Sentiment Analysis Implementation is a crucial step in understanding customer sentiments within AI workflows. By harnessing the power of AI, businesses can transform unstructured text data into actionable insights. This approach allows organizations to pinpoint customer attitudes, preferences, and pain points, leading to enhanced decision-making processes.
In this section, we will explore the methodologies for effective sentiment analysis implementation, from data preparation to integration within existing applications. Understanding the various AI tools available is essential for building robust sentiment detection models, ensuring that businesses can respond dynamically to customer feedback and market trends. As we delve into implementation techniques, prepare to unlock the potential of sentiment analysis in optimizing your AI workflows.
Analyze qualitative data. At Scale.
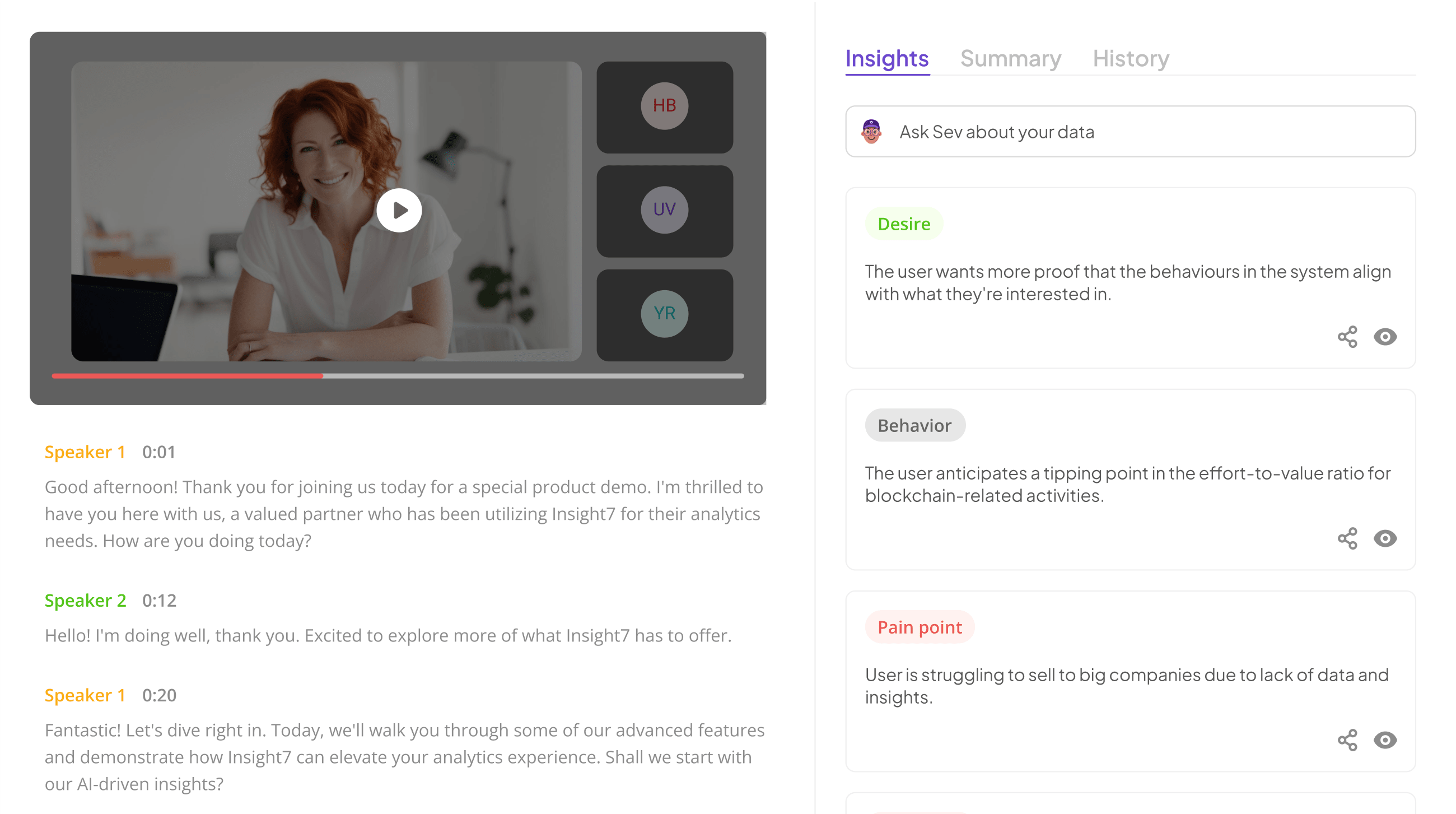
Setting Up Your Sentiment Analysis Implementation Environment
To successfully set up your sentiment analysis implementation environment, it's vital to establish a solid foundation. Start by ensuring you have access to appropriate data sources to inform your sentiment analysis. Collect diverse datasets that represent various sentiments, including positive, negative, and neutral feedback. This initial step lays the groundwork for accurate sentiment detection and analysis.
Next, focus on cleaning and preprocessing the data. Remove any irrelevant information and standardize formats to enhance the quality of your datasets. Once this is done, organize and label your data effectively for easier analysis. This process can significantly impact the efficiency of your sentiment analysis implementation, making it easier to draw reliable insights from the data you collect. By taking these steps, you create a robust environment that supports effective sentiment detection workflows powered by AI tools.
Step-by-Step Guide to Preparing Your Data
Preparing your data is crucial for successful sentiment analysis implementation. Start by collecting relevant data sources such as conversation transcripts, social media posts, or customer feedback. Once gathered, cleaning the data is essential to remove noise and irrelevant details. This process involves correcting inconsistencies, removing duplicates, and filtering out extraneous information, ensuring that the dataset is both comprehensive and accurate.
Next, labeling your data for sentiment detection is fundamental. Assign sentiment tagsโsuch as positive, negative, or neutralโto your dataset based on context and intent. This often involves some manual effort or the use of automated tools to ensure accuracy. By meticulously preparing your data in these stages, you set a solid foundation for building an effective sentiment analysis model. A well-prepared dataset enhances model performance, leading to more reliable insights and stronger outcomes.
- Collecting and Cleaning Data
To achieve successful sentiment analysis implementation, careful data collection and cleaning are essential. First, gather relevant data from various sources, such as customer reviews, social media posts, and feedback forms. This diverse dataset can provide crucial insights into overall sentiment and allow your model to recognize patterns in language. However, raw data often contains noise, such as irrelevant content, which can hinder analysis.
Once the data is collected, the next critical step is cleaning it to ensure accuracy. This process involves removing duplicates, correcting misspellings, and filtering out irrelevant information. You should also consider tokenization, where textual data is split into individual words or phrases. Each of these steps enhances the quality of the dataset, ultimately ensuring that your sentiment analysis implementation is robust and effective. By following these practices, you set a strong foundation for a successful sentiment detection workflow using AI tools.
- Labeling Data for Sentiment Analysis Implementation
Labeling data is a crucial step in sentiment analysis implementation, as it determines the accuracy and effectiveness of the model. To begin, gather a diverse set of text samples from various sources that inherently contain sentiment, such as customer reviews or social media posts. This variety aids in capturing a broad spectrum of emotional expressions. Once collected, each sample should be assigned a sentiment label, typically categorized as positive, negative, or neutral. Consistency in labeling is vital to ensure the model learns effectively.
Next, consider enlisting multiple reviewers to label the data independently. This helps reduce bias and improves the reliability of the labeled dataset. Additionally, incorporating clear labeling guidelines can assist reviewers in making subjective decisions more objective. Following these practices not only enhances the dataset's quality but also lays a strong foundation for effective sentiment analysis implementation, ultimately yielding more insightful results from your AI tools.
Essential AI Tools for Sentiment Analysis Implementation
Choosing the right AI tools for sentiment analysis implementation is crucial for effective sentiment detection workflows. Various solutions cater to different needs, ranging from open-source libraries to robust enterprise-grade platforms. Each tool offers unique capabilities that enhance the analysis of textual data, allowing businesses to glean actionable insights from customer feedback or market research.
Key tools include NLTK and TextBlob, which are great for basic sentiment analysis using simple algorithms. VADER is particularly useful for social media text analysis. More advanced options, like IBM Watson Natural Language Understanding and Google Cloud Natural Language API, provide comprehensive features, including emotion detection. Selecting the appropriate tool depends on your specific requirements, such as data volume and complexity, ultimately determining the success of your sentiment analysis implementation.
- insight7
In the realm of sentiment analysis implementation, understanding the intricacies of customer feedback is vital. By effectively managing the data generated from customer interactions, organizations can gain valuable insights that drive business strategies. Notably, utilizing AI tools allows for efficient processing of this data, transforming raw feedback into meaningful sentiment scores.
To succeed in this endeavor, consider three integral components:
- Data Preparation: The collection and cleaning of data serves as the backbone of sentiment analysis implementation. Ensure your data is accurate and organized to facilitate effective analysis. This process establishes a strong foundation for the subsequent steps.
- Model Selection: Choosing an appropriate sentiment detection model is essential. Your model should be capable of understanding various nuances in customer language, facilitating precise sentiment classification outcomes.
- Integration: Finally, for effective outcomes, seamlessly integrate the model into your applications. This allows for real-time sentiment detection, enhancing user experience and driving actionable insights. By focusing on these components, organizations can streamline their sentiment analysis implementation and respond adeptly to customer needs.
- NLTK
Natural Language Toolkit (NLTK) is a powerful library tailored for processing human language data. It provides essential functionalities for performing tasks like tokenization, parsing, and stemming, which are crucial for sentiment analysis implementation. NLTK offers an assortment of pre-trained models and classifiers that can be fine-tuned to assess sentiment in text effectively. By leveraging NLTK, users can build robust models that identify emotional tones and nuances in customer feedback or social media posts.
To implement NLTK in a sentiment detection workflow, consider the following steps:
Installation and Setup: Begin by installing NLTK and downloading necessary datasets, ensuring you have the tools needed to perform sentiment analysis.
Data Preparation: Cleanse your data by removing irrelevant information and formatting it for analysis. This involves techniques like lowercasing text, removing stop words, and tokenizing.
Model Training: Utilize NLTK's built-in classifiers to train your sentiment analysis model on annotated datasets. This step is integral to tuning the model for accuracy.
Evaluation and Testing: After training, evaluate your model using validation datasets. Adjust parameters to enhance performance and ensure reliable sentiment predictions.
NLTK's versatility and comprehensive documentation make it a go-to choice for those seeking effective sentiment analysis implementation.
- TextBlob
To employ TextBlob in your sentiment analysis implementation effectively, you must first understand its capabilities and features. TextBlob is a Python library designed for processing textual data, which excels in providing straightforward sentiment analysis. The library simplifies sentiment detection by returning polarity and subjectivity scores, facilitating quick interpretation of sentiments expressed in texts.
When implementing TextBlob, a few steps stand out. First, ensure you have the library installed, which can be done using pip in your Python environment. Next, initiate TextBlob with your text data, allowing the library to parse and analyze it. From there, you can extract sentiment scores. Polarities range from -1 (negative sentiment) to +1 (positive sentiment), while subjectivity scores indicate how subjective or objective the text is. This provides a dual insight into both the sentiment and the emotion expressed, aligning perfectly with the objectives of sentiment analysis implementation. By incorporating TextBlob into your workflows, you enhance the accessibility and effectiveness of sentiment detection processes.
- VADER
VADER, which stands for Valence Aware Dictionary and sEntiment Reasoner, is a powerful sentiment analysis tool specifically designed for social media texts and other short-form content. Its strength lies in its simple and effective approach to determining sentiment polarity, which can be crucial for businesses looking to implement sentiment analysis workflows effectively.
To successfully deploy VADER in sentiment analysis implementation, several key steps are essential. First, ensure you have the necessary Python environment with the NLTK library installed, as VADER is included within this package. Next, prepare your text data by cleaning it, removing noise, and formatting it appropriately. After that, the VADER sentiment analysis model can be easily applied to your text, generating polarity scores for positive, negative, and neutral sentiments. Finally, interpreting the results effectively will allow you to derive actionable insights from the analyzed data. Embracing VADER can enhance your organization's understanding of customer sentiment and improve interaction strategies significantly.
- IBM Watson Natural Language Understanding
IBM Watson Natural Language Understanding offers robust functionalities for sentiment analysis implementation, making it a valuable tool in AI workflows. By processing text data, it identifies the emotional tone behind user-generated content, helping businesses gain insights into customer opinions and attitudes. This capability enables organizations to better understand their audience and enhance engagement strategies effectively.
To leverage this tool, start by integrating the API for seamless sentiment detection within your applications. Once integrated, you can analyze sentiment across various datasets, from customer feedback to social media interactions. Moreover, the system provides detailed reports on sentiment trends, helping you gauge public perception over time. By implementing this technology, businesses can make data-driven decisions, refine their marketing strategies, and ultimately improve customer satisfaction.
- Google Cloud Natural Language API
The Google Cloud Natural Language API offers an effective solution for sentiment analysis implementation within various applications. This API allows developers to harness powerful machine learning algorithms that analyze text and discern sentiments expressed within it. Using the API is straightforward, enabling easy integration into existing systems. Users can quickly send text data to the API and receive insightful analysis in return.
When setting up the API, you'll need to begin by authenticating your project via API keys. Next, develop your application logic to send requests that include the text for analysis. The API not only provides overall sentiment scores but also offers insights into emotional tones, enabling deeper understanding. Implementing sentiment analysis with this tool can transform raw data into actionable insights, enhancing your application's performance and responsiveness to user sentiment. By utilizing the Google Cloud Natural Language API, you can significantly streamline your sentiment analysis implementation process and drive valuable business decisions.
Extract insights from interviews, calls, surveys and reviews for insights in minutes
Designing the Workflow for Sentiment Analysis Implementation
Designing an effective workflow for sentiment analysis implementation is crucial for extracting actionable insights. Start by building a sentiment detection model tailored to your target data. Selecting the appropriate model architecture, such as supervised or unsupervised learning, is essential for capturing nuanced sentiment. Once you choose the architecture, training and testing the model with the prepared data ensures it accurately identifies sentiments expressed in textual inputs.
Next, seamlessly integrate sentiment analysis into your applications. This involves employing API integration to facilitate real-time sentiment detection, which enhances user experience and decision-making processes. Consider various use cases, such as monitoring customer feedback on products or analyzing social media sentiments, to understand how sentiment analysis can inform your business strategies. By designing a comprehensive workflow, you empower your team to leverage sentiment analysis effectively and derive meaningful insights.
Building the Sentiment Detection Model
Building the Sentiment Detection Model involves crucial steps that transform raw data into meaningful insights. Selecting the right model architecture is the first step in this process. Various models, like neural networks or traditional machine learning classifiers, can be employed depending on your data type and volume. Next, the model must be trained using your prepared dataset to recognize patterns of sentiment. This requires ample data and a robust training process to ensure the model learns effectively.
Testing the model with separated data is essential to evaluate its accuracy. Once testing is complete, you can refine the model based on its performance metrics. If results are subpar, adjustments in the training data or model architecture may be necessary. This iterative process is key to achieving an effective sentiment analysis implementation that reliably captures sentiments across different contexts. By investing time in model building and evaluation, your applications can deliver accurate and actionable insights from customer feedback.
- Selecting the Right Model Architecture
Choosing the appropriate model architecture is crucial for successful sentiment analysis implementation. This crucial decision hinges on the requirements of your specific project and the nature of your data. Various architectures, such as Convolutional Neural Networks (CNNs) and Recurrent Neural Networks (RNNs), offer distinct advantages depending on the complexity of sentiment nuances you wish to capture.
Begin by assessing the volume and type of data at your disposal. A CNN may excel with text classification tasks that require an understanding of local features, while RNNs are beneficial for sequential data, enabling the model to learn contextual dependencies. Moreover, Transformer-based architectures like BERT can enhance performance by comprehending deeper linguistic structures. Ultimately, aligning your model architecture with the specific goals of sentiment analysis will pave the way for a robust and accurate workflow.
- Training and Testing the Model with Prepared Data
Training and testing the model with prepared data is a crucial phase in the sentiment analysis implementation process. This phase involves fine-tuning the model to accurately interpret sentiments in various texts. Initially, split your dataset into training and testing subsets to ensure that the model learns effectively from the training data while also being evaluated on unseen data. The training set allows the model to learn patterns, while the testing set assesses its performance on data it hasnโt encountered before.
Once you have completed this step, you will need to assess the model's accuracy. Metrics like precision, recall, and F1 scores are vital for understanding how well your model is performing. Use this evaluation to refine your model further, making adjustments to improve accuracy and reduce errors. By iterating through this process, you ensure that your sentiment analysis implementation delivers reliable and actionable insights.
Integrating Sentiment Analysis into Your Applications
Integrating sentiment analysis into your applications can significantly enhance user engagement and decision-making capabilities. Initially, it's vital to consider API integration, which allows your application to detect sentiment in real-time. Developing an application that utilizes sentiment analysis means users can receive immediate feedback or insights based on emotional cues from text inputs, such as reviews or social media interactions.
Moreover, implementing sentiment analysis can lead to various practical applications. For instance, businesses can analyze customer feedback to identify satisfaction levels and react accordingly. Additionally, monitoring sentiment trends over time helps organizations adapt their strategies to meet customer needs, ultimately fostering stronger connections. The effectiveness of sentiment analysis implementation not only streamlines communication but also personalizes user experiences, making it an invaluable asset in todayโs data-driven landscape.
- API Integration for Real-Time Sentiment Detection
Integrating APIs for real-time sentiment detection is a crucial step in your sentiment analysis implementation. This process allows businesses to derive immediate insights from customer feedback and social media interactions. By connecting your applications to an API, you can automatically analyze opinions and emotions as data is generated, rather than waiting for manual analysis. This leads to quicker responses and adjustments to business strategies, enhancing overall user engagement.
To effectively utilize this integration, follow these key steps. First, select an appropriate sentiment analysis API that suits your needs. Ensure that it can process large volumes of data efficiently. Next, set up authentication to secure your access and data. Finally, implement error handling to manage any discrepancies during the data processing. By ensuring these steps are completed, businesses can streamline their sentiment analysis workflow, significantly improving decision-making processes in real time.
- Use Cases and Practical Applications
Sentiment Analysis Implementation is pivotal in diverse fields where understanding emotional tone is crucial. Organizations utilize this technology to gauge customer sentiment from reviews, social media interactions, and feedback. By analyzing sentiments, businesses can tailor their marketing strategies, enhance customer service, and refine product offerings. For instance, a retail company can identify trends in customer satisfaction or dissatisfaction, enabling rapid responses to emerging issues.
In addition to retail, industries like healthcare and finance also benefit. In healthcare, sentiment analysis enhances patient feedback mechanisms, improving service quality. Similarly, financial institutions analyze sentiments related to market conditions, allowing them to adjust strategies proactively. By deploying AI-powered sentiment detection, organizations can not only automate their data analysis but also derive actionable insights that significantly enhance decision-making processes. Overall, the practical applications of sentiment analysis span multiple sectors, showcasing its importance in todayโs data-driven landscape.
Conclusion on Sentiment Analysis Implementation
Sentiment Analysis Implementation plays a crucial role in understanding customer emotions and feedback. By harnessing AI tools, businesses can shift from merely collecting data to truly comprehending it. Successful implementation not only enhances decision-making but also fosters stronger relationships with consumers.
As organizations increasingly adopt advanced sentiment detection workflows, they should prioritize the continuous refinement of their methodologies. This iterative approach allows businesses to adapt to changing sentiment dynamics and improves the accuracy of insights. By leveraging sentiment analysis, companies can navigate market trends more effectively and respond to customer needs in a timely manner.