Advanced Approaches to Statistical Analysis of Questionnaire Data
-
Hello Insight
- 10 min read
Sophisticated Survey Analytics offers a powerful framework for extracting valuable insights from questionnaire data. As organizations strive to make informed decisions, understanding these advanced statistical techniques becomes essential. In today’s data-driven world, the ability to analyze survey results effectively can illuminate trends, highlight customer preferences, and drive strategic initiatives.
This section serves as an entry point into the complexities of survey analytics. By integrating methodologies such as multivariate analysis and structural equation modeling, researchers can uncover hidden relationships within data. Embracing these sophisticated approaches not only enhances the quality of insights but also ensures that organizations remain competitive in their respective fields.
Analyze & Evaluate Calls. At Scale.
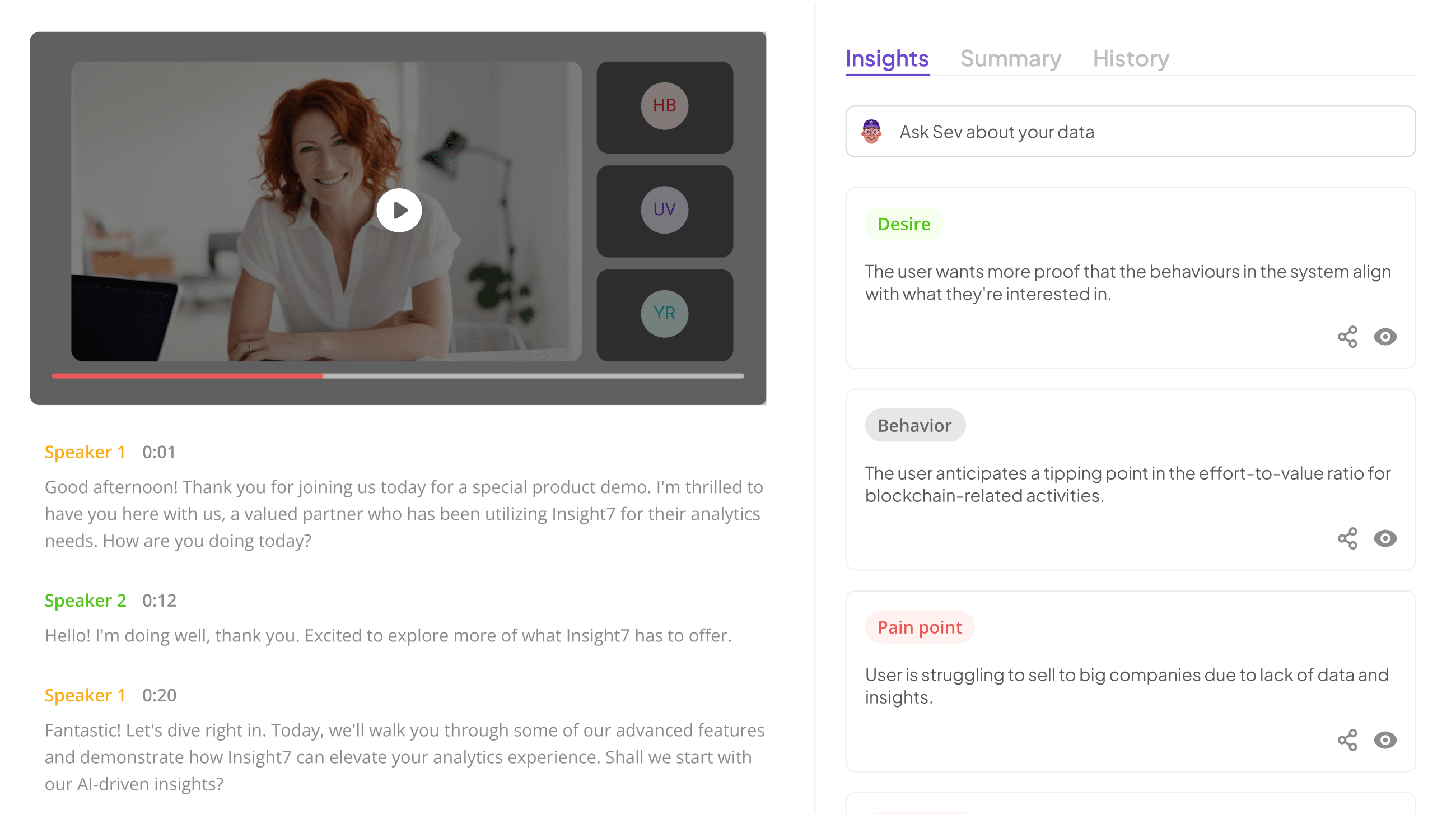
Fundamental Concepts in Sophisticated Survey Analytics
Sophisticated Survey Analytics forms the backbone of effective data-driven decision-making. Understanding fundamental concepts starts with grasping the significance of survey design and the nuances of statistical analysis. Analyzing questionnaire data requires a keen insight into how questions are framed and how responses are interpreted. Proper framing of survey questions can lead to actionable insights, ultimately influencing strategic business decisions.
Additionally, differentiating between qualitative and quantitative data is essential. Each type requires distinct analytical techniques and methods. For instance, qualitative data often needs thematic analysis, while quantitative data benefits from statistical tests. Understanding these variations allows researchers to choose the right tools and approaches, enhancing the overall quality of insights gained. Sophisticated Survey Analytics enables organizations to transform raw data into meaningful stories that drive engagement and operational effectiveness.
Importance of Statistical Analysis in Questionnaire Data
Statistical analysis plays a crucial role in transforming raw questionnaire data into meaningful insights. By employing sophisticated survey analytics, researchers can uncover trends and patterns that might otherwise remain hidden. This process not only informs decision-making but also enhances the overall quality of data interpretation. Statistical techniques allow for a deeper understanding of respondents' behaviors and preferences, providing organizations with vital knowledge that guides strategic planning.
Moreover, accurate statistical analysis helps address potential biases in the data. By applying advanced methods, researchers can refine their findings, ensuring that conclusions drawn from the data are both reliable and valid. This level of scrutiny is essential, especially in an era where data-driven decisions are paramount. Ultimately, the importance of statistical analysis cannot be overstated, as it empowers organizations to harness the full potential of their questionnaire data, leading to more effective outcomes.
Key Principles for Designing Effective Surveys
Designing effective surveys centers around a few key principles that can dramatically enhance data quality and relevance. Firstly, clarity in question formulation is essential. Survey questions should be straightforward, avoiding jargon and ambiguity to ensure participants fully understand their intent. This simplicity allows for more accurate responses, which is crucial for sophisticated survey analytics.
Secondly, consider the flow and structure of your survey. An intuitive order can guide respondents logically through the survey, encouraging completion and reducing dropout rates. Additionally, using a mix of closed and open-ended questions can yield rich qualitative insights alongside quantitative data. It's important to pre-test the survey, gathered feedback can help refine questions and identify potential biases, ultimately ensuring that the survey captures the intended insights effectively. By implementing these principles, organizations can better utilize their survey data, turning information into actionable strategies.
Advanced Techniques in Sophisticated Survey Analytics
Advanced techniques in sophisticated survey analytics play a pivotal role in extracting actionable insights from complex questionnaire data. By employing methods such as multivariate analysis and structural equation modeling (SEM), researchers can unlock deeper relationships between variables. This not only enhances the richness of the insights derived but also aids in understanding the underlying factors affecting survey responses.
When applying these advanced techniques, practitioners should first focus on understanding how different variables depend on one another. Following this, implementing factor analysis can help simplify data complexity by identifying underlying patterns. Subsequently, building and interpreting SEM models allows researchers to test theoretical frameworks and gauge their accuracy in representing real-world situations. Mastery of these advanced approaches empowers analysts to derive meaningful conclusions, establishing a competitive edge in data-driven decision-making. By embracing sophisticated survey analytics, organizations can convert insights into strategic actions, ultimately leading to improved outcomes and stakeholder satisfaction.
Extract insights from interviews, calls, surveys and reviews for insights in minutes
Multivariate Analysis Approaches
Multivariate analysis approaches serve as essential tools in sophisticated survey analytics, enabling researchers to unravel complex relationships within questionnaire data. These methods allow for the simultaneous examination of multiple variables, addressing various dependencies that exist in real-world scenarios. For instance, understanding how demographic factors influence consumer preferences can provide valuable insights for targeted marketing strategies.
Among the prominent techniques used are factor analysis and structural equation modeling (SEM). Factor analysis helps in identifying underlying factors that explain the variance in observed data, simplifying complex datasets into interpretable components. SEM, on the other hand, allows researchers to construct and test theoretical models containing observed and latent variables. By employing these advanced techniques, users can extract deeper insights from questionnaire data, enhancing decision-making processes and informing strategic initiatives. Understanding multivariate analysis is crucial for maximizing the potential of sophisticated survey analytics.
Step 1: Understanding Dependencies Between Variables
Understanding the dependencies between variables is crucial when engaged in sophisticated survey analytics. This step involves recognizing how various factors interact and influence one another, shaping the overall outcome of your questionnaire data analysis. Identifying these relationships helps researchers uncover meaningful patterns that reveal the underlying dynamics in the data, such as the correlation between customer satisfaction and product usage.
To illustrate this process, consider three key areas to focus on: 1. Correlation Analysis, which allows for the assessment of the strength and direction of the relationship between variables; 2. Dependency Analysis, helping to clarify how one variable may affect another; and 3. Causal Analysis, aimed at establishing direct cause-and-effect relationships. Each of these analyses contributes to a more comprehensive understanding of the complexities inherent in survey responses, ultimately guiding better decision-making and insights in the context of advanced statistical methods.
Step 2: Implementing Factor Analysis
To effectively implement factor analysis, one must begin by outlining the objective of the research. Understanding the underlying structure of data is crucial in sophisticated survey analytics, as it helps identify latent variables that can influence respondents' answers. The next step involves selecting the right variables for the analysis, ensuring they align with the overall research question.
Once the variables are chosen, researchers should proceed with data preparation, including normalization and handling missing values. This stage is vital as it directly impacts the results obtained from the factor analysis. After preparing the data, the application of factor extraction methods can reveal patterns and relationships among variables. Finally, interpreting the factor loadings provides insights into how individual items contribute to the identified factors, enabling researchers to draw informed conclusions and enhance decision-making processes.
Structural Equation Modeling (SEM)
Structural Equation Modeling (SEM) serves as a powerful technique for analyzing complex relationships in questionnaire data. This method allows researchers to simultaneously evaluate multiple linear equations, helping to uncover patterns and interdependencies within the data. Through SEM, researchers can validate theoretical constructs and explore causal relationships, making it a vital approach in sophisticated survey analytics.
To build and interpret a SEM model effectively, researchers typically follow a two-step process. First, they design a path diagram that illustrates how variables may interact with one another, allowing for visual insight into their relationships. Next, utilizing statistical software, they estimate the parameters in the model, leading to an interpretation of the results. Understanding these relationships enhances the potential for deriving meaningful insights from survey data, thereby advancing the field of statistical analysis.
Step 1: Building a SEM Model
Building a SEM model is a foundational step in sophisticated survey analytics. This process begins by defining your research questions and hypotheses. Clarifying these elements guides the selection of constructs and variables, allowing you to articulate the expected relationships among them. Essentially, you are constructing a visual representation of how variables interact, which serves as the backbone of your analysis.
Next, it's essential to gather data that aligns with your identified constructs and variables. This involves careful survey design, ensuring that each question effectively captures the intended information. As you compile the data, prepare for model specification, which focuses on determining the structural and measurement components of your SEM model. This step is critical as it influences how accurately your model reflects the underlying theory and captures the nuances of respondents' insights. By following these guidelines, you can develop a robust SEM framework that enhances your analysis of questionnaire data.
Step 2: Interpreting SEM Results
Interpreting Structural Equation Modeling (SEM) results is crucial for understanding the relationships within your questionnaire data. This step allows researchers to evaluate complex models that depict how various factors influence each other. By examining path coefficients, researchers can identify direct and indirect effects, enhancing their comprehension of potential correlations. Understanding these results can lead to actionable insights that drive decision-making.
💬 Questions about Advanced Approaches to Statistical Analysis of Questionnaire Data?
Our team typically responds within minutes
To effectively interpret SEM results, consider the following key aspects:
Model Fit: Assess indices such as RMSEA and CFI to evaluate how well the model represents the data.
Path Coefficients: Analyze the strengths and directions of relationships between variables to draw conclusions about their influence.
Significance Levels: Determine which relationships are statistically significant to focus on the most impactful variables.
Direct vs. Indirect Effects: Differentiate between these effects to understand how variables interact within the model.
By mastering these elements, you can better leverage sophisticated survey analytics to extract meaningful conclusions from your data. This enhances the overall effectiveness of your research and offers valuable insights for future endeavors.
Tools for Conducting Sophisticated Survey Analytics
When exploring sophisticated survey analytics, utilizing effective tools is integral to derive meaningful insights from questionnaire data. Various platforms and software can help streamline data analysis, making it easier to identify trends and significant patterns. One prominent tool is insight7, which simplifies the data analysis process while allowing users to handle extensive datasets with ease. This tool integrates AI capabilities, enhancing the overall efficiency of the analytical workflow.
Another valuable resource is R, an open-source language extensively used for statistical analysis. Equipped with a vast array of packages, R empowers users to conduct complex statistical computations seamlessly. Additionally, Python libraries such as Pandas and SciPy provide user-friendly environments where analysts can manipulate large datasets effectively. For traditional statistical tasks, SPSS remains a robust choice, favored for its intuitive interface and comprehensive features. Finally, employing QGIS is particularly beneficial for enhancing geospatial questionnaire data, adding another layer of depth to survey insights. By leveraging these tools, analysts can enhance their approach to sophisticated survey analytics, leading to actionable insights that inform strategic decision-making.
insight7: Gain Insights with Ease
In the realm of sophisticated survey analytics, gaining insights should feel seamless and effortless. The goal is to transform raw questionnaire data into actionable findings without requiring extensive statistical expertise. By utilizing user-friendly platforms, stakeholders can easily navigate through data, extract key themes, and identify crucial pain points. This democratizes access to insights, empowering everyone in an organization to contribute to informed decision-making.
To foster a smooth analysis experience, consider these crucial aspects for effective data utilization. First, ensure the platform offers visualizations for easy comprehension of results. Next, incorporate tools that analyze multiple data sources simultaneously, streamlining the insight extraction process. Finally, implement mechanisms that provide contextual evidence behind insights, ensuring transparency and reliability in decision-making. By focusing on these elements, organizations can leverage sophisticated survey analytics to unpack complex data and drive impactful business outcomes.
R: Comprehensive Statistical Computing
In the realm of sophisticated survey analytics, R stands out as a premier tool for comprehensive statistical computing. Its flexibility and extensive libraries empower analysts to perform intricate data manipulations and analyses, crucial for deriving meaningful insights from questionnaire data. R facilitates various statistical methods, from basic descriptive statistics to complex multivariate analyses. This is essential for understanding the relationships between variables and uncovering underlying patterns.
Utilizing R requires some familiarity with coding, yet its advantages are significant. Analysts can automate repetitive tasks, visualize data effectively, and employ advanced techniques like structural equation modeling. The combination of these capabilities allows users to handle large datasets efficiently and output clear, interpretable results that guide decision-making. As organizations continuously seek deeper insights into survey responses, the comprehensive statistical computing offered by R becomes indispensable for sophisticated survey analytics, driving impactful outcomes.
Python Libraries: Seamless Analytics with Pandas and SciPy
Python libraries, specifically Pandas and SciPy, bring forth a new era of seamless analytics for sophisticated survey analytics. These libraries facilitate the management and manipulation of large datasets effortlessly. With Pandas, users can conduct diverse data manipulations such as filtering, grouping, and merging, tailored to the needs of analyzing questionnaire data. It transforms raw data into structured datasets, making it easier to derive meaningful insights.
SciPy complements Pandas by enabling advanced statistical analysis and mathematical modeling. Users can perform complex computations, hypothesis testing, and even implement machine learning algorithms to identify patterns. Together, these libraries provide a powerful toolkit that enhances the analytical depth of survey data. By leveraging these capabilities, researchers can dive deeper into the nuances of their findings, ensuring robust conclusions that inform decision-making and strategy development. Through the lens of Python, sophisticated survey analytics is not just achievable; it becomes an efficient and insightful process.
SPSS: Powerful Tool for Statistical Analysis
SPSS stands out as a powerful tool for statistical analysis, particularly suitable for handling sophisticated survey analytics. Its intuitive interface and comprehensive functionalities allow researchers to analyze questionnaire data efficiently, diving deep into patterns and correlations. With SPSS, users can engage in multivariate analysis, providing insights that are often missed in simpler statistical methods.
One of the hallmark features of SPSS is its ability to handle large datasets seamlessly. This capability enables researchers to extract meaningful information swiftly while minimizing the risk of error. Furthermore, SPSS’s extensive array of statistical tests and visualization tools offers users the flexibility to tailor their analyses based on specific research needs. As a result, whether assessing consumer feedback or exploring demographic trends, SPSS remains an essential asset in the realm of advanced questionnaire data analysis.
QGIS: Enhance Geospatial Questionnaire Data
Incorporating geospatial data enhances the richness of questionnaire analysis, paving the way for sophisticated survey analytics. QGIS, a robust geographic information system, facilitates the visualization of survey data through maps, allowing researchers to uncover spatial patterns that may be missed with traditional methods. By plotting responses geographically, analysts can identify trends related to specific regions, leading to deeper insights into demographic-specific issues.
To effectively leverage QGIS for improving questionnaire data, consider the following pointers. First, data integration is crucial. Combine geospatial data with your survey results to create a unified dataset that reveals correlations between location and respondents' answers. Next, layer analysis allows for the incorporation of various data layers, such as socioeconomic information, which can enhance understanding. Finally, interactive mapping provides a more engaging approach, enabling stakeholders to explore data visually and draw meaningful conclusions. By embracing these techniques, QGIS not only enriches analytical capabilities but also contributes to a more nuanced understanding of survey findings.
Conclusion: Bringing It All Together in Sophisticated Survey Analytics
In concluding our exploration of sophisticated survey analytics, it's essential to recognize the interconnectedness of the methodologies discussed. Each analytical approach serves as a building block, enabling researchers to draw meaningful insights from complex questionnaire data. By employing techniques such as multivariate analysis and structural equation modeling, practitioners can unveil hidden relationships between variables, ultimately enhancing their understanding of respondents’ perspectives.
Moreover, the effective use of tools like R and Python empowers analysts to automate tasks, visualize results, and communicate findings succinctly. As we bring together these advanced techniques and tools, it becomes clear that sophisticated survey analytics is not merely about data collection, but about transforming raw data into actionable insights that influence decision-making.
💬 Questions about Advanced Approaches to Statistical Analysis of Questionnaire Data?
Our team typically responds within minutes