AI Agents for Predictive Strategic Decision Making in Banking
-
Bella Williams
- 10 min read
Predictive Banking Intelligence is reshaping the landscape of strategic decision-making in the financial sector. As banks navigate an ever-evolving market, the ability to predict trends and customer behavior becomes essential. AI agents equipped with analytics capabilities can process vast amounts of data, providing insights that help organizations make informed choices and enhance operational efficiency.
Harnessing Predictive Banking Intelligence allows financial institutions to tailor their services more effectively. By integrating advanced algorithms, banks can anticipate customer needs, mitigate risks, and optimize their resources. Embracing this technology not only strengthens decision-making processes but also fosters trust and loyalty among clients, positioning banks as leaders in the competitive financial environment.
Extract insights from Customer & Employee Interviews. At Scale.
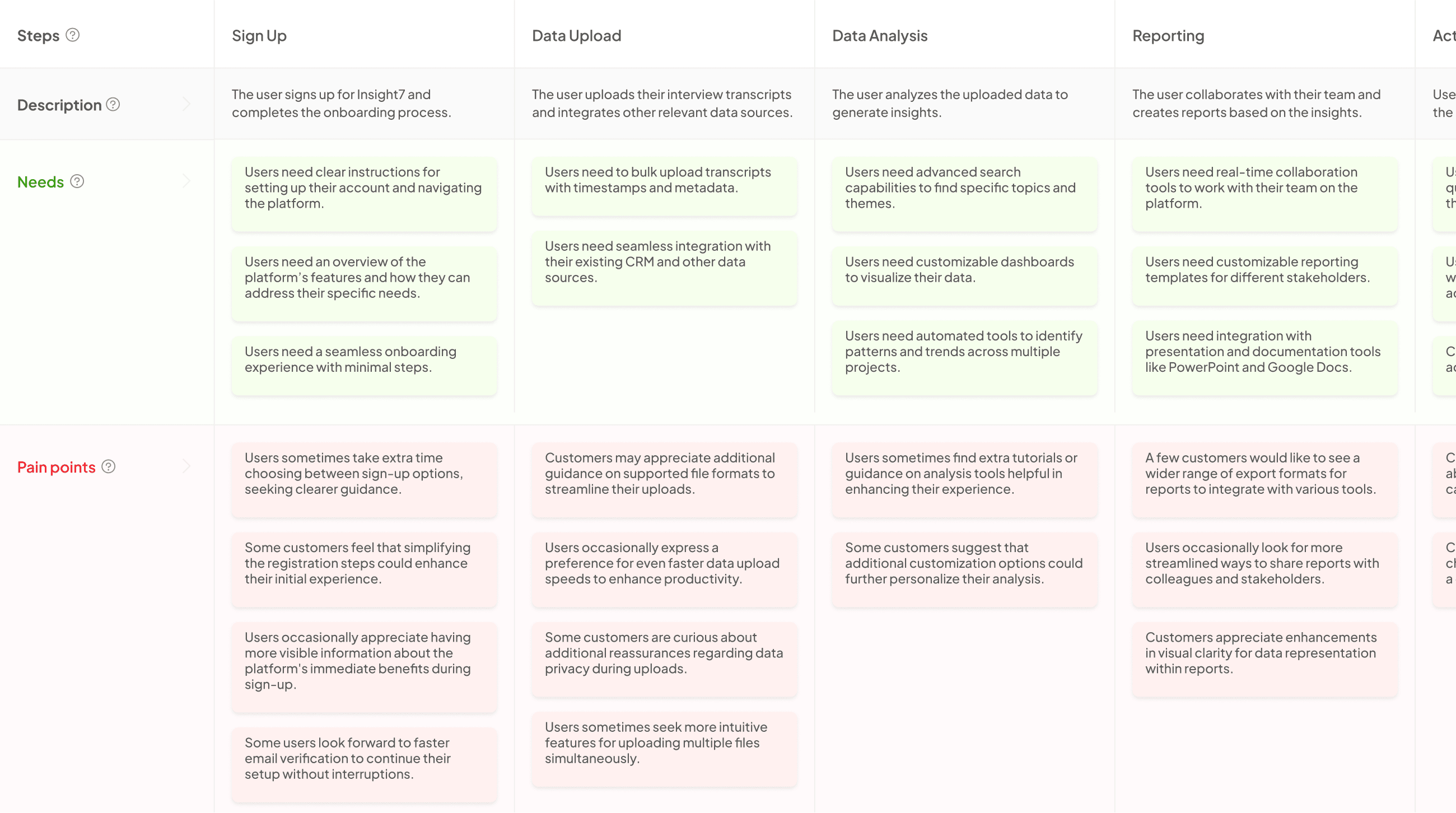
The Role of AI Agents in Predictive Banking Intelligence
AI agents play a pivotal role in enhancing predictive banking intelligence, driving more informed strategic decisions. These intelligent systems can analyze vast amounts of data to identify patterns and trends, enabling banks to anticipate customer needs and market shifts. By dynamically processing this data, AI agents deliver actionable insights that guide decision-makers in formulating effective strategies.
Moreover, AI agents improve operational efficiency by optimizing processes, reducing human error, and enabling quicker response times. They can provide real-time analytics, which supports immediate decision-making, enhancing overall agility in a rapidly changing environment. This capability fosters a proactive approach to risk management and customer satisfaction, essential for maintaining competitive advantage in the banking sector. As institutions increasingly integrate AI agents, they unlock the potential for transformative predictive banking intelligence, ultimately reshaping their strategic landscapes.
Understanding AI Agents for Predictive Banking Intelligence
AI agents play a pivotal role in cultivating predictive banking intelligence, serving as sophisticated tools that enhance decision-making processes. These agents utilize vast amounts of data to discern patterns and trends, offering insights that can inform banking strategies. By automating data analysis, they free up human agents to focus on complex tasks that require human intuition and judgment.
Understanding how these AI agents function is essential for banks looking to maintain a competitive edge. They constantly monitor and evaluate market conditions, customer behaviors, and other key variables. This constant vigilance allows financial institutions to make informed decisions swiftly, helping them to anticipate market shifts and customer needs effectively. The integration of AI agents transforms traditional banking practices, ultimately leading to smarter strategies derived from predictive banking intelligence.
Benefits of AI in Strategic Decision Making
The integration of AI in strategic decision-making brings transformative advantages to the banking sector. First, AI enhances the accuracy of data analysis, allowing for insightful predictions and enabling decision-makers to act with greater confidence. This capability, known as Predictive Banking Intelligence, utilizes vast amounts of data to forecast trends, customer behaviors, and market shifts. As a result, banks can better align their strategies to meet evolving demands and seize new opportunities.
Furthermore, AI facilitates more rapid decision-making processes. By automating data collection and analysis, banks can reduce the time it takes to arrive at strategic decisions. This efficiency not only streamlines operations but also aids in proactive risk management, ensuring that organizations can adapt swiftly to potential challenges. Ultimately, the incorporation of AI fosters a culture of continuous improvement, where strategic decisions are informed by real-time intelligence rather than relying solely on historical data or intuition.
Generate Journey maps, Mind maps, Bar charts and more from your data in Minutes
Implementing Predictive Banking Intelligence: Key Steps
To implement Predictive Banking Intelligence effectively, it’s essential to follow a structured approach. The first step involves comprehensive data collection and analysis. Gathering relevant data from various sources ensures that insights are based on robust information. This includes customer interactions, market trends, and internal performance metrics. Accurate analysis of this data forms the foundation for predictive models, guiding informed decision-making.
Next, integrating AI agents into existing systems is crucial. These agents facilitate real-time analysis, offering predictive insights that empower teams to make timely and strategic decisions. AI tools can be adjusted to fit specific banking needs, allowing for customization. Finally, evaluating strategic outcomes is necessary to assess the effectiveness of these initiatives. Continuous monitoring and refining strategies based on performance data guarantee that predictive banking intelligence evolves alongside market dynamics. By following these key steps, organizations can maximize the benefits of this advanced technology.
Step 1: Data Collection and Analysis
Data collection and analysis serve as pivotal foundations for Predictive Banking Intelligence. The first step involves gathering relevant data from diverse sources, including customer interactions, transaction histories, and external market conditions. A thorough compilation of this information will set the stage for insightful analysis. Furthermore, categorizing data appropriately can help identify significant trends and relationships that influence banking decisions.
Following data gathering, rigorous analysis must occur to transform raw data into actionable insights. This process involves examining patterns and anomalies within the data, ensuring accurate interpretation. Key analytical methods, such as statistical analysis and data visualization, allow decision-makers to distill complex information into clear, strategic insights. By understanding these trends, banks can foster improved customer experiences and optimize resource allocation. Ultimately, effective data collection and analysis are essential in empowering AI agents to generate impactful predictive outcomes in the banking sector.
Step 2: Integrating AI Agents
Integrating AI agents into banking systems is crucial for fostering predictive banking intelligence. This step involves seamless collaboration between these agents and existing databases to enhance decision-making processes. By harnessing the power of AI, financial institutions can analyze vast amounts of data quickly and accurately, enabling them to anticipate market trends and client needs.
AI agents must be trained and deployed strategically to maximize their effectiveness. Start by identifying the key areas where predictive insights are most needed, such as risk assessment and customer relationship management. Next, ensure that AI systems can access the right data sources and maintain high levels of accuracy. Regular updates and performance assessments are essential to optimize the agents' ability to provide relevant insights. As a result, integrating AI agents can transform banking practices, making them more responsive and data-driven.
Step 3: Evaluating Strategic Outcomes
In Step 3: Evaluating Strategic Outcomes, it is crucial to assess the effectiveness of AI Agents in facilitating Predictive Banking Intelligence. This evaluation process enables decision-makers to understand how accurately these agents forecast trends, assess risks, and identify opportunities in the banking sector. By systematically analyzing the outcomes, organizations can determine if the strategic objectives were met and how predictive models can be refined for better performance.
Evaluating outcomes involves several key components. First, performance metrics should be established to measure the success of the predictions made by AI Agents. Second, feedback mechanisms are necessary to gather insights from stakeholders affected by these predictions. Finally, iterative assessments must be conducted to ensure continuous improvement in the predictive models. By following this comprehensive evaluation framework, banks can enhance their strategic decision-making capabilities, driving growth and efficiency in an increasingly complex financial landscape.
💬 Questions about AI Agents for Predictive Strategic Decision Making in Banking?
Our team typically responds within minutes
Top Tools for Predictive Banking Intelligence
The tools used for predictive banking intelligence are essential for enhancing decision-making processes within financial institutions. These tools utilize advanced AI capabilities to analyze vast amounts of data, enabling banks to identify trends and make informed strategic choices. Understanding how these tools operate is crucial for maximizing their potential, as they provide valuable insights that guide banking practices.
Some top contenders in this space include IBM Watson, known for its robust analytics capabilities, and Microsoft Azure Machine Learning, which offers extensive integration options for various banking systems. DataRobot is another notable tool, specializing in automating machine learning processes, while H2O.ai provides an open-source platform for users to develop custom models. Each of these tools contributes uniquely to predictive banking intelligence, ultimately allowing banks to anticipate market changes and enhance customer experiences. Embracing these technologies opens the door to innovative solutions in banking strategy.
insight7
AI-driven Predictive Banking Intelligence offers a transformative approach to decision-making in the banking sector. By utilizing sophisticated algorithms and machine learning techniques, banks can analyze vast amounts of data to identify trends and forecast future outcomes. This predictive analysis enables financial institutions to make informed strategic decisions, reduce risk, and enhance operational efficiency.
To effectively implement Predictive Banking Intelligence, banks should focus on several key areas. Firstly, they must prioritize data collection and analysis, ensuring clean and relevant datasets. Next, integrating AI agents into existing systems is crucial, allowing real-time insights and adaptability to changing market conditions. Finally, evaluating strategic outcomes through ongoing metrics and KPIs will help refine predictive models and enhance their effectiveness. By embracing these strategies, banks can leverage the power of AI to drive better business performance and meet the evolving needs of their customers.
IBM Watson
IBM Watson has revolutionized the approach to predictive banking intelligence by harnessing advanced machine learning and natural language processing capabilities. By analyzing vast amounts of data, it empowers banks to make informed strategic decisions based on predictive analytics. This integration allows financial institutions to anticipate customer needs, streamline operations, and enhance risk management, ultimately fostering a more proactive business model.
Moreover, the ability to synthesize real-time insights helps institutions stay competitive in an ever-evolving financial landscape. By employing AI agents, banks can automate data interpretation, providing tailored recommendations that guide decision-making processes. As predictive insights become integral to strategic planning, the role of such intelligent systems will continue to expand, shaping the future of banking. Embracing predictive banking intelligence through these advancements will lead to improved customer satisfaction and operational efficiency.
Microsoft Azure Machine Learning
Microsoft Azure Machine Learning is transforming the landscape of predictive banking intelligence. This robust platform enables banks to develop, deploy, and manage machine learning models effectively. By leveraging its rich toolset, financial institutions can analyze vast datasets, uncover trends, and gain insights that inform strategic decision-making.
A key feature of Azure Machine Learning is its scalability, allowing banks to process and analyze extensive customer data in real-time. Additionally, the platform’s user-friendly interface enables non-technical staff to build models, democratizing data-driven decision-making across the organization. The integration of AI agents into Azure enhances predictive capabilities, allowing banks to forecast market changes and customer needs accurately. This proactive approach to decision-making is crucial for staying competitive in the ever-evolving banking industry.
As institutions harness Microsoft Azure Machine Learning, they can create tailored solutions that enhance customer experiences and drive growth in predictive banking intelligence.
DataRobot
Data manipulation and analysis have transformed significantly in recent years, with advanced platforms emerging to support predictive banking intelligence. These tools facilitate a deeper understanding of patterns and trends in the ever-evolving financial landscape. An intuitive interface enables banking professionals to easily generate actionable insights from vast data sets. By integrating AI agents, the process of predictive analysis becomes streamlined, helping institutions make informed strategic decisions.
The functionality of these platforms extends beyond mere data processing. Users can configure reports to align with specific business metrics, optimizing their decision-making processes. Furthermore, they can engage in custom evaluations, allowing for tailored insights that meet unique organizational needs. This adaptability enhances the strategic foresight necessary in the banking sector, positioning institutions to respond effectively to market changes and customer demands. Embracing these technologies aids in fostering a proactive approach to financial management and customer engagement.
H2O.ai
H2O.ai delivers advanced machine learning capabilities tailored for the financial sector, enhancing predictive banking intelligence. The platform enables banks to analyze vast streams of data, thus transforming raw information into actionable insights. By employing sophisticated modeling techniques, financial institutions can predict customer behaviors, assess risks, and optimize their strategic decisions accordingly.
One of the key advantages of H2O.ai is its user-friendly interface, allowing analysts to engage with complex data without extensive coding experience. Moreover, the platform supports various data sources, making it versatile for diverse banking applications. Financial organizations can leverage these insights to refine their approach to customer service, compliance, and overall operational efficiency. As the banking industry evolves, integrating such AI-driven solutions will be essential for maintaining competitiveness and driving growth in the rapidly changing landscape of financial services.
Conclusion: The Future of AI-Driven Predictive Banking Intelligence
The future of AI-driven predictive banking intelligence promises to transform decision-making processes within the banking sector. As financial institutions embrace advanced analytical capabilities, AI agents will enhance their ability to forecast trends and identify opportunities. These intelligent systems can analyze vast amounts of data more rapidly and accurately than traditional methods, enabling banks to respond proactively to market changes.
Moreover, implementing predictive banking intelligence will empower institutions to tailor their services to customer needs effectively. The integration of AI not only improves efficiency but also fosters a proactive approach to risk management and customer engagement. In this evolving landscape, organizations that embrace these technologies will undoubtedly gain a competitive edge.
💬 Questions about AI Agents for Predictive Strategic Decision Making in Banking?
Our team typically responds within minutes