AI Agent for Predictive Data Interpretation
-
Bella Williams
- 10 min read
Predictive Intelligence Tool is revolutionizing how organizations interpret and utilize data. In a world awash with information, extracting actionable insights has become essential for informed decision-making. This innovative tool enables users to analyze complex datasets, uncovering patterns and trends that would otherwise remain hidden. By automating data interpretation, it saves valuable time, allowing teams to focus on strategic initiatives.
Moreover, the Predictive Intelligence Tool facilitates deeper engagement with customers. By identifying key themes and pain points, organizations can adapt their strategies effectively. This capability not only enhances customer satisfaction but also drives business growth. As the landscape of data continues to evolve, embracing such tools will ensure that businesses remain competitive and responsive in their respective markets.
Analyze & Evaluate Calls. At Scale.
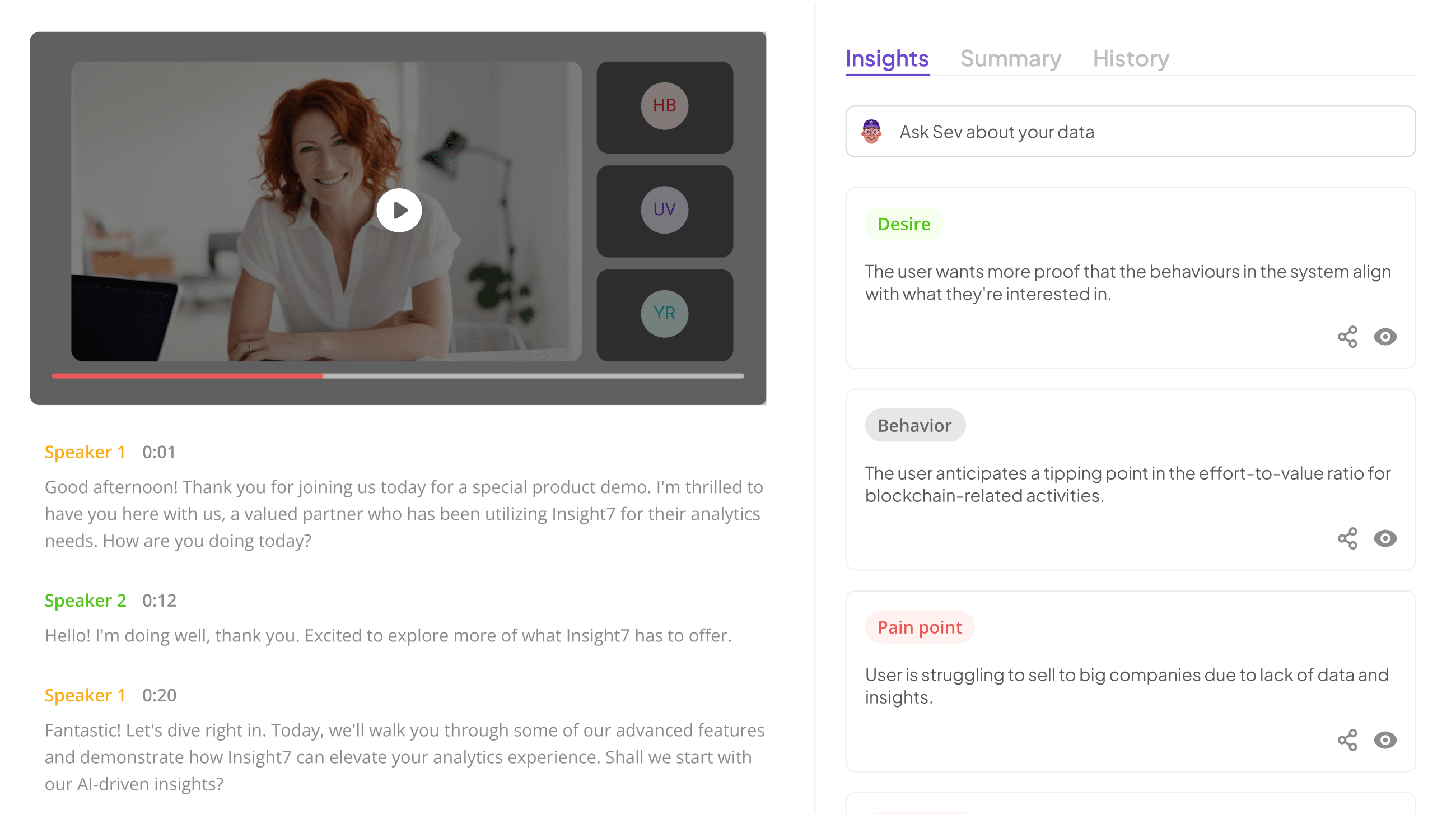
Understanding the Predictive Intelligence Tool Landscape
The realm of predictive intelligence tools is rapidly evolving, revolutionizing how organizations analyze and interpret data. These tools are designed to transform raw information into actionable insights, allowing businesses to make informed decisions based on trends and patterns. A key aspect of these tools is their accessibility; they aim to empower all team members, regardless of technical ability, to draw insights independently.
Understanding predictive intelligence tools involves recognizing various attributes that distinguish effective options in this space. Firstly, user-friendliness is paramount, enabling seamless engagement without extensive training. Secondly, efficient data organization and visualization help teams scrutinize insights thoroughly. Lastly, robust analytical capabilities are essential, allowing users to extract meaningful information that drives strategic initiatives. As companies increasingly adopt these tools, integrating them into workflows can lead to enhanced productivity and data-driven decision-making across departments.
AI Agent Fundamentals in Predictive Data Interpretation
In predictive data interpretation, understanding the fundamentals of an AI agent is crucial. An AI agent functions as a predictive intelligence tool, utilizing algorithms to analyze data patterns, trends, and correlations. Its ability to transform raw data into actionable insights helps organizations make informed decisions. By employing machine learning models, the AI agent can anticipate future outcomes based on historical data.
The role of the AI agent also involves continuous learning and adaptation. As it processes more data, the agent refines its predictions, enhancing accuracy over time. It is essential for users to provide specificity during interactions, as defined criteria shape the predictive quality of the insights. In this context, embracing a consultative approach can significantly improve the effectiveness of AI tools in meeting strategic objectives. Understanding these fundamentals lays the groundwork for successful predictive data interpretation.
Developing the Predictive Intelligence Tool Architecture
The development of the Predictive Intelligence Tool architecture is critical for effective data interpretation. This architecture serves as the backbone of the system, enabling seamless integration of various data sources while ensuring user-friendliness. Architects must prioritize accessibility, empowering users from all backgrounds to extract valuable insights without extensive technical knowledge. A successful architecture should facilitate intuitive workflows and provide quick access to features, transforming complex data analysis into manageable tasks.
Key elements must be considered during the architecture development process. First, the tool should incorporate robust data libraries, allowing users to visualize and analyze datasets efficiently. Next, it is crucial to implement advanced algorithms for deriving insights, such as recognizing customer pain points and behaviors. Lastly, the architecture must support project collaboration, enabling users to analyze multiple files simultaneously. By focusing on these aspects, the Predictive Intelligence Tool can significantly enhance data interpretation capabilities, guiding strategic decisions.
Extract insights from interviews, calls, surveys and reviews for insights in minutes
Key Components of an Effective Predictive Intelligence Tool
An effective Predictive Intelligence Tool must be grounded in several key components that drive its functionality. First, data collection and preprocessing play a crucial role in ensuring the accuracy and relevance of the analysis. This phase involves gathering diverse datasets from various sources and cleaning them to eliminate noise, which sets the foundation for insightful predictions.
๐ฌ Questions about AI Agent for Predictive Data Interpretation?
Our team typically responds within minutes
Additionally, predictive modeling techniques are essential for interpreting complex data patterns. By applying algorithms that learn from historical data, the tool can make informed forecasts about future trends. This dual focus on quality data and robust modeling enables organizations to extract actionable insights, helping users navigate challenges and seize opportunities.
In summary, a successful Predictive Intelligence Tool combines meticulous data management with advanced analytical capabilities, ensuring that users can effectively harness the power of data-driven decision-making.
Data Collection and Preprocessing
Data collection and preprocessing serve as foundational steps in building a predictive intelligence tool. These processes involve gathering rich and diverse datasets that inform the AI agent about various patterns and trends. By effectively harnessing data from multiple sources, the AI agent can provide more accurate and actionable insights.
The preprocessing phase is equally crucial. It involves cleaning and organizing data to enhance its quality, ensuring it is free of errors and inconsistencies. This may include standardizing formats, handling missing values, and filtering out irrelevant information. A well-prepared dataset empowers the predictive intelligence tool, enabling it to deliver meaningful interpretations and predictions. As such, a thoughtful approach to both data collection and preprocessing significantly enhances the AI agent's effectiveness.
Predictive Modeling Techniques
Predictive modeling techniques are essential to harnessing data effectively, enabling users to forecast outcomes based on historical trends. By employing sophisticated algorithms, these techniques analyze complex datasets, allowing organizations to make informed decisions with increased accuracy. Common methods include regression analysis, decision trees, and neural networks, each serving a unique purpose in predicting various scenarios.
Understanding which technique to apply depends on the specific needs of the data and the desired accuracy of predictions. For instance, regression analysis is particularly valuable for capturing relationships between variables. In contrast, decision trees offer visual interpretations that enhance decision-making processes. Neural networks excel in handling large volumes of data, identifying intricate patterns that simpler models may overlook. The integration of these predictive modeling techniques within a predictive intelligence tool significantly transforms how we interpret data and derive actionable insights, ultimately leading to better strategic outcomes.
Conclusion: The Future of AI Agent in Predictive Intelligence Tools
As we look ahead, the role of AI agents in predictive intelligence tools is set to expand significantly. These agents will not only enhance data interpretation but also streamline decision-making processes. Their ability to analyze vast datasets with remarkable accuracy provides organizations with insights that were once unimaginable, leading to smarter strategies and improved outcomes.
Furthermore, advancements in technology will allow these agents to become even more intuitive and user-friendly. As intuitive interfaces evolve, users will be empowered to harness the full potential of predictive intelligence tools without requiring extensive technical knowledge. The future promises a landscape where AI agents will play a vital role in driving innovative functions, making predictive intelligence an indispensable asset across various industries.
๐ฌ Questions about AI Agent for Predictive Data Interpretation?
Our team typically responds within minutes