Predictive Scorecards Implementation transforms the way organizations assess performance and forecast outcomes. By utilizing artificial intelligence, these scorecards offer data-driven insights that help businesses make informed decisions. Imagine having the capability to predict customer behavior or identify potential market trends before they occurโthis is the power of predictive scorecards.
In implementing these systems, itโs crucial to understand the key components that drive their success. This section will guide you through the foundational concepts and importance of predictive scorecards. You will learn how these tools can enhance your decision-making processes and empower teams, regardless of their technical expertise, to leverage data for improved strategy and performance.
Analyze qualitative data. At Scale.
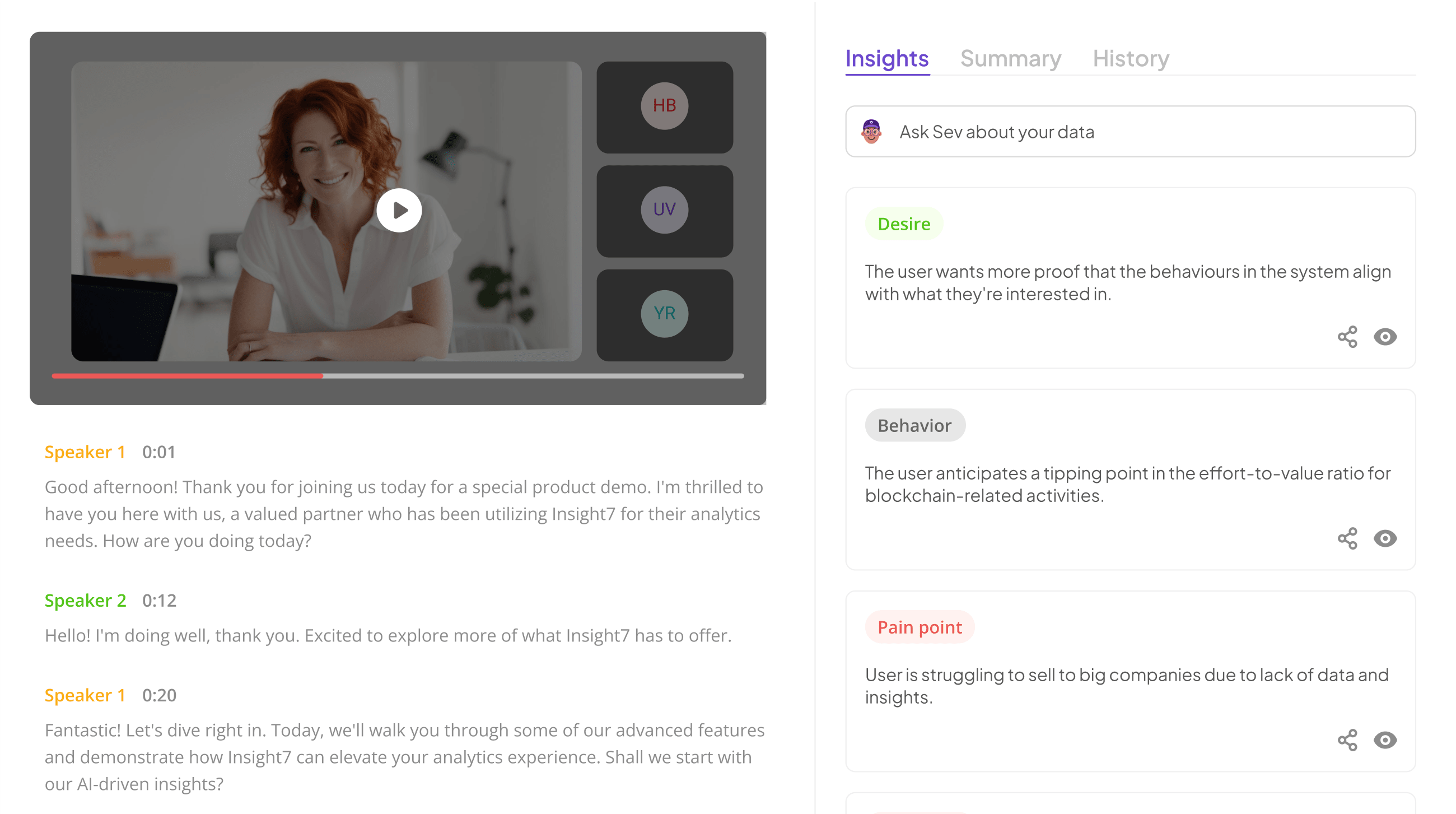
Understanding Predictive Scorecards Implementation
Understanding Predictive Scorecards Implementation is crucial for organizations aiming to leverage AI for enhanced performance analysis. The implementation process begins with identifying the specific performance criteria that align with your objectives. Each criterion must reflect the nuances of the roles being evaluated, whether focusing on customer interaction or technical skill.
Once these criteria are established, data must be collected that supports meaningful analysis. This stage often involves gathering qualitative and quantitative data to ensure a comprehensive view of performance. After data collection, AI tools can be employed to analyze patterns and generate actionable insights. By consistently monitoring these scorecards, organizations can better understand employee strengths and weaknesses, leading to informed decisions that drive performance improvement.
Overall, Predictive Scorecards Implementation not only empowers organizations to evaluate performance but also enhances overall employee development strategies in a structured manner.
What Are AI-Based Predictive Performance Scorecards?
AI-based predictive performance scorecards are innovative tools that harness the power of artificial intelligence to evaluate and forecast key performance indicators. These scorecards analyze historical and current data, allowing organizations to measure agents' performance effectively. By leveraging advanced algorithms, they enable decision-makers to retrieve actionable insights quickly, which is crucial for enhancing operational efficiency.
In implementing these predictive scorecards, organizations can identify strengths and weaknesses within their teams, track engagement, and assess overall performance systematically. This data-driven approach also aids in setting realistic goals and adapting strategies based on predicted outcomes. Notably, this process involves a combination of selecting the right data sources, appropriate AI tools, and precise design, all pivotal for achieving successful scorecard implementation. As a result, AI-based predictive performance scorecards empower organizations to make informed decisions, ensuring continuous improvement and alignment with business objectives.
The Importance of Implementing Predictive Scorecards
Implementing predictive scorecards is crucial for organizations striving to enhance their decision-making processes. Predictive scorecards implementation transforms raw data into actionable insights, enabling teams to identify future trends and performance gaps. By leveraging historical data and AI algorithms, these scorecards help organizations track progress toward their goals effectively.
The importance of predictive scorecards lies in their ability to foster a data-driven culture. They provide a clear framework for evaluating team performance against predefined criteria, ensuring accountability and clarity. Furthermore, predictive scorecards facilitate continuous improvement by highlighting areas needing attention. This proactive approach allows organizations to adjust tactics and strategies, ultimately leading to improved outcomes. Embracing predictive scorecards is not just about data collection; itโs about empowering teams with the insights necessary to excel in an increasingly competitive landscape.
Extract insights from interviews, calls, surveys and reviews for insights in minutes
Key Steps in Predictive Scorecards Implementation
Successful predictive scorecards implementation requires a systematic approach to ensure effective performance tracking. The first step is defining clear objectives and goals; understanding what metrics need evaluation is crucial for alignment. Identifying the right data sources follows, which involves collecting comprehensive datasets that accurately represent the business context. This data serves as the foundation for your scorecards.
Next, selecting appropriate AI tools for insightful predictive analytics is essential. Various platforms offer unique features; choices like IBM Watson Analytics and Microsoft Azure can enhance analysis capabilities. Once tools are in place, designing and building the scorecards becomes the focus. Craft user-friendly and visually appealing scorecards that convey information effectively. After construction, it is vital to test and validate the AI model to ensure its reliability. Finally, launching and continuously monitoring performance enables businesses to refine their scorecards, adapting to changing needs over time.
Step 1: Defining Objectives and Goals
Defining objectives and goals is the foundational step in Predictive Scorecards Implementation. Begin by identifying specific business challenges that predictive performance scorecards aim to address. This clarity helps in understanding what metrics matter most for measuring success. Engaging stakeholders during this phase enhances alignment and ensures that everyone shares a common vision. Consider both short-term and long-term objectives to create a comprehensive framework for evaluation.
Next, articulate measurable goals that reflect the desired outcomes. These goals should be specific, achievable, and time-bound, providing a clear direction for the implementation process. By establishing benchmarks, organizations can easily track progress and make necessary adjustments. Ultimately, a well-defined objectives phase sets the stage for successful scorecards that drive decision-making and enhance performance across various departments, creating a culture of data-driven excellence.
Step 2: Selecting the Right Data Sources
Choosing the right data sources is essential for effective predictive scorecards implementation. Data plays a crucial role in determining the accuracy and reliability of your scorecards. Start by assessing various types of data, such as transactional records, customer feedback, and market trends that align with your specific objectives. Selecting diverse and relevant sources enhances your ability to uncover meaningful insights, ultimately improving decision-making processes.
Consider the quality, availability, and timeliness of the data you choose to integrate. Not all data sources are created equal; thus, establishing partnerships with reputable data providers can be invaluable. Ensure that the data is well-structured and standardized, which will facilitate smoother integration into your predictive models. By carefully selecting data sources, you lay a solid foundation for your predictive analytics efforts, enabling more accurate forecasting and performance evaluation.
Step 3: Choosing AI Tools for Insightful Predictive Analytics
In Step 3 of the Predictive Scorecards Implementation, the selection of AI tools significantly influences the quality of insights derived from your predictive analytics. The objective here is to choose tools that not only analyze data effectively but also present the findings in an easily interpretable format. Begin by assessing your organizational needs and the types of data you plan to utilize. This ensures that the tools you choose align well with your business objectives.
When evaluating potential AI tools for insightful predictive analytics, consider factors such as ease of use, flexibility, and the capability to handle diverse data types. Some recommended options include IBM Watson Analytics, which offers powerful data visualization features, and Microsoft Azure Machine Learning, known for its robust machine learning models. Tools like RapidMiner and SAS Advanced Analytics can also facilitate deeper insights into customer behaviors, further enhancing your scorecardsโ effectiveness. Selecting the right platforms will enable your team to interpret data meaningfully and drive better business decisions.
insight7
In the context of predictive scorecards implementation, understanding the need for insightful predictive analytics is paramount. Organizations looking to implement such scorecards must first establish their objectives clearly to ensure alignment with their business goals. This not only sets a firm foundation but also guides the selection of relevant data sources crucial for effective performance measurement.
Next, choosing the right AI tools for building scorecards enhances the analytical capabilities necessary for informed decision-making. These tools help organizations sift through vast amounts of customer data, translating insights into actionable strategies. Additionally, designing and validating these scorecards is critical for ensuring they address the defined objectives effectively. Ultimately, launching the scorecards and monitoring their performance allows organizations to reap the benefits of predictive insights continuously. By adhering to these steps, organizations can leverage predictive scorecards to elevate their strategic initiatives.
IBM Watson Analytics
IBM Watson Analytics serves as a pivotal tool in the Predictive Scorecards Implementation journey. Designed to enhance data visualization and insights extraction, it allows organizations to transform raw data into actionable intelligence. Users can easily analyze vast datasets, identifying key patterns and trends that inform decision-making processes. This analytical capability empowers teams to visualize both positive and negative customer feedback effectively, providing a holistic view of performance metrics.
To successfully implement predictive scorecards, organizations must focus on several key elements. First, the tool enables the selection of diverse data sources that are crucial for accurate forecasting. Next, it allows users to execute complex queries across different datasets, facilitating deeper insights into customer behavior. Finally, its interactive interface promotes collaboration among team members, making it easier to build and validate the predictive models necessary for enhancing overall performance. By integrating this powerful analytics platform, organizations can significantly elevate their predictive analysis capabilities.
Microsoft Azure Machine Learning
In the realm of predictive scorecards implementation, Microsoft Azure Machine Learning offers a powerful suite of tools and services designed to facilitate effective data analysis. This platform enables users to build, train, and deploy machine learning models quickly and efficiently. By leveraging the capabilities of Azure, organizations can harness vast amounts of data to create AI-driven insights that enhance decision-making processes.
Furthermore, Azure Machine Learning provides a user-friendly interface and comprehensive tools for model management. It allows teams to automate workflows, monitor performance, and integrate seamlessly with various data sources. As organizations embark on their predictive scorecards implementation journey, utilizing Azure can significantly streamline their operations. By integrating AI into business strategies, companies can gain actionable insights and drive performance improvements across their operations.
RapidMiner
RapidMiner serves as a powerful tool in the realm of predictive scorecards implementation. It provides intuitive data preparation, which allows users to clean and format data easily. This essential feature enhances the accuracy of predictive models, ensuring that insights derived from the data are reliable and actionable. Users can visually create workflows, making the entire process more efficient and less prone to errors.
Moreover, RapidMiner offers advanced machine learning algorithms, enabling organizations to build robust predictive models. With its user-friendly interface, teams can experiment with different models and parameters to find the best fit for their specific objectives. This flexibility is crucial in predictive scorecards implementation, as it helps adapt to changing business needs. By leveraging such capabilities, organizations can gain valuable insights from their data, driving better decision-making and strategic planning.
SAS Advanced Analytics
SAS Advanced Analytics provides robust capabilities to enhance the Predictive Scorecards Implementation process. By utilizing advanced statistical methods and machine learning algorithms, SAS can transform raw data into actionable insights. This analytical platform enables organizations to identify patterns and correlations, allowing for more accurate predictive modeling. Its powerful tools facilitate the selection of relevant data sources, ensuring that the predictive models are grounded on solid foundations.
Moreover, SAS offers visualization features that make data interpretation straightforward. It allows users to explore various predictive metrics easily, presenting the information in an easily digestible format. Key findings from predictive scorecards can thus be communicated effectively to stakeholders, influencing strategic decisions. Leveraging SAS Advanced Analytics in the implementation of predictive scorecards not only enhances performance tracking but also aligns organizational goals with data-driven insights, leading to better business outcomes.
Step 4: Designing and Building the Scorecards
In Step 4, the focus shifts to the crucial task of designing and building the scorecards. The design must align with the specific objectives and desired outcomes identified in earlier steps. Begin by collaborating with relevant stakeholders to gather input on the metrics that will effectively gauge performance. This collaborative approach ensures that the scorecards reflect the unique needs of different teams and functions, facilitating buy-in and practical utility.
Once the relevant metrics are determined, the next phase is to create the visual layout of the scorecards. Employ user-friendly designs and intuitive dashboards for clarity. Incorporate data visualizations, such as graphs and charts, to effectively communicate insights. By focusing on usability and clarity, organizations can enhance the adoption of these tools, making Predictive Scorecards Implementation not just a technical endeavor, but a strategic initiative that drives informed decision-making across all levels of the organization.
Step 5: Testing and Validating the AI Model
Testing and validating the AI model is a critical step in the predictive scorecards implementation. This phase ensures that the model is reliable and capable of generating accurate predictions. Begin by dividing your data into training and testing datasets. The training dataset will help the model learn patterns, while the testing dataset will evaluate its performance in real-world scenarios.
Conduct various tests to validate the model's accuracy and robustness. Common techniques include cross-validation, confusion matrices, and performance metrics like precision and recall. Evaluate your model's predictions against actual outcomes to ascertain its reliability. Additionally, ensure to test for biases that may skew results, which can undermine decision-making. By rigorously testing and validating your AI model, you enhance its credibility and effectiveness, making it a valuable asset in your predictive scorecards implementation process.
Step 6: Launching and Monitoring Performance
Launching your AI-based predictive performance scorecards marks a pivotal moment in your implementation journey. Begin by carefully deploying the scorecards across your organization, ensuring all stakeholders are familiar with their functionality and potential impact. Comprehensive training sessions can enhance user confidence and facilitate smoother adoption. This initial launch phase is crucial for ensuring your team understands how to engage with the scorecards effectively.
Following the launch, monitoring performance is essential to gauge their effectiveness and efficacy. Establish key performance indicators (KPIs) that align with your original goals to track progress systematically. Regular check-ins and data analysis will allow you to make informed adjustments and ensure continuous improvement. Utilize feedback from users to refine the scorecards further and maximize their benefits. Emphasizing a culture of optimization will empower your team to fully leverage predictive scorecards implementation for enhanced decision-making and performance outcomes.
Conclusion: Mastering Predictive Scorecards Implementation
Successfully mastering predictive scorecards implementation requires a clear understanding of your goals and the data at your disposal. By emphasizing straightforward user access, organizations can enhance collaboration and ensure everyone can generate valuable insights. This democratization of information not only streamlines processes but also fosters a culture of data-driven decision-making.
Iterating through the development steps of your predictive scorecards and maintaining a focus on actionable outcomes will ensure continuous improvement. Regularly reviewing performance metrics and adapting your approach will codify the benefits and reinforce the role of predictive analytics in shaping the future success of your initiatives. Ultimately, a well-executed implementation can transform how organizations understand and respond to performance data.