AI-Driven Evaluations are reshaping the landscape of call centers, offering a fresh approach to performance assessments. The integration of artificial intelligence into evaluation processes allows for a more comprehensive analysis of agent interactions. By leveraging advanced algorithms, organizations can gain insights into various performance metrics, such as greeting style, customer engagement, and issue resolution.
This innovative method not only streamlines the evaluation process but also enhances accuracy and consistency. With AI-driven evaluations, call centers can effectively monitor employee performance and identify areas for improvement, ultimately fostering a more productive work environment. Embracing this technology signifies a crucial step toward advancing customer service and operational excellence.
Analyze qualitative data. At Scale.
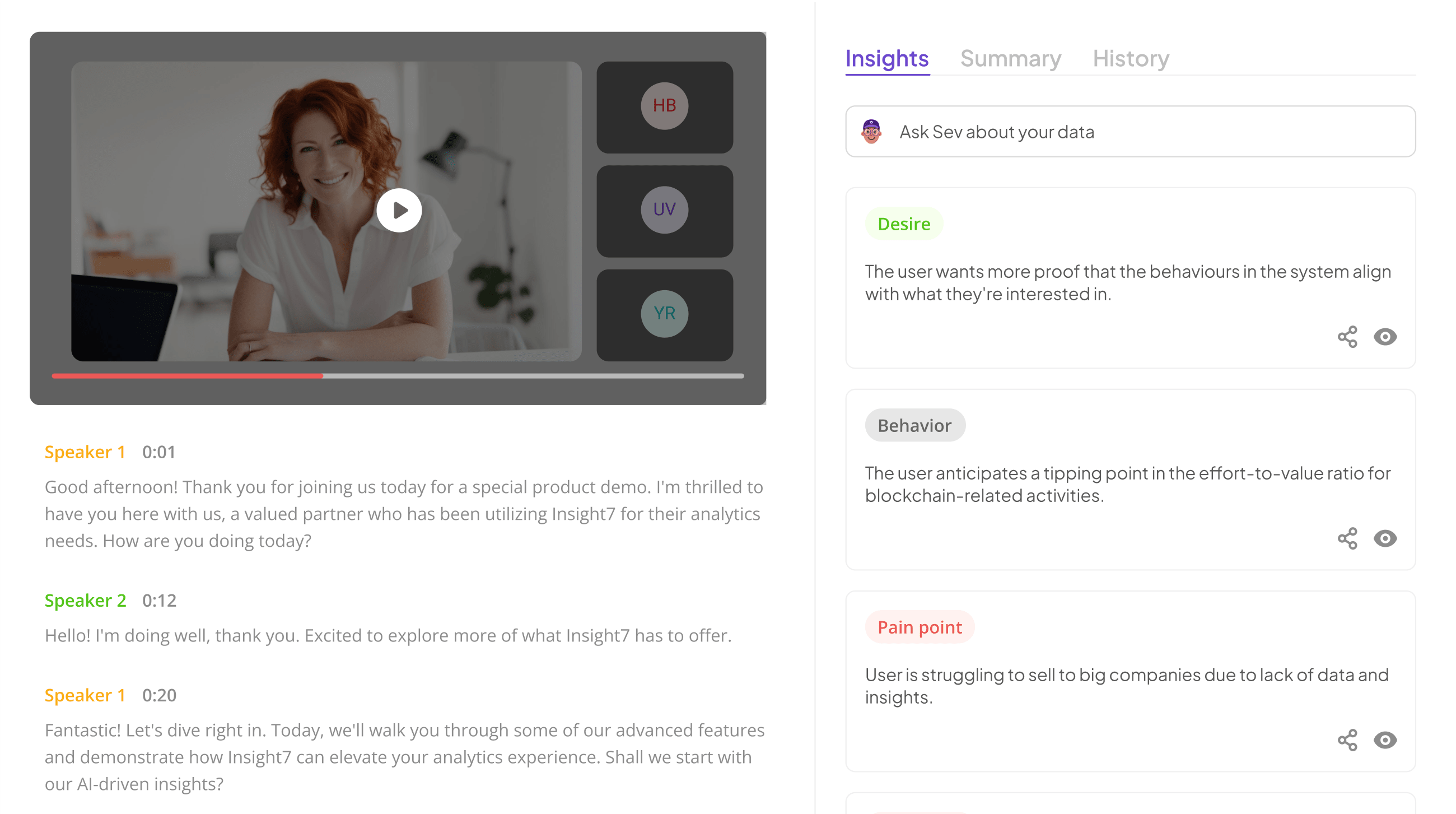
Enhancing Traditional Evaluation Methods with AI-Driven Evaluations
Traditional evaluation methods in call centers often rely on subjective assessments. By incorporating AI-Driven Evaluations, organizations can achieve a more objective and comprehensive analysis of agent performance. Machine learning tools can analyze vast amounts of data, pinpoint patterns, and provide insights that manual evaluations might overlook. This shift not only refines the evaluation process but also enhances agent training and overall performance.
To enhance these traditional methods effectively, organizations can focus on three key aspects. First, defining clear evaluation criteria ensures a consistent standard for assessments. Next, integrating AI systems facilitates real-time performance tracking, enabling supervisors to make informed decisions swiftly. Lastly, employing feedback loops allows for continuous improvement, ensuring that agents receive timely, constructive feedback. Together, these elements create a more structured and efficient evaluation process, paving the way for higher performance standards within call centers.
The Role of Machine Learning in AI-Driven Evaluations
Machine learning plays a pivotal role in enhancing AI-driven evaluations within call centers. By analyzing vast amounts of interaction data, machine learning algorithms identify patterns and trends that human evaluators may overlook. This capability allows for a more refined assessment of agent performance, promoting consistency and accuracy in evaluations. Effective AI-driven evaluations leverage this technology to deliver real-time insights, enabling teams to address performance gaps promptly.
Moreover, machine learning models continuously learn from new data, ensuring evaluations remain relevant over time. These models can easily adapt to changes in customer expectations and business objectives. This adaptability enhances the training process for agents, as they receive tailored feedback based on their interactions. Consequently, machine learning not only transforms performance assessments but also fosters a culture of continuous improvement in call center operations. By integrating AI-driven evaluations, organizations can refine their service strategies while enhancing overall customer satisfaction.
Benefits of AI-Driven Evaluations Over Traditional Methods
AI-Driven Evaluations offer several advantages over traditional performance evaluation methods in call centers. First, they provide consistency through automated scoring systems that assess calls based on predefined criteria. This reduces human biases and creates a fairer evaluation environment. With advanced algorithms, these evaluations can analyze large volumes of interactions quickly, ensuring that every call is reviewed rather than just a select few.
Moreover, AI-Driven Evaluations enhance feedback quality by identifying specific strengths and weaknesses in agent performances. This targeted feedback empowers agents to improve and adapt their skills in real-time. Additionally, these evaluations facilitate ongoing learning based on trends rather than one-off assessments. As a result, organizations can not only improve agent performance but also boost overall customer satisfaction through more personalized service. Embracing AI in call center evaluations signals a step towards a more future-focused, effective, and client-centered operational strategy.
Extract insights from interviews, calls, surveys and reviews for insights in minutes
Key Steps to Implementing AI-Driven Evaluations in Call Centers
To successfully implement AI-Driven Evaluations in call centers, organizations must follow a strategic approach. First, data collection and pre-processing are critical. Collecting diverse and high-quality data from past calls ensures the AI models can recognize patterns effectively. Ensuring that this data is cleaned and formatted properly will lay a strong foundation for analysis.
Next, applying machine learning algorithms is essential. This step involves selecting the right models to analyze call data against predefined criteria. Continuous monitoring and improvement must follow, as AI models benefit from real-time feedback. Regular assessments will identify areas for refinement, ensuring that the evaluations remain relevant and accurate. By systematically progressing through these steps, call centers can enhance their evaluation processes, leading to improved performance and customer satisfaction.
Step 1: Data Collection and Pre-Processing
In the journey towards AI-Driven Evaluations, the first step is meticulous data collection and pre-processing. This phase is pivotal, as it establishes the foundation for the machine learning models that will analyze agent performance effectively. Data can be sourced from various channels, including call recordings, customer feedback, and interaction logs, creating a rich dataset for analysis.
Once collected, the data undergoes pre-processing to enhance its quality. This process includes cleaning the data by removing irrelevant information, handling missing values, and normalizing metrics for consistent interpretation. Properly pre-processed data allows AI algorithms to extract valuable insights, identifying patterns that reflect agent performance and customer satisfaction. Ensuring accuracy in this step not only streamlines the evaluation process but also maximizes the effectiveness of subsequent AI-driven strategies, providing call centers with actionable insights that promote continuous improvement.
Step 2: Applying Machine Learning Algorithms
Step 2 involves a pivotal phase in the journey towards AI-driven evaluations in call centers. This step focuses on applying machine learning algorithms to the data collected from customer interactions. Machine learning provides the necessary backbone to identify patterns and trends that may otherwise go unnoticed. By employing various algorithms, call centers can enhance understanding of customer sentiments, performance metrics, and feedback mechanisms.
During this phase, several key techniques come into play. First, the analysis of sentiment helps in categorizing customer emotions, enabling agents to respond appropriately. Second, predictive analytics uses historical data to forecast future performance, aiding in better decision-making. Third, clustering algorithms sort interactions into distinct groups, revealing areas that may require additional training or resources. The integration of these machine learning techniques in performance evaluations transforms traditional evaluation methods, making them more accurate and actionable. This innovative approach ultimately optimizes overall call center effectiveness.
Step 3: Continuous Monitoring and Improvement
Continuous monitoring and improvement are essential components of AI-driven evaluations in call centers. Regularly assessing performance metrics allows organizations to identify areas that require enhancement. This practice helps in adapting training programs to ensure that customer service representatives meet evolving expectations and standards. Analyzing the data generated by AI systems provides insights that guide necessary adjustments and strategies.
To effectively implement continuous monitoring, consider the following steps:
Establish Key Performance Indicators (KPIs): Define specific metrics that reflect service quality and effectiveness. KPIs serve as benchmarks for evaluating performance trends.
Regularly Review Data: Set a recurring schedule for data analysis. Frequent reviews allow teams to quickly pinpoint issues and address them.
Gather Feedback: Continuously solicit input from call center staff and customers. This feedback loop provides real-time insights into service quality and areas of concern.
Iterate on Strategies: Use the insights gained from data analysis and feedback to refine performance evaluation methods. This iterative process fosters ongoing improvement and enhanced service delivery.
By focusing on these steps, call centers can harness AI-driven evaluations to achieve a culture of continuous growth and responsiveness.
Leading Tools for AI-Driven Evaluations
AI-Driven Evaluations are revolutionizing the way call center performance is assessed, enhancing accuracy and efficiency. Various leading tools are emerging in this domain, each offering unique features tailored to optimize evaluation processes. One essential tool is Insight7, which excels in capturing customer sentiments through advanced analytical capabilities. This allows call centers to understand emotional responses better and adjust their service strategies accordingly.
Another notable tool is IBM Watson, which combines natural language processing and machine learning to offer valuable insights into conversations. Its ability to identify patterns in agent behavior can pinpoint areas needing improvement. Google Cloud Contact Center AI streamlines evaluations by integrating AI-driven analytics, producing actionable results quickly. Finally, Amazon Connect provides a user-friendly interface that supports seamless integration with existing call center operations. Together, these tools set a powerful foundation for implementing AI-Driven Evaluations, ensuring improved performance in the fast-paced call center environment.
Insight7
AI-Driven Evaluations are reshaping how call centers assess their performance and drive improvement. By utilizing advanced algorithms, these evaluations sift through vast amounts of customer interactions, producing insights that traditional methods often overlook. The real-time analysis enables managers to identify improvement areas swiftly, fostering a proactive approach.
One significant advantage of AI-driven evaluations is their ability to enhance agent performance metrics beneficially. Instead of relying solely on quantitative data, qualitative aspects such as customer sentiment are integrated into evaluations. This holistic approach not only streamlines feedback but also supports training and development. Furthermore, by automating data analysis, call centers reduce the time agents and supervisors spend on evaluations, allowing them to focus more on customer engagement and satisfaction strategies. As AI continues to evolve, its potential to transform call center evaluations will undoubtedly lead to enhanced operational efficiencies and better customer experiences.
IBM Watson
IBM Watson plays a pivotal role in revolutionizing call center performance evaluations through AI-driven evaluations. By utilizing advanced algorithms and natural language processing, it can analyze conversations with remarkable accuracy. This capability allows organizations to assess agent performance and customer interactions more thoroughly than traditional methods could achieve. The use of such technology enables a real-time evaluation of engagement metrics and compliance, providing managers with instant access to valuable insights.
This AI-driven approach not only enhances the evaluation process but also assists in identifying training opportunities for agents. It generates detailed reports that highlight each agent's strengths and areas for improvement. Moreover, it reduces the time spent on manual evaluations, thereby freeing up supervisors to focus on meaningful interactions with their teams. Embracing this technology signifies a shift toward more efficient and effective call center operations.
Google Cloud Contact Center AI
Google Cloud Contact Center AI serves as a transformative resource for call centers looking to implement AI-driven evaluations. With powerful machine learning capabilities, it helps organizations enhance their performance evaluation processes. The platform enables the analysis of customer interactions, allowing businesses to gain deeper insights into agent performance and customer satisfaction. By transcribing and analyzing calls, it turns raw data into actionable feedback that can drive improvements.
Utilizing this technology, call centers can shift from traditional evaluation methods to a more data-driven approach. The system automatically identifies trends and areas for improvement, enabling quicker responses to evolving customer needs. This fosters a culture of continuous learning among agents, ultimately improving service quality and customer experience. By embracing AI-powered solutions, call centers can streamline their operations and make more informed decisions regarding agent training and customer engagement strategies.
Amazon Connect
Amazon Connect serves as a pivotal tool for enhancing call center performance evaluations through AI-driven evaluations. With its cloud-based framework, it facilitates seamless integration of machine learning technologies that can analyze and assess call interactions in real-time. Utilizing advanced algorithms, this platform transforms how agents are evaluated, providing insights that traditional methods simply cannot match.
One of the standout features is its ability to automatically capture customer sentiment and agent performance metrics, which leads to more objective evaluations. By employing AI-driven evaluations, managers can identify training needs and improve overall call quality effectively. Furthermore, the platform offers detailed reporting features that enable supervisors to monitor performance trends over time, ensuring continuous improvement.
The combination of real-time analysis and enhanced performance tracking positions Amazon Connect as a leader in the industry, unlocking unprecedented operational efficiency and customer satisfaction. As call centers increasingly adopt these technologies, the impact on performance evaluations will undoubtedly reshape the future of customer service.
Conclusion: The Future of AI-Driven Evaluations in Call Centers
AI-Driven Evaluations represent a pivotal advancement in the realm of call centers. As these systems continue to evolve, they promise unprecedented accuracy and efficiency in assessing agent performance. The integration of machine learning allows for a more nuanced understanding of customer interactions, enabling call centers to refine their evaluation processes significantly. By focusing on specific criteria, organizations can ensure that agents are held accountable to established standards.
In the future, AI-Driven Evaluations will not only streamline performance assessments but also foster a culture of continuous improvement. As AI tools gain capabilities, they will provide actionable insights, transforming raw data into valuable feedback. This shift will empower organizations to cultivate a more consultative approach, ultimately enhancing both employee and customer satisfaction. Embracing these technologies will be essential for call centers aiming to thrive in an increasingly competitive landscape.