The Role of Predictive Analytics in Call Center QA Evaluations
-
Bella Williams
- 10 min read
Predictive QA Analytics revolutionizes how call centers approach quality assurance evaluations. By employing advanced analytical methods, organizations can forecast outcomes based on historical data, enabling them to identify patterns that influence performance. This proactive strategy not only promotes compliance adherence but also enhances customer satisfaction through improved service delivery.
Understanding Predictive QA Analytics empowers call center managers to refine evaluation processes fundamentally. By utilizing predictive models, companies can develop customized criteria that assess agent performance meaningfully. The application of these analytics leads to targeted training initiatives, promoting continuous improvement and fostering a culture of excellence within call centers.
Analyze & Evaluate Calls. At Scale.
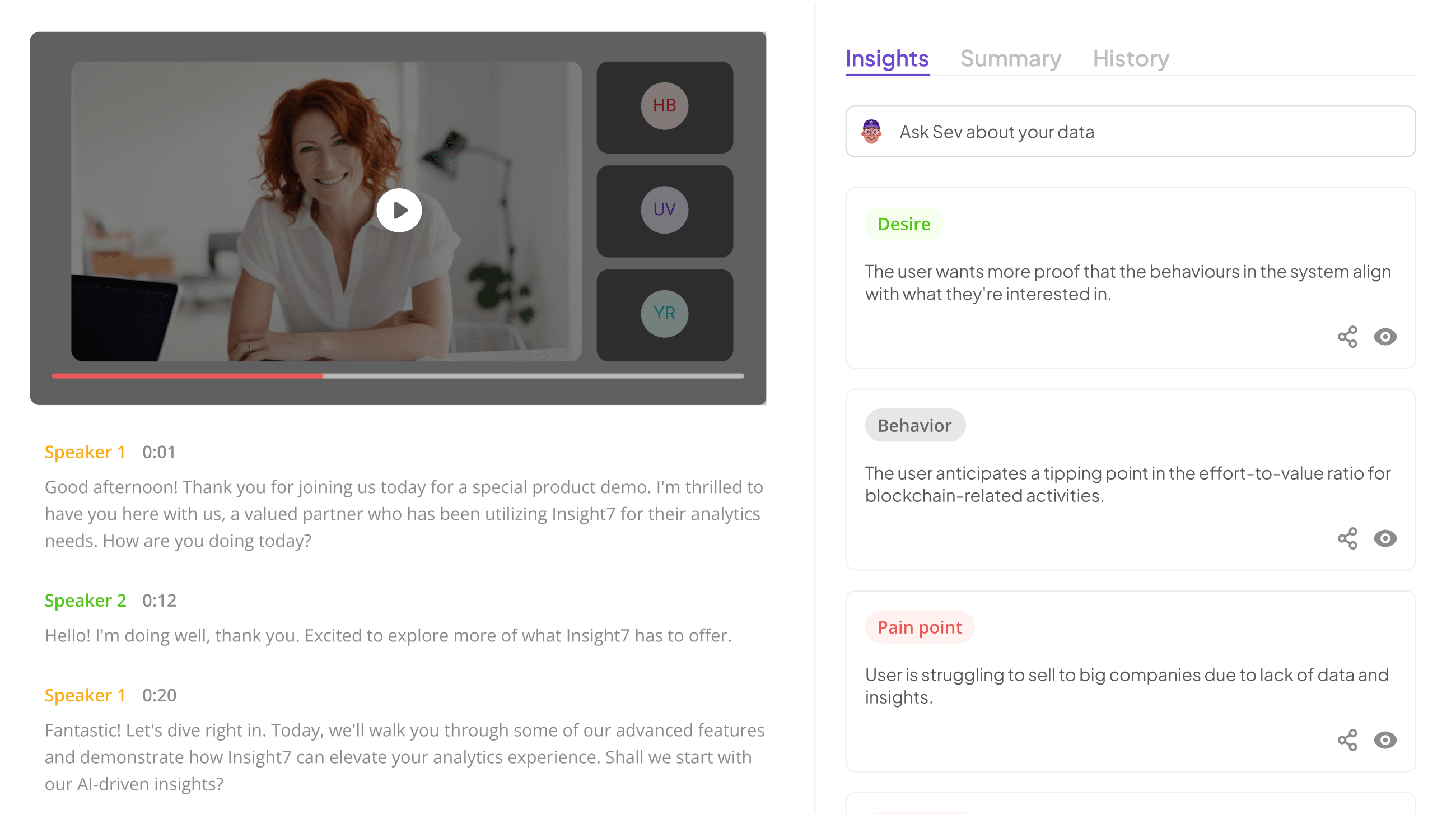
Harnessing Predictive QA Analytics for Enhanced Call Center Performance
Implementing Predictive QA Analytics can revolutionize call center performance, significantly improving how teams evaluate customer service interactions. By utilizing data-driven insights, organizations can better understand their team's effectiveness, compliance with training protocols, and even customer satisfaction levels. This analytical approach allows for swift assessments of customer service representatives based on predefined metrics, limiting the time spent on manual evaluations of lengthy calls.
Furthermore, predictive analytics facilitate the identification of trends and common inquiries from customers, allowing call centers to tailor their training programs to address the most frequent concerns. This data enables decision-makers to adopt a proactive strategy in quality assurance, refining processes in real-time. Enhanced insights can lead to a more engaged workforce, ultimately fostering a stronger connection between customer service representatives and their clientele. By harnessing these analytics, call centers can not only streamline operations but also elevate overall customer experiences.
Step-by-Step Implementation of Predictive QA Analytics
To implement predictive QA analytics effectively, organizations must follow a structured approach. First, data collection and integration are paramount. This involves gathering relevant data from various sources, including call recordings, customer interactions, and agent performance metrics. Subsequently, this data should be integrated into a centralized system to facilitate efficient analysis and reporting.
Next, organizations should focus on model development and deployment. This step entails creating predictive models specifically designed for QA evaluations. These models can identify trends, issues, and opportunities for improvement. Following the model creation, real-time deployment and monitoring are essential. This allows for immediate insights during calls, ensuring agent performance aligns with quality standards.
By systematically executing these steps, organizations can harness the full potential of predictive QA analytics, leading to better call center performance and enhanced customer satisfaction.
- Data Collection and Integration
Data collection and integration serve as the foundation for effective predictive QA analytics. Gathering relevant data from diverse sources is crucial for understanding customer interactions and identifying performance trends. This process typically involves collecting data from call logs, customer feedback, and agent evaluations to create a comprehensive picture of call center operations.
Once data is gathered, it must be integrated into a centralized system for analysis. This integration allows for seamless access to insights derived from various data points. By employing advanced analytical tools, organizations can uncover patterns and pinpoint areas for improvement in agent performance and customer satisfaction. Proper data integration not only enhances the quality of insights but also ensures that decision-makers have a clear understanding of the performance metrics that matter most. This systematic approach lays the groundwork for building predictive models designed to maximize QA evaluation efficiency.
- Gathering relevant data from various sources.
Gathering relevant data from various sources is crucial for effective Predictive QA Analytics in call center evaluations. This process begins by identifying diverse data types, such as customer interactions, agent performance metrics, and market trends. Each source offers unique insights that contribute to a comprehensive understanding of service quality and areas for improvement.
Once data is identified, it should be collected systematically and integrated into a centralized platform. This enables seamless analysis and fosters collaboration among teams. In addition to call transcripts, customer surveys, and feedback data can be synthesized to form a more holistic view. By utilizing various information sources, call centers can make informed decisions, fine-tune training programs, and enhance overall service delivery. Ultimately, the effectiveness of Predictive QA Analytics hinges on the richness and diversity of data gathered, making this step indispensable for successful evaluations.
- Integrating data into a centralized system.
Integrating data into a centralized system is vital to harnessing the power of predictive QA analytics. This process involves gathering diverse data from various sources and consolidating it into a single, manageable system. By streamlining data collection, call centers can enhance their ability to evaluate performance objectively and accurately.
A centralized data system allows for efficient real-time analysis, enabling quick decision-making. It also promotes consistency in metrics and insights across the organization. Moreover, integrating disparate data sources ensures a holistic view of call center operations, paving the way for data-driven enhancements in service quality. Through these integrations, predictive analytics can identify trends and provide insights, ultimately improving customer satisfaction and operational efficiency. Implementing this approach prepares call centers for future advancements in quality assurance technology, allowing for continual improvement in performance evaluations.
- Model Development and Deployment
The process of developing and deploying predictive models is essential for effective quality assurance evaluations in call centers. Initially, organizations focus on crafting models tailored to their unique needs for QA analytics. This meticulous process involves selecting appropriate algorithms and training the models using historical call data. By leveraging past interactions, companies can uncover patterns and predict future customer behaviors, ensuring that call center representatives meet established quality benchmarks.
Once the models are developed, they must be deployed into operational environments. This step requires continuous monitoring to ensure optimal performance and accuracy. Real-time analysis enables organizations to adjust strategies and improve call handling processes based on predictive insights. Ultimately, the integration of predictive QA analytics enhances the overall evaluation framework, providing valuable feedback that drives continuous improvement. This proactive approach empowers businesses to elevate customer service standards by aligning their operations with customer expectations.
- Creating predictive models suited for QA evaluations.
Creating predictive models suited for QA evaluations involves a systematic approach that focuses on enhancing the quality assurance process in call centers. Predictive QA Analytics enables organizations to effectively analyze historical data and spot patterns, which leads to more informed evaluations. These models are designed to assess various aspects of call performance, improving both customer satisfaction and operational efficiency.
The first stage involves identifying relevant metrics, such as compliance with scripts and customer handling techniques. Next, the data is compiled and analyzed to uncover trends that indicate strengths and weaknesses in agent performance. Finally, the predictive models are refined through continuous testing to ensure they remain accurate and relevant. This proactive method not only supports the immediate evaluation of calls but also fosters long-term improvements in training and feedback mechanisms. Ultimately, the integration of predictive models into QA evaluations equips call centers with the insights needed to elevate service quality and drive customer loyalty.
- Deploying and monitoring models in real-time.
Deploying and monitoring models in real-time is a critical aspect of predictive QA analytics in call center evaluations. This process involves first establishing models that assess call quality and customer interactions based on previously collected data. When these models are deployed, they provide immediate insights into ongoing calls, ensuring that agents adhere to established standards.
๐ฌ Questions about The Role of Predictive Analytics in Call Center QA Evaluations?
Our team typically responds within minutes
Real-time monitoring allows for timely adjustments and interventions. For instance, if an agent's performance dips, supervisors can receive alerts to provide coaching instantly. This dynamic approach not only enhances agent performance but also improves customer satisfaction. By continuously evaluating call metrics in real-time, organizations can refine their evaluations and adapt to changing customer needs swiftly, ultimately leading to a more efficient and effective QA process.
Tools for Effective Predictive QA Analytics
To effectively implement predictive QA analytics, call centers can utilize several advanced tools. These tools are designed to enhance data analysis and drive insights that improve service quality. One key tool is Insight7, which helps in aggregating customer feedback to identify trends and performance gaps. CallMiner offers robust analytics capabilities, enabling detailed evaluation of call interactions to enhance agent training. NICE Nexidia focuses on speech analytics, allowing teams to gauge customer sentiment efficiently.
Additionally, Verint Systems and Genesys Cloud CX provide comprehensive monitoring solutions that facilitate real-time evaluations of agent performance. By integrating these tools into daily operations, call centers can harness the power of predictive analytics to not just assess past performance but also to forecast future outcomes. This proactive approach ultimately enhances customer satisfaction and strengthens service delivery. The careful selection and implementation of these tools are pivotal in driving a successful predictive QA analytics framework in call centers.
Extract insights from interviews, calls, surveys and reviews for insights in minutes
Key Tools to Enhance Predictive QA Analytics in Call Centers
To enhance predictive QA analytics in call centers, organizations should focus on key technological tools that streamline their evaluation processes. These tools enable the automation of quality assessments and improve the accuracy of performance metrics. As a result, they provide valuable insights that can shape training programs and adapt operational strategies.
Key tools include call analysis software that utilizes machine learning to identify patterns in customer interactions and feedback. For instance, platforms like CallMiner and NICE Nexidia can analyze thousands of calls, extracting actionable insights regarding customer inquiries and agent responses. This capability allows call centers to swiftly adapt to emerging trends and improve overall service quality. Additionally, integrating analytics tools with your existing CRM systems can facilitate seamless data flow, enriching predictive models and ensuring they remain relevant to evolving customer needs. By prioritizing these tools, call centers can significantly advance their predictive QA analytics, ultimately enhancing customer satisfaction and operational efficiency.
- Insight7
In the evolving landscape of call center quality assessments, Insight7 explores the transformative power of predictive analytics. Predictive QA Analytics serves as a key driver for enhancing performance, enabling organizations to proactively identify patterns and anticipate issues. As companies collect more customer data than ever before, traditional analysis methods often fall short, leading to missed opportunities and slow response times.
The implementation of Predictive QA Analytics typically encompasses several crucial steps. First, organizations must focus on data collection and integration, ensuring that they gather relevant data from diverse sources and centralize it effectively. Next, model development and deployment come into play, where tailored predictive models are created and monitored to provide real-time insights. This structured approach not only streamlines operations but also fosters more efficient collaboration, allowing customer service teams to respond swiftly and accurately to customer needs. Ultimately, embracing these advanced analytics tools can lead to significant improvements in both customer satisfaction and the overall efficiency of call center operations.
- CallMiner
CallMiner stands out as an essential tool in the realm of predictive analytics for call center QA evaluations. It efficiently analyzes vast amounts of call data to extract meaningful insights. This capability is crucial for identifying trends, potential compliance issues, and performance gaps among agents. Utilizing advanced algorithms, it streamlines the evaluation process, ensuring that evaluators can focus on calls that matter most.
Organizations can discern patterns in customer interactions and agent performance through the integration of CallMiner with existing systems. The predictive analytics features allow call centers to anticipate issues before they escalate, thereby improving overall service quality. By focusing on predictive QA analytics, call centers can implement proactive training and compliance measures that significantly enhance operational efficiency and customer satisfaction. This strategic approach leads to better decision-making based on data-driven insights, ultimately supporting the call center's success.
- NICE Nexidia
The integration of advanced technology in call centers significantly enhances the evaluation process, especially through Predictive QA Analytics. Engaging with the right tools facilitates the analysis of audio files, enabling teams to derive insights efficiently. By automating the transcription of conversations, quality assurance teams can swiftly access critical data. This automation allows for comprehensive reviews, enabling a deeper understanding of customer interactions and agent performances.
Focusing on ease of use, modern solutions are designed for users without technical expertise. This accessibility encourages continuous improvement in service quality. Additionally, these tools often allow users to extract specific insights and pain points from conversations, offering evidence-backed data for better decision-making. The future of call center operations hinges on such innovative technologies, ensuring enhanced performance and optimized quality assurance evaluations. As call centers continue to adopt these technologies, Predictive QA Analytics will play a crucial role in shaping their success.
- Verint Systems
Verint Systems plays a pivotal role in the evolution of predictive analytics within call centers. As organizations strive for optimized quality assurance evaluations, the integration of innovative technologies becomes essential. Predictive QA analytics establishes a framework that helps call centers accurately assess customer interactions. This ultimately leads to improved service delivery and customer satisfaction.
The utilization of predictive analytics tools facilitates the collection and analysis of vast amounts of data. By examining historical interactions, call centers can identify patterns and trends in customer behavior. This insight allows organizations to proactively address potential issues and enhance the quality of their customer service. Implementing these analytical techniques ensures continuous improvement in operational performance while fostering a culture of data-driven decision-making. With the support of robust analytics systems, call centers are better equipped to adapt to ever-changing customer needs.
- Genesys Cloud CX
The integration of advanced analytics into call center quality assurance has transformed how organizations evaluate their performance and customer interactions. Central to this change is a powerful tool that streamlines data management and insights extraction. By enabling the analysis of vast datasets, this platform aids in identifying trends that inform coaching and training strategies, ultimately enhancing the quality of service. Teams can shift from reactive evaluations to proactive insights, allowing for continuous improvement in customer engagement.
Utilizing this technology allows call centers to enhance performance through clearer visibility of interactions. Through its intuitive dashboards, teams can quickly access valuable information to drive coaching initiatives and refine their approach. This predictive environment fosters a culture of continuous learning, where data informs decision-making and agents are better equipped to assist customers. By focusing on strategic insights, organizations pave the way for a more tailored and satisfying customer experience.
Conclusion: The Future Impact of Predictive QA Analytics on Call Centers
Predictive QA Analytics will shape the future of call centers in transformative ways, enhancing operational efficiency and customer satisfaction. By automating the evaluation of call quality, these analytics enable teams to quickly identify performance trends, training opportunities, and areas for improvement. The ability to assess large volumes of interactions can lead to more insightful data-driven decision-making.
As organizations adopt these technologies, we can expect a shift toward more personalized customer interactions. Predictions based on customer behavior and preferences will guide the development of tailored training programs, ensuring agents can address client needs effectively. This advancement not only benefits the agents but also significantly enhances the overall customer experience.
๐ฌ Questions about The Role of Predictive Analytics in Call Center QA Evaluations?
Our team typically responds within minutes