How to Optimize Predictive Customer Behavior Analysis Using AI Agents
-
Bella Williams
- 10 min read
In today's dynamic marketplace, understanding how customers behave is more crucial than ever. Predictive Behavior Optimization emerges as a key strategy, harnessing the power of AI agents to transform data into actionable insights. By analyzing vast amounts of customer interactions, businesses can identify patterns and preferences that inform sales and marketing strategies. With this approach, organizations become equipped to anticipate customer needs, ultimately enhancing engagement and loyalty.
The implementation of Predictive Behavior Optimization allows companies to move beyond conventional analytics methods. As businesses gather unprecedented amounts of data, traditional techniques often fall short in delivering timely insights. By integrating AI technologies, firms can process this information efficiently, leading to faster decision-making and strategic planning. This transformation not only improves operational efficiency but also fosters a proactive approach to customer interaction, positioning companies ahead of their competition.
Analyze & Evaluate Calls. At Scale.
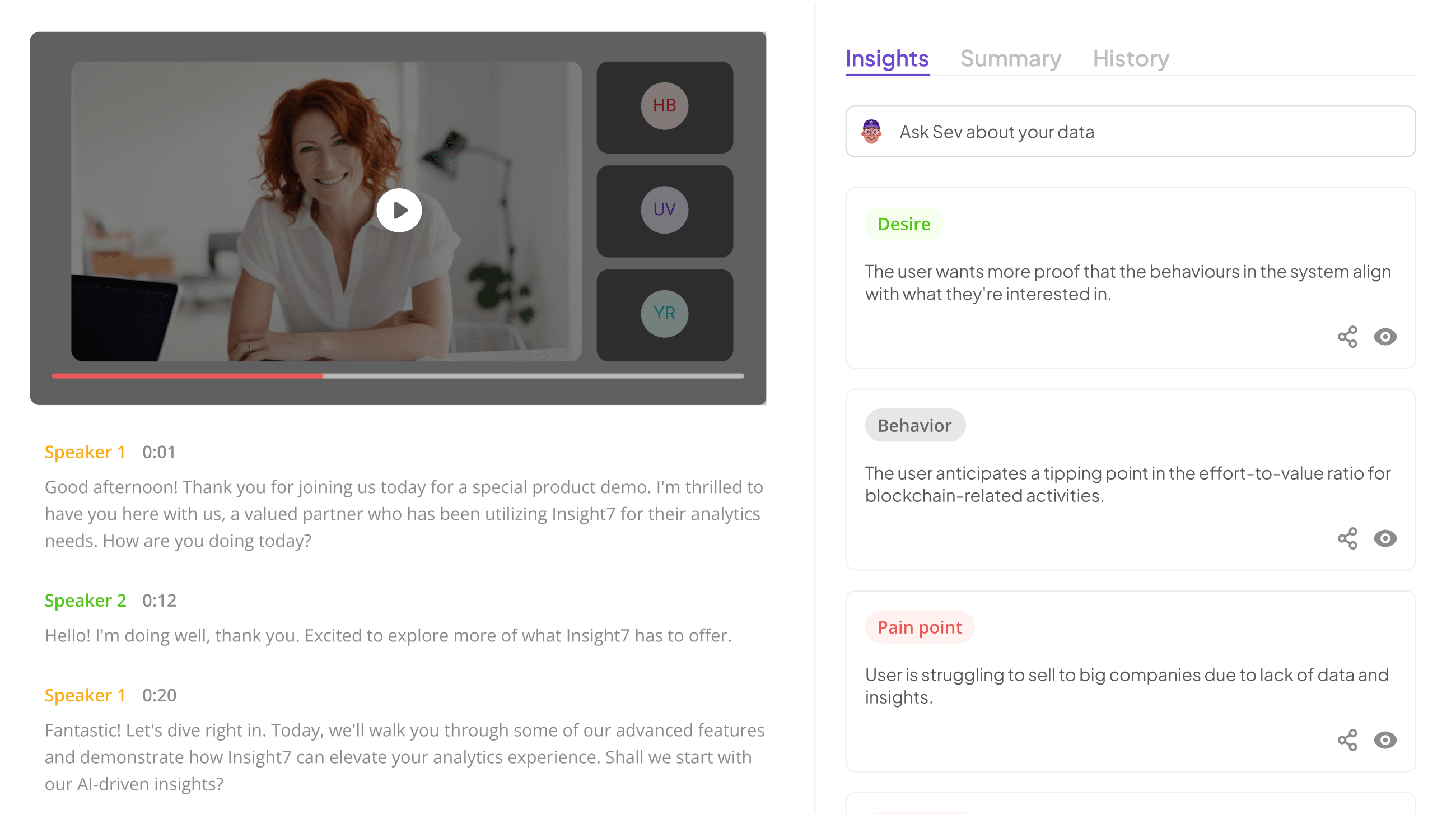
Understanding Predictive Behavior Optimization with AI Agents
Predictive Behavior Optimization involves leveraging AI agents to enhance our understanding of customer tendencies. By analyzing data patterns, businesses can anticipate customer needs and preferences with remarkable accuracy. This process hinges on the ability of AI agents to swiftly process vast amounts of information, delivering insights that are not only timely but also actionable.
The optimization begins by identifying key customer behavior patterns. AI agents sift through historical data to uncover underlying trends, providing a solid foundation for predictive modeling. Once these patterns are established, businesses can deploy robust AI models tailored to specific customer segments. Engaging these AI agents in real-time data processing allows organizations to adjust their strategies dynamically, improving customer satisfaction and driving conversions. Ultimately, understanding how to effectively implement predictive behavior optimization empowers businesses to tailor their offerings, fostering loyalty and sustained growth.
The Role of AI in Enhancing Predictive Behavior
AI plays a pivotal role in enhancing predictive behavior by analyzing vast amounts of customer data. This capability allows businesses to anticipate customer needs and preferences, leading to improved service delivery. As AI agents integrate with existing systems, they identify patterns in customer interactions that can optimize predictive behavior, making it more effective and accurate.
Furthermore, real-time data processing significantly impacts predictive analysis. With AI, organizations can gather and assess customer behavior in the moment, enabling timely responses to emerging trends. This immediacy helps maintain customer satisfaction and fosters loyalty, as clients feel their needs are promptly addressed. By focusing on these advancements, businesses can harness AI's potential to elevate their predictive behavior strategies, ensuring sustained growth and competitiveness in the market.
- Integrating AI Agents: Core Advantages
Integrating AI agents in customer behavior analysis offers transformative advantages that enhance predictive behavior optimization. First, AI agents provide advanced data processing capabilities, allowing for real-time insights. With the ability to analyze vast datasets rapidly, businesses can identify trends and patterns that might be missed by traditional methods. This immediacy facilitates timely decision-making, helping organizations respond swiftly to consumer needs and expectations.
Moreover, AI agents enhance personalization, which is crucial for improving customer relationships. By leveraging detailed customer profiles, AI systems can tailor interactions, resulting in higher engagement and satisfaction rates. This strategic application of AI fosters loyalty, ultimately driving revenue growth. Additionally, the scalability of AI solutions means they can adapt as business needs evolve. Companies can therefore maintain a competitive edge by continuously refining their predictive behavior optimization efforts.
By integrating these advanced technologies, organizations can fully harness the power of customer insights, leading to more effective marketing strategies and improved customer experiences.
- Real-time Data Processing: A Game Changer
Real-time data processing fundamentally transforms how businesses analyze and respond to customer behavior. By enabling immediate insights into customer interactions, organizations can swiftly adapt to emerging trends and preferences. This immediacy allows brands to optimize predictive behavior strategies and make data-driven decisions that resonate with their audience.
To harness the power of real-time data, consider the following key factors. First, ensure your data sources are diverse and comprehensive, capturing a wide range of customer interactions. Next, implement advanced analytics tools that can efficiently process this data, providing actionable insights almost instantaneously. Additionally, fostering a culture of agility within your organization encourages teams to act on insights promptly, enhancing customer engagement and satisfaction. Ultimately, embracing real-time data processing leads to informed strategies and improved connections with customers, setting the stage for ongoing success.
Implementing Predictive Behavior Optimization
Effective implementation of predictive behavior optimization begins with identifying key customer behavior patterns. By examining historical data, businesses can pinpoint trends and preferences that influence customer decisions. This foundational step allows for the development of AI models tailored specifically to the target audience, improving engagement and conversion rates.
Moreover, building robust AI models is crucial for predictive behavior optimization. These models should be trained on diverse datasets to ensure they can recognize and adapt to varying customer behaviors. Testing and refining these models regularly helps to maintain their accuracy and relevance over time. A symmetrical operation of data collection, model training, and deployment ensures that organizations can anticipate customer needs more effectively. By continuously optimizing these processes, businesses can establish a dynamic approach to understanding and predicting customer behavior.
Key Steps to Successful AI Deployment
Successful AI deployment starts by identifying customer behavior patterns. This foundational step involves analyzing existing data to uncover trends that inform AI models. By understanding these behaviors, organizations can ensure that their AI systems are responsive and aligned with customer needs. After recognizing these patterns, the next critical step involves building robust AI models that accurately reflect this data. These models must be continuously refined and tested to improve predictive accuracy.
To further enhance predictive behavior optimization, organizations should focus on ongoing monitoring and adjustments. This allows organizations to address any unforeseen challenges as they arise, ensuring that the AI remains effective over time. Additionally, integrating feedback from users can help fine-tune the models, making them more attuned to actual customer behaviors. These steps collectively ensure that AI deployment not only launches successfully but also evolves to meet the dynamic landscape of customer interactions.
- Identifying Customer Behavior Patterns
Understanding customer behavior is crucial in optimizing predictive behavior analysis. Identifying customer behavior patterns enables businesses to tailor their strategies effectively. Companies can analyze customer interactions, feedback, and preferences to uncover trends that might not be immediately apparent. This comprehensive understanding allows businesses to anticipate needs and respond proactively.
๐ฌ Questions about How to Optimize Predictive Customer Behavior Analysis Using AI Agents?
Our team typically responds within minutes
To achieve effective identification, organizations should focus on three critical aspects. First, they must gather and analyze diverse data sourcesโcustomer surveys, social media interactions, and purchase histories. Next, employing AI agents to process this data will enhance efficiency and uncover deeper insights. Lastly, establishing a feedback loop ensures that customer behavior remains continuously monitored and understood, allowing for ongoing refinement of strategies. By mastering these steps, businesses can significantly improve their predictive behavior optimization efforts, ultimately leading to enhanced customer satisfaction and increased loyalty.
- Building Robust AI Models
Building robust AI models is crucial for achieving effective predictive behavior optimization. To begin, the model must be designed with clarity regarding specific objectives and customer behavior patterns. Establishing clear performance benchmarks allows for meaningful assessments of the model's output, ensuring that it meets the intended standards and expectations.
Furthermore, a robust AI model utilizes diverse datasets, which enhances its capability to recognize patterns and adapt to new information. Continuous training on current data is essential, as it helps in maintaining accuracy and relevance over time. Models also benefit from incorporating feedback mechanisms, which allow for iterative improvements based on real-world results. By focusing on these key aspects, organizations can develop AI agents that effectively optimize predictive behavior, ultimately leading to more informed decision-making processes and improved customer experiences.
Extract insights from interviews, calls, surveys and reviews for insights in minutes
Top Tools for Predictive Behavior Optimization
In the realm of predictive behavior optimization, selecting the right tools is paramount for effective analysis. A powerful tool can harness data from customer interactions, allowing organizations to extract actionable insights. For instance, platforms like insight7 facilitate easy analysis of large data sets, helping businesses understand customer sentiment efficiently. This tool promotes a self-service model, enabling teams to analyze interviews and conversations at scale.
Additionally, solutions such as IBM Watson Customer Experience Analytics stand out by offering sophisticated data processing capabilities. This tool enables companies to track and analyze customer behaviors in real-time, enhancing the speed of insights. Similarly, Salesforce Einstein Analytics provides businesses with intelligent analytics, allowing for deep dives into behavioral patterns. Lastly, SAS Customer Intelligence 360 empowers teams with robust customer insights, ensuring organizations can pivot their strategies based on emerging trends. By leveraging these tools, companies can transform the way they approach predictive behavior optimization.
- insight7
To effectively implement Predictive Behavior Optimization, businesses must first develop a deep understanding of their customer base. This involves analyzing behavioral data to identify patterns and trends. AI agents play a vital role in this process by sifting through large volumes of data to unearth insights that support better decision-making. The insights generated can guide marketing strategies, product development, and customer engagement efforts.
Integrating AI in behavior analysis not only enhances accuracy but also allows for real-time responsiveness to customer needs. Organizations should consider training AI models with historical data to predict future behaviors. This proactive approach ensures that companies stay ahead of the competition, allowing for timely interventions that can positively influence the customer journey. Embracing these strategies positions businesses to thrive in a data-driven marketplace, ensuring lasting customer relationships and loyalty.
- IBM Watson Customer Experience Analytics
IBM Watson Customer Experience Analytics empowers businesses to enhance their understanding of customer behavior through advanced data analytics. By integrating AI technologies, businesses can gain insights that allow for better interactions and more personalized experiences. This platform excels in processing vast amounts of information, enabling organizations to track customer journeys and identify trends in real time.
To optimize predictive behavior analysis, businesses should focus on analyzing different data points to create a comprehensive view of customer interactions. This involves examining engagement metrics, preferences, and feedback to tailor services effectively. By utilizing AI-driven analytics, companies can not only anticipate customer needs but also refine their strategies to foster loyalty and satisfaction. Ultimately, harnessing the capabilities of AI and analytics can significantly improve predictive behavior optimization, leading to enhanced customer relationships and business success.
- Salesforce Einstein Analytics
Salesforce Einstein Analytics plays a pivotal role in refining customer behavior prediction through intuitive and comprehensive data analysis. This powerful tool empowers businesses to extract insights from vast datasets, providing clarity and precision in understanding customer actions. By employing advanced AI agents, organizations can foster an environment conducive to Predictive Behavior Optimization, effectively tailoring their strategies to meet customer needs.
Integrating Salesforce Einstein Analytics into your workflow involves several steps. First, businesses should focus on aligning their data sources to ensure seamless interaction with the analytics platform. Second, leveraging its visualization capabilities allows for effective interpretation of customer trends, making it easier to identify patterns. Finally, utilizing predictive models within the platform enables organizations to anticipate future customer behaviors and tailor their offerings accordingly. This holistic approach to data-driven decision-making can significantly enhance customer engagement and satisfaction over time.
- SAS Customer Intelligence 360
SAS Customer Intelligence 360 offers powerful tools for optimizing Predictive Behavior Optimization. By harnessing advanced analytics, it enables businesses to understand customer motivations, preferences, and patterns. This platform facilitates the intricate analysis of customer data, allowing organizations to predict future behaviors accurately. Moreover, it empowers companies to transition from a reactive to a proactive stance in engagement, refining strategies to meet evolving consumer demands.
The platform excels in providing actionable insights through its user-friendly interface. Users can access customizable dashboards that visualize data trends and facilitate decision-making. Additionally, the integration of AI capabilities enhances real-time data processing, making it possible to respond swiftly to market changes and customer needs. As a result, organizations can foster deeper customer relationships, increase loyalty, and ultimately drive growth through informed decision-making rooted in robust predictive analysis.
Conclusion: Transforming Customer Behavior Analysis through Predictive Behavior Optimization
The journey toward transforming customer behavior analysis through predictive behavior optimization is pivotal for any organization seeking a competitive edge. By integrating AI agents, businesses can harness vast amounts of data, analyzing customer signals efficiently. This shift not only accelerates insights but also fosters a culture of proactive engagement, where understanding customer needs becomes central to strategy.
As organizations adopt these advanced methodologies, they realize the importance of effective data utilization. Predictive behavior optimization becomes a catalyst for change, streamlining decision-making processes and enhancing customer interactions. Ultimately, this transformation enables businesses to not only meet but anticipate customer needs, ensuring sustained growth and relevance in a dynamic market landscape.
๐ฌ Questions about How to Optimize Predictive Customer Behavior Analysis Using AI Agents?
Our team typically responds within minutes