Data imputation techniques play a crucial role in quantitative research by addressing instances of missing data. When data is incomplete, it can skew results and undermine the integrity of research findings. Therefore, understanding various data imputation techniques is essential for researchers aiming to maintain the accuracy and reliability of their analyses.
In this section, we will explore the significance of data imputation and its different methodologies. Researchers can choose from various imputation strategies, such as mean substitution and regression imputation, to address missing values effectively. By implementing robust data imputation techniques, researchers not only enhance their study's credibility but also improve their overall research outcomes, making their findings more reliable and actionable.
Analyze qualitative data. At Scale.
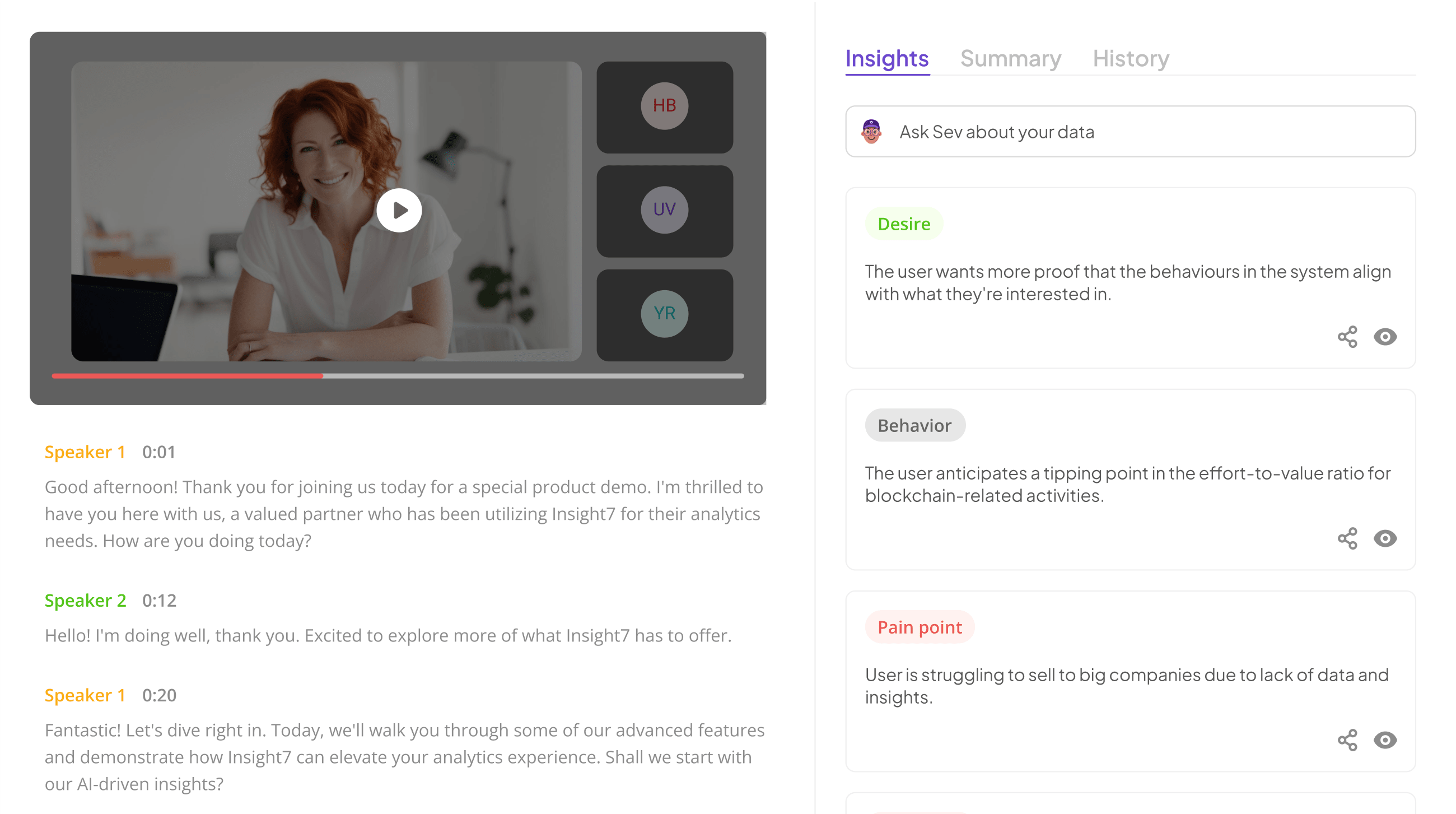
Understanding the Importance of Addressing Missing Data
Addressing missing data in quantitative research is crucial for the overall credibility of findings. Incomplete datasets can lead to biased results, affecting the conclusions drawn from research. Researchers must understand that inaccuracies caused by missing data can diminish the reliability of their analysis and ultimately misguide decision-making.
To effectively manage this issue, employing data imputation techniques is essential. These techniques provide methods for replacing or estimating missing values to ensure a more robust dataset. By doing so, researchers can conduct analyses that genuinely reflect their underlying populations. Furthermore, acknowledging the importance of addressing missing data fosters transparency in research practices, enhancing the trustworthiness of the outcomes.
In essence, understanding and addressing missing data not only improves the quality of research but also contributes to informed decision-making and policy formulation in various fields.
The Impact of Missing Data on Research Validity
Missing data presents a significant challenge in quantitative research, impacting the overall validity of study findings. When key information is absent, the conclusions drawn from the research can be misleading. Researchers may encounter biases that stem from systematically missing data, which can distort the results and raise doubts about their reliability. This uncertainty affects not only the immediate study but can also undermine future research efforts built on flawed data.
To mitigate these issues, data imputation techniques come into play. These methods allow researchers to estimate missing values based on available information, helping to maintain the integrity of the dataset. However, it is crucial to select the appropriate technique, such as mean substitution or multiple imputation, based on the data's characteristics and the reasons for the missingness. By carefully employing these strategies, researchers can minimize the adverse effects of missing data, thereby enhancing the validity and credibility of their research findings.
Common Causes of Missing Data in Quantitative Studies
Missing data in quantitative studies can arise from a variety of factors that researchers need to understand. One common cause is participant dropout, where individuals cease to participate due to personal issues or dissatisfaction with the study process. This not only skews results but can also lead to biased conclusions if not addressed properly.
Another significant factor is survey design flaws. Poorly designed questions or complicated response formats may lead to incomplete answers, as participants may abandon questions they find challenging. Additionally, data entry errors can occur during the transcription of survey results, further contributing to missing data. Recognizing these causes is essential for implementing effective data imputation techniques that help maintain the integrity of research findings. Understanding the nature of missing data also enables researchers to choose appropriate methods for dealing with these gaps, ensuring more reliable and valid results in their studies.
Extract insights from interviews, calls, surveys and reviews for insights in minutes
Exploring Various Data Imputation Techniques
Data imputation techniques serve as essential methods in quantitative research for addressing missing data effectively. One of the most widely used strategies is single imputation, which includes techniques such as mean substitution and regression imputation. Mean substitution involves replacing missing values with the average of the available data, preserving overall data integrity. On the other hand, regression imputation predicts missing values based on relationships observed within the dataset, thus providing a more nuanced approach to filling gaps.
Another critical area is multiple imputation techniques, which enhance the robustness of the data analysis. This process involves creating several complete datasets by simulating missing values based on statistical models. Its implementation can be streamlined through specialized software, allowing researchers to gain more reliable insights. Understanding and applying these data imputation techniques not only helps maintain the validity of the research findings but also furthers a deeper comprehension of the dataset as a whole.
Single Imputation Methods
Single imputation methods serve as a foundational approach in data imputation techniques, focusing on replacing missing values with a single, estimated value. These methods are crucial for maintaining the integrity and usability of datasets in quantitative research. The most common single imputation methods include mean substitution and regression imputation.
Mean substitution involves replacing missing values with the overall mean of the observed cases. This technique is straightforward but may distort the data distribution, especially if many values are missing. On the other hand, regression imputation predicts missing values based on other variables in the dataset. This method utilizes relationships within the data to provide a more accurate estimate, though it assumes that intrinsic correlations exist.
While convenient, single imputation methods also have limitations, as they do not account for variability in the data. Researchers should weigh these methods against their specific research context to choose the most suitable approach.
Mean Substitution
Mean substitution is one of the simplest and most commonly used methods in data imputation techniques for handling missing data. This approach involves replacing any missing values in a dataset with the mean of the available data points for that variable. While it seems straightforward, mean substitution can introduce bias and reduce variability in the data, potentially affecting the overall analysis.
To effectively apply mean substitution, consider the following steps:
- Calculate the Mean: First, compute the mean of the existing data for the variable where values are lacking.
- Substitute Missing Values: Replace each missing entry with the calculated mean, ensuring the dataset remains complete.
- Assess the Impact: It is vital to analyze how this substitution affects the overall dataset, including standard deviation and correlations, to maintain the integrity of your results.
By recognizing the strengths and limitations of mean substitution within data imputation techniques, researchers can decide when it is appropriate to use this method and understand its implications on research outcomes.
Regression Imputation
Regression imputation stands out as a powerful method in the realm of data imputation techniques. By leveraging relationships between variables, this technique estimates missing values through predicted values derived from regression models. In essence, it utilizes existing data to provide a more informed estimate, making it a preferred choice for researchers aiming to maintain the integrity of their datasets.
The process begins with a regression analysis, where the variable with missing values serves as the dependent variable. Researchers select independent variables that are related to the missing data based on their statistical significance. Once the regression equation is developed, it can then predict the missing values effectively. This method tends to outperform simpler techniques like mean substitution, especially in datasets where the relationships among variables are strong. However, itโs crucial to recognize that improper model specifications can lead to biased imputations, ultimately affecting the quality of analysis.
Multiple Imputation Techniques
Multiple imputation techniques offer a robust approach for addressing missing data in quantitative research. This method aims to create several plausible datasets by replacing missing values with estimates derived from the observed data. Each imputed dataset allows researchers to analyze the data as if there were no missing values, which enhances the reliability of their results.
The process begins with generating multiple copies of the dataset, each containing different imputed values. Analysts then perform the desired statistical analyses on each dataset and combine the results to produce overall estimates. This combination reflects the uncertainty associated with the missing data more accurately than single imputation methods. Ultimately, using multiple imputation techniques helps maintain the statistical power of research while fostering a more comprehensive understanding of missing data's impact on findings.
Overview of the Multiple Imputation Process
Multiple imputation is a sophisticated method used in data imputation techniques to handle missing data effectively. The process creates multiple complete datasets by filling in missing values through predictive modeling. This approach acknowledges the uncertainty inherent in missing data by providing a range of plausible values for each missing entry, instead of relying solely on mean or median fill-ins.
The multiple imputation process typically involves three main steps: the imputation of missing values, the analysis of each completed dataset, and the pooling of results into a final output. Initially, a statistical model predicts missing values while taking into account the existing data. Once several imputed datasets are generated, researchers analyze each one separately. Lastly, the results are combined, producing estimates that reflect both the uncertainty of the imputed values and the inherent variability of the collected data. By applying multiple imputation, researchers not only maintain the integrity of their analyses but also enhance the reliability of their findings in quantitative research.
Implementing Using Specialized Software
Implementing specialized software for missing data management can significantly improve research outcomes. Many researchers utilize Data Imputation Techniques to fill gaps in their datasets efficiently. Various software solutions are designed to automate this process, minimizing human error and enhancing accuracy. These tools can streamline the imputation process, applying sophisticated algorithms that handle missing values effectively.
Key functionalities of specialized software include the ability to conduct mean substitution, regression imputation, and more advanced multiple imputation techniques. Each method has its own strengths, and the choice of which to implement depends on the specific needs of your study. By leveraging these tools, researchers can maintain data integrity and derive reliable insights from their quantitative analyses. Embracing technology not only simplifies the handling of missing data but also empowers researchers to focus on deriving meaningful conclusions from their work.
Tools for Effective Data Imputation
Selecting the right tools for effective data imputation is crucial for maintaining the integrity of quantitative research. Various software options can streamline this process, enhancing accuracy and efficiency. One powerful tool is R Statistical Software, which offers extensive packages for handling missing data. Similarly, Python libraries such as Pandas and Scikit-learn provide advanced methods tailored for diverse imputation techniques.
IBM SPSS is widely recognized for its user-friendly interface, making it accessible to researchers of varying skill levels. Stata is another effective option, especially favored in fields that require complex statistical analyses. Each of these tools enables researchers to handle missing data with confidence, ensuring that their findings remain robust and reliable. By utilizing these various data imputation techniques, researchers can effectively turn incomplete datasets into valuable insights, ultimately strengthening their research outcomes.
insight7
Data imputation techniques serve as essential tools for addressing missing data in quantitative research. By utilizing these techniques, researchers can enhance the integrity of their datasets, leading to more accurate and reliable findings. The two primary categories are single imputation methods and multiple imputation techniques, each with its own strengths and applications.
Single imputation methods, such as mean substitution and regression imputation, offer straightforward ways to fill gaps in datasets. Mean substitution involves replacing missing values with the average of observed data, while regression imputation predicts missing values based on relationships with other variables. However, these approaches may oversimplify data variability. On the other hand, multiple imputation techniques involve creating several plausible datasets, filling in missing values based on statistical models, and analyzing them separately before combining results. This approach accounts for uncertainty around missing data, offering more robust conclusions. By understanding and applying these data imputation techniques, researchers can better navigate challenges posed by missing data and significantly enhance their research's validity.
IBM SPSS
Data imputation techniques are essential for handling missing data, and specific software can significantly enhance this process. One such tool provides various options for anticipating and compensating for missing values in datasets. Users can navigate to imputation functions easily, offering flexibility in selecting a technique that fits their data needs.
Within this software, researchers can apply methods such as mean substitution and regression imputation. These techniques allow for the replacement of missing values based on existing data. You can also perform multiple imputation, which generates several possible datasets, reflecting uncertainty about the missing values more accurately. This comprehensive approach ensures researchers maintain the integrity of their quantitative results while addressing issues of missing data effectively.
Ultimately, utilizing these data imputation techniques significantly enhances the reliability of quantitative research findings. By incorporating software capabilities, researchers are better equipped to handle the complexities of missing data.
R Statistical Software
R Statistical Software is a powerful tool for researchers dealing with missing data in quantitative studies. Among various analytical applications, it offers robust data imputation techniques that cater to different datasets and research designs. Rโs versatility lies in its extensive library of packages, making it an ideal choice for handling missing data efficiently.
One popular method in R is the use of Multiple Imputation Techniques, which provide a systematic approach to deal with gaps in data. Through packages like mice
and missForest
, researchers can perform comprehensive analyses that create several imputed datasets. This approach not only enhances the accuracy of statistical estimates but also accounts for the uncertainty inherent in missing data. R's user-friendly syntax enables researchers to easily employ these techniques without extensive programming knowledge, thereby easing the transition into more advanced statistical methodologies. By leveraging R, researchers can ensure that their findings remain valid and reliable amidst the challenges posed by incomplete datasets.
Python Libraries (Pandas, Scikit-learn)
Python provides powerful libraries, notably Pandas and Scikit-learn, which offer essential tools for handling missing data. These libraries help streamline data imputation techniques, ensuring that analyses remain robust and reliable. Pandas excels in data manipulation, offering functions to fill or drop missing values effortlessly. With methods such as fillna()
, users can quickly replace NaN values with specified statistics, like the mean or median, thus facilitating smoother data workflows.
Scikit-learn complements Pandas by providing sophisticated imputation strategies. Through its SimpleImputer
and KNNImputer
, users can implement both basic and advanced data imputation techniques seamlessly. SimpleImputer enables straightforward approaches such as mean or mode imputation, while KNNImputer employs more complex methods using the nearest neighbors for filling gaps. Together, these libraries not only enhance data quality but also contribute to the integrity of quantitative research outcomes.
Stata
Stata is a powerful statistical software widely used in quantitative research, particularly for handling missing data effectively. One of its key features is the ability to implement various data imputation techniques. Organizations can leverage Stata to conduct imputations using methods such as mean substitution or regression imputation, ensuring that their analyses remain valid and reliable despite missing entries.
Within Stata, users can easily execute multiple imputation techniques, which offer a more sophisticated approach to addressing missing data. This process involves generating multiple complete datasets, analyzing them individually, and then pooling results to account for uncertainty. By employing these data imputation techniques, researchers can enhance the integrity of their findings and draw more accurate conclusions from their data. Overall, Stata serves as an essential tool for researchers aiming to navigate the complexities of missing data in quantitative studies.
Conclusion: The Role of Data Imputation Techniques in Enhancing Research Integrity
Data imputation techniques play a crucial role in enhancing the integrity of quantitative research. By addressing the issue of missing data, researchers can ensure their findings are both reliable and valid. Proper imputation methods fill gaps in datasets, preventing biases that could distort conclusions. This not only strengthens the research outcomes but also upholds the credibility of the entire research process.
Moreover, employing robust imputation techniques fosters trust in quantitative studies. It signals to stakeholders that researchers are committed to maintaining high standards of data quality and integrity. Consequently, effective imputation not only refines analyses but also encourages responsible use of data in decision-making processes.