AI Strategic Decisioning embodies a paradigm shift in how modern enterprises approach decision-making. As organizations encounter an unprecedented volume of data, traditional methods often fall short. By harnessing AI, businesses can navigate complexities with enhanced precision and agility.
With AI Strategic Decisioning, companies can analyze vast datasets, identifying patterns and insights that inform strategic choices. This not only streamlines decision processes but also fosters a culture of data-driven innovation. Implementing these models requires clarity in objectives, thoughtful data management, and the selection of appropriate AI tools. Embracing this approach can lead to sustainable growth and a competitive edge in a rapidly evolving market.
Analyze qualitative data. At Scale.
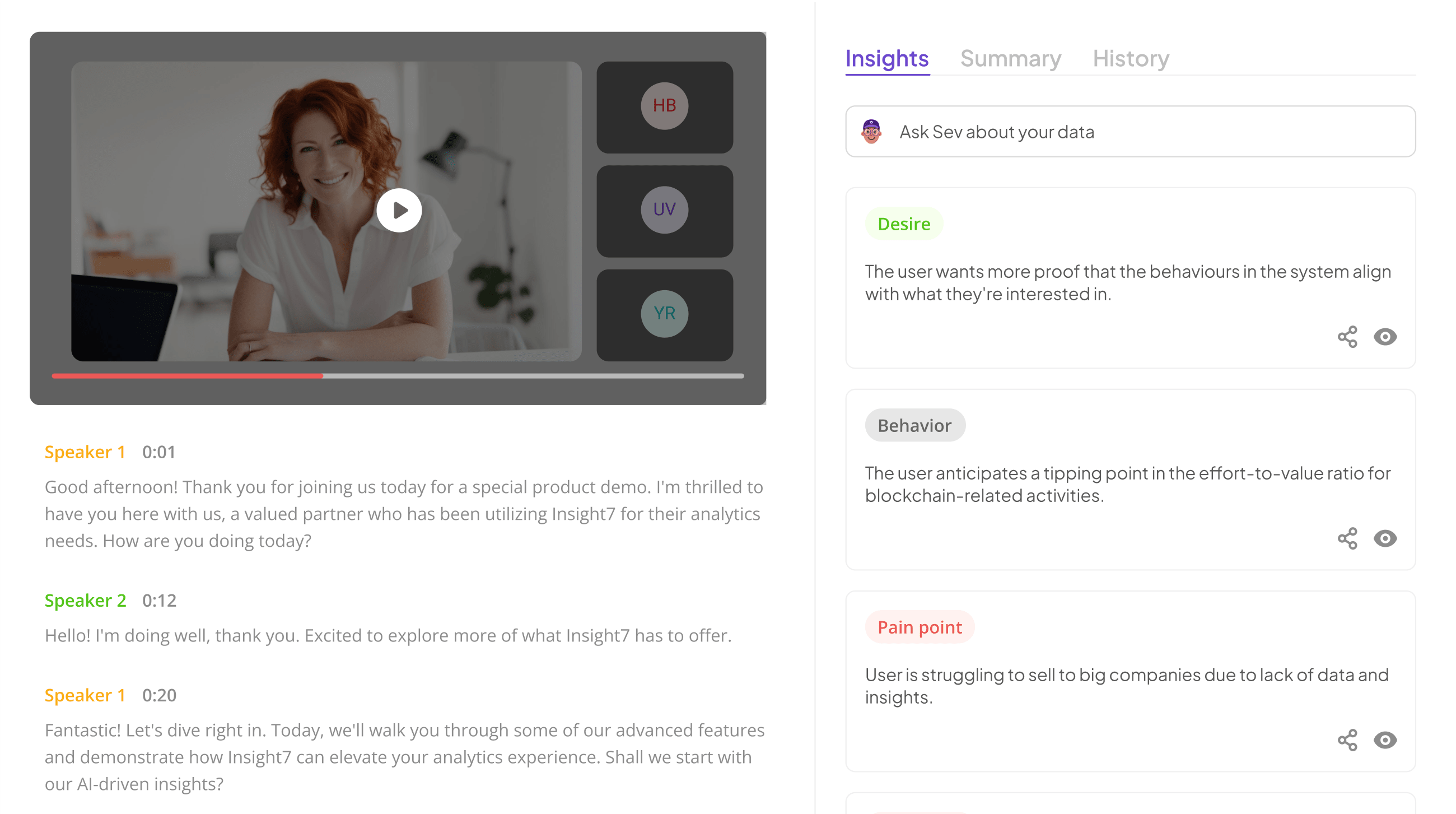
Understanding AI Strategic Decisioning Models
AI Strategic Decisioning encompasses a structured approach to integrate artificial intelligence into the decision-making process. Understanding this model requires familiarity with several key elements, including data analysis, predictive analytics, and outcome optimization. This strategic framework empowers organizations to leverage AI technologies to make informed decisions efficiently and effectively.
To fully grasp AI Strategic Decisioning, it's crucial to explore its components. First, the role of data is fundamental; high-quality, relevant data serves as the backbone for AI analysis. Next, understanding predictive analytics helps in forecasting potential outcomes based on historical data. Finally, the continuous monitoring and adjusting of AI models allow businesses to refine their strategies, ensuring that decisions align closely with evolving market conditions. Engaging with these elements prepares organizations to implement AI-driven strategies successfully and maximizes the benefits of this innovative approach.
The Concept of AI in Decision Making
AI strategic decisioning empowers organizations to harness data for informed choices. By integrating AI into decision-making processes, businesses can analyze vast datasets efficiently, leading to quicker, more accurate outcomes. This approach allows leaders to identify patterns and trends that may be overlooked in traditional methodologies.
The role of AI in decision making extends beyond mere data processing; it involves predictive analytics and machine learning. These technologies enable organizations to simulate various scenarios, assess risks, and optimize strategies. As a result, businesses can focus not just on immediate goals but also on long-term sustainability. Implementing AI strategic decisioning fosters a culture of data-driven leadership, enhancing organizational agility and responsiveness to market changes.
Understanding these core benefits is essential for organizations aiming to innovate and stay competitive in a rapidly evolving landscape.
Benefits of Implementing AI Strategic Decisioning
Implementing AI Strategic Decisioning offers numerous benefits that can significantly enhance operational efficiency and decision-making quality. First, businesses can utilize AI to analyze vast amounts of data quickly and accurately, thus identifying patterns and trends that may not be readily apparent to human analysts. This capability enables organizations to make data-driven decisions that are timely and informed, ultimately leading to better outcomes.
Additionally, AI Strategic Decisioning reduces the risk of human error in analysis and forecasting, which can often result from cognitive biases or oversight. By relying on AI technologies, companies can standardize decision-making processes and maintain consistency across various departments. Furthermore, this approach fosters a culture of innovation, encouraging teams to explore creative solutions without being limited by traditional constraints. Overall, the integration of AI in strategic decision-making not only streamlines processes but also positions companies to respond more swiftly to market dynamics.
Steps to Implement AI-Powered Strategic Decision Models
To effectively implement AI-powered strategic decision models, organizations must follow a systematic approach. First, clearly define business objectives that the AI models will address. These objectives guide the decision-making process and ensure alignment with organizational goals. Knowing what you want to achieve allows stakeholders to remain focused on desired outcomes, making it easier to measure success.
Next, prioritize data collection and processing. Gathering high-quality data is crucial for building accurate models. This involves identifying the data sources that best suit your objectives, cleaning and organizing the data to improve its usability, and ensuring it is representative of the scenarios the AI will analyze. Following this, select the right AI tools tailored to your business needs. Tools like IBM Watson Studio and Google AutoML provide various functionalities that can enhance AI strategic decisioning. With these steps, organizations can successfully harness AI for improved decision-making.
Extract insights from interviews, calls, surveys and reviews for insights in minutes
Step 1: Defining Business Objectives
In the journey of implementing AI-powered strategic decision models, the first step involves defining clear business objectives. This phase lays the groundwork for successful decision-making by identifying what the organization aims to achieve through AI strategic decisioning. Establishing specific goals helps ensure that all subsequent efforts are aligned with the broader vision of the organization.
To effectively define these objectives, consider the following key aspects:
Understanding Stakeholder Needs: Engage with stakeholders to gather their insights and requirements. This collaboration fosters alignment and sets clear expectations.
Establishing Measurable Goals: Formulate objectives that are both ambitious yet quantifiable. Measurable goals facilitate tracking progress and determining success.
Identifying Key Performance Indicators (KPIs): Determine the KPIs that will be used to assess the effectiveness of AI initiatives. This step aids in consistently evaluating success against the defined objectives.
By clarifying business objectives at the outset, organizations can effectively leverage AI strategic decisioning to enhance their decision-making processes and drive meaningful improvements.
Step 2: Data Collection and Processing
Effective AI-powered strategic decisioning relies heavily on the quality of data collection and processing. This step involves gathering relevant data from various sources to gain a comprehensive view of the problem at hand. It is essential to identify which data types will inform your AI model effectively, as different datasets can yield various insights. Structured data, such as databases and spreadsheets, as well as unstructured data, like customer feedback and social media comments, should be considered during collection.
Once data is collected, the processing phase is crucial to ensure its usability. This includes cleaning the data to remove inaccuracies, normalizing data formats, and structuring it appropriately for analysis. By applying strong preprocessing techniques, you enhance the reliability of the insights generated. Consistent and high-quality data processing sets the foundation for AI strategic decisioning to yield actionable results that align with your business objectives.
Step 3: Selecting the Right AI Tools
Selecting the right AI tools is crucial for effective AI-powered strategic decision-making. Begin by identifying your specific needs and objectives. This will guide you in evaluating which tools align best with your business goals. Consider factors such as scalability, ease of use, and integration capabilities. Look for tools that specialize in AI strategic decisioning to ensure they provide the necessary functionalities to enhance your decision-making processes.
Next, evaluate the recommended tools based on capabilities and user feedback. For instance, platforms like DataRobot and IBM Watson Studio offer robust modeling features, while H2O.ai excels in predictive analytics. Test these tools in small projects to gauge their effectiveness in addressing your unique challenges. This systematic selection process will not only streamline your strategy but also enhance the overall impact of AI on your organizational decision-making efforts.
Recommended Tools for AI Strategic Decisioning
Selecting the appropriate tools is vital for effective AI strategic decisioning. Various platforms offer robust features tailored for AI-driven insights. Noteworthy options include insight7, DataRobot, H2O.ai, Google AutoML, and IBM Watson Studio. Each of these tools has distinct functionalities that can enhance decision-making processes.
Insight7 excels in customer insights, simplifying data analysis and making it accessible for strategic planning. DataRobot provides an automated environment for building, deploying, and maintaining models quickly and efficiently. Meanwhile, H2O.ai is perfect for users who seek an open-source alternative that fosters deep learning applications. Google AutoML stands out for its user-friendly design and scalability, enabling both novices and experts to create custom models. Finally, IBM Watson Studio combines data science tools and collaborative features to streamline decision-making. Choosing the right tool depends on specific organizational needs, ensuring efficient and informed AI strategic decisioning.
- insight7
In the realm of AI Strategic Decisioning, organizations must prioritize a structured approach to implement effective models. Start by defining clear business objectives that will guide the decision-making process. This creates a foundation for aligning AI capabilities with desired outcomes, ensuring that the technology supports strategic goals.
Next, focus on gathering relevant data efficiently. Quality data is essential for training AI models, as it influences the insights derived from analyses. Once the data is in place, selecting the appropriate AI tools becomes crucial. Identifying tools that complement your specific needs will enhance the effectiveness of your AI-powered models.
Lastly, developing and testing your models should be an iterative process. By continuously monitoring performance, organizations can adapt and refine their strategies over time. This proactive approach to AI Strategic Decisioning not only strengthens operational capabilities but also positions businesses to remain competitive in a rapidly evolving landscape.
- DataRobot
DataRobot facilitates AI strategic decisioning by providing powerful tools for data analysis and predictive modeling. As organizations increasingly turn to AI to enhance their decision-making processes, the platform offers a user-friendly interface that streamlines model development. This is particularly beneficial for teams looking to harness the power of machine learning without extensive technical expertise.
To effectively implement AI-powered strategic decision models using DataRobot, it's essential to consider the following points:
Data Integration: Consolidate diverse datasets from multiple sources to ensure comprehensive analysis. This step is crucial for creating a rich foundation for predictive models.
Automated Model Selection: The platform automatically evaluates numerous algorithms to identify the best fit for your specific objectives. This eliminates the guesswork and manual effort typically required in model selection.
Performance Monitoring: Once models are deployed, continuous monitoring is necessary to ensure they adapt to changing business environments. The platform provides insights that help refine models over time.
By following these recommendations, organizations can effectively leverage DataRobot for AI strategic decisioning, driving informed choices that enhance operational efficiency and foster growth.
- H2O.ai
When exploring AI strategic decisioning, it's essential to consider robust analytical tools that enhance decision-making processes significantly. Among the available options, one prominent choice stands out for its advanced features and user-friendly interface, facilitating comprehensive data analysis. These tools empower organizations to harness vast datasets to derive actionable insights, streamlining strategies that lead to informed business decisions.
The attractiveness of AI strategic decisioning tools lies primarily in their capability to automate and optimize workflows. They assist in efficiently processing large quantities of data, generating predictive analytics that drive strategic planning. By integrating these tools into existing business systems, users can gain a competitive advantage, transforming raw data into valuable insights that guide future endeavors. Ultimately, adopting such technology can significantly bolster an organization's strategic framework, aligning actions with market demands more effectively.
- Google AutoML
Harnessing the power of automation can significantly enhance your AI strategic decisioning process. This technology allows users to create and optimize machine learning models without deep expertise in data science. By automating the model creation process, organizations can save valuable time, allowing teams to focus on strategic analysis rather than technical complexities. This approach helps turn raw data into actionable insights that drive effective decision-making.
The platform offers visual tools and pre-built templates to streamline the process of training models. Users can simply upload relevant datasets, define objectives, and initiate training cycles to fine-tune their models. This facilitates rapid experimentation and iteration, which is crucial for adapting to rapidly changing business environments. Integrating such tools into your strategic decision-making framework can thus empower teams to uncover insights that directly impact business outcomes and inform future strategies.
- IBM Watson Studio
IBM Watson Studio serves as a pivotal resource for organizations aiming to implement AI-powered strategic decision models. This platform provides a user-friendly interface that democratizes access to advanced analytics, enabling team members at all levels to harness insights without requiring extensive training. With its capabilities, teams can efficiently aggregate and analyze data from numerous sources to inform their strategic decision-making processes.
Within this platform, users can execute a variety of tasks, such as transcribing customer interactions or visualizing insights from multiple datasets. By utilizing the built-in analytics, organizations can identify key pain points, customer desires, and behavior patterns. This data-driven approach enhances collaborative efforts in addressing strategic challenges and improves overall decision-making. As organizations evolve, integrating such tools into daily operations will be crucial for maintaining a competitive edge in AI strategic decisioning.
Step 4: Model Development and Testing
In Step 4 of implementing AI-powered strategic decision models, the focus shifts to model development and testing. This stage is critical for transforming your strategy into actionable AI models that support decision-making. Start by selecting a suitable algorithm that aligns with your business objectives, ensuring it can analyze data efficiently and accurately. The model should be trained on the data gathered in earlier steps, followed by validating it using a distinct dataset to ensure robustness and reliability.
Testing is essential to identify any flaws or biases within the model. Here, iterative testing and fine-tuning come into play, allowing for adjustments based on performance metrics. It's vital to continuously monitor the model's effectiveness and refine it as new data becomes available or market conditions change. Success in this phase sets the foundation for effective AI strategic decisioning, enabling your organization to harness insights for informed choices.
Step 5: Deployment and Monitoring
Deployment and monitoring are crucial for the success of any AI strategic decisioning model you implement. After developing your model, the next step is to deploy it effectively within your business environment. This involves integrating the model into existing workflows and ensuring that it operates smoothly in real-time decision-making scenarios. A well-structured deployment will facilitate access to AI-driven insights and foster a culture of data-driven decision-making.
Once deployed, continuous monitoring is essential. This phase involves tracking the performance of your AI model to ensure it meets the established objectives. Regular evaluations help identify any discrepancies, enabling timely adjustments and optimizations. Utilize feedback mechanisms and performance metrics to assess how well the model is functioning. This proactive approach not only helps maintain model accuracy but also enhances trust in the AI strategic decisioning process within your organization.
Conclusion: The Future of AI Strategic Decisioning in Business
The future of AI strategic decisioning in business looks exceptionally promising, with the potential to transform how companies operate. As organizations increasingly adopt AI solutions, the ability to make data-driven decisions will enhance efficiency and drive profitability. This shift not only streamlines operations but also empowers teams to focus on strategic initiatives rather than routine tasks, enabling a more proactive business approach.
Moreover, the integration of AI in decision-making processes provides unprecedented insights into consumer behavior and market trends. By harnessing these insights, companies can adapt quickly to changes in the marketplace. Thus, businesses that invest in developing robust AI-powered strategic decision models will not only stay competitive but also thrive in an ever-evolving landscape.