Implementing Predictive Decision Systems Using AI Agents
-
Bella Williams
- 10 min read
AI Predictive Systems have emerged as transformative tools in today's fast-paced decision-making environment. They empower organizations to analyze vast amounts of data, drawing insights that were previously beyond reach. By harnessing these advanced systems, businesses can anticipate trends and make informed choices, ultimately enhancing productivity and efficiency across various operations.
Understanding the mechanics of AI Predictive Systems is crucial for success. These systems leverage intricate algorithms and data integration techniques to generate actionable forecasts. As organizations look to implement these technologies, a clear grasp of their capabilities will ensure that decision-makers can utilize AI effectively. Embracing this innovation will not only streamline operations but also foster a culture of proactive decision-making.
Discover the transformative potential of AI Predictive Systems in modern decision-making processes and understand their role in enhancing business efficiency.
AI Predictive Systems represent a revolutionary leap in modern decision-making, reshaping how businesses operate. These systems harness vast data to generate actionable insights, helping organizations foresee trends, identify risks, and make informed choices. By analyzing historical data, AI Predictive Systems enable companies to streamline processes and enhance efficiency, allowing for timely interventions and better resource allocation.
Moreover, the integration of AI predictive capabilities transforms traditional decision-making approaches. With predictive analytics, businesses can anticipate customer needs and market shifts, which fosters proactive strategies over reactive measures. This anticipatory nature not only boosts operational efficiency but also strengthens competitive positioning. As organizations embrace AI Predictive Systems, they can unlock new levels of agility, ensuring they are well-prepared to navigate an ever-evolving market landscape.
Analyze & Evaluate Calls. At Scale.
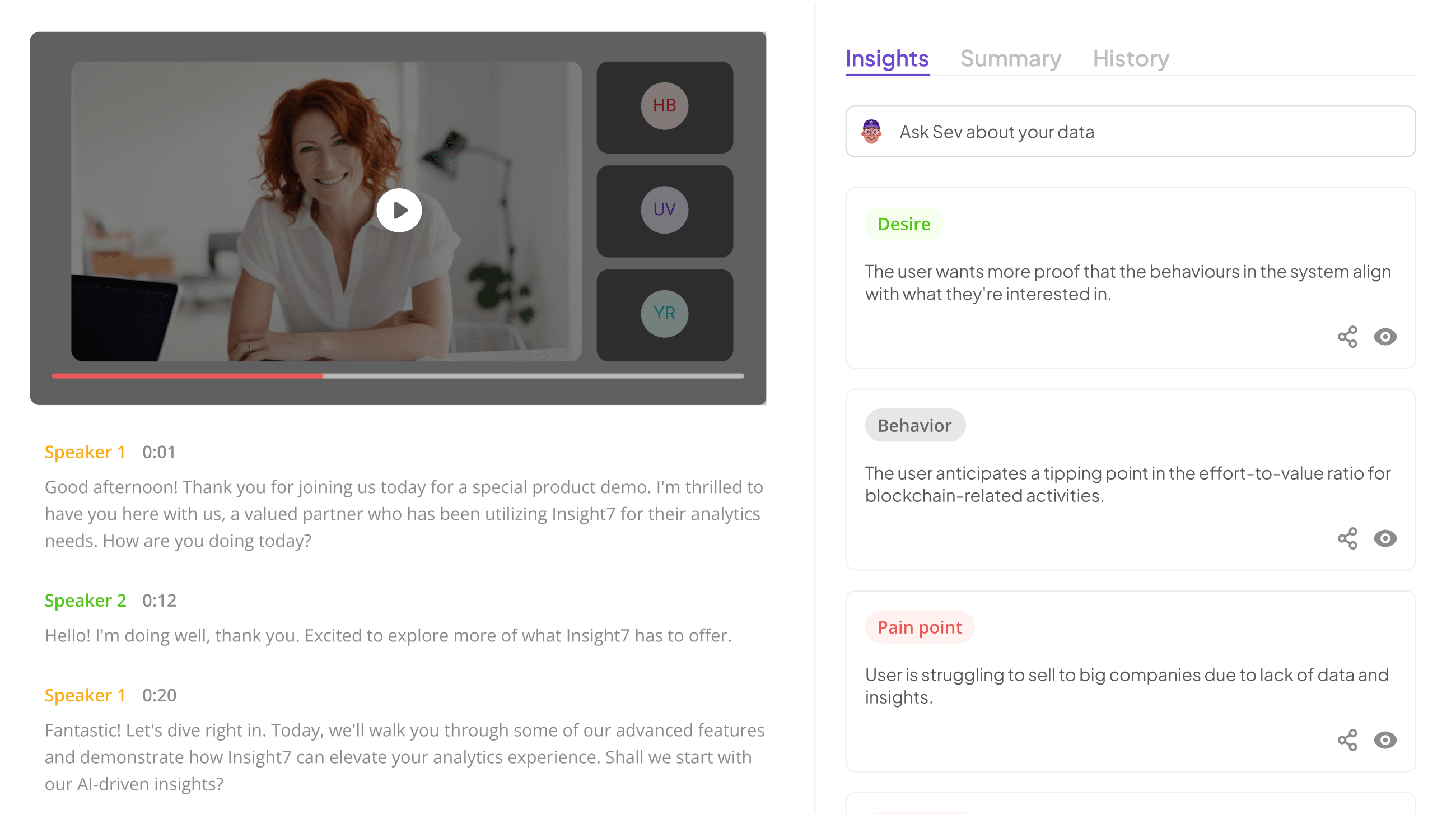
Harnessing the Power of AI Agents in Predictive Decision Systems
AI agents play a pivotal role in predictive decision systems, enabling organizations to navigate vast data landscapes with greater efficiency. By harnessing their capabilities, businesses can transform raw data into actionable insights that inform strategic decisions. These intelligent entities analyze patterns, draw correlations, and predict future outcomes, ultimately enhancing decision-making efficacy.
To effectively harness the power of AI agents in predictive decision systems, consider these essential elements. First, defining specific objectives is crucial to guide the AI's analytical process. Second, establishing clear criteria for evaluation allows organizations to assess the accuracy and reliability of AI-generated insights. Lastly, continuous monitoring and refinement ensure that the AI remains aligned with evolving business needs and external factors. Through these steps, organizations can truly leverage AI predictive systems to achieve unprecedented levels of insight and informed decision-making.
Learn how AI agents serve as the backbone of robust predictive decision systems, driving intelligent insights and automated outcomes.
AI agents play a pivotal role in enhancing the effectiveness of predictive decision systems. These intelligent entities analyze vast datasets, uncovering insights that drive informed decision-making. By continuously learning from data patterns, AI agents not only predict future trends but also adapt to changing circumstances, ensuring that organizations remain agile in their strategies.
The backbone of AI predictive systems is built on core functionalities. Firstly, data integration allows diverse information sources to be consolidated for a comprehensive analysis. Secondly, sophisticated algorithms empower AI agents to process and interpret complex data efficiently. Thirdly, user interfaces make insights accessible, facilitating quick and reliable decision-making. In leveraging these components, businesses can automate outcomes and react proactively to market shifts, enhancing performance and minimizing risks. Ultimately, the synergy between AI agents and predictive decision systems propels organizations toward superior operational efficiency and insight-driven actions.
Key Components of AI Predictive Systems
AI Predictive Systems rely on several critical components that work together to enhance decision-making capabilities. The first component is data integration, foundational for these systems as they aggregate and preprocess data from various sources. This ensures that the system has access to comprehensive and relevant information for accurate predictions.
Another essential element involves sophisticated algorithms, which analyze the integrated data and derive actionable insights. These algorithms play a vital role in identifying patterns and trends that may not be immediately apparent. Lastly, intuitive user interfaces are crucial, allowing stakeholders to interact seamlessly with the system and make informed decisions based on the predictions provided. Together, data integration, algorithms, and user interfaces form the backbone of effective AI Predictive Systems, significantly improving organizational efficiency.
Implementing these components thoughtfully can transform traditional decision-making processes, paving the way for enhanced performance and competitive advantage.
Examine the critical elements that form effective AI Predictive Systems, including data integration, algorithms, and user interfaces.
Effective AI Predictive Systems rely on three critical elements: data integration, algorithms, and user interfaces. First, data integration is paramount, as it brings together disparate data sources into a cohesive framework. This allows for more accurate predictions by ensuring that relevant and diverse data informs the decision-making process. Rather than relying on isolated datasets, a comprehensive approach enables systems to capture a fuller picture of the variables impacting outcomes.
Next, the choice of algorithms plays a decisive role in the functionality of AI Predictive Systems. Sophisticated algorithms analyze integrated data to uncover patterns and trends that would otherwise go unnoticed. These intelligent mechanisms continuously learn and adapt, enhancing their predictive capabilities over time. Lastly, user interfaces must be intuitive and user-friendly, facilitating interactions between users and the predictive systems. A well-designed interface ensures that users can easily interpret and act upon insights, ultimately maximizing the efficacy of AI-driven predictions. Overall, these elements work synergistically to create robust and effective AI Predictive Systems.
Implementing AI Agents for Superior Decisions
Implementing AI agents into existing decision-making frameworks can elevate decision quality significantly. This process begins by assessing the current capabilities of your system, ensuring a solid foundation for integration. Understanding existing processes is crucial, as it allows for identifying gaps where AI can add value. Once these capabilities are evaluated, a structured design for the AI agent should be developed, tailored to the specific needs of the organization and its objectives.
Following the design phase, it's essential to seamlessly integrate the AI agents into the existing workflows. This involves collaboration among team members to ensure all components work harmoniously. Finally, monitoring and optimizing the performance of these AI agents will ensure continual improvement. By embracing this structured approach, organizations can leverage AI predictive systems effectively to achieve superior decision-making outcomes.
Explore the step-by-step approach to incorporating AI agents into existing decision-making frameworks for optimal results.
Incorporating AI agents into existing decision-making frameworks requires a structured approach to ensure effective integration and optimal results. The first step involves assessing current system capabilities. Itโs essential to identify existing processes, technologies, and data sources, which helps determine how AI can be most beneficial.
Next, you need to design the AI agent structure. This involves defining the specific roles of the AI agents, focusing on their goals and the criteria for success. Once the structure is established, integrate the AI agents with existing workflows. Creating seamless interactions between human decision-makers and AI allows for enhanced collaboration. Finally, continuous monitoring and performance optimization are crucial for improving accuracy and efficiency over time. By following this structured path, AI can transform decision-making processes into data-driven frameworks that yield superior results.
- Step 1: Assess Current System Capabilities
To effectively implement AI Predictive Systems, it is essential to begin by assessing current system capabilities. This initial step provides a comprehensive understanding of existing data sources, processes, and infrastructure. Identifying these elements allows organizations to pinpoint gaps, enabling more informed decisions as they transition to AI-driven solutions. Understanding what systems are already in place helps ensure that new AI agents can integrate seamlessly with existing workflows.
A detailed evaluation focuses on three key areas: data quality, system integration, and user needs. First, analyze the quality and reliability of the data at hand; this determines the efficacy of AI models. Next, assess how current systems communicate with each other, as smooth integration is crucial for successful implementation. Finally, engaging with end-users provides insights into their challenges and requirements, ensuring that the predictive systems developed address real needs. By thoroughly assessing current capabilities, organizations can create a solid foundation for deploying effective AI Predictive Systems.
- Step 2: Design AI Agent Structure
Designing an effective AI agent structure is crucial for the successful implementation of AI Predictive Systems. The first step is to define the specific role of the AI agent in the decision-making process. This includes determining its functionalities, such as data analysis, decision support, or user interaction. By clearly outlining these roles, organizations can ensure that the AI agent effectively meets their predictive needs.
Next, focus on the architecture of the AI agent. This involves selecting appropriate algorithms and data sources that align with the intended tasks. Additionally, consider the integration of user interfaces to facilitate interaction between the AI agent and end-users. An intuitive interface enhances user engagement and helps in translating complex data insights into actionable decisions. This thoughtful design enhances the overall performance of AI Predictive Systems and empowers businesses to leverage data-driven strategies effectively.
- Step 3: Integrate with Existing Workflows
Integrating AI predictive systems into existing workflows is a pivotal step in harnessing their full potential. Initially, evaluate current processes to identify pain points that these systems can address. Understand how AI can streamline workflows by automating repetitive tasks, enabling teams to focus on high-value activities. This integration should foster seamless collaboration between AI agents and human intuition, enhancing decision-making quality.
To achieve this, consider the following steps:
- Map Current Workflows – Document existing processes to uncover where AI intervention could yield immediate benefits.
- Identify Integration Points – Determine specific touchpoints where AI systems can be introduced to automate or augment decision-making.
- Deploy with User Training – Ensure personnel are equipped to work alongside AI tools through focused training sessions on new technology.
- Monitor User Feedback – Collect ongoing feedback to understand user experience and make necessary adjustments to improve system efficacy.
By systematically weaving AI predictive systems into the fabric of current workflows, organizations can empower their teams with insights that drive efficient and informed decisions.
- Step 4: Monitor and Optimize Performance
Monitoring and optimizing performance within AI Predictive Systems is crucial for experiencing their full potential. Regular assessment helps identify bottlenecks, inaccuracies, and areas for enhancement. By analyzing how AI agents are performing against established benchmarks, organizations can fine-tune their algorithms to better align with evolving business needs and market conditions.
To effectively monitor performance, consider the following steps:
Collect Data Continuously: Ensure that you gather performance data consistently to track your AI system's efficiency over time. This helps in identifying trends and making informed adjustments.
๐ฌ Questions about Implementing Predictive Decision Systems Using AI Agents?
Our team typically responds within minutes
Set Clear Metrics: Define measurable KPIs that align with your business objectives. These metrics will serve as a foundation to evaluate the AIโs effectiveness.
Feedback Loop Implementation: Create a process for regular feedback. This could include user experiences or automated system reviews, allowing proactive updates and refinements.
By maintaining a cycle of continuous improvement, organizations ensure that AI Predictive Systems stay relevant and impactful in their decision-making processes. Adaptability in monitoring strategies will foster better outcomes.
Extract insights from interviews, calls, surveys and reviews for insights in minutes
Top Tools for Implementing AI Predictive Systems
When implementing AI Predictive Systems, selecting the right tools is crucial for success. A few standout options can significantly enhance the predictive capabilities of decision-making frameworks. Insight7, for instance, offers user-friendly interfaces that democratize access to valuable insights, enabling anyone in an organization to engage with data effectively. This feature is essential for fostering a culture around data-driven decisions.
Another powerful tool is TensorFlow, which provides robust frameworks for developing machine learning models. Its flexibility allows businesses to train and deploy AI Predictive Systems that cater precisely to their unique needs. H2O.ai stands out for its automated machine learning capabilities, enabling users to build models quickly and efficiently. Additionally, platforms like IBM Watson and RapidMiner provide comprehensive solutions that combine data analytics with AI, streamlining the predictive modeling process from start to finish. By employing these tools, organizations can ensure their AI Predictive Systems are not only effective but also adaptable to evolving market demands.
Access a curated list of top tools that facilitate the creation and management of AI Predictive Systems.
Accessing a curated list of top tools is essential for anyone looking to create and manage AI Predictive Systems effectively. These tools provide invaluable resources, enabling users with varying expertise to access powerful functionalities without extensive technical training. By selecting tools designed for intuitive use, businesses can ensure broader participation in data-driven decision-making processes.
Our curated list showcases significant tools that stand out in facilitating predictive systems. For instance, platforms like TensorFlow and H2O.ai offer robust frameworks for building machine learning models. Similarly, IBM Watson provides comprehensive analytics capabilities, making it simpler to derive actionable insights from complex data sets. RapidMiner also supports the integration of various data sources, empowering organizations to enhance their predictive accuracy. By exploring these tools, businesses can significantly streamline operations and harness the full potential of AI in decision-making systems.
Featured Tools for Efficient AI Integration
Efficient AI integration is essential for successful implementation of predictive decision systems. Specific tools can significantly enhance this process by providing user-friendly interfaces and powerful capabilities. Notably, tools like TensorFlow and IBM Watson offer robust frameworks for building AI applications that seamlessly integrate into existing workflows. Their ease of use democratizes access, enabling team members without technical expertise to utilize AI effectively.
In addition, platforms such as RapidMiner and H2O.ai simplify data analytics, making it easier to derive insights from a variety of sources. These tools not only streamline data management but also fine-tune AI predictive systems to produce reliable outcomes. Enabling such targeted integration empowers businesses to harness the full potential of AI agents, enhancing decision-making processes across the organization. By employing these tools, companies can achieve remarkable efficiency and gain a competitive advantage in their markets.
Dive into specific tools that streamline the predictive decision-making process.
Exploring the right tools is essential in enhancing the predictive decision-making process. Several AI Predictive Systems can significantly streamline operations, making them accessible to a broader audience. One prominent tool is insight7, which democratizes data analysis through an intuitive interface, ensuring users can gather vital insights without deep technical expertise.
Another noteworthy option is TensorFlow, renowned for its robust machine learning capabilities. This framework allows developers to build custom predictive models tailored to their unique data sets. While H2O.ai offers automated machine learning, simplifying the model selection process greatly, IBM Watson excels in natural language processing, enabling insightful interpretations of customer feedback. Lastly, RapidMiner provides an end-to-end data science platform, combining preparation, modeling, and deployment functionalities seamlessly. Collectively, these tools empower businesses to make smarter, data-driven decisions effectively.
- insight7
Integrating AI Predictive Systems into decision-making processes represents a significant step towards modern efficiency. These systems utilize advanced algorithms and data integration techniques to transform vast pools of information into actionable insights. Organizations are increasingly recognizing that timely access to insights can propel them ahead of competitors, enabling smarter, faster decision-making.
The challenges many businesses face, such as inefficient collaboration and slow data analysis, underscore the need for robust AI solutions. By systematically incorporating AI agents, organizations can enhance their decision frameworks. For example, they must first assess their current capabilities to identify gaps. Subsequent steps include designing an effective AI agent structure and ensuring seamless integration with existing workflows. Finally, ongoing monitoring and optimization are crucial for maintaining peak performance. This strategic approach not only streamlines operations but also fosters a culture of data-driven decision-making.
- TensorFlow
TensorFlow stands out as a pivotal tool in implementing AI Predictive Systems, due to its robust capabilities in machine learning and deep learning. This open-source library enables developers to construct complex algorithms that can analyze vast datasets efficiently. In the realm of predictive systems, TensorFlow allows for advanced modeling, making it easier to derive insights from historical data.
Utilizing TensorFlow, AI agents can process and learn from patterns in data, leading to more accurate forecasts. Its architecture supports both CPU and GPU computing, ensuring rapid processing times that are essential for real-time applications. Furthermore, its extensive community and documentation provide significant resources for developers looking to enhance their AI Predictive Systems. By choosing TensorFlow, teams gain access to a proven platform that can significantly improve decision-making processes.
In summary, TensorFlow serves as a critical enabler of innovative AI Predictive Systems, equipping developers with the tools needed to transform data into actionable insights effectively.
- H2O.ai
In the realm of AI Predictive Systems, innovative technologies play a crucial role in shaping decision-making processes. One such technology is transforming data analysis through its efficient algorithms, making it easier for organizations to derive insights and predict outcomes. These systems utilize vast amounts of data to generate predictive models that help in understanding trends and guiding decisions.
To implement these AI Predictive Systems effectively, organizations can follow key steps. First, assessing current system capabilities allows for identifying gaps in functionality. Next, designing a structured AI agent aligned with the organizationโs goals ensures clarity in implementation. Following this, integrating AI agents with existing workflows promotes seamless operation and maximum efficiency. Finally, regular monitoring and optimization of performance lead to sustained improvements and accuracy over time.
By focusing on these strategies, companies can create robust decision-making frameworks powered by advanced AI technologies. This not only enhances efficiency but also positions organizations to stay ahead in competitive markets.
- IBM Watson
IBM Watson stands out as an advanced tool for developing AI predictive systems, offering robust capabilities that enhance decision-making. In the realm of data analysis, it enables users to harness vast datasets effectively, transforming raw information into actionable insights. By utilizing natural language processing and machine learning, it empowers organizations to predict trends, customer behaviors, and operational efficiencies, all of which are crucial for competitive advantage.
With its user-friendly interface, workplaces can integrate this technology seamlessly into their existing frameworks. The AI-powered platform can automatically analyze data points, providing immediate feedback and recommendations. This immediacy allows teams to make informed decisions swiftly, guiding strategic planning and resource allocation. Such innovative functionality positions it as an invaluable partner in implementing AI predictive systems for businesses aiming to thrive in a data-driven environment.
- RapidMiner
RapidMiner serves as a powerful tool specifically designed for building AI Predictive Systems. Utilizing a user-friendly interface, it simplifies the process of data analysis, making it accessible to a wider range of users. This platform enables organizations to integrate various data sources seamlessly, allowing for comprehensive insights that fuel decision-making.
One of the standout features of this tool is its project-centric approach. Users can create projects that compile different datasets, facilitating a thorough analysis of trends and patterns. Furthermore, the integration options with external data sources enhance the platform's versatility. This capability ensures that organizations can harness real-time data to refine their predictive models, leading to more informed decisions. Overall, the combination of ease-of-use and robust functionality positions RapidMiner as a critical asset in the development and implementation of effective AI Predictive Systems.
Conclusion on AI Predictive Systems
AI Predictive Systems represent a significant advancement in decision-making, allowing organizations to navigate complex data landscapes with enhanced accuracy. By harnessing intelligent algorithms and real-time data analysis, these systems pave the way for informed strategies that can adapt to changing market conditions. As businesses increasingly rely on these advancements, the need for effective implementation becomes paramount.
In conclusion, the integration of AI Predictive Systems equips organizations with powerful tools to make data-driven decisions. Embracing this technology not only fosters operational efficiency but also promotes a proactive approach to challenges and opportunities, ensuring a competitive edge in the dynamic business environment.
Reflect on how leveraging AI Predictive Systems can propel organizations towards smarter, data-driven decision-making for a competitive edge.
AI Predictive Systems are transforming how decisions are made in todayโs complex environments. By analyzing vast amounts of data, these systems offer insights that go beyond mere observations, providing predictive outcomes that organizations can rely on. This capability allows decision-makers to move away from intuition-based strategies and adopt a more data-driven approach. Such informed decisions can lead to enhanced efficiency, reduced risks, and ultimately, a significant competitive edge.
To effectively integrate AI Predictive Systems, a structured approach is essential. First, organizations should assess their current data capabilities and decision-making processes. Next, designing the right AI agent structure helps tailor predictions to specific needs. Following integration with existing workflows, ongoing monitoring ensures the systems adapt and improve over time. By embracing this technology, organizations can not only stay ahead of market trends but also respond swiftly to changes, fostering a culture of agility and innovation.
Analyze & Evaluate Calls. At Scale.
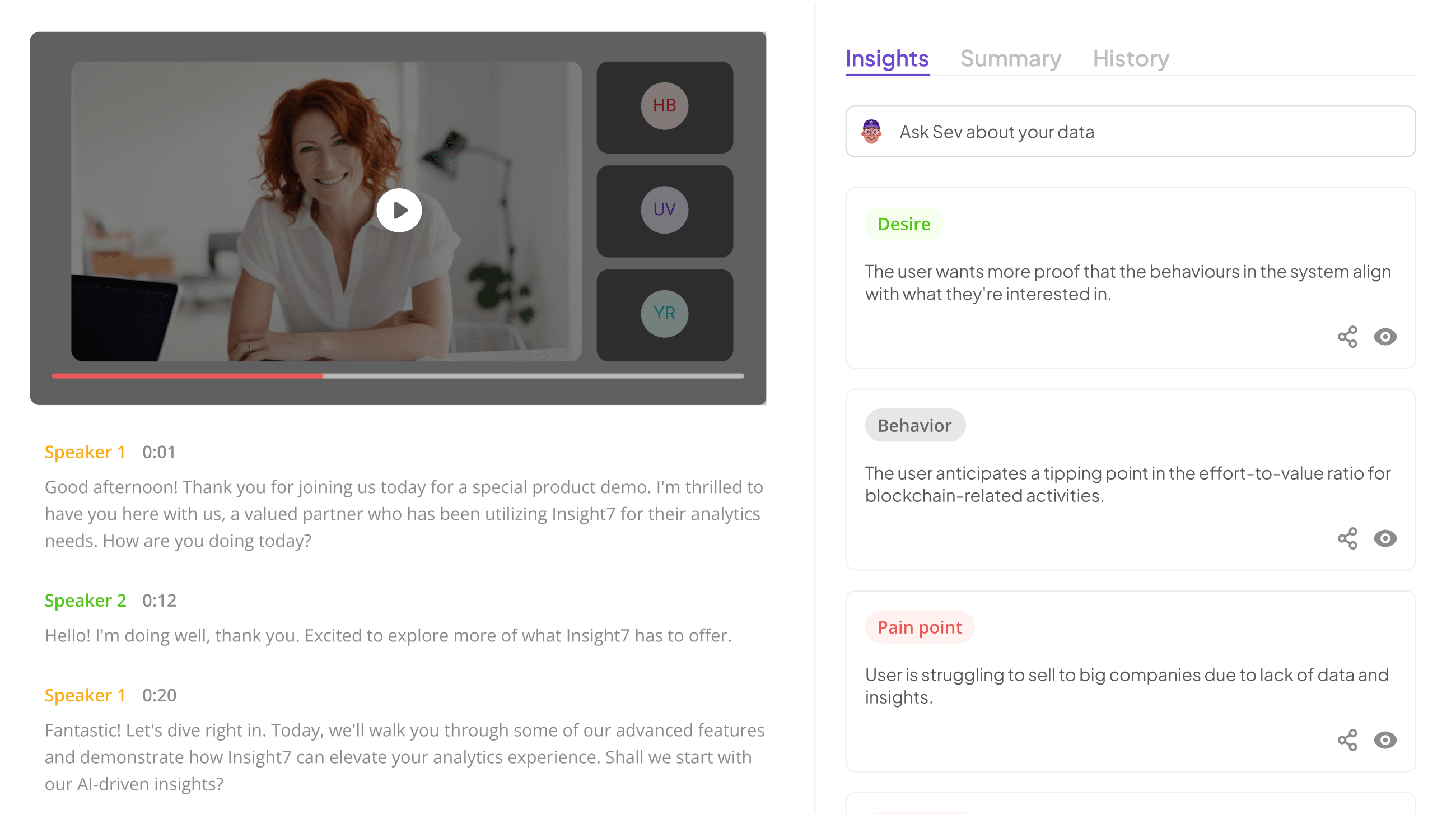
๐ฌ Questions about Implementing Predictive Decision Systems Using AI Agents?
Our team typically responds within minutes