Predictive Behavior Insights can transform the way businesses understand their customers. Imagine being able to anticipate client needs before they even arise, capturing valuable insights that can drive decision-making and strategizing. By harnessing the power of AI agents, organizations can effectively analyze patterns and trends, leading to more informed actions.
This section serves as an introduction to the significance of predictive behavior insights. With advancements in technology, the ability to gain a deeper understanding of consumer behavior has never been more accessible. This evolution signifies not just a competitive edge, but an opportunity for businesses to foster meaningful interactions and drive growth. Understanding and implementing these insights is crucial for staying ahead in todayโs dynamic market.
Analyze qualitative data. At Scale.
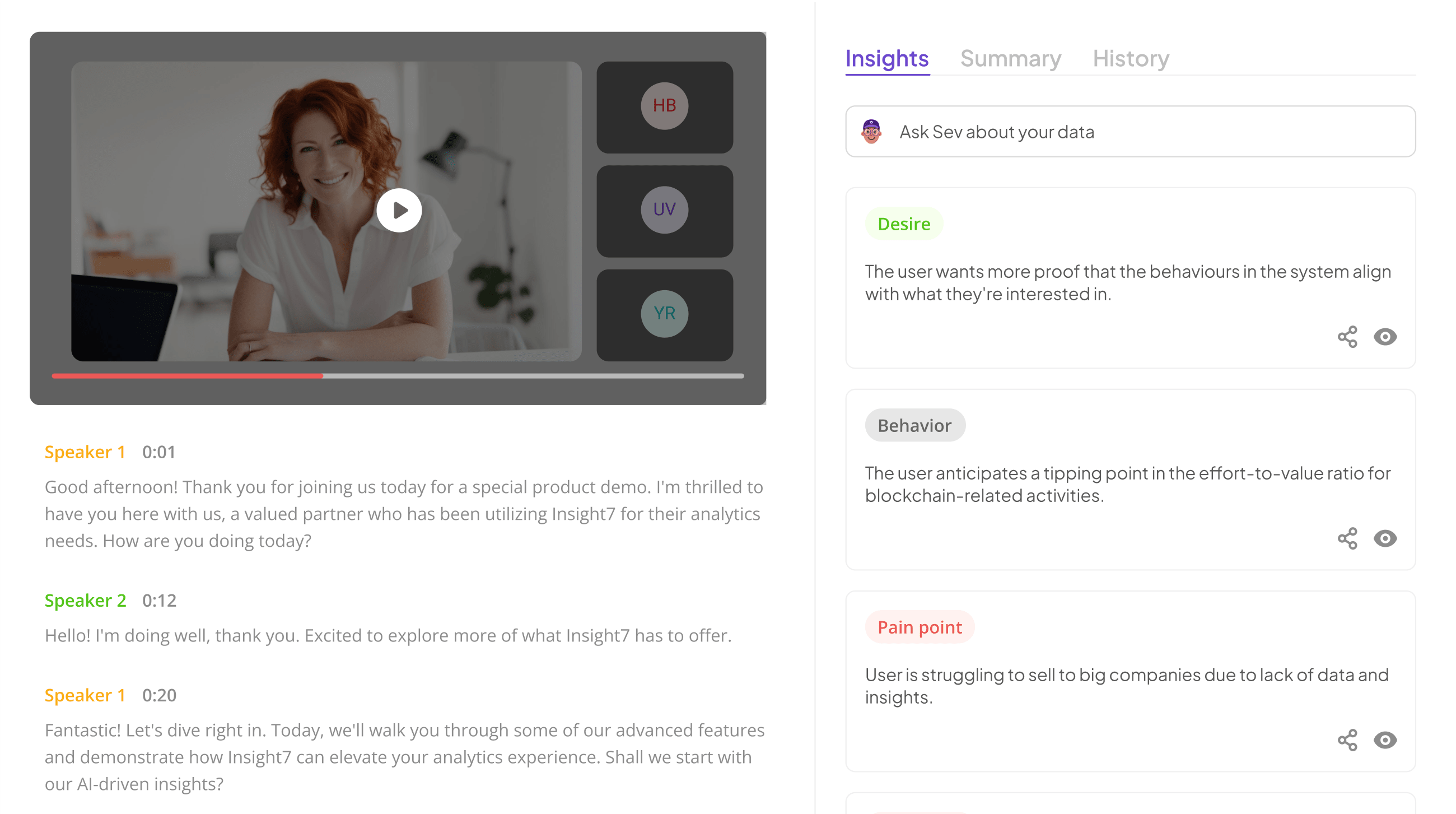
Leveraging AI Agents for Enhanced Predictive Behavior Insights
AI agents have transformed the field of predictive behavior insights, enabling organizations to better understand patterns and forecast future actions. By processing vast amounts of data, these intelligent systems can analyze user interactions, preferences, and even emotional responses. This ability helps businesses harness predictive behavior insights to tailor offerings, improve customer experiences, and ultimately drive growth.
To utilize AI agents effectively, organizations should focus on data quality and relevance. This involves collecting comprehensive data sets and ensuring accuracy throughout. Following this, model training becomes paramount; AI agents need to learn from past data to generate accurate predictions. Finally, deploying these agents to make real-time predictions can lead to dynamic insights that inform decision-making processes and strategies. By integrating AI into their frameworks, organizations can unlock valuable behavioral insights that offer a competitive edge.
Understanding the Role of AI in Behavior Prediction
AI plays a pivotal role in behavior prediction, enabling organizations to gain critical insights into customer actions and preferences. By utilizing advanced algorithms and machine learning techniques, AI agents analyze historical data to forecast future behaviors and trends. For instance, they can identify patterns in customer purchasing habits, allowing businesses to tailor their strategies accordingly. This predictive capability helps organizations remain proactive in their decision-making processes.
In the journey toward harnessing predictive behavior insights, it is essential to understand how AI works and the underlying data management processes. AI algorithms require substantial amounts of quality data to make accurate predictions. Therefore, organizations must establish effective data collection routines and ensure data integrity. Once the data is gathered, these AI systems can be trained and tested, culminating in deployment for real-time predictions. This collaborative effort between data and AI ultimately fosters a deeper understanding of consumer behavior and drives informed business decisions.
Steps to Implement AI for Predictive Behavior Insights
Implementing AI for predictive behavior insights involves a systematic approach to ensure meaningful outcomes. First, data collection and management is essential. Gather comprehensive datasets that reflect user behaviors, preferences, and interactions. Organize and clean this data to eliminate inaccuracies and redundancies. This foundational step is crucial, as quality data directly influences the effectiveness of predictions made later.
Next, proceed to model training and testing. Develop algorithms capable of analyzing the collected data and identifying patterns related to predictive behavior. It's imperative to test these models rigorously, refining them based on their performance. Utilize various techniques, such as machine learning and deep learning, to enhance your models. Once satisfactory results are achieved, the final step involves deploying AI agents for predictions. Integrate these agents into existing platforms, allowing real-time analysis of user behavior and insights. This targeted implementation can drive better decision-making and strategy development based on predictive behavior insights.
- Step 1: Data Collection and Management
The collection and management of data serve as the backbone for generating predictive behavior insights. First, it's vital to gather relevant data from various sources, including customer interactions, surveys, and marketplace trends. By creating a structured database, you enable efficient analysis that reveals underlying patterns and trends. Organizing this data effectively ensures that the AI agents can access and process it seamlessly for better insights.
Next, data management plays a crucial role in maintaining accuracy over time. Regularly updating and cleaning your dataset helps eliminate inconsistencies that could skew results. Using advanced analytical tools allows you to dig deeper into the data, facilitating the extraction of meaningful insights. This structured approach not only supports efficient analysis but also lays the groundwork for effective predictive modeling. Providing rich, high-quality data enhances the potential of AI agents to deliver metrics that drive informed decision-making.
- Step 2: Model Training and Testing
In Step 2, the focus shifts to model training and testing, a crucial phase in extracting predictive behavior insights. This process involves selecting appropriate algorithms and feeding them the prepared data. Itโs essential to ensure that the model learns from diverse datasets to capture various behavior patterns effectively. Familiarizing yourself with different training techniques, such as supervised or unsupervised learning, helps tailor the model to specific predictive tasks.
Once the model is trained, testing its performance on unseen data is vital. This step allows you to evaluate accuracy, precision, and recall, ensuring that the predictions made align with actual outcomes. Regularly refining the model based on testing feedback ensures sustained performance and reliability in generating insights. Iteration through training and testing cycles creates a robust predictive model, ultimately leading to valuable behavioral data insights.
- Step 3: Deploying AI Agents for Predictions
Deploying AI agents for predictions marks a crucial step in extracting valuable insights from behavioral data. This process involves integrating advanced algorithms into your systems to analyze patterns in user activity and make informed forecasts. Initially, it's essential to ensure that the AI agents are well-trained with high-quality data, as this significantly affects the accuracy of their predictions.
Once deployed, these AI agents can continuously learn from incoming data, adapting their models to reflect changing user behaviors. To effectively utilize predictive behavior insights, organizations must also define clear objectives for what they aim to achieve through these predictions. This clarity promotes targeted strategies, allowing businesses to enhance user engagement and optimize decision-making processes. By systematically deploying AI agents, companies can turn behavioral predictions into actionable insights, fostering strategic growth.
Extract insights from interviews, calls, surveys and reviews for insights in minutes
Tools for Generating Predictive Behavior Insights with AI
In the quest for predictive behavior insights, various AI tools play a crucial role. These platforms not only aggregate large volumes of data but also analyze it in meaningful ways. For instance, tools such as IBM Watson and Google AI Platform allow users to deploy sophisticated models tailored to specific business challenges. By utilizing these platforms, organizations can identify trends and patterns in behavior, ultimately leading to more informed decision-making.
Moreover, tools like H2O.ai and DataRobot streamline the process of model training and testing. These platforms enable users to experiment with different algorithms, ensuring that the predictive models are robust and accurate. Each tool comes equipped with user-friendly interfaces and extensive documentation, making it easier for businesses to implement them without needing advanced data science skills. As companies increasingly rely on predictive behavior insights, selecting the right tools fosters a data-driven culture that promotes continuous improvement and innovation.
Top Platforms for AI Predictive Analytics
When exploring the top platforms for AI predictive analytics, businesses can unlock powerful predictive behavior insights. These platforms provide the tools necessary to analyze large datasets, unveiling patterns and trends that inform decision-making. By employing advanced algorithms, they enhance accuracy in forecasting customer behavior, thus enabling businesses to effectively tailor their strategies.
Popular choices include platforms that prioritize ease of use and accessibility. This democratizes data insights, allowing teams without extensive technical backgrounds to generate meaningful analyses. Each platform offers unique features, from data processing capabilities to visualization tools, that cater to diverse business needs. Selecting the right platform can significantly streamline the process of gathering and interpreting predictive data, ultimately leading to better business outcomes.
- insight7
In this section, we will delve into the significance of predictive behavior insights and how they can be effectively harnessed through AI agents. Predictive behavior insights offer a glimpse into future customer actions based on historical data patterns and behaviors. By leveraging these insights, organizations can anticipate customer needs and adapt their strategies accordingly.
To maximize the effectiveness of predictive behavior insights, it is crucial to follow several key steps. First, ensure comprehensive data collection and management, as high-quality data serves as the backbone for AI analysis. Next, model training and testing are essential to refine algorithms and enhance prediction accuracy. Finally, deploying AI agents for real-time predictions allows organizations to respond swiftly to changing customer behaviors, ensuring they remain competitive in a dynamic market. By effectively navigating these steps, businesses can unlock the full potential of predictive behavior insights, ultimately driving informed decision-making and improved customer engagement.
- IBM Watson
IBM Watson offers a powerful suite designed for generating predictive behavior insights through advanced data analysis. Users can efficiently collect, organize, and analyze varying datasets, transforming raw data into actionable intelligence. With built-in tools for sentiment analysis, businesses can identify customer emotions and feedback trends, enabling tailored strategies that resonate with their target audience.
To maximize the effectiveness of this platform, consider the following steps:
Data Collection: Gather holistic datasets that reflect customer interactions and sentiments.
Sentiment Analysis: Utilize the analytical tools to extract insights regarding both positive and negative feedback.
Predictive Modeling: Train models to identify patterns and predict future customer behaviors based on historical data.
By following these steps, users can harness this technology to generate meaningful insights that enhance decision-making processes and customer engagement strategies. This proactive approach to understanding consumer behavior ultimately drives better business performance.
- Google AI Platform
The Google AI Platform offers advanced tools and services for organizations aiming to gain predictive behavior insights. Its infrastructure supports the entire AI lifecycle, allowing users to build, deploy, and manage machine learning models efficiently. Leveraging features like AutoML, teams can automate the model training process and improve accuracy without needing extensive data science expertise.
This platform excels in facilitating data collection, model training, and deployment, essential for extracting meaningful insights from complex datasets. By offering scalable analytics and integration capabilities, businesses can utilize it to track user behavior and trends in real time. The insights generated can inform strategic decisions, helping organizations predict future behaviors with confidence and adapt their marketing strategies accordingly.
In summary, the Google AI Platform empowers organizations to transform data into actionable predictive behavior insights, enhancing their ability to stay ahead in a competitive landscape.
- H2O.ai
Harnessing advanced tools is essential to derive effective predictive behavior insights. The focus is on optimizing AI technologies. By using state-of-the-art platforms, organizations can effectively analyze behavioral data and draw actionable conclusions. Through their intuitive interfaces and powerful algorithms, these platforms simplify the complex processes involved in data analysis.
Several platforms lead the charge in providing robust predictive analytics. Firstly, insight7 excels in delivering user-friendly analytics tailored for detailed insights. Secondly, IBM Watson stands out with its machine learning capabilities, enhancing prediction accuracy. Google AI Platform is noteworthy for its seamless integration of AI across applications. Platforms such as DataRobot and Microsoft Azure AI also provide diverse functionalities that cater to varying business needs. Adopting these tools enables businesses to transform raw data into coherent predictive insights, driving smarter decision-making.
- DataRobot
In the realm of AI-driven analytics, the platform under discussion is crucial for enhancing predictive behavior insights. Users benefit from advanced tools that streamline the analysis of vast datasets, enabling businesses to discern various behavioral patterns. This process includes identifying both positive and negative customer feedback, which informs product development and strategic decisions based on real-time insights.
To effectively harness the power of this platform, it's essential to understand its primary functionalities. First, the platform facilitates data visualization, allowing users to explore patterns across different segments. Second, it supports seamless querying of extensive datasets, making it easier to extract actionable insights. Lastly, businesses can compare and analyze data from various locations, providing a comprehensive perspective on user behaviors. By implementing these features, organizations can significantly enhance their predictive analytics capabilities and make informed decisions grounded in reliable data.
- Microsoft Azure AI
Microsoft Azure AI offers powerful capabilities to extract predictive behavior insights from diverse data streams. Utilizing advanced machine learning algorithms, it empowers organizations to identify patterns and trends that inform strategic decisions. Whether analyzing customer interactions, sales data, or market trends, Azure AI enhances the ability to predict future behaviors effectively.
To engage with Azure AI for these insights, users can follow several key steps. First, it's essential to gather and manage relevant data efficiently. Next, training and testing predictive models ensure accuracy in forecasting outcomes. Finally, deploying AI agents allows for real-time predictions that drive actionable results. With these functionalities, Azure AI stands out as a robust tool for businesses aiming to leverage predictive behavior insights in their strategies.
Conclusion: Harnessing AI for Reliable Predictive Behavior Insights
Harnessing AI for reliable predictive behavior insights is a transformative step for any organization seeking to understand its users better. By effectively integrating AI agents, businesses can analyze vast amounts of behavioral data, revealing patterns and trends that would otherwise remain unnoticed. This capability empowers companies to make data-driven decisions, enhancing customer experiences and improving operational efficiency.
As organizations embrace AI tools, it is crucial to focus on the accuracy and reliability of the insights generated. Continuous learning and adaptation of AI models ensure that predictive behavior insights remain relevant and actionable. Ultimately, organizations that effectively utilize AI will not only stay competitive but also foster deeper connections with their customers, paving the way for sustained growth and innovation.