Top 10 AI Agents for Predictive Strategic Decision Making
-
Hello Insight
- 10 min read
Predictive AI Strategy is revolutionizing the landscape of strategic decision-making. In an era driven by data, organizations are discovering that the insights offered by predictive algorithms can significantly enhance decision-making processes. Gone are the days of relying solely on intuition; businesses now harness data to forecast trends, understand customer behaviors, and optimize operations.
Implementing a robust predictive AI strategy involves understanding critical components such as data collection and analysis. By leveraging advanced machine learning models, decision-makers can gain actionable insights that not only inform current strategies but also shape future initiatives. As we explore the top AI agents in this domain, it becomes evident that these tools are essential for organizations aiming to stay competitive in a data-centric world.
Analyze & Evaluate Calls. At Scale.
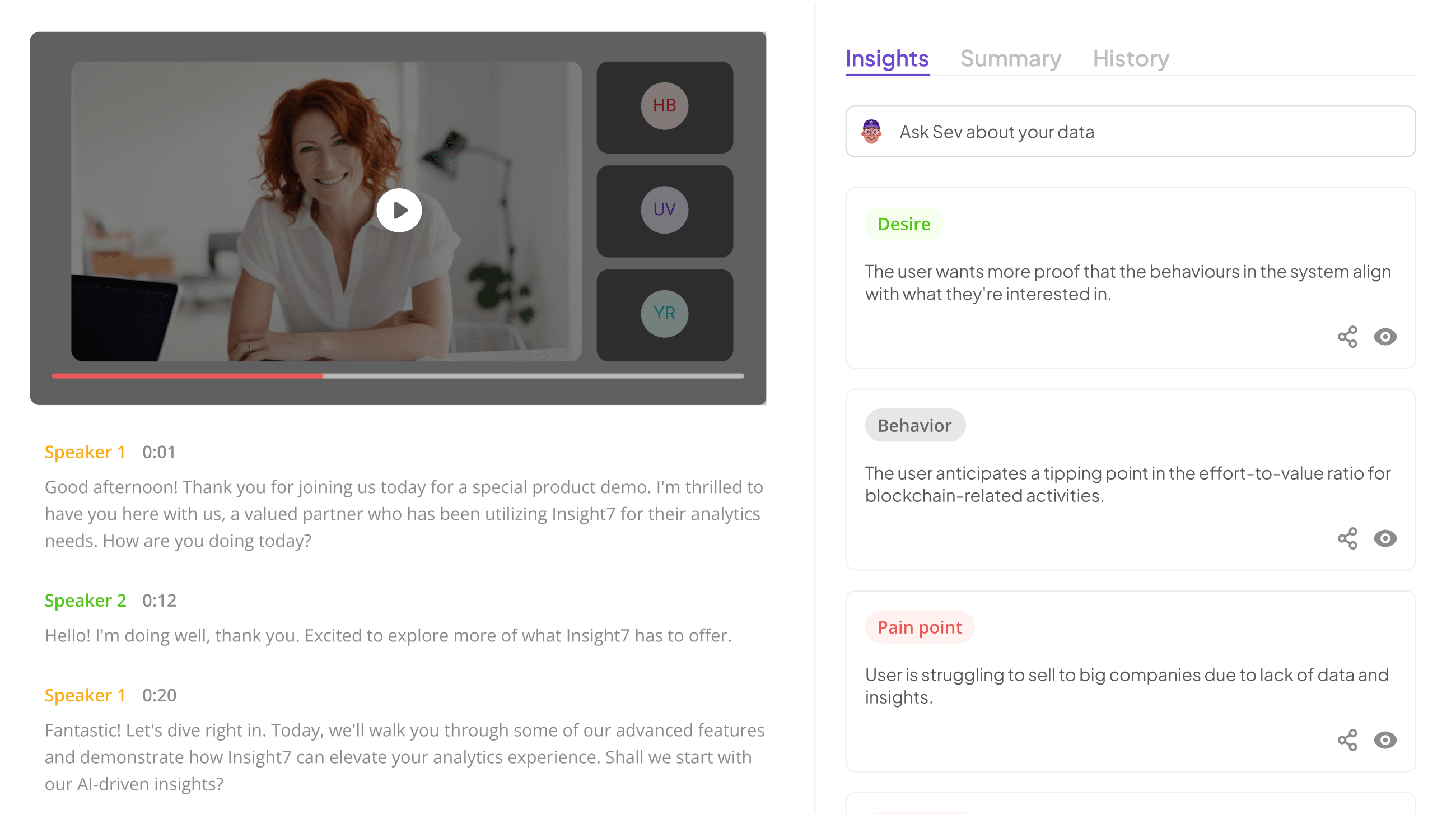
Understanding Predictive AI Strategy in Decision Making
Understanding Predictive AI Strategy in Decision Making is essential for organizations looking to refine their decision-making processes. Predictive AI strategy integrates data analytics and machine learning, making it possible to forecast future trends and behaviors. By leveraging this approach, businesses can make informed decisions that drive growth and efficiency.
Key components of a successful predictive AI strategy include data collection and analysis, which form the backbone of effective forecasting. Machine learning models help in interpreting complex data sets and generating insights. Organizations must invest in these technologies to remain competitive. Furthermore, being specific in objectives and the data needed significantly enhances the accuracy of predictions. As companies embrace this strategy, they will better manage risks and seize opportunities, paving the way for more strategic, data-driven decisions.
What is Predictive AI Strategy?
Predictive AI Strategy refers to the systematic approach of leveraging artificial intelligence to anticipate outcomes and guide decisions. In today’s competitive landscape, organizations cannot afford to rely solely on intuition or historical data; they must embrace systematic predictions rooted in data analysis. This strategy emphasizes the importance of understanding market trends, customer behavior, and operational efficiencies to drive informed decision-making.
To implement a successful Predictive AI Strategy, companies should focus on two key components: robust data collection and advanced machine learning algorithms. First, organizations need to gather relevant data consistently, ensuring it is thorough and diverse. Second, employing effective machine learning models enables the interpretation of this data, identifying patterns that help forecast future events. By integrating these elements into their strategic planning, businesses can enhance their decision-making processes and stay ahead in their respective industries.
- Definition and significance in strategic planning.
Strategic planning requires a clear understanding of potential outcomes, and that's where a Predictive AI Strategy comes into play. This approach integrates advanced algorithms and data analytics to forecast future trends and facilitate informed decision-making. By leveraging historical data and real-time insights, organizations can identify opportunities and mitigate risks, ultimately aligning their strategic goals with predicted market behaviors.
The significance of this strategy in decision-making cannot be overstated. It enhances the ability to make proactive choices rather than reactive ones, allowing businesses to adapt to changes quickly. Furthermore, predictive AI tools assist in segmenting the market, understanding consumer behavior, and optimizing resource allocation. In today's fast-paced environment, having a robust Predictive AI Strategy is not merely an advantage; it is essential for sustaining competitive edge and driving innovation.
- How predictive models enhance decision-making processes.
Predictive models significantly enhance decision-making processes by transforming data into actionable insights. These models analyze historical data, uncover patterns, and simulate various scenarios, allowing decision-makers to foresee outcomes effectively. By employing a Predictive AI strategy, organizations reduce uncertainty and improve strategic planning. This means leaders can allocate resources wisely and anticipate market trends more accurately.
Moreover, decision-making becomes agile as predictive models offer real-time analytics. As businesses face rapid changes, these models adapt to new data inputs, ensuring strategies remain relevant. Organizations benefit from improved forecasting accuracy, enabling them to respond proactively rather than reactively. Ultimately, integrating predictive models into decision-making not only streamlines processes but also validates choices with data-backed evidence, leading to enhanced overall performance.
Key Components of Predictive AI Strategy
A successful Predictive AI Strategy begins with strategic data collection and analysis. Understanding what data is essential for predictions hinges on the specific outcomes you aim to achieve. By leveraging historical data and identifying relevant variables, organizations can build a solid foundation for effective predictive modeling. Additionally, the integration of advanced analytics empowers leaders with insights on customer behavior, market trends, and operational efficiency.
Machine learning models and algorithms play a pivotal role in the overall Predictive AI Strategy framework. Selecting the appropriate models requires an understanding of their strengths and limitations relative to the task at hand. From supervised learning techniques like regression to unsupervised methods such as clustering, each choice should align with the desired strategic objectives. By deploying these models effectively, organizations can harness the power of predictive analytics, driving informed decisions and sustainable growth.
- Data collection and analysis.
Data collection and analysis are crucial steps in crafting a successful predictive AI strategy. To ensure meaningful results, organizations must focus on gathering high-quality data from various sources. This includes customer feedback, market trends, and competitive insights, allowing for a comprehensive understanding of the environment. By analyzing this data, AI agents can identify patterns, make predictions, and inform strategic decisions that align with business objectives.
The process involves several key steps. First, organizations should establish their data collection frameworks, ensuring they capture relevant information. Next, data analysis techniques, such as sentiment analysis or trend analysis, enable businesses to extract actionable insights. By leveraging these insights, companies can refine their strategies and enhance decision-making processes, ultimately leading to improved outcomes. In a rapidly changing business landscape, understanding data collection and analysis is vital for staying competitive and informed.
- Machine learning models and algorithms used in predictions.
Machine learning models and algorithms are central to developing a robust Predictive AI Strategy in any strategic decision-making process. Among the most commonly used models are linear regression and decision trees, each offering unique advantages for interpreting complex datasets. Linear regression allows for straightforward predictions based on relationships between variables, while decision trees provide a visual representation, making them user-friendly for understanding decision pathways.
Additionally, ensemble methods, such as random forests and gradient boosting, enhance prediction accuracy by combining the outputs of multiple models. Neural networks also play a significant role because of their capability to capture intricate patterns in large datasets. Understanding and selecting the appropriate models is critical, as different algorithms can yield distinct insights based on the nature of the data at hand. This careful selection is essential to formulating effective predictive strategies that drive informed decisions and foster organizational success.
Extract insights from interviews, calls, surveys and reviews for insights in minutes
Top AI Agents for Predictive Strategic Decision Making
In today's data-driven landscape, selecting the right AI agents for predictive strategic decision-making is crucial. These AI tools harness vast amounts of data to enhance business strategies and optimize outcomes. The focus should be on how each agent operates within a Predictive AI Strategy, driving meaningful insights and foresight.
Understanding the capabilities of each AI agent can significantly impact strategic planning processes. First, H2O.ai stands out with its innovation in Automated Machine Learning, enabling users to swiftly create robust predictive models. Then, there's DataRobot, which simplifies model creation for businesses by democratizing access to machine learning. RapidMiner excels by providing comprehensive data science solutions, facilitating end-to-end project execution. Lastly, IBM Watson harnesses the power of AI to generate actionable insights, supporting strategic forecasting that aligns with business objectives. Selecting the appropriate agents can propel organizations towards achieving their long-term goals through informed decision-making.
insight7: Leading the Charge
In the realm of strategic decision-making, insight7 is at the forefront, paving the way for organizations striving to harness the power of predictive AI. Leading the charge means equipping businesses with advanced tools to sift through vast amounts of data, transforming raw insights into actionable strategies. This capability is essential for staying competitive in today’s rapidly evolving marketplace.
The significance of a sound Predictive AI Strategy lies in its ability to provide insights that are timely and relevant. Organizations that effectively implement such strategies enhance their decision-making processes with data-driven approaches. By employing predictive models, companies can anticipate market changes and develop strategies that are not only reactive but proactive, thus ensuring sustained success in their respective industries.
- Overview of insight7’s capabilities.
The overview of capabilities highlights how this platform empowers users to make informed decisions through advanced predictive AI strategies. Designed with user accessibility in mind, the interface allows anyone in the organization to gather insights without requiring specialized training or technical skills. Users can easily access tools that facilitate the analysis of customer interactions, ensuring that data-driven reports and insights are just a few clicks away.
Central to the platform's effectiveness is its ability to consolidate various data points from customer conversations. By automatically extracting pain points, desires, and behavioral patterns, it enables teams to visualize and analyze trends in real-time. This seamless approach not only enhances the understanding of customer needs but also aids strategic decision-making by providing evidence-based insights. Ultimately, the platform serves as a powerful resource for businesses aiming to implement a cohesive predictive AI strategy for informed, strategic choices.
- Key features that set it apart in predictive strategic decision making.
In the realm of predictive strategic decision-making, certain distinctive features significantly differentiate leading AI agents. One of the main attributes is their ability to democratize data insights. These platforms are designed for ease of use, enabling anyone in the organization to access and interpret data without needing specialized expertise. This accessibility fosters a culture of data-driven decision-making, empowering team members to generate actionable insights independently.
Another key feature is advanced analytical capabilities. Leading AI agents employ powerful algorithms to sift through vast amounts of data, extracting valuable patterns and trends effectively. This enables organizations to anticipate market shifts and consumer behavior with high precision. Additionally, these technologies often incorporate real-time processing, allowing decision-makers to respond rapidly to evolving conditions. Collectively, these attributes form a robust predictive AI strategy that not only enhances decision quality but also aligns with organizational goals, paving the way for sustainable growth and innovation.
Other Notable AI Agents
Several notable AI agents complement the leading platforms in predictive strategic decision-making. These agents contribute significantly to developing a robust predictive AI strategy that can effectively inform organizational decisions. Each one brings unique capabilities that businesses can leverage to enhance their forecasting and analytical capacities.
H2O.ai stands out for its innovation in automated machine learning. This agent simplifies the complex modeling processes, allowing engineers with limited expertise to create high-quality predictive models. DataRobot excels in streamlining the predictive model creation process, empowering businesses to harness machine learning effortlessly. RapidMiner offers comprehensive solutions designed for end-to-end data science and machine learning tasks, making it accessible for teams looking to adopt predictive capabilities without extensive resources. Lastly, IBM Watson integrates advanced AI for actionable insights, enhancing strategic forecasting through intelligent data analysis. Together, these AI agents broaden the landscape for organizations seeking to implement a predictive AI strategy, ensuring they remain competitive and informed in their decision-making processes.
- H2O.ai: Innovation in Automated Machine Learning (AutoML).
Automated Machine Learning (AutoML) represents a transformative shift in how organizations approach machine learning tasks. This innovation simplifies the implementation of predictive AI strategies, enabling users to generate accurate models without requiring in-depth technical expertise. By streamlining data processing and automating model selection, businesses can focus on strategic decision-making rather than getting bogged down in complex algorithms.
One significant advantage is decreased time-to-insight. Organizations can quickly deploy predictive models, allowing for real-time analysis and rapid adjustments to business strategies. This responsiveness is crucial in today’s fast-paced market. As teams utilize automated tools to gather and analyze data efficiently, they unlock new opportunities for growth and adaptation, highlighting AutoML's role in enhancing strategic decision-making frameworks. Such innovations make it possible for organizations to thrive in an increasingly competitive landscape.
- DataRobot: Simplifying predictive model creation for businesses.
For businesses aiming to bolster their predictive AI strategy, creating effective models becomes crucial. A user-friendly platform allows team members across various departments to generate insights without needing extensive background knowledge. This ease of access democratizes data analysis, ensuring everyone can participate. Individuals can simply upload datasets, ask questions, and extract valuable insights from customer interactions and other relevant data sources.
The process is designed to be intuitive, providing tools to analyze conversations, identify pain points, and visualize outcomes. As companies harness these capabilities, they can easily collaborate on projects that pool insights from multiple sources. This flexibility promotes a data-driven culture where decisions are guided by evidence rather than intuition, enabling organizations to enhance their overall strategic planning. Thus, simplifying predictive model creation supports a more informed and agile approach to decision-making.
- RapidMiner: Providing end-to-end data science and machine learning solutions.
RapidMiner offers comprehensive solutions for organizations looking to integrate predictive AI strategy into their decision-making processes. Its end-to-end data science and machine learning capabilities streamline data handling from collection to analysis. This platform enables users to build, evaluate, and deploy predictive models efficiently, making it an invaluable tool for data-driven strategies. Organizations can easily tailor their data projects, ensuring alignment with specific business goals.
The intuitive project management features simplify the workflow for users of various skill levels. With advanced analytics options, it helps uncover meaningful patterns and insights, contributing to informed strategic planning. By harnessing the power of machine learning, organizations can enhance their predictive capabilities, ultimately improving decision-making quality. The focus on user experience ensures accessibility, making predictive analytics a practical approach for a variety of sectors. This integration fosters an environment where data informs strategy, enabling organizations to remain competitive.
- IBM Watson: Leveraging AI for actionable insights and strategic forecasting.
Predictive AI strategy is transforming how organizations approach actionable insights and strategic forecasting. By harnessing advanced technologies, businesses can analyze vast data sets to derive meaningful conclusions. This process often involves the integration of various algorithms and models that learn from historical patterns to predict future trends, enabling organizations to make informed decisions with enhanced accuracy.
One of the standout features of this strategy is its user-friendly design, which allows all team members to access critical insights without needing extensive training. This democratization of data empowers employees to engage directly with the platform, identifying pain points and opportunities within customer interactions. As insights are extracted, stakeholders can visualize key themes, driving objective discussions and strategic alignment towards organizational goals. By actively incorporating predictive analytics into decision-making processes, businesses position themselves to adapt swiftly to market changes while maintaining a competitive edge.
Implementing Predictive AI Strategy
Implementing a Predictive AI Strategy begins with defining clear objectives and expectations. Establishing specific goals ensures that the AI tools selected align with the organization's strategic vision. This clarity helps in setting measurable benchmarks to assess the effectiveness of the AI deployment. Collecting and preparing data is the next crucial step. Quality data significantly enhances the accuracy of predictive algorithms, making it essential to establish robust data governance protocols.
Selecting the right AI tools and technologies cannot be overstated. Various options exist within the market, each offering unique strengths for predictive analytics. Organizations should closely analyze their specific needs and choose tools that integrate seamlessly with their existing systems. Besides, challenges such as data quality issues and implementation pitfalls may arise, requiring proactive strategies to mitigate risks. A well-structured approach to overcoming these challenges is vital for successful AI strategy implementation.
Steps for Successful Implementation
Successful implementation of a Predictive AI Strategy demands clarity and organization throughout the process. Begin by defining clear objectives and expectations for your predictive models. Articulating specific goals helps align the efforts of teams and ensures that everyone understands their roles and responsibilities. This clarity guides the subsequent steps, creating a roadmap towards effective decision-making.
Next, gathering and preparing your data efficiently is crucial. High-quality, relevant data serves as the foundation for accurate predictions. Invest time in data cleaning and preprocessing to eliminate inconsistencies that could skew results. Finally, selecting the appropriate AI tools and technologies empowers your team to leverage advanced machine learning capabilities. Evaluate potential solutions critically, ensuring they meet the unique needs of your organization and align with your strategic goals. By following these steps, you enhance the likelihood of a successful predictive AI implementation.
- Define clear objectives and expectations.
To define clear objectives and expectations, organizations must align their goals with the capabilities of their predictive AI strategy. Start by articulating what you aim to achieve. For instance, specify target metrics such as improved forecasting accuracy or enhanced decision-making speed. Clear objectives not only guide the implementation process but also serve as a benchmark for evaluating success.
Next, establish realistic expectations about the AI agents you plan to implement. Predictive AI can significantly influence strategic decision-making, but it requires time to demonstrate its value. Be prepared for a learning curve as your team adapts to new tools and processes. By setting these clear objectives and expectations upfront, you create a roadmap that enhances collaboration and drives measurable results. This clarity not only boosts confidence in the technology but also fosters a culture of data-informed decision-making within the organization.
- Gather and prepare data efficiently.
In the realm of predictive strategic decision-making, gathering and preparing data efficiently is crucial for success. When implementing a Predictive AI Strategy, organizations should begin by identifying relevant data sources that contain valuable insights. This process involves ensuring that data is accurate, complete, and up to date. Poor data quality can lead to misguided predictions and ineffective decision-making, so it's essential to vet all data before analysis.
Once you've gathered the necessary data, the next step is to preprocess it for analysis. This may include cleaning the data, normalizing formats, and organizing it into accessible formats. Various tools and technologies can facilitate this process, allowing teams to aggregate information quickly. By efficiently preparing data, businesses can leverage it more effectively in their predictive models, ultimately leading to more informed and strategic decisions. A streamlined data preparation process enhances the overall Predictive AI Strategy, allowing for better analysis and insights as businesses navigate their competitive landscapes.
- Choose the right AI tools and technologies.
Choosing the right AI tools and technologies is crucial for effective predictive AI strategy implementation. First, assess your specific needs, such as the nature of the data and desired outcomes. Different tools excel in various areas, so it’s important to align them with your strategic objectives. For instance, tools specializing in automated machine learning can streamline model creation, while those focused on data visualization can enhance insight communication.
Next, consider scalability and collaboration features. As your organization grows, the AI tools must adapt to evolving demands and integrate seamlessly with existing systems. Finally, prioritize user-friendliness; tools that require extensive training can hinder prompt decision-making. By carefully evaluating available options, you empower your team to leverage predictive AI effectively, thus enhancing strategic decision-making.
Challenges and How to Overcome Them
Implementing a predictive AI strategy can be met with various challenges that organizations must navigate. One major issue is ensuring data quality and integrity; poor data can lead to inaccurate predictions. To address this, it is essential to establish robust data governance frameworks that enforce data accuracy and consistency. Another common challenge involves the complexity of integrating AI tools into existing systems. Seamless integration requires thoughtful planning and adequate training for team members to adapt to new workflows.
Additionally, organizations may face resistance to adopting AI-driven approaches due to a lack of understanding or fear of change. Overcoming this requires a cultural shift; education and awareness campaigns can demystify AI’s role in decision-making. By emphasizing the benefits, organizations can foster an environment more conducive to embracing predictive AI strategy, transforming challenges into opportunities for growth and innovation in strategic decision making.
- Common pitfalls in predictive AI strategies.
In a robust predictive AI strategy, pitfalls can hinder your outcomes and misguide decision-making. One common mistake is failing to establish clear objectives. Without defined goals, data may be analyzed without direction, leading to inconclusive results. Moreover, neglecting to assess data quality often leads to flawed insights. Poor-quality data can cause algorithms to generate inaccurate predictions, which may lead to misguided strategies.
Another significant pitfall is the absence of specificity in model requirements. Vague parameters can result in algorithms that function poorly and fail to tackle the intended problems. To avoid these missteps, stakeholders should engage in continuous review of the predictive models in practice. Regular evaluations can help in correcting any deviations in strategy, ensuring your predictive AI efforts lead to informed and strategic decisions. Adopting best practices will also facilitate a more effective implementation of predictive AI, steering clear of these common pitfalls.
- How to ensure data quality and integrity.
Ensuring data quality and integrity is essential for a successful Predictive AI Strategy. High-quality data serves as the foundation for effective decision-making, significantly influencing the accuracy of predictive models. Organizations should begin by systematically collecting data from reliable sources, ensuring that the data is complete and relevant to the objectives at hand. Regular audits and quality checks can help identify inconsistencies and rectify errors before they impact analysis outcomes.
It's crucial to establish robust data governance policies that outline roles, responsibilities, and best practices for data management. Investing in appropriate tools for ongoing monitoring and cleansing can further enhance data integrity. Additionally, integrating feedback loops allows for continuous improvement, ensuring that any emerging issues are addressed quickly. By prioritizing data quality in these ways, organizations can confidently utilize artificial intelligence to drive strategic decisions, thereby maximizing the benefits of their Predictive AI Strategy.
Conclusion on Embracing Predictive AI Strategy
Embracing a predictive AI strategy is essential for organizations aiming to enhance their decision-making capabilities. By integrating advanced AI agents, businesses can accurately forecast trends and make informed choices that align with their strategic goals. These tools not only streamline data analysis but also uncover valuable insights that drive innovation and efficiency.
To fully benefit from this strategic approach, organizations must commit to understanding the intricacies of predictive AI. By establishing clear objectives and utilizing effective data collection methods, companies can harness the power of predictive AI agents. This commitment ultimately leads to enhanced performance and a significant competitive edge in the marketplace. Embracing a predictive AI strategy is not just an option; it's a necessity for sustainable growth.