Contact center forecasting is essential for optimizing operations and enhancing customer experiences. Understanding how different forecasting models function can significantly impact staffing and resource management. Proper predictions not only help in meeting service levels but also contribute to employee satisfaction by reducing instances of overwork or understaffing.
In this section, we will explore various forecasting models that have proven effective in transforming contact center operational planning. From time series analysis to more advanced machine learning techniques, these models provide valuable insights that enable contact centers to better anticipate customer needs and adapt accordingly. Embracing these methods can lead to improved efficiency, strategic planning, and ultimately, a more responsive customer service environment.
Analyze qualitative data. At Scale.
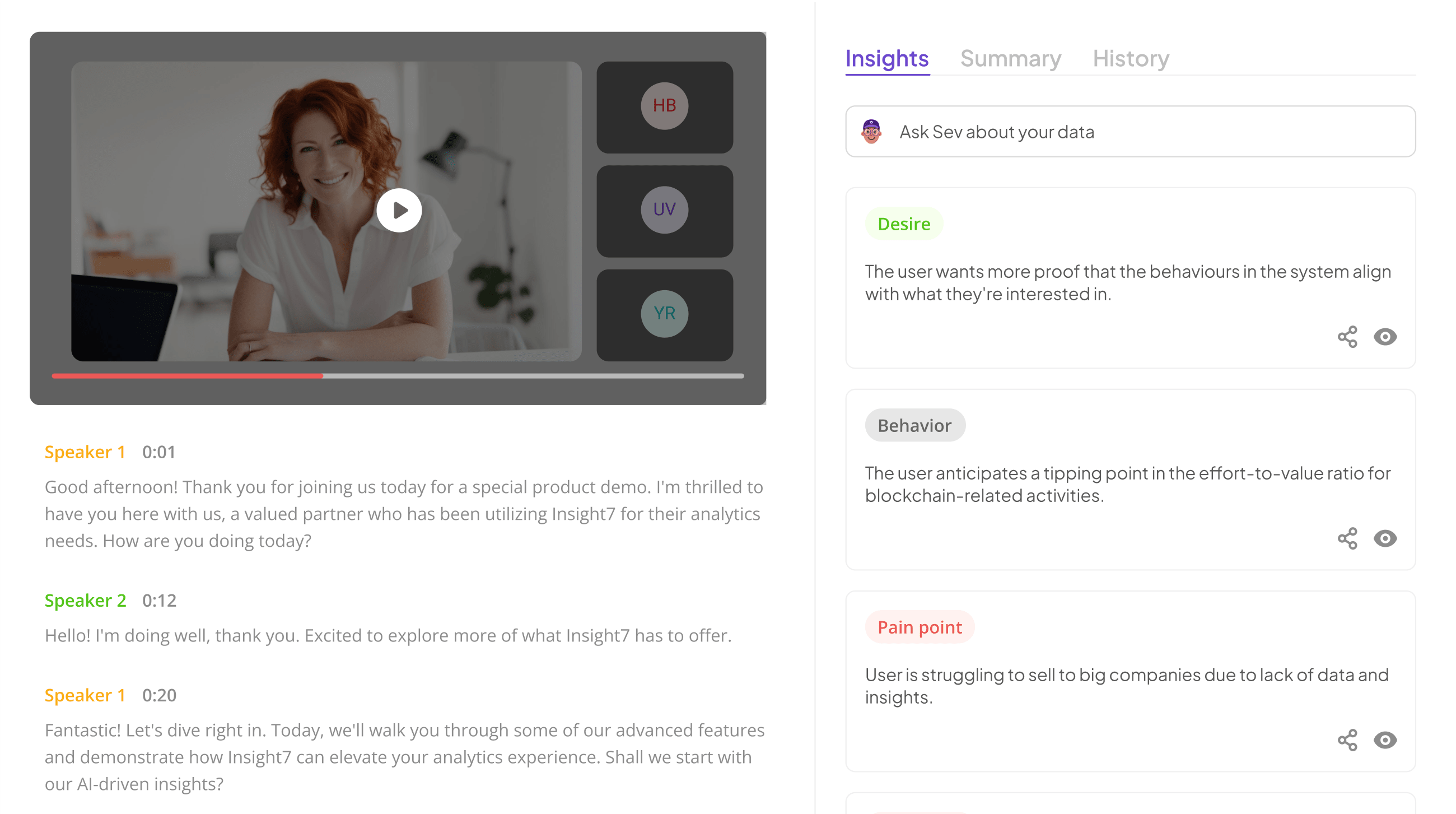
Understanding Contact Center Forecasting Needs
Understanding Contact Center Forecasting Needs involves recognizing the vital role accurate predictions play in optimizing operations. To create a successful forecasting strategy, it is essential to identify unique variables that impact call volumes and customer interactions. These factors might include seasonal trends, promotional campaigns, or changes in customer behavior that directly affect demand.
A well-structured approach begins with gathering historical data, which offers insights into past performance. Analyzing this data helps identify patterns and trends, enabling contact centers to make informed predictions. Additionally, engaging with stakeholders allows for a more accurate reflection of operational needs, aligning workforce management with customer expectations. Ultimately, mastering Contact Center Forecasting equips organizations to enhance resource allocation, reduce costs, and elevate customer satisfaction through timely and informed decision-making.
The Role of Accurate Predictions in Contact Center Operations
Accurate predictions play a crucial role in contact center operations, enabling organizations to optimize their resources and enhance customer satisfaction. By implementing effective contact center forecasting techniques, managers can anticipate call volumes, identify staffing needs, and streamline processes. This foresight significantly reduces both operational costs and customer wait times, creating a win-win scenario for both employees and clients.
In practice, accurate predictions allow contact centers to manage varying demand levels throughout the day or week. Utilizing historical data and advanced forecasting models, decision-makers can determine peak hours and allocate resources accordingly. Furthermore, proactive planning based on reliable forecasts allows teams to identify training needs and performance gaps among customer service representatives. Overall, accurate predictions are essential for improving efficiency and maintaining high standards of service in contact center operations.
Key Metrics for Contact Center Forecasting Success
Understanding the key metrics for successful contact center forecasting is essential for improving operational efficiency. Metrics such as call volume, average handling time, and service level agreements provide insights into customer service performance. By tracking these metrics, contact centers can better anticipate customer demands and optimize staffing levels, ensuring that representatives are available when needed most.
Another crucial metric is the forecast accuracy, which measures how closely actual call volumes align with predictions. High accuracy leads to better resource allocation and improved customer satisfaction. Additionally, monitoring trends in customer behavior, such as peak contact times, can help in adjusting strategies for handling inquiries. By focusing on these key metrics, contact centers can enhance their forecasting capabilities and ultimately achieve greater operational success.
Seven Forecasting Models Transforming Contact Center Planning
To effectively transform contact center planning, implementing specific forecasting models is essential. These seven models provide contact centers with a structured approach to accurately predict demand and optimize resource allocation. By harnessing these forecasting techniques, organizations can enhance operational efficiency, elevate customer service experiences, and ultimately drive business growth.
The first model, Time Series Analysis, utilizes historical data patterns to forecast future traffic volumes. Following this, Machine Learning Models enhance predictions by analyzing complex data sets for deeper insights. Other notable models include ARIMA, which combines autoregressive and moving average components, and Exponential Smoothing, which emphasizes recent data trends. Each of these forecasting methods plays a crucial role in refining contact center operations, ultimately ensuring that agents are available when customers need them most. By adopting these models, firms can stay ahead in a competitive environment, facilitating better responses to ever-changing customer demands.
Extract insights from interviews, calls, surveys and reviews for insights in minutes
Time Series Analysis for Contact Center Forecasting
Time series analysis plays a vital role in contact center forecasting by examining historical data trends. This approach allows organizations to anticipate call volumes, wait times, and resource needs effectively. By systematically analyzing past performance, businesses can optimize staffing and improve customer service levels. Data preparation is the groundwork of this process, ensuring that the information is clean and accurately reflects historical patterns.
Following data preparation, model selection becomes the focus. Various models, including ARIMA and Exponential Smoothing, can capture different patterns in the data. These techniques help forecast future needs while accounting for seasonal fluctuations and trends. Effective contact center forecasting not only enhances operational planning but also strengthens customer satisfaction through timely responses to demand shifts. By integrating insights from time series analysis, contact centers can better prepare for fluctuations in traffic and improve overall efficiency.
Step 1: Data Preparation
Data preparation is a critical first step in contact center forecasting. This stage involves organizing and cleaning the data to ensure accuracy and reliability. The data collected should include various metrics, such as call volumes, customer interactions, and operational performance indicators. Properly structured data allows forecasts to reflect reality, enabling better decision-making.
Next, it's essential to identify and address any gaps or inconsistencies in the data. This requires thorough analysis and possibly enriching the dataset with external factors like seasonal trends or market conditions. Once the data is clean, it can be analyzed to generate insights regarding customer behavior and staffing needs. By preparing the data meticulously, organizations can set a solid foundation for effective forecasting methods, ultimately enhancing contact center performance.
Step 2: Model Selection
Selecting the right model is a crucial step in effective contact center forecasting. Various forecasting techniques offer unique advantages, and understanding these can lead to better operational planning. When determining which model to use, consider factors such as data availability, forecasting horizon, and the specific operational goals of the contact center.
There are several key models to explore. Time Series Analysis is beneficial for identifying trends and seasonal patterns in historical data. Machine Learning Models, on the other hand, can adapt to complex data structures and learn from larger datasets to improve accuracy. Additionally, methods like ARIMA and Exponential Smoothing are particularly useful for incorporating them into broader operational strategies. By assessing the strengths and weaknesses of each approach, managers can make informed decisions that will positively impact overall performance and efficiency within the contact center.
Machine Learning Models for Improved Contact Center Efficiency
Machine learning models significantly enhance the efficiency of contact centers by automating various processes and providing deeper insights. These models analyze historical data to predict call volumes, customer inquiries, and agent performance metrics. With this valuable information, managers can optimize staffing levels and improve training programs tailored to specific customer needs.
One effective approach to improving contact center forecasting involves implementing models like ARIMA and Exponential Smoothing. ARIMA (AutoRegressive Integrated Moving Average) efficiently analyzes time-series data, helping predict future calls by understanding seasonal patterns. Exponential Smoothing, on the other hand, adjusts predictions based on the most recent data trends, providing a more responsive approach to forecasting. Together, these methods enable contact centers to adapt quickly to changing demands, ensuring efficient operations and enhanced customer satisfaction.
Insight7 – Harnessing AI for Better Forecasts
Artificial Intelligence is revolutionizing Contact Center Forecasting by providing precise insights and enhancing decision-making processes. With AI technologies, contact centers can now analyze vast amounts of data more efficiently, leading to improved demand predictions and staffing solutions. This shift is crucial as the volume of customer interactions continues to grow, making traditional forecasting methods inadequate.
AI-driven models can identify patterns and trends in customer behavior that human analysts might miss. These models not only streamline operations but also help in crafting personalized customer experiences by forecasting needs and preferences accurately. By integrating AI into forecasting practices, contact centers can ensure they remain agile and responsive, ultimately leading to heightened customer satisfaction and operational efficiency. The future of Contact Center Forecasting lies in embracing these advanced technologies to stay competitive in today's dynamic environment.
ARIMA Models
ARIMA models, or AutoRegressive Integrated Moving Average models, are pivotal in enhancing contact center forecasting. By analyzing time series data, these models help identify patterns and trends crucial for operational planning. The strength of ARIMA lies in its ability to incorporate historical data, enabling contact centers to predict future demand with greater accuracy.
The ARIMA process is composed of several key steps. First, data preparation involves cleaning and organizing historical call data to ensure its quality. Then, the model selection phase requires choosing the appropriate ARIMA parameters, which include auto-regressive terms, integrated parameters, and moving average terms. Finally, once the model is trained, it can be utilized to generate forecasts that inform staffing and resource allocation, ultimately leading to improved customer service and operational efficiency. Using ARIMA models effectively helps contact centers meet fluctuating demands while maintaining high standards of service.
Exponential Smoothing
Exponential smoothing is a vital statistical technique that enhances contact center forecasting by providing reliable predictions based on historical data. This method applies decreasing weights to older observations while giving more importance to recent data points, making it exceptionally effective for time series data. By focusing on the trends in recent call volumes, contact centers can achieve more nuanced and accurate forecasts.
The effectiveness of exponential smoothing rests on its simplicity and adaptability. Businesses can apply different smoothing constants depending on their specific needs, allowing for levels of smoothing that can be adjusted to match changing contact patterns. For instance, a contact center may choose higher weights for recent data during peak seasons, ensuring that their predictions align closely with actual traffic. Implementing this approach can significantly reduce errors and lead to more informed operational planning, ultimately enhancing customer satisfaction and resource management.
Conclusion: Enhancing Operational Planning with Contact Center Forecasting
In conclusion, effective operational planning in contact centers hinges on the intelligent application of contact center forecasting. By utilizing various forecasting models, organizations can anticipate demand trends, streamline resource allocation, and enhance overall service quality. Implementing these predictive strategies allows for a proactive approach in addressing customer needs and optimizing performance metrics.
Furthermore, contact center forecasting aids in continually refining training programs based on analyzed data. By identifying common customer inquiries, teams can align their training efforts to focus on relevant skills, thus improving customer service representatives' performance. Ultimately, embracing this forecasting approach leads not only to improved operational efficiency but also to greater customer satisfaction.