Predictive CX Optimization in call centers marks a transformative shift in how businesses approach customer interactions. By harnessing the power of AI, organizations can analyze vast amounts of data to identify patterns and enhance overall customer experiences. This proactive approach enables call centers to anticipate customer needs, leading to more efficient service and improved satisfaction.
In today's fast-paced environment, the ability to utilize predictive analytics is crucial. It not only streamlines training processes for customer service representatives but also helps tailor interactions based on customers' preferences. As businesses adopt these innovative strategies, they are poised to revolutionize customer service, significantly reducing response times and elevating the overall experience.
Analyze qualitative data. At Scale.
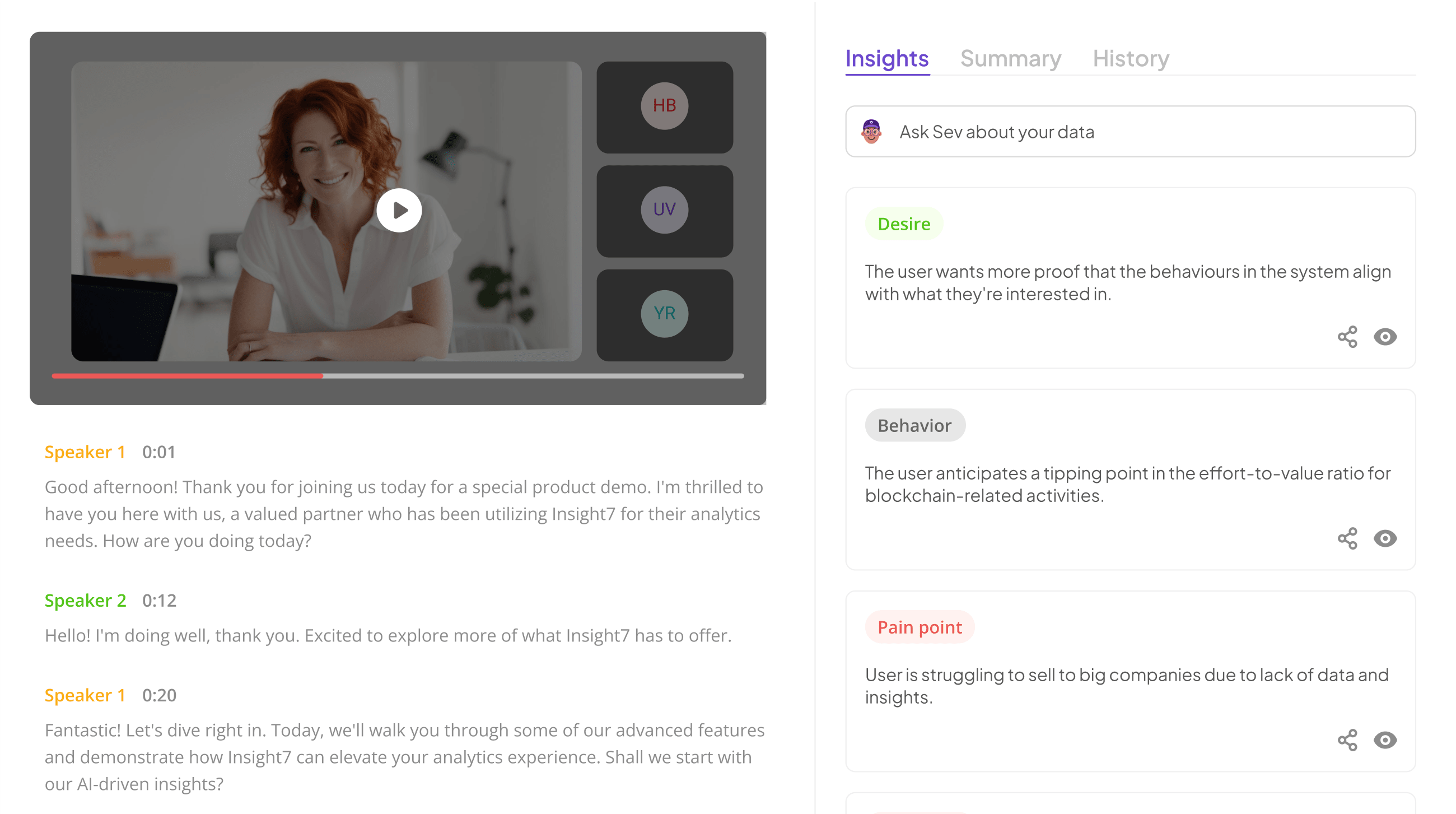
AI-Driven Predictive CX Optimization: Enhancing Customer Experience
AI-Driven Predictive CX Optimization plays a transformative role in enhancing customer experience within call centers. By harnessing the power of data analytics, businesses can anticipate customer needs and tailor interactions accordingly. This proactive approach not only boosts satisfaction but also fosters deeper customer relationships, making them feel valued and understood.
To effectively optimize customer experience, organizations must first identify key data sources and gather actionable insights. Next, leveraging AI algorithms allows for sophisticated predictive modeling that accurately forecasts customer behaviors and preferences. Finally, implementing the strategy and assessing its results is crucial, providing a framework for continuous improvement. This cycle of insight, action, and evaluation leads to refined customer interactions and operational efficiencies, ultimately positioning businesses ahead of their competition in delivering exceptional service.
Understanding Predictive CX Modeling
Predictive CX optimization is a crucial concept in enhancing customer interactions within call centers. This modeling process focuses on utilizing data analytics to interpret customer behavior accurately. By analyzing patterns, organizations can forecast customer needs, which significantly improves service delivery and customer satisfaction.
The first step in understanding predictive CX modeling is recognizing the value of data-driven insights. Call center interactions generate vast amounts of data, capturing everything from call durations to customer sentiments. This information forms the backbone of predictive analytics, allowing businesses to tailor their strategies based on actual customer feedback and trends.
Next, it's essential to identify key components that drive success in predictive modeling. These include effective data collection, advanced AI algorithms, and continuous evaluation of results. With these components in place, organizations can create a responsive and proactive customer experience, ultimately shaping a favorable brand impression. This comprehensive approach to predictive modeling ensures that customer needs are anticipated and met with precision, paving the way for long-term loyalty and engagement.
- The Role of Data Analytics in Predictive Modeling
Data analytics plays a pivotal role in the realm of predictive modeling, directly influencing Predictive CX Optimization. This process involves extracting insights from varied data sources, such as customer interactions and feedback. By analyzing this data, organizations can identify patterns and trends that inform better decision-making, ultimately enhancing customer experiences.
Predictive modeling utilizes advanced algorithms to forecast future customer behaviors and preferences effectively. In the context of call centers, it enables teams to anticipate customer needs before they arise. Understanding these behaviors facilitates proactive engagement strategies, thus improving overall satisfaction. Additionally, integrating AI-driven analytics further amplifies the accuracy of these predictions, transforming raw data into actionable insights that can fuel refined customer interactions and service offerings. By focusing on data analytics, businesses can not only optimize their call center operations but also substantially minimize risks associated with customer disengagement or dissatisfaction.
- Key Components of AI-Powered CX Optimization
To successfully implement AI-powered CX optimization, it is crucial to focus on several key components. These components not only enhance customer experience but also streamline processes within call centers. One major aspect is data analytics, which serves as the backbone for making informed decisions. Understanding customer behavior through data helps to create more personalized interactions, thereby driving customer satisfaction.
Another essential component includes AI algorithms, which play a pivotal role in predictive modeling. These algorithms analyze past interactions to forecast future behaviors and trends. By integrating AI tools that focus on key indicators, call centers can proactively address customer needs. Ultimately, this approach not only strengthens customer relationships but also aids in risk assessment by identifying potential issues before they escalate. Together, these components form the foundation of effective predictive CX optimization.
Steps to Implement Predictive CX Optimization in Call Centers
To effectively implement predictive CX optimization in call centers, it is crucial to adopt a systematic approach. First, identify the data sources that will provide valuable customer insights. This might include customer interaction logs, feedback surveys, and historical call data. Gathering this information is fundamental to understanding customer behavior and preferences.
Next, leverage AI algorithms to analyze the collected data and create predictive models. These models help anticipate customer needs and improve service delivery. Following this, implement the strategy by integrating insights into operational practices while continuously assessing results. This iterative process ensures that your predictive strategies stay relevant and effective. Regular evaluations will not only highlight successes but also identify areas for enhancement, ensuring that your call center remains aligned with customer expectations and experiences. By focusing on these steps, organizations can harness predictive CX optimization to enhance their overall customer service effectiveness.
- Identify Data Sources and Gather Customer Insights
To successfully identify data sources and gather customer insights, itโs essential to start with a comprehensive understanding of available data. Different data sources, such as call transcripts, surveys, social media interactions, and customer feedback, are vital for a holistic view of customer behavior. Integrating these diverse sources enables businesses to capture a wide range of customer sentiments, preferences, and pain points, paving the way for Predictive CX Optimization.
Once the data is collected, analyzing it becomes paramount. By leveraging advanced analytics tools, businesses can identify patterns and trends that highlight customer needs. This process not only informs strategies for improving customer interactions but also supports ongoing adjustments based on real-time insights. Ultimately, gathering and analyzing this data effectively leads to a better understanding of the customer journey, providing valuable insights for risk assessment and targeted improvements in the call center experience.
- Leverage AI Algorithms for Predictive Modeling
AI algorithms play a crucial role in predictive modeling, particularly within call centers. By analyzing historical customer interactions and behaviors, these algorithms can forecast future trends and customer needs. This predictive capability enables organizations to enhance customer experience significantly by anticipating issues before they arise, thus ensuring timely interventions. Integrating predictive modeling helps refine service processes and empowers agents with relevant insights, ultimately leading to improved satisfaction rates.
To maximize the benefits of predictive modeling, consider focusing on the following aspects:
Data Collection and Analysis: Utilize robust data collection methods to gather comprehensive insights. This forms the foundation for accurate predictions and informs your customer experience strategy.
Algorithm Selection: Choose the right algorithms tailored to your specific needs. Whether employing machine learning or statistical models, selecting efficient algorithms is key to achieving accurate results.
Continuous Improvement: Implement feedback loops to refine the predictive models regularly based on new data and changing customer behaviors. This practice ensures that your predictive CX optimization remains relevant and effective.
By prioritizing these elements, organizations can leverage AI algorithms to not only enhance predictive modeling but also pave the way for a remarkable customer experience.
- Implement the Strategy and Assess Results
Implementing the strategy of AI-driven predictive customer experience (CX) optimization requires a structured approach. Firstly, it is crucial to identify relevant data sources and gather insights about your customers. This data will form the foundation for your predictive modeling, allowing for a more tailored customer engagement strategy. Once you have the data, the next step is leveraging AI algorithms for accurate predictions, which can drive proactive customer service enhancements.
After deploying the strategy, assessing results emerges as a vital phase. This involves not only analyzing customer feedback but also evaluating how well the predictive models perform in real-time scenarios. Regularly measuring key performance indicators will help identify gaps and highlight areas for improvement, ensuring that the optimization process remains dynamic and responsive to customer needs. By continuously iterating on this feedback loop, organizations can enhance customer satisfaction and operational efficiency, ultimately achieving meaningful results in predictive CX optimization.
Extract insights from interviews, calls, surveys and reviews for insights in minutes
Tools for AI-Driven Predictive CX Optimization and Risk Assessment
Various tools exist to enhance predictive CX optimization and streamline risk assessment in call centers. These tools utilize advanced analytics to identify customer trends and behaviors effectively. Implementing AI-driven platforms allows for deeper insights into customer experiences, revealing pain points and improving overall satisfaction.
Key tools include comprehensive analytics platforms like insight7, which provide powerful insights and detailed reporting features. Other notable options are Salesforce Einstein, known for its robust CRM capabilities, and Microsoft Dynamics 365, which integrates AI with customer engagement solutions. Additionally, Genesys Cloud offers advanced call center features tailored for predictive analytics, ensuring dynamic responsiveness to customer needs.
To optimize these tools effectively, businesses must customize them to align with their specific requirements and invest in team training. This approach ensures that organizations maximize the potential of predictive CX optimization, enhancing customer experiences while minimizing risks.
Leading Tools for Predictive CX Modeling
In the realm of predictive CX optimization, leading tools play a pivotal role in shaping a call center's operations. These solutions facilitate the analysis of customer data, allowing companies to identify patterns and predict behavior. From comprehensive analytics platforms to dedicated customer service automation, the variety of tools available is designed to streamline processes and enhance customer interactions. Tools like Salesforce Einstein and Microsoft Dynamics 365 integrate seamlessly into existing workflows, empowering teams to harness AI insights effectively.
Another notable option is Genesys Cloud, which specializes in advanced call center solutions that incorporate predictive analytics. This tool analyzes past interactions to anticipate future customer needs, thereby improving engagement strategies. Similarly, Pega and Oracleโs offerings support businesses in managing end-to-end customer experiences through tailored insights. By adopting these leading tools, organizations can optimize their predictive modeling efforts, ultimately leading to improved customer satisfaction and operational efficiency.
- insight7: Comprehensive AI-Driven Analytics Platform
A comprehensive AI-driven analytics platform plays a vital role in optimizing predictive customer experience (CX) in call centers. By harnessing real-time data, these platforms help businesses analyze customer interactions and derive actionable insights. They mitigate the inefficiencies associated with traditional data handling methods, ensuring faster, more accurate decision-making processes. Understanding customer needs in-depth allows companies to enhance their service quality significantly.
Implementing such platforms involves several critical steps. First, businesses must identify relevant data sources to gather customer insights effectively. Next, they utilize advanced AI algorithms to engage in predictive modeling that anticipates customer behaviors. Finally, assessing the results of these strategies is essential to refine approaches over time. By adopting a comprehensive AI-driven analytics platform, organizations can transform scattered data into coherent strategies that foster lasting customer relationships and optimize overall service delivery.
- Salesforce Einstein: CRM and AI-Powered Customer Insights
Salesforce Einstein revolutionizes the way organizations harness customer data, transforming traditional CRM into a powerful tool for predictive CX optimization. By integrating artificial intelligence, businesses can gain deep insights into customer behavior, preferences, and trends. This technology enables the identification of patterns that may not be immediately visible, creating opportunities for enhanced customer engagement and satisfaction.
To effectively utilize these AI-powered customer insights, organizations should adopt a strategic approach. First, they must establish robust data collection practices, ensuring they capture relevant customer interactions. Next, applying AI algorithms can help forecast customer needs and potential issues before they arise. Finally, implementing an actionable strategy based on these insights allows companies to refine their customer experience continuously, ultimately minimizing risks and maximizing satisfaction. This ongoing process empowers call centers to shift from order-taking roles to becoming trusted consultants, leading to more meaningful customer relationships.
- Microsoft Dynamics 365: Integrated AI Solutions for Customer Engagement
Microsoft Dynamics 365 offers a suite of integrated AI solutions, focusing on optimizing customer engagement. By harnessing advanced analytics and machine learning, it enhances predictive CX optimization capabilities. This platform enables businesses to anticipate customer needs and tailor interactions accordingly, enriching the overall customer experience.
With its powerful tools, organizations can analyze vast amounts of data to identify patterns and trends. This allows customer service representatives to be proactive rather than reactive, engaging customers on a deeper level. Rather than waiting for inquiries, they can uncover potential needs and suggest solutions that may not be apparent to the customer. By utilizing these integrated AI solutions, businesses can transform their customer engagement strategies, ultimately driving loyalty and satisfaction.
- Genesys Cloud: Advanced Call Center Solutions for Predictive Analytics
Advanced call center solutions empower businesses to enhance customer experiences through predictive analytics. By analyzing vast amounts of data, these solutions can identify patterns and trends that directly affect customer satisfaction and retention. Understanding customer behaviors allows organizations to shift from merely responding to inquiries to proactively addressing potential concerns and tailoring interactions.
To achieve effective predictive CX optimization, there are several components to consider. First, integrating data analytics is crucial, as it transforms raw data into actionable insights. Next, utilizing sophisticated AI algorithms can refine the predictive modeling process, resulting in a more personalized experience for customers. Finally, continuous assessment of implemented strategies ensures that call centers remain adaptive to evolving customer needs. This approach underscores the importance of dynamic engagement, turning customer interactions into meaningful relationships that drive loyalty and success.
- Pega Customer Service: AI-Powered Customer Service Automation
In today's fast-paced environment, effective customer service automation is crucial for enhancing customer experience. AI-powered customer service solutions facilitate streamlined operations, enabling organizations to optimize their customer interactions. By leveraging automation, businesses can achieve predictive CX optimization that helps anticipate customer needs and deliver timely responses.
Transformative AI technologies analyze vast amounts of customer data, providing insights that drive informed decision-making. These solutions allow organizations to create personalized experiences, leading to improved customer satisfaction and loyalty. Implementing AI-driven automation not only enhances operational efficiency but also empowers agents with valuable insights. As businesses seek to adapt to changing consumer expectations, AI-powered customer service automation becomes essential in delivering exceptional customer experience while proactively addressing potential risks associated with service encounters.
- Oracle CX Cloud: End-to-End CX Management with Predictive Analytics
In the realm of Customer Experience (CX) management, adopting predictive analytics is vital for enhancing client interactions. By integrating predictive methodologies, businesses can identify potential customer needs and address them proactively. This transformation from reactive to proactive engagement empowers call centers to build stronger relationships with clients through informed decision-making.
The approach involves several key elements. First, organizations must collect and analyze vast data from customer interactions. This enables the identification of patterns that can drive tailored solutions. Second, implementing advanced algorithms allows for the simulation of various scenarios, helping predict outcomes based on customer behaviors. Lastly, continuous assessment and iteration of strategies ensure that the solutions provided are effective and aligned with customer expectations. Emphasizing Predictive CX Optimization leads to a more personalized and efficient experience, ultimately driving customer satisfaction and loyalty.
Best Practices in Utilizing Predictive Tools
To maximize the benefits of predictive tools, it is crucial to establish tailored practices that align closely with your organization's unique needs. The first step in Best Practices in Utilizing Predictive Tools involves understanding your customer data sources. Collecting and analyzing these insights establishes a foundation for effective predictive CX optimization, helping to identify pain points and customer preferences.
Training your team is another vital best practice. Equip staff with the skills needed to navigate and extract value from predictive tools. Regularly updating training materials ensures they are aware of new features and analytics capabilities. Additionally, implementing a feedback loop can help refine your predictive models, adapting them to evolving customer behaviors and market dynamics. By following these practices, organizations can effectively harness the power of predictive tools, driving both improved customer experience and operational efficiency.
- Customizing AI Solutions for Specific Business Needs
Customizing AI solutions for specific business needs involves tailoring predictive CX optimization strategies to meet unique organizational objectives. It is crucial to start by identifying the key challenges and pain points that your business faces. Understanding these factors helps in selecting the right AI tools and developing a framework that aligns with your operational goals.
Next, stakeholders should collaborate to articulate clear objectives and desired outcomes. This approach ensures that the AI systems are integrated meaningfully into existing workflows. Training teams to adapt to these customized tools is equally important. They must understand how to interpret data insights effectively to enhance customer experience. Ultimately, a personalized AI implementation can significantly improve operational efficiency and customer satisfaction, thereby driving better business results.
- Training Teams to Effectively Use Predictive Tools
Training teams to effectively use predictive tools is essential in optimizing customer experience (CX) within call centers. As organizations adopt AI-driven predictive models, it becomes critical to ensure that staff understands how to leverage these technologies. By integrating thorough training sessions, employees can develop confidence in utilizing predictive tools to gather actionable insights and improve service levels.
To effectively train teams, consider implementing these strategies:
- Hands-On Workshops: Encourage practical experience by allowing team members to interact with the predictive tools directly.
- Case Studies: Utilize real-world examples to showcase how predictive analytics leads to improved outcomes.
- Continuous Learning: Foster a culture of ongoing education, ensuring staff stays updated on new features and best practices.
By prioritizing these training methodologies, teams can master predictive CX optimization, thus enhancing customer satisfaction and identifying areas for continuous improvement.
Conclusion: The Future of Predictive CX Optimization in Call Centers
The future of Predictive CX Optimization in call centers relies heavily on the advancement of AI-driven technologies. These innovations not only streamline interactions but also enhance training by providing precise insights into customer inquiries. With the ability to analyze extensive call data, organizations can identify trends and improve service strategies. Rapid response to emerging patterns will empower teams to address customer needs effectively.
As predictive modeling becomes more sophisticated, call centers can expect increased personalization in customer interactions. This proactive approach will ultimately foster better relationships with customers while optimizing operational efficiency. The integration of these technologies will usher in a new era where data-driven decisions become the standard, paving the way for sustained success in customer experience management.