Predictive Behavior Analytics serves as a vital framework for understanding and forecasting actions within diverse user groups. As organizations increasingly rely on artificial intelligence, the fusion of these analytical insights with AI agents can unveil patterns and trends that drive decision-making. Businesses today face the challenge of interpreting vast amounts of data, and predictive analytics plays a crucial role in streamlining this process.
Integrating Predictive Behavior Analytics into AI systems enhances their ability to interpret user behavior, leading to more personalized experiences. This technology empowers organizations to align their strategies with customer needs effectively, ultimately fostering deeper connections and improving overall engagement. By leveraging data-driven insights, businesses can anticipate market shifts and adapt quickly, ensuring they remain competitive in a rapidly evolving landscape.
Analyze qualitative data. At Scale.
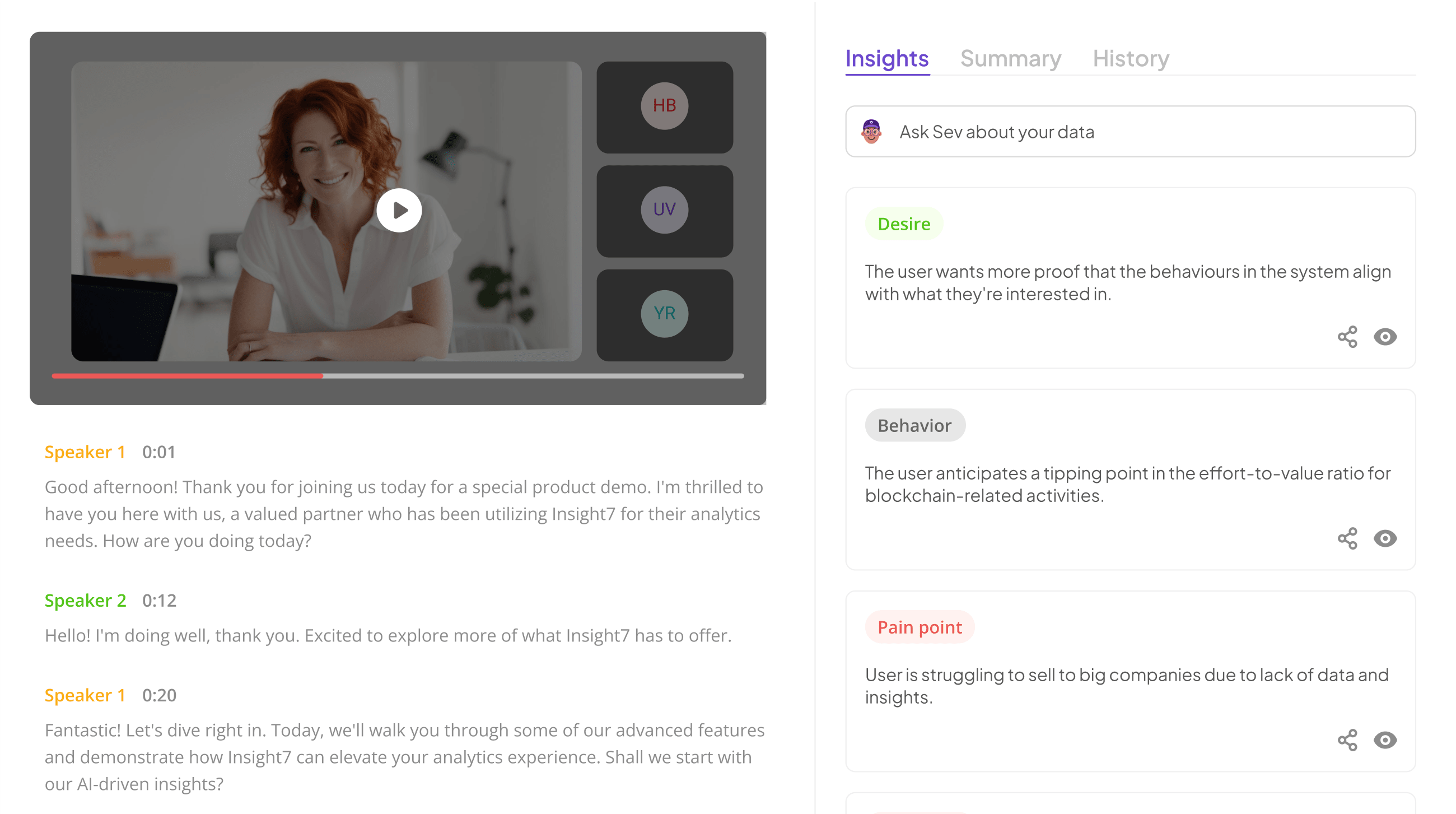
Understanding Predictive Behavior Analytics
Predictive Behavior Analytics offers valuable insights into customer actions by analyzing historical data patterns. This analytical approach empowers businesses to anticipate future customer behaviors, leading to informed decision-making. Key techniques involve statistical algorithms and machine learning models that refine understanding of trends and preferences.
Understanding how these analytics operate is crucial for maximizing their potential. Firstly, data collection remains integral, as accurate insights depend on the quality and relevance of the information gathered. Secondly, applying the right analytical tools enhances the ability to visualize trends effectively, making it easier to derive actionable insights. Finally, regular updates and model adjustments ensure the predictive capabilities evolve with changing consumer behaviors, maintaining their relevance in a fast-paced market.
By grasping the elements of Predictive Behavior Analytics, businesses can harness the power of data-driven decision-making to enhance customer experiences and drive growth.
What is Predictive Behavior Analytics?
Predictive Behavior Analytics is a powerful method that uses data analysis to anticipate future actions based on past behavior. This approach combines historical data with advanced analytical techniques to identify patterns, enabling businesses to make informed decisions. By focusing on customer behavior, organizations can forecast trends and tailor their strategies to meet evolving demands.
One key aspect of Predictive Behavior Analytics involves the analysis of large datasets to draw actionable insights. Utilizing AI models, businesses can refine their understanding of customer preferences and address potential challenges before they escalate. This proactive stance not only enhances customer satisfaction but also promotes sustainable growth. Furthermore, these analytics allow for personalized marketing initiatives, improving engagement and retention rates.
In summary, Predictive Behavior Analytics serves as a vital tool for businesses seeking to navigate complex markets effectively and efficiently. By employing this analytical framework, organizations can transform their data into strategic assets that support decision-making and drive long-term success.
Why is it Important for Businesses?
In the rapidly evolving business world, understanding customer behavior is crucial. Predictive behavior analytics allows businesses to anticipate future actions and preferences of their clients. This strategic foresight can help companies tailor their products and services, ultimately enhancing customer satisfaction and fostering loyalty.
By utilizing these analytics, organizations can identify trends, assess risks, and make informed decisions. Having reliable data not only aids in optimizing marketing strategies but also improves operational efficiencies. Businesses can allocate resources more effectively, respond to market changes swiftly, and maintain a competitive edge. In essence, embracing predictive behavior analytics transforms how businesses interact with customers, paving the way for innovative solutions and long-term growth.
Overall, the integration of these insights is not just beneficial; it is essential for staying relevant and successful in todayโs dynamic market environment.
Extract insights from interviews, calls, surveys and reviews for insights in minutes
Top AI Agents for Predictive Behavioral Data Insights
In exploring the top AI agents for predictive behavioral data insights, businesses must understand the power they hold in transforming data into actionable insights. These AI agents utilize advanced algorithms to analyze historical and real-time data, providing organizations with a clearer understanding of customer behaviors. By leveraging predictive behavior analytics, companies can anticipate trends, enhance customer experiences, and ultimately drive revenue growth.
Several leading AI solutions stand out in this domain. Firstly, IBM Watson Predictive Analytics excels in processing vast amounts of data, delivering precise insights that inform better decision-making. Then we have Google Cloud AI Platform, which offers robust tools for integrating predictive models into existing workflows. Microsoft Azure Machine Learning follows suit, providing flexible options for businesses, allowing them to adapt their predictive analytics strategies as they grow. Lastly, SAS Customer Intelligence 360 stands out with its customer-centric approach, ensuring that predictive insights align closely with business goals. Together, these AI agents empower organizations to decode consumer behavior effectively and tailor their strategies accordingly.
insight7: Leading the Way
In the dynamic realm of Predictive Behavior Analytics, understanding consumer behaviors is pivotal for businesses. This section highlights the significance of AI agents in navigating complexities and extracting valuable insights. These agents utilize advanced algorithms to interpret vast amounts of data. As organizations strive to remain competitive, they must prioritize tools that can efficiently analyze behavioral trends.
Leading the way in this arena involves recognizing key challenges that firms face in data analysis. Firstly, businesses encounter difficulties in managing and interpreting overwhelming data volumes. Secondly, traditional analysis methods often prove inadequate, slowing down decision-making processes. Finally, the integration of insights into actionable strategies remains a hurdle. By addressing these problems, Predictive Behavior Analytics not only enhances customer engagement but also ensures that organizations adapt swiftly to market changes. Embracing innovative AI solutions is essential for fostering agility in todayโs data-driven landscape.
IBM Watson Predictive Analytics
IBM Watson Predictive Analytics provides businesses with sophisticated tools for understanding customer behavior through data interpretation. This platform excels in predictive behavior analytics by utilizing advanced algorithms that assess historical data trends. By analyzing these trends, businesses can effectively anticipate customer needs and preferences.
The core functionality centers around machine learning and natural language processing, allowing users to derive meaningful insights from complex datasets. For example, it enables organizations to examine customer feedback, identifying both positive and negative sentiments across different interactions. The ability to compare data from various sources, such as service centers or demographic locations, enhances insight generation. By strategically leveraging these insights, businesses are better equipped to make informed decisions that drive engagement and loyalty.
In summary, effective implementation of predictive behavior analytics through this platform can lead to actionable strategies that profoundly impact customer satisfaction and overall business performance.
Google Cloud AI Platform
The Google Cloud AI Platform provides robust tools for organizations striving to enhance their predictive behavior analytics capabilities. By integrating machine learning models with user-friendly interfaces, businesses gain valuable insights into customer behavior patterns. The platform enables the ingestion and processing of vast amounts of data, resulting in accurate predictions that drive efficient decision-making.
Additionally, this platform supports various applications, such as real-time analytics and interactive dashboards. Organizations can visualize trends and anomalies, empowering teams to act swiftly. Data privacy and compliance are central to its architecture, ensuring that user information remains secure. Through continuous updates and technological advancements, the Google Cloud AI Platform positions itself as a leading choice for companies focused on transforming analytical insights into actionable strategies.
In summary, leveraging this platform not only enhances predictive capabilities but also aligns organizations with market demands, setting them on the path to long-term success.
Microsoft Azure Machine Learning
Microsoft Azure Machine Learning stands out as a powerful platform for applying predictive behavior analytics. This intuitive tool allows users to easily build, deploy, and manage machine learning models, enhancing their ability to derive meaningful insights from data. By leveraging automated machine learning capabilities, organizations can quickly identify trends and patterns that inform strategic decisions.
Additionally, the platform offers a comprehensive suite of data processing tools. Users can integrate data from various sources, clean it efficiently, and prepare it for analysis. This streamlined process facilitates the identification of critical behavioral trends among customers, ultimately enhancing predictive capabilities. With built-in support for popular programming languages and frameworks, teams can seamlessly collaborate on projects, ensuring that insights from predictive behavior analytics are both actionable and reliable.
SAS Customer Intelligence 360
SAS Customer Intelligence 360 offers an advanced platform dedicated to transforming raw data into actionable insights. By deploying sophisticated algorithms, it excels in Predictive Behavior Analytics, enabling businesses to anticipate customer needs and preferences more effectively. With its intuitive interface, users can easily access detailed dashboards that visualize key trends and segmented data, providing a clearer understanding of shifting consumer behaviors.
The platformโs strength lies in its ability to analyze vast amounts of data from multiple sources in real time. It empowers organizations to create tailored marketing strategies based on predicted outcomes, improving customer engagement significantly. Through continuous learning from diverse customer interactions, the system refines its predictive models, ensuring that marketing efforts resonate authentically with target audiences. Businesses can rely on these insights to make informed decisions, enhancing their overall marketing effectiveness and customer satisfaction.
Step-by-Step Guide to Implementing Predictive Behavior Analytics
To implement Predictive Behavior Analytics effectively, begin by clearly defining your objectives. Identify the specific outcomes you want to achieve, ensuring they align with your business goals. Communicate these objectives to your team to foster a shared understanding and commitment.
Next, select the right tools that suit your analytical needs. Research various platforms to find one that balances functionality with user-friendlinessโcritical for ensuring accessibility across the organization. Once equipped with the appropriate tools, focus on data collection and preparation. Gather relevant data sources while cleaning and organizing them to ensure accuracy and reliability.
Finally, as you build and test your analytical models, continuously analyze the results to refine your insights. Utilize the findings to scale insights across departments. This iterative process will enhance your predictive accuracy and empower your business decisions. By following this structured approach, you can ensure that your organization fully reaps the benefits of Predictive Behavior Analytics.
Planning Your Predictive Analytics Strategy
To effectively plan your predictive analytics strategy, start by defining your key objectives. This means identifying what you want to achieve with predictive behavior analytics. Clear goals can help you focus your efforts and resources on deriving actionable insights. Whether you're aiming to enhance customer engagement, forecast sales trends, or streamline operations, having defined objectives is crucial for guiding your analytics initiatives.
Next, selecting the right tools is essential. The market offers a variety of AI agents to meet different predictive analytics needs. Conduct thorough research to find the tools that align best with your business objectives. Collecting and preparing data also plays a pivotal role. Ensure your data is accurate, relevant, and well-structured for analysis. Focusing on these foundational steps will set you on the right path for successful insights through predictive behavior analytics.
Step 1: Define Your Objectives
To effectively harness the potential of predictive behavior analytics, the first step is to define your objectives clearly. Understanding precisely what you aim to achieve allows you to focus your efforts, ensuring that your use of AI agents aligns with your business goals. Begin by identifying the specific behaviors or trends you wish to predict. This clarity will guide the selection of the right AI tools and data sources later in the process.
Moreover, setting measurable and achievable objectives aids in evaluating the success of your predictive analytics initiatives. Consider the broader impact on your organization, such as enhancing customer experience or optimizing marketing strategies. Take time to reflect on the questions of who your target audience is and how their behavior influences your business outcomes. By effectively articulating your objectives, you lay a solid foundation for implementing predictive behavior analytics that delivers actionable insights and drives informed decision-making.
Step 2: Choose the Right Tools
When embarking on your journey into predictive behavior analytics, selecting the appropriate tools is crucial. Start by understanding your specific needs; the right tools should align seamlessly with your objectives and offer the features that cater to your data requirements. Evaluate different platforms by considering their capabilities, ease of use, and integration options. This ensures that youโre equipped to extract meaningful insights from the data at hand.
Next, prioritize tools that emphasize data security and analysis. Many leading platforms not only provide robust analytics features but also maintain compliance with data protection regulations. Look for tools that allow you to visualize data in actionable formats, enhancing your decision-making process. With the right tools in place, you will enable your team to generate insights efficiently, ultimately driving your strategy forward with predictive behavioral data.
Step 3: Data Collection and Preparation
Data collection and preparation are vital components of predictive behavior analytics. This step focuses on gathering the right types of data needed to inform predictive models effectively. Start by identifying the data sources available, such as customer transactions, feedback, and behavioral patterns. Ensure that the chosen data is comprehensive and relevant to the objectives you have set.
Next, data cleaning is essential. This involves removing inconsistencies, duplicates, and irrelevant information from the datasets collected. Proper preparation not only enhances the accuracy of the predictive models but also builds trust in the insights generated. Once the data is cleaned and formatted, it becomes much easier to analyze, allowing for meaningful predictions about future behaviors. Adhering to these steps ensures that the foundation for predictive behavior analytics is robust and ready for subsequent analysis and model building.
Executing and Refining Insights
To effectively execute and refine insights from predictive behavior analytics, businesses must adopt a systematic approach. The first step involves model building and testing, which ensures that the analytical models accurately reflect real-world behaviors. It is crucial to validate these models using historical data, as this provides a benchmark for future predictions. Testing different algorithms can reveal which one offers the most reliable outcomes while addressing specific predictive needs.
Once models are in place, the next step is analyzing and scaling insights. This process involves interpreting the output generated from the predictive models to uncover meaningful patterns and trends. It is essential to transmute raw data into actionable findings that inform strategic decision-making. As businesses implement these insights, continuous monitoring and refinement are necessary to adapt to new behavioral data and evolving market conditions. By doing so, organizations can enhance their understanding and ultimately drive better business outcomes.
Step 4: Model Building and Testing
In Step 4: Model Building and Testing, the focus shifts toward creating reliable predictive models for understanding behavior patterns. This stage is crucial for making informed decisions based on the insights gathered from data. First, you will select appropriate algorithms that align with your objectives and the nature of your data. The choice of model significantly impacts the accuracy of predictive behavior analytics.
Next, you will train your model using a subset of your data. This process helps the algorithm learn and identify patterns within the dataset. After training, it's essential to test the model using a different data subset to check its effectiveness. Evaluating performance involves metrics such as accuracy, precision, recall, and F1 score. Depending on these results, you may need to refine or adjust your model before final implementation. By ensuring rigorous testing, you enhance the reliability of your predictive behavior analytics solutions.
Step 5: Analyzing and Scaling Insights
As you progress to Step 5, it's crucial to focus on analyzing and scaling insights derived from your predictive behavior analytics. Initiating this step involves aggregating data from various sources to identify trends and draw significant conclusions. By employing AI agents, you can automate data extraction, allowing for robust analysis that highlights key behavioral patterns within your customer base. This not only saves time but also enhances accuracy in recognizing pivotal trends that may influence future strategies.
The next stage focuses on scaling these insights effectively. Once you have a clear understanding of the data, the emphasis shifts to implementing actionable findings that can drive business decisions. Deploying visual dashboards and generating comprehensive reports helps convey insights to stakeholders, ensuring everyone is aligned on strategies. Additionally, constantly revisiting your analytics framework allows for real-time adjustments, amplifying the relevance and impact of your insights. To better understand your customers' evolving needs, prioritize feedback loops that fine-tune your predictive models consistently.
Conclusion: Harnessing the Power of Predictive Behavior Analytics
Predictive Behavior Analytics represents a transformative approach to understanding customer actions and intentions. By incorporating advanced AI agents, businesses can glean actionable insights from vast amounts of behavioral data. This ability to forecast future trends not only aids in refining marketing strategies but also enhances overall customer experiences.
As organizations strive for greater competitive advantage, the integration of predictive behavior analytics becomes essential. It empowers teams to anticipate needs, address pain points, and foster deeper customer relationships. Ultimately, harnessing these insights is about turning data into a powerful tool for driving informed decision-making and achieving meaningful business growth.