AI-driven text analysis is transforming how researchers approach text-based data. In an era where information is abundant, extracting meaning from massive datasets can be daunting. Traditional methods often fall short in efficiently sifting through nuances of language and context. Here, AI steps in, utilizing advanced algorithms to not only process vast volumes of text but also to identify patterns that might go unnoticed by human analysts.
By employing natural language processing technologies, researchers can enhance their ability to collect and interpret data. This shift allows for quicker insights, improving the overall quality of research outcomes. As AI-driven text analysis becomes more integrated within research practices, it offers unprecedented opportunities for innovation and discovery, paving the way for more effective methodologies in various fields.
Analyze qualitative data. At Scale.
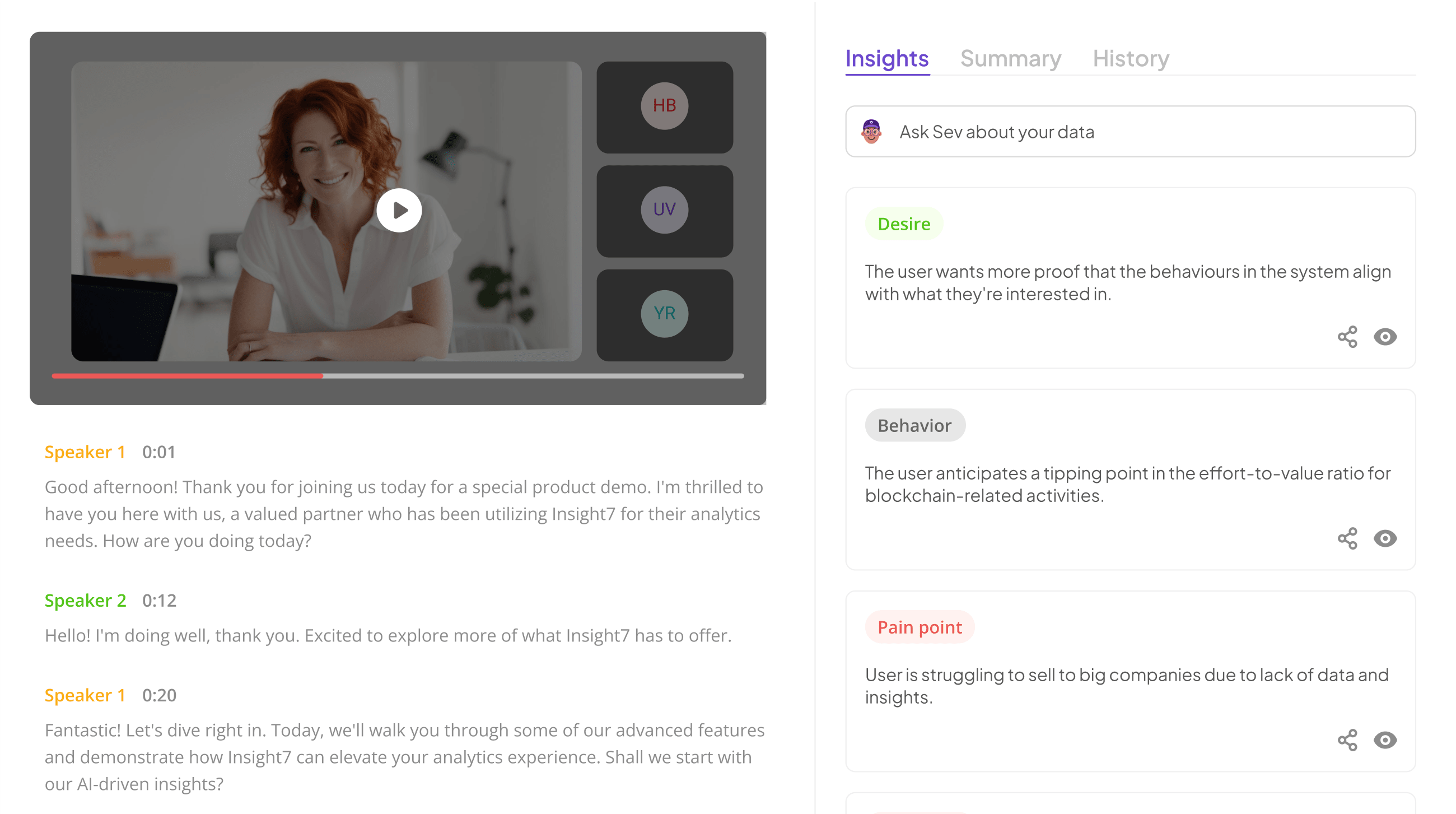
Transforming Traditional Research Methods with AI-Driven Text Analysis
Traditional research methods often struggle to keep pace with the increasing volume of data available today. AI-driven text analysis transforms these conventional approaches by automating the collection and processing of vast text-based datasets. This innovative technology employs natural language processing (NLP) to analyze and interpret text data, significantly enhancing efficiency and accuracy. Researchers can now quickly gather insights from interviews, surveys, and publications, which previously required lengthy manual efforts.
Moreover, AI-driven text analysis utilizes advanced machine learning algorithms to identify patterns and trends within the data. This capability accelerates the interpretation process, allowing researchers to draw actionable conclusions faster than ever before. As companies produce more customer signals, the capacity to process and analyze this information efficiently becomes crucial. Ultimately, integrating AI into research methodologies can lead to timely insights that empower organizations to maintain a competitive edge.
Enhancing Data Collection and Processing
AI-driven text analysis is fundamentally transforming how researchers collect and process data. First, AI technologies enable the gathering of vast amounts of text-based information from various sources, such as social media, surveys, and academic articles. This wealth of data offers unprecedented insights into consumer behavior and societal trends, allowing researchers to stay ahead of the curve.
Subsequently, natural language processing (NLP) plays a crucial role in streamlining data processing. By automating the analysis of language and context in large datasets, researchers can now interpret data more swiftly and accurately. This efficiency not only saves time but also improves the reliability of insights derived from textual data, leading to better-informed decision-making in various fields. Ultimately, leveraging these advanced techniques enables deeper understanding and more effective responses to the complexities inherent in todayโs data-rich environment.
- Adopting AI technologies for gathering vast volumes of text-based data.
Adopting AI technologies for gathering vast volumes of text-based data fundamentally changes how researchers access and utilize information. Traditional methods often fall short in handling the sheer volume of data available today. AI-driven text analysis streamlines data collection, enabling efficient extraction of relevant content from diverse sources, such as interviews, articles, and social media platforms.
Moreover, using advanced algorithms, AI tools can analyze data in real-time, pulling key themes and sentiment analysis from thousands of documents. This capability not only speeds up the research process but also enhances the accuracy of insights by highlighting significant trends. As organizations increasingly recognize the value of AI in text-based research, integrating these technologies becomes imperative to stay ahead in their respective fields. This transformation allows researchers to focus more on strategic decision-making rather than getting bogged down in data collection tasks.
- Streamlining data processing through natural language processing (NLP).
Natural language processing (NLP) fundamentally shifts how we approach text-based data analysis. Through AI-driven text analysis, researchers can now process overwhelming amounts of customer feedback and conversational data more efficiently. By automating the review of large datasets, NLP helps to significantly reduce the time spent on manual analysis, enabling insights to emerge rapidly from customer conversations.
One key benefit of NLP is its ability to identify trends and patterns that might go unnoticed in traditional approaches. This technology not only enhances accuracy but also ensures consistency in data interpretation. Furthermore, NLP tools facilitate collaboration among teams by centralizing insights in manageable formats, allowing for quicker decision-making processes. As organizations embrace these advancements, the potential for innovation and responsiveness grows, ultimately creating a competitive edge in the fast-paced world of market research.
Improving Textual Analysis and Interpretation
AI-driven text analysis is transforming how researchers interpret vast amounts of textual data. By harnessing machine learning algorithms, researchers can efficiently identify patterns and trends that might otherwise go unnoticed. This innovation enhances the accuracy of interpretations, enabling researchers to draw meaningful insights from large datasets more swiftly than traditional methods allow.
Moreover, AI technology significantly improves the overall process of textual analysis. The automation of data processing reduces the time spent manually sifting through information, freeing researchers to focus on strategic interpretation. As a result, AI-driven text analysis not only streamlines workflows but also allows for better collaboration, as insights can be easily shared and integrated across various platforms. This evolution thus represents a critical advancement in text-based research, empowering researchers to stay ahead in an increasingly data-driven world.
- Utilizing machine learning algorithms to identify patterns and trends.
Machine learning algorithms play a crucial role in AI-driven text analysis by identifying patterns and trends in vast datasets. These algorithms can analyze text from various sources, such as social media, customer reviews, and academic papers, to uncover insights that would be impossible to detect manually. By processing and categorizing information quickly, machine learning enables researchers to spot emerging trends, sentiment shifts, and customer preferences, significantly enhancing the quality and depth of analysis.
One significant advantage of utilizing these algorithms is their adaptability. As they learn from new data, they become more proficient at recognizing nuanced patterns and correlations. This continuous improvement enables researchers to stay ahead of evolving topics and sentiments in their fields. Moreover, by embracing AI-driven text analysis, researchers can focus on interpreting insights rather than spending excessive time cleaning and organizing data, ultimately leading to more informed decisions and innovative approaches in their respective domains.
- Increasing accuracy and efficiency in interpreting large datasets.
Interpreting large datasets can be a daunting task, but AI-driven text analysis is changing that significantly. By utilizing advanced algorithms, researchers can quickly process and analyze vast amounts of text data, allowing for timely insights that were previously difficult to obtain. The integration of natural language processing streamlines this process, enabling accurate data interpretation while reducing the risk of human error.
Moreover, these AI technologies empower researchers to uncover hidden patterns and trends within datasets, enhancing decision-making capabilities. For instance, within a matter of seconds, keywords and sentiments can be extracted from numerous documents, providing a comprehensive overview of the data landscape. By automating these processes, AI-driven text analysis not only increases the efficiency of research but also enhances its overall accuracy, enabling researchers to focus on deriving meaningful conclusions rather than getting lost in data volumes. This revolutionary approach is truly reshaping the landscape of text-based research.
Extract insights from interviews, calls, surveys and reviews for insights in minutes
Tools Revolutionizing AI-Driven Text Analysis in Research
The landscape of AI-driven text analysis is rapidly evolving, with various tools emerging as game-changers for researchers. These tools are designed to automate and enhance the way data is processed and analyzed, allowing researchers to focus on deriving meaningful insights. For instance, platforms like Insight7 streamline the analysis of customer interviews, making it easier to synthesize findings from large volumes of text.
Other notable tools include IBM Watson, which excels in predictive analytics, and Google Cloud Natural Language, known for its ability to perform syntax and sentiment analysis. OpenAI's GPT is particularly powerful in language generation, enabling researchers to summarize dense information efficiently. Additionally, MonkeyLearn's flexibility makes it suitable for various text analysis applications, while TextRazor specializes in entity extraction and keyword tagging. Collectively, these tools are revolutionizing how AI-driven text analysis is conducted in research, ensuring that professionals stay ahead in a data-driven world.
Insight7 and Other Leading Tools
AI-driven text analysis is transforming the ways researchers gather and interpret data. Insight7 offers an intuitive, self-service platform designed to streamline the analysis of customer conversations and interviews at scale. With this tool, organizations can efficiently synthesize valuable insights from vast amounts of unstructured data, significantly speeding up the decision-making process. In doing so, they can stay ahead of competitors who may still rely on outdated methods of analysis.
Other leading tools complement this AI-driven approach. IBM Watson enhances predictive analytics, helping researchers make informed forecasts. Google Cloud Natural Language excels in syntax and sentiment analysis, providing nuanced understanding. OpenAI's GPT offers advanced language generation capabilities that can summarize information effectively. Meanwhile, MonkeyLearn and TextRazor deliver flexible text analysis solutions, addressing diverse research needs through features like entity extraction and keyword tagging. Together, these tools are reshaping the landscape of text-based research, making insights more accessible than ever.
- Insight7: Highlights features and benefits for researchers.
AI-driven text analysis offers researchers a revolutionary way to streamline their workflows and deepen their insights. First, it enables the effortless organization and analysis of vast amounts of text-based data. With user-friendly interfaces, researchers can begin extracting valuable insights without prior expertise, democratizing access to data interpretation. For instance, by integrating features like transcription and visualization, researchers can uncover themes, behaviors, and pain points effectively.
Second, the ability to analyze multiple files or calls simultaneously enhances efficiency significantly. Researchers can analyze trends across datasets, allowing for a holistic view of the insights derived. These capabilities not only save time but also improve the accuracy of findings, ensuring researchers can make informed decisions based on robust data analysis. By embracing AI-driven text analysis, researchers can transform their methodologies into more agile, precise, and insightful practices.
AI-driven text analysis is fundamentally transforming how researchers approach their work. By employing sophisticated algorithms, this technology enhances the speed and effectiveness of analyzing vast amounts of textual data. As traditional methods often struggle to manage the growing influx of information, AI offers a powerful solution that increases both accuracy and efficiency in interpreting complex data.
One of the notable advantages of AI-driven text analysis lies in its ability to identify patterns and trends that might otherwise go unnoticed. This capability allows researchers to derive meaningful insights from large datasets more quickly than ever before. Additionally, leveraging natural language processing techniques streamlines the data collection process, making it easier to handle diverse types of text-based information. As a result, researchers can focus more on developing strategies grounded in these insights rather than being bogged down by processing tasks. This shift not only elevates the quality of research but also broadens the horizon for innovation in various fields.
Additional Tools for AI-Driven Research
AI-driven text analysis has opened up a plethora of tools that can enhance research capabilities and streamline processes. These additional tools, each with unique features, offer researchers the ability to analyze text data more effectively, ensuring that insights are accurate and actionable. For example, IBM Watson is notable for its predictive analytics, allowing researchers to forecast trends based on historical data.
Google Cloud Natural Language is another crucial tool, providing seamless syntax and sentiment analysis to uncover the emotional tone of the text. OpenAIโs GPT stands out with its robust language generation and summarization capabilities, which can transform lengthy documents into concise summaries. Moreover, MonkeyLearn offers customizable text analysis tailored to diverse research needs, while TextRazor excels in entity extraction and automatic keyword tagging, making data handling more efficient. By incorporating these tools into projects, researchers can utilize AI-driven text analysis to unlock deeper insights and enhance their overall research effectiveness.
- IBM Watson: Enhances predictive analytics capabilities.
As AI-driven text analysis continues to advance, its impact on predictive analytics is becoming more apparent. By employing sophisticated algorithms, the technology enables researchers to sift through massive datasets quickly, identifying patterns that inform future outcomes. This capability is particularly beneficial in fields requiring rapid decision-making based on user feedback and trends.
The power of AI-driven analytics lies in its ability to not only automate data processing but also enhance the accuracy of predictions. By integrating natural language processing, researchers can uncover subtle sentiments within data that traditional methods might overlook. The ability to analyze large volumes of text simultaneously allows for a comprehensive understanding of the underlying dynamics, making it an invaluable tool in any researcher's toolkit. By employing such advanced analytical techniques, organizations can optimize their strategies and enhance their data-driven decision-making processes significantly.
- Google Cloud Natural Language: Facilitates syntax and sentiment analysis.
AI-driven text analysis has become a cornerstone in understanding the nuances of language, including syntax and sentiment. By utilizing advanced capabilities of natural language processing, researchers can seamlessly analyze large datasets for unique insights. This method enables a more profound comprehension of how language feels, not just what it says, allowing for richer, more informed interpretations.
These tools provide two major functions. First, they facilitate syntax analysis, breaking down sentence structures to reveal grammatical relationships. This enhances clarity and precision in textual evaluation. Secondly, they offer sentiment analysis, assessing emotional tone and context within texts. As a result, researchers can better understand audience reactions and intentions. Such AI-driven text analysis not only enhances research accuracy but also empowers businesses and academics to make decisions rooted in nuanced comprehension.
- OpenAIs GPT: Offers powerful language generation and summarization.
OpenAI's language generation model has transformed the domain of text-based research by providing robust tools for AI-driven text analysis. With its powerful capabilities, researchers can efficiently generate coherent text and summarize vast amounts of information. This results in quicker insights and allows scholars to focus on higher-level analysis instead of being bogged down by manual data processing.
The model excels in taking complex subjects and distilling them down to their key points. For instance, when analyzing extensive literature or data sets, it can extract essential themes and insights rapidly. This capability not only enhances the accuracy of interpretations but also supports researchers in making informed decisions based on comprehensive narratives. As AI continues to evolve, harnessing such advanced language generation technologies will only further revolutionize the way text-based research is conducted, paving the way for innovative discoveries and informed strategies.
- MonkeyLearn: Provides flexible text analysis for diverse needs.
AI-driven text analysis is revolutionizing the way organizations approach data interpretation and insight generation. Flexible text analysis solutions empower teams to transform unstructured data into actionable insights without requiring extensive training or expertise. This democratization of data science enables anyone within a company to engage with the platform, streamlining the process of gaining meaningful insights into customer experiences or identifying friction points.
One of the key strengths of this approach is its adaptability to various needs. Users can import calls or documents and quickly visualize the conversations. The platform can unveil critical themes, pain points, and sentiments while providing accessible evidence from actual customer quotes. As AI-driven text analysis transforms traditional research methods, organizations are realizing the potential to extract deeper insights from their data, driving informed decisions that enhance overall performance and customer satisfaction.
- TextRazor: Delivers entity extraction and automatic keyword tagging.
The innovative role of AI-driven text analysis is reflected in the ability to perform entity extraction and automatic keyword tagging. By utilizing advanced algorithms, this process identifies relevant entities such as names, dates, and places within text data. This capability transforms extensive blocks of text into easily navigable information, enabling researchers to uncover vital insights efficiently.
Furthermore, automatic keyword tagging adds another layer of intelligence to this process. By tagging important themes and concepts, researchers can quickly locate and index relevant information across vast datasets. This significantly enhances the efficiency of textual analysis, allowing users to focus on critical insights rather than becoming overwhelmed by the sheer volume of data. As these tools evolve, they increasingly empower researchers to make data-driven decisions, marking a substantial step forward in the realm of AI-driven research methodologies.
Conclusion: The Future of AI-Driven Text Analysis in Research
AI-driven text analysis is set to redefine the future of research by enhancing our ability to sift through vast amounts of data with unparalleled efficiency. Continued advancements in natural language processing are likely to empower researchers, enabling them to uncover hidden insights and trends previously inaccessible. This transformation will not only streamline the research process but also enhance collaboration among scholars, allowing for more diverse perspectives and innovative findings.
As AI technologies improve, the potential applications for AI-driven text analysis will expand. From predictive analytics to sentiment evaluation, researchers will be equipped with powerful tools to engage with their data more dynamically. Ultimately, the integration of AI in text-based research promises a more informed approach to understanding complex topics, thereby driving qualitative advancements in various fields.