment-based insights, organizations can make informed decisions to improve customer experience and enhance quality standards.
Conclusion: Enhancing QA Efficiency Through Sentiment-Based Prioritization
In conclusion, enhancing QA efficiency through sentiment-based prioritization offers a powerful approach for streamlining review processes. By analyzing customer sentiment, teams can identify which areas require immediate attention, thus focusing resources where it matters most. This strategic alignment not only improves the quality of reviews but also fosters a better understanding of customer needs.
Implementing sentiment-based QA prioritization empowers organizations to make informed decisions. By utilizing predictive sentiment analysis, teams can effectively reduce time spent on less critical areas, allowing for a more targeted approach. Ultimately, embracing this method can lead to improved product quality and heightened customer satisfaction.
๐ฌ Questions about How to Prioritize QA Reviews Based on Predictive Sentiment Analysis?
Our team typically responds within minutes