AI-Driven Call QA is transforming the way call centers ensure quality and compliance. In an era where customer experience is paramount, AI technologies offer a systematic approach to analyze calls, evaluate agent performance, and provide actionable insights. By harnessing the power of AI, organizations can streamline their quality assurance processes, ensuring consistent service delivery and enhanced customer satisfaction.
As call centers face ever-increasing demands for efficiency, AI-Driven Call QA becomes indispensable. These models assess interactions with a level of precision that manual evaluations often miss. They gather comprehensive data about agent performance, engagement levels, and compliance with company standards, making it easier for management to identify areas for improvement and coach their teams effectively. Embracing AI in quality assurance not only boosts productivity but also elevates the overall customer experience, ultimately leading to greater loyalty and business success.
Analyze & Evaluate Calls. At Scale.
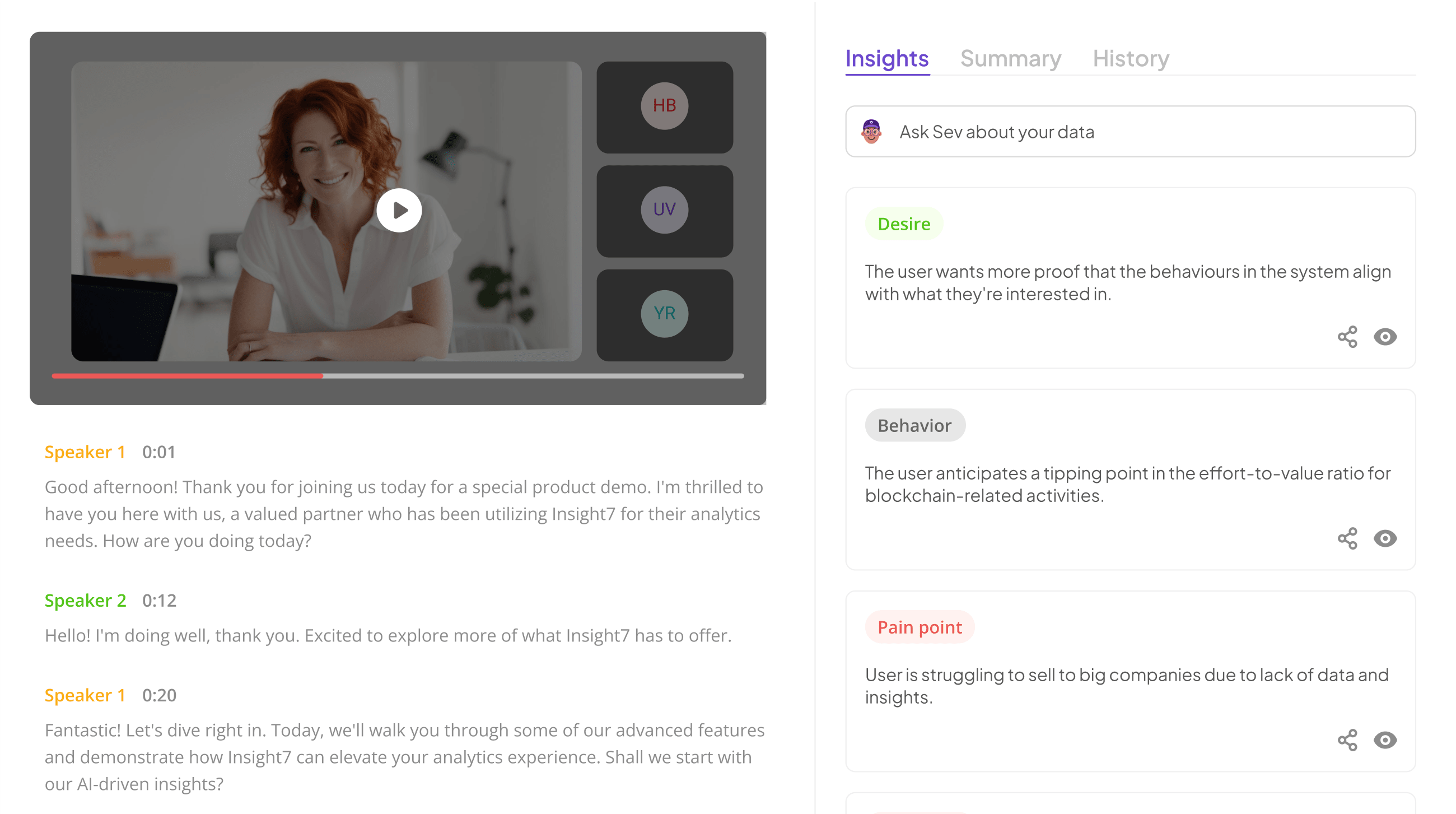
Understanding AI-Driven Call QA Systems
AI-Driven Call QA systems transform the way call centers evaluate agent performance. By automating the quality assurance process, these systems analyze conversations with remarkable accuracy. Typically, they utilize natural language processing to detect key phrases and engagement metrics. Moreover, they can assess compliance by aligning agent responses with company standards. This thorough evaluation offers insight into both individual agent performance and broader team trends.
Implementing AI-Driven Call QA involves several key steps. First, itโs essential to identify the specific needs of the call center. Understanding what metrics matter most can tailor the implementation to deliver the best results. Next, selecting the right AI tools is crucial; choosing one that integrates seamlessly with current systems ensures smoother operations. Finally, collecting and analyzing data from calls enables teams to provide accurate feedback and actionable insights, ultimately enhancing the customer experience.
What is an AI-Driven Call QA Model?
An AI-Driven Call QA Model utilizes advanced algorithms to analyze call interactions between agents and customers. This model processes vast amounts of audio data, identifying key performance indicators like engagement levels and compliance adherence. By harnessing machine learning, it can detect trends, flag issues, and rank agent performance, ultimately enhancing the quality of customer service.
To facilitate effective quality assurance, the AI-driven model typically encompasses several key elements. First, voice recognition technology identifies the agents speaking during calls, which allows for accurate performance evaluations. Second, the system generates detailed scorecards highlighting each agent's strengths and weaknesses. Third, report generation is simplified, enabling compliance officers to provide actionable feedback swiftly. Understanding these components is essential for any organization looking to implement AI-Driven Call QA effectively.
Key Benefits of AI-Driven Call QA
AI-Driven Call QA offers numerous advantages that enhance the efficiency of call center operations. First, it automates the evaluation process, drastically reducing the time spent on reviewing individual calls. This automation allows supervisors to focus on strategic training initiatives rather than routine assessments, ultimately leading to a more proficient workforce. Furthermore, by providing consistent analysis, AI-driven models ensure that all representatives are adhering to established protocols and standards.
Another significant benefit is the capacity for real-time data insights. These insights help identify trends and common customer inquiries, guiding training and process improvements. By recognizing frequently asked questions, call centers can better prepare agents and refine their service offerings. Additionally, AI-driven systems can enhance customer satisfaction by ensuring agents are equipped with the knowledge and skills required to address customer needs effectively. Overall, embracing AI-Driven Call QA can lead to streamlined operations, improved agent performance, and higher levels of customer engagement.
Implementing AI-Driven Call QA Models Step by Step
Implementing AI-Driven Call QA involves a structured approach to enhance call center quality assurance processes. Start by identifying the specific needs of your call center, focusing on elements like compliance and customer satisfaction metrics. Understanding these needs helps tailor the AI solution effectively.
Next, choose the right tools that align with your objectives. Several platforms, such as Chorus.ai and Observe.AI, offer various features to automate transcription and evaluation. Once the tools are selected, integrate them into your existing systems to ensure a seamless transition. This integration is crucial for leveraging the full potential of AI-Driven Call QA, allowing for consistent monitoring and analysis of customer interactions.
Finally, regularly refine your model based on feedback and outcomes. This iterative approach ensures that the AI system constantly adapts to evolving quality assurance criteria, ultimately improving service outcomes and customer satisfaction.
Extract insights from interviews, calls, surveys and reviews for insights in minutes
Step 1: Identifying Call Center Needs
To effectively implement AI-Driven Call QA, the first step involves thoroughly identifying your call centerโs unique needs. Begin by assessing both the current performance of your customer service representatives and the standards you wish to achieve. Engage in conversations with team members and gather insights on their experiences and challenges. This approach aids in clearly defining objectives for your AI integration, ensuring that it aligns with your overall goals and enhances customer satisfaction.
Additionally, consider the types of data you want to analyze and how it will inform your training strategies. For instance, identifying common customer inquiries or issues allows you to tailor training programs that improve service delivery. Reviewing previous call records can also shed light on trends and opportunities for efficiency. By pinpointing these specific needs, you set a solid foundation for a successful AI-Driven Call QA implementation that delivers real value to your organization.
Step 2: Selecting the Right Tools for AI-Driven Call QA
Selecting the right tools for AI-Driven Call QA is a critical step in enhancing call center operations. The effectiveness of your quality assurance program hinges on the tools you choose. Firstly, evaluation features are essential. Look for solutions that can provide comprehensive analysis, including call transcriptions and sentiment analysis. This capability allows quality assurance teams to assess agent performance and customer interactions effectively.
Secondly, customization options play a significant role. Opt for platforms that let you tailor templates and evaluation criteria to align with your specific business objectives. For instance, being able to create unique compliance templates can help ensure calls are evaluated against the most relevant standards for your organization. Finally, integration capabilities are vital. Choose tools that seamlessly integrate with existing systems, allowing for smoother data flow and streamlined processes. By focusing on these key aspects, you can select the most suitable tools for implementing AI-Driven Call QA.
insight7
The integration of AI-Driven Call QA models significantly transforms how call centers ensure quality assurance. Traditional methods that relied heavily on manual analysis have become increasingly inefficient and time-consuming. With AI, call centers can analyze vast amounts of customer interactions rapidly, offering actionable insights that drive business decisions. This advancement not only enhances efficiency but also improves customer satisfaction by enabling representatives to engage more effectively.
Understanding the specific needs of a call center is crucial for successful implementation. Organizations must identify key areas where AI can significantly impact call quality, such as real-time feedback and conversation analysis. After establishing these needs, the next step is selecting appropriate AI tools tailored to handle the identified challenges. The evolution of AI-Driven Call QA has made it essential for businesses to embrace these technologies to remain competitive, as the demand for timely insights continues to grow.
Chorus.ai
Chorus.ai provides an innovative approach to implementing AI-driven call QA. The platform simplifies the analysis of conversations at scale, empowering users to efficiently extract actionable insights. By initially transcribing audio calls, organizations can dive into a comprehensive library of call data where they can visualize individual conversations seamlessly.
Users can leverage intuitive templates that guide them in pulling out specific insights such as customer pain points. This automatic extraction makes it easy to analyze bulk files, ensuring no critical information is overlooked. Once the calls are analyzed, users can summarize key discussions, highlighting essential keywords and trends, which helps in understanding voice-of-the-customer elements effectively. Through these capabilities, Chorus.ai exemplifies how AI-driven call QA can enhance quality assurance processes, ultimately leading to improved customer interactions and satisfaction.
Observe.AI
The implementation of AI-driven call quality assurance (QA) systems significantly enhances the efficiency of call center operations. These systems utilize sophisticated algorithms to analyze agent-customer interactions, ensuring compliance with company standards and improving overall service quality. The ability to automatically assess hundreds of calls in a short timeframe provides invaluable insights into agent performance and customer engagement levels.
Moreover, AI-driven tools can generate detailed performance reports, highlighting areas of strength and opportunities for improvement. This allows management to provide targeted coaching for agents, fostering a culture of continuous development. By integrating AI-driven QA systems, organizations can streamline their evaluation processes, ensuring that quality assurance is proactive rather than reactive. Ultimately, these advancements contribute to a better customer experience, driving loyalty and satisfaction.
When implementing these systems, consider the following key points:
- Data Accuracy: Ensure that the AI can accurately identify agents and their speeches.
- Customization: Tailor reports to meet specific compliance needs.
- Streamlined Reporting: Choose formats that allow easy editing and feedback sharing.
By addressing these factors, a call center can harness the full potential of AI-driven call QA, making informed decisions based on robust performance analytics.
CallRail
CallRail provides a robust platform for implementing AI-Driven Call QA, enhancing the call center experience by automating quality assurance tasks. This platform facilitates easy access to call data, allowing teams to analyze customer interactions without requiring extensive technical training. Users can quickly transform call recordings into actionable insights that inform improvements in service delivery.
One of the standout features is the library where all recordings are stored, enabling team members to visualize conversations. By analyzing these calls, the platform identifies pain points, customer desires, and behaviors. The clear presentation of insights allows for efficient data-driven decisions, improving overall customer experience and operational efficiency. With CallRail, integrating AI-Driven Call QA becomes a straightforward process that empowers all employees to engage with data and enhance their understanding of customer interactions.
Talkdesk
With the rise of AI-driven call QA models, organizations can now streamline their quality assurance processes more effectively. The ability to analyze conversations at scale has revolutionized how call centers evaluate interactions. Using intuitive platforms, teams can easily upload audio files and swiftly generate actionable insights. This capability ensures that every customer interaction is scrutinized, ultimately enhancing service delivery.
The process typically begins with transcription, where voice recordings are converted to text. After transcribing, teams can utilize various tools to explore specific insights, such as identifying customer pain points. This streamlined approach not only improves efficiency but also supports quality assurance by allowing for real-time analysis and strategic adjustments. Incorporating these AI-driven call QA models enables organizations to foster an environment of continuous improvement, ensuring that each customer experience is not just satisfactory, but exceptional.
Step 3: Integrating AI-Driven Call QA within Existing Systems
Integrating AI-Driven Call QA within existing systems is a crucial step in enhancing overall quality assurance processes. To successfully accomplish this integration, it is vital to assess how the AI system will interact with current workflows. Begin by examining existing processes, identifying areas where AI solutions can provide measurable improvements. This understanding will allow for smoother transitions and will promote acceptance among staff who will rely on these new systems.
Next, ensure the technology is compatible with existing software and infrastructure. While many AI-Driven Call QA tools are designed for easy integration, some may require customization to fit unique operational needs. Training your team on these systems is also essential, as it fosters a better understanding and increased utilization. A well-executed integration will empower agents, improve customer interactions, and ultimately drive up compliance ratings, making the call center more efficient.
Challenges and Solutions in AI-Driven Call QA Implementation
Implementing AI-Driven Call QA presents several challenges that organizations must navigate to achieve successful outcomes. Common issues include the accuracy of AI in identifying agent performance, resistance from staff, and the complexity of integrating these systems with existing technologies. For instance, inaccuracies in detecting who is speaking can lead to unreliable evaluations, causing misjudgments in agent performance.
To overcome these hurdles, organizations must adopt effective solutions tailored to their specific needs. Firstly, enhancing training datasets with agent identifiers can significantly improve AI accuracy. Secondly, fostering a culture of transparency regarding changes can alleviate resistance from staff and cultivate acceptance of the new system. Lastly, developing seamless integrations with existing call center technologies ensures a smoother transition. By addressing these challenges proactively, businesses can fully leverage the benefits of AI-Driven Call QA, leading to more efficient quality assurance processes and improved customer interactions.
Common Challenges of AI-Driven Call QA Implementation
Implementing AI-Driven Call QA often presents various challenges that organizations must navigate effectively. One major hurdle is ensuring accurate identification of call agents during interactions. Misidentification can lead to incorrect evaluations and diminish the overall trust in the AI system. Additionally, while AI has the potential to analyze calls at scale, the accuracy of such analyses heavily depends on the quality of the training data. Inconsistent data inputs may produce skewed results, complicating performance assessments.
Another significant challenge is the resistance to change within call center teams. Many employees may feel apprehensive about AI's role in their evaluation processes. This resistance can hinder the seamless integration of AI-driven systems. Furthermore, lack of customization options in AI tools can restrict organizations from tailoring the systems to their unique QA needs, thus limiting their effectiveness. Addressing these challenges is critical for a successful transition to AI-Driven Call QA.
Effective Solutions for Overcoming Implementation Challenges
Implementation of AI-Driven Call QA models often presents a range of challenges. Organizations may face resistance from staff who are accustomed to traditional methods. To address these concerns, it's essential to emphasize the benefits, such as improved accuracy and efficiency in quality assurance processes. Engaging employees through training that clarifies how AI enhances their roles can significantly ease this transition.
Another challenge is ensuring seamless integration with existing systems. Selecting the right tools is crucial, as they must complement current workflows. Establishing feedback loops allows for continuous improvement of AI models, refining their understanding of quality benchmarks. Additionally, providing ongoing support and clarifying AI's role in enhancing customer interactions fosters a collaborative environment. By tackling these issues head-on, companies can successfully navigate the complexities of implementing AI-Driven Call QA systems, paving the way for improved service quality and operational effectiveness.
Conclusion on the Impact of AI-Driven Call QA Systems
AI-driven call QA systems are reshaping the way call centers ensure quality and compliance. By providing accurate assessments of agent performance, these systems facilitate data-driven improvements that enhance customer interactions. The automation of feedback generation significantly reduces the time spent on evaluations, allowing teams to focus on enhancing their strategies and training programs.
The impact of AI-driven call QA extends beyond operational efficiency. It fosters a culture of continuous improvement by providing agents with instant feedback and actionable insights. As organizations embrace these technologies, they position themselves for better customer satisfaction and increased loyalty, ultimately driving business success in a competitive landscape.