How AI Agents Optimize Data-Driven Decision Pathways
-
Bella Williams
- 10 min read
AI Decision Optimization plays a pivotal role in enhancing data-driven decision pathways, where every choice is informed by analysis and insight. As organizations strive for efficiency and accuracy, understanding how AI can transform decision-making processes becomes crucial. By utilizing AI, businesses can analyze historical data patterns and predict outcomes with remarkable precision.
Moreover, AI agents streamline complex workflows, allowing decision-makers to focus on strategic initiatives rather than being bogged down by manual analysis. Optimized decision pathways not only improve operational efficiency but also foster better customer insights. Therefore, embracing AI in decision processes is essential for organizations aiming to thrive in a data-centric world.
Analyze & Evaluate Calls. At Scale.
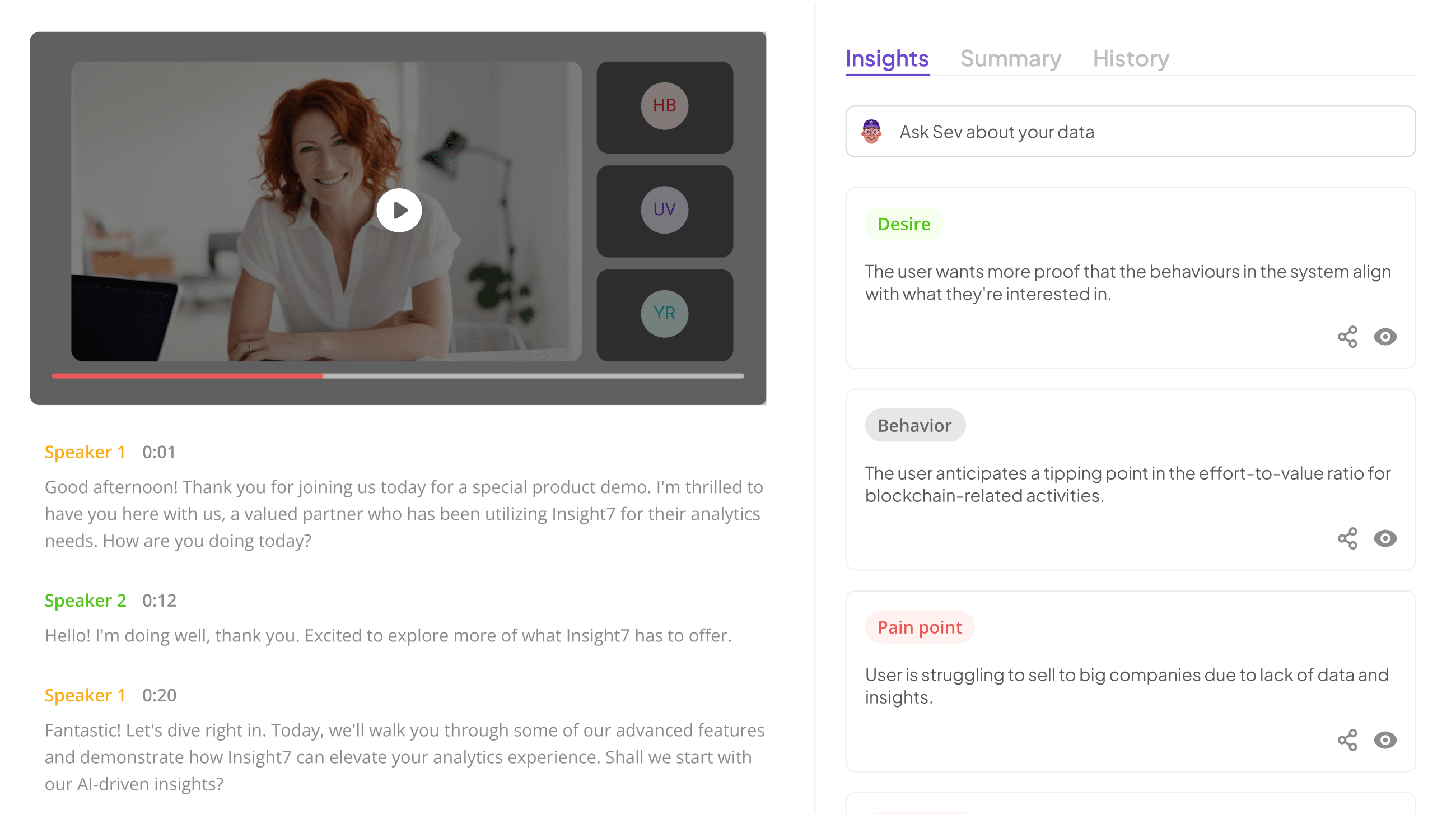
Understanding Data-Driven Decision Pathways
Data-driven decision pathways represent the structured approach organizations take to make informed choices based on quantitative evidence rather than intuition. Understanding these pathways enables businesses to identify critical data points, analyze trends, and draw actionable insights that drive effective strategies. When effectively mapped out, decision pathways transition raw data into meaningful narratives, enhancing clarity in the decision-making process.
AI Decision Optimization plays a pivotal role in refining these pathways. By harnessing advanced algorithms, AI tools can swiftly analyze vast datasets, uncover patterns, and even predict future outcomes. This optimization not only streamlines workflows but also enhances the accuracy of business decisions. Ultimately, a deep understanding of data-driven decision pathways empowers organizations to stay agile, respond to market dynamics, and fulfill customer needs more effectively.
The Role of AI in Modern Decision Making
Artificial intelligence plays a critical role in modern decision making by optimizing data-driven pathways. With the ability to analyze vast amounts of information quickly, AI facilitates timely and informed decisions in various sectors. By employing advanced algorithms, businesses can identify patterns and trends in data that would otherwise go unnoticed. This optimization not only enhances efficiency but also significantly reduces the risks associated with decision making.
One key benefit of AI decision optimization is its capability to provide actionable insights based on real-time data analysis. For instance, it can forecast potential outcomes by simulating various scenarios, allowing organizations to choose paths that align with their strategic goals. Furthermore, AI can gauge customer sentiment and market dynamics effectively, enabling companies to remain agile in a competitive landscape. As organizations increasingly integrate AI into their decision-making processes, they achieve greater accuracy, efficiency, and adaptability.
AI Decision Optimization Tools and Techniques
AI decision optimization tools and techniques are essential for enhancing the efficacy of data-driven decision pathways. By employing sophisticated algorithms and machine learning models, these tools help organizations analyze vast amounts of data, identifying patterns and insights that drive better strategic decisions. This technology not only streamlines decision-making processes but also empowers teams to respond swiftly to changing market conditions.
Among the widely used tools, key categories include predictive analytics platforms, decision support systems, and machine learning frameworks. Predictive analytics provides forecasts based on historical data, enabling proactive strategies. Decision support systems focus on guiding decision-makers by integrating data and analytics into actionable insights. Machine learning frameworks further enhance capabilities by learning from new data, resulting in continuous improvements in the decision-making processes. As AI decision optimization evolves, these tools become increasingly crucial in navigating complex decision landscapes.
Extract insights from interviews, calls, surveys and reviews for insights in minutes
Insight7: Leading the Charge in Decision Optimization
AI Decision Optimization stands at the forefront of transforming how organizations analyze and act on customer insights. As businesses generate unprecedented amounts of data, the ability to harness this information is crucial. Companies are now recognizing the importance of optimizing their decision-making pathways through AI technologies. This shift empowers them to respond swiftly to market changes and customer needs, enhancing competitive advantage.
At the core of this transformation is the integration of advanced AI agents that streamline the decision-making process. These agents sift through vast amounts of customer data, enabling organizations to discern patterns and derive actionable insights efficiently. Enhanced analytics capabilities ensure timely and precise decisions, moving businesses beyond traditional methods that often lag behind. In this evolving landscape, those who embrace AI-driven decision optimization will not only keep pace but lead their industries.
Other Essential AI Tools for Data-Driven Decisions
AI decision optimization is not just about individual tools; it encompasses a suite of resources that enhance data-driven decision-making. Various essential AI tools play a significant role in refining analysis, improving accuracy, and delivering actionable insights. By incorporating tools tailored to specific needs, organizations can ensure their decision pathways are enhanced and data-driven.
Among these tools, sentiment analysis software provides businesses with the ability to gauge customer opinions and feedback effectively. It analyzes social media, reviews, and surveys, allowing companies to understand public sentiment and adjust strategies accordingly. Additionally, data visualization tools create intuitive and interpretable representations of complex datasets, facilitating easier decision processes. Finally, predictive analytics platforms leverage historical data to forecast future trends, empowering businesses to make proactive and informed decisions. By utilizing these essential AI tools, organizations can significantly enhance their strategic capabilities and make smarter decisions.
- Tool A: Brief overview and functionality
Tool A is designed to enhance AI Decision Optimization through user-friendly functionality that democratizes data access. Its primary focus is on simplifying the decision-making process for users with varying levels of technical expertise. Users can easily engage with the platform without requiring extensive training, making it accessible for anyone in an organization. With intuitive features, it allows users to quickly generate actionable insights.
In its core functionality, Tool A provides a library for managing files, facilitating the analysis of calls, and extracting meaningful insights. Users can visualize conversations, identify customer pain points, and generate reports swiftly. This efficient approach to data management fosters informed decision-making across teams, ensuring alignment with company goals while addressing customer needs effectively. By streamlining workflows, Tool A becomes an essential asset in guiding organizations toward AI-powered decisions, ultimately optimizing data-driven pathways.
- Tool B: Brief overview and functionality
Tool B provides a versatile platform designed to streamline data analysis for informed decision-making. It enables users to access insights without requiring specialized training, fostering a democratic approach to data utilization. The intuitive interface allows anyone in the organization to start generating reports and analyzing customer experiences effortlessly. Users can focus on specific workflows, like transcribing calls or identifying friction points in customer journeys.
๐ฌ Questions about How AI Agents Optimize Data-Driven Decision Pathways?
Our team typically responds within minutes
The functionality of Tool B extends beyond simple reporting. By grouping multiple files into projects, users can analyze large datasets efficiently. It automatically extracts key insights, highlighting customer pain points, desires, and behaviors while providing evidence from conversations. This capability empowers teams to ask questions relevant to their projects, facilitating deeper understanding and actionable intelligence. In essence, Tool B enhances AI Decision Optimization by making data-driven insights accessible and actionable for all team members, ultimately improving decision pathways.
- Tool C: Brief overview and functionality
Tool C provides an intuitive platform designed to optimize decision-making processes through AI. It empowers users to analyze multiple datasets without requiring technical expertise. Accessible to anyone within an organization, it democratizes data insights, enabling diverse teams to contribute to strategic initiatives effectively.
The functionality of Tool C includes a central library where users can upload call recordings and files. As a result, users can easily retrieve and transcribe conversations, pinpointing key points such as customer pain areas and desires. This system not only identifies insights but also encapsulates them into easily digestible cards, supported by evidence from the conversations. Users can group projects, analyze trends across numerous calls, and derive actionable insights rapidly. By harnessing these capabilities, organizations can significantly enhance their data-driven decision pathways.
Steps for Implementing AI Decision Optimization
Implementing AI decision optimization involves several essential steps that can enhance data-driven decision pathways significantly. The first step is data collection and preparation, which sets the foundation for effective AI deployment. It involves gathering relevant data from various sources and ensuring it is organized and pre-processed for analysis. High-quality, clean data is crucial, as AI systems thrive on accurate information.
Next, choosing the right AI tools is vital. Not all tools are created equal, and selecting ones that align with your specific needs can make a substantial difference. After that, it is essential to integrate these tools with existing systems, ensuring they work seamlessly together. Finally, continuous monitoring and improvement of the AI systems will help in fine-tuning their performance. This iterative process allows organizations to adapt and enhance their decision-making capabilities over time, ensuring AI decision optimization remains effective and relevant.
Step 1: Data Collection and Preparation
Effective data collection and preparation is the cornerstone of AI decision optimization. First, organizations must identify relevant data sources that align with their objectives. This can involve internal databases, market research, and customer feedback analysis. Ensuring the data is accurate and comprehensive is crucial, as even small inaccuracies can skew results.
Next, data cleaning and preprocessing come into play. Removing duplicates, handling missing values, and formatting data properly prepare it for analysis. Once data is refined, it can be organized into a structured format that AI algorithms can easily interpret. This meticulous groundwork enhances the performance of AI models and lays a solid foundation for insightful decision-making. By prioritizing these steps, businesses can unlock the full potential of AI in their decision-making processes.
Step 2: Selecting the Right AI Tools
Finding the right AI tools is essential for AI decision optimization in data-driven pathways. Begin by evaluating your organizationโs specific needs, goals, and existing systems. Each AI tool has unique features and capabilities, making it crucial to match tools with your decision-making frameworks and objectives. Focus on those tools that not only analyze data but also facilitate intuitive insights and actionable outcomes.
Secondly, consider factors such as ease of integration, user support, and scalability. Compliance with data security standards is also paramount, especially when dealing with sensitive information. A well-selected AI tool should enhance operational efficiency and improve the quality of your decision-making processes. By carefully evaluating options and choosing tools tailored to your needs, your organization can leverage AI to optimize decision pathways effectively.
Step 3: Integrating AI with Existing Systems
Integrating AI with existing systems is crucial for achieving effective AI decision optimization. Organizations often have various legacy systems that require seamless integration to leverage the potential of AI technology. By carefully mapping out current workflows, companies can identify friction points where AI can enhance efficiency and accuracy. This process enables organizations to maintain continuity while improving decision-making capabilities.
The integration process can be broken down into three key areas: data interoperability, system compatibility, and user training. First, data interoperability ensures diverse data sources can communicate effectively with AI tools, facilitating cohesive analytics. Second, system compatibility emphasizes the need for AI solutions to work harmoniously with existing infrastructures, helping avoid disruptions. Lastly, emphasizing user training ensures employees are equipped to utilize AI-enhanced systems, maximizing adoption and operational benefits. Together, these factors pave the way for successful AI decision optimization, propelling organizations toward informed, data-driven decisions.
Step 4: Continuous Monitoring and Improvement
Continuous monitoring and improvement are essential components of successful AI decision optimization. AI systems thrive on data, making it crucial to regularly assess and analyze performance metrics. Regularly reviewing these insights allows organizations to identify trends and areas for improvement, enabling data-driven decisions that adapt to evolving market conditions.
A structured approach to continuous monitoring includes several key practices: First, establish performance benchmarks to measure against your AI's outcomes. Second, collect real-time feedback that informs necessary adjustments. Third, leverage advanced analytics to uncover insights that can guide subsequent decision-making processes. Finally, implement iterative testing to optimize strategies continually. By adopting this proactive stance, organizations enhance their ability to refine AI models, ensuring they remain effective and aligned with business objectives. Emphasizing ongoing evaluation and adaptation is vital for fostering a culture of continuous improvement in AI decision optimization.
Conclusion: The Future of AI Decision Optimization in Data-Driven Decision Pathways
As we look toward the future, AI decision optimization will continue to reshape data-driven decision pathways significantly. With advancements in machine learning and algorithm refinement, businesses will gain tools to enhance their decision-making processes. These technologies will allow organizations to analyze data with unprecedented accuracy, making it easier to uncover insights that drive better outcomes.
Moreover, collaboration between humans and AI will become paramount. As decision-makers embrace AI tools, they will leverage data insights while maintaining a clear focus on strategic goals. This synergy between technology and human intelligence will pave the way for more effective and responsive decision-making in an ever-evolving data landscape.
๐ฌ Questions about How AI Agents Optimize Data-Driven Decision Pathways?
Our team typically responds within minutes