In today's data-driven world, researchers often grapple with the delicate Privacy-Personalization Balance. This balance is essential as it determines how personalized services are delivered while safeguarding individual privacy. Striking a harmonious relationship between these two aspects can significantly enhance user experience, fostering trust and engagement in data research.
Understanding how to navigate this balance is crucial for various stakeholders, including researchers, businesses, and consumers. By prioritizing privacy while implementing effective personalization strategies, researchers can generate valuable insights while respecting the privacy rights of individuals. This section will delve into the complexities of achieving this equilibrium, laying the groundwork for a responsible approach towards data usage in research.
Analyze qualitative data. At Scale.
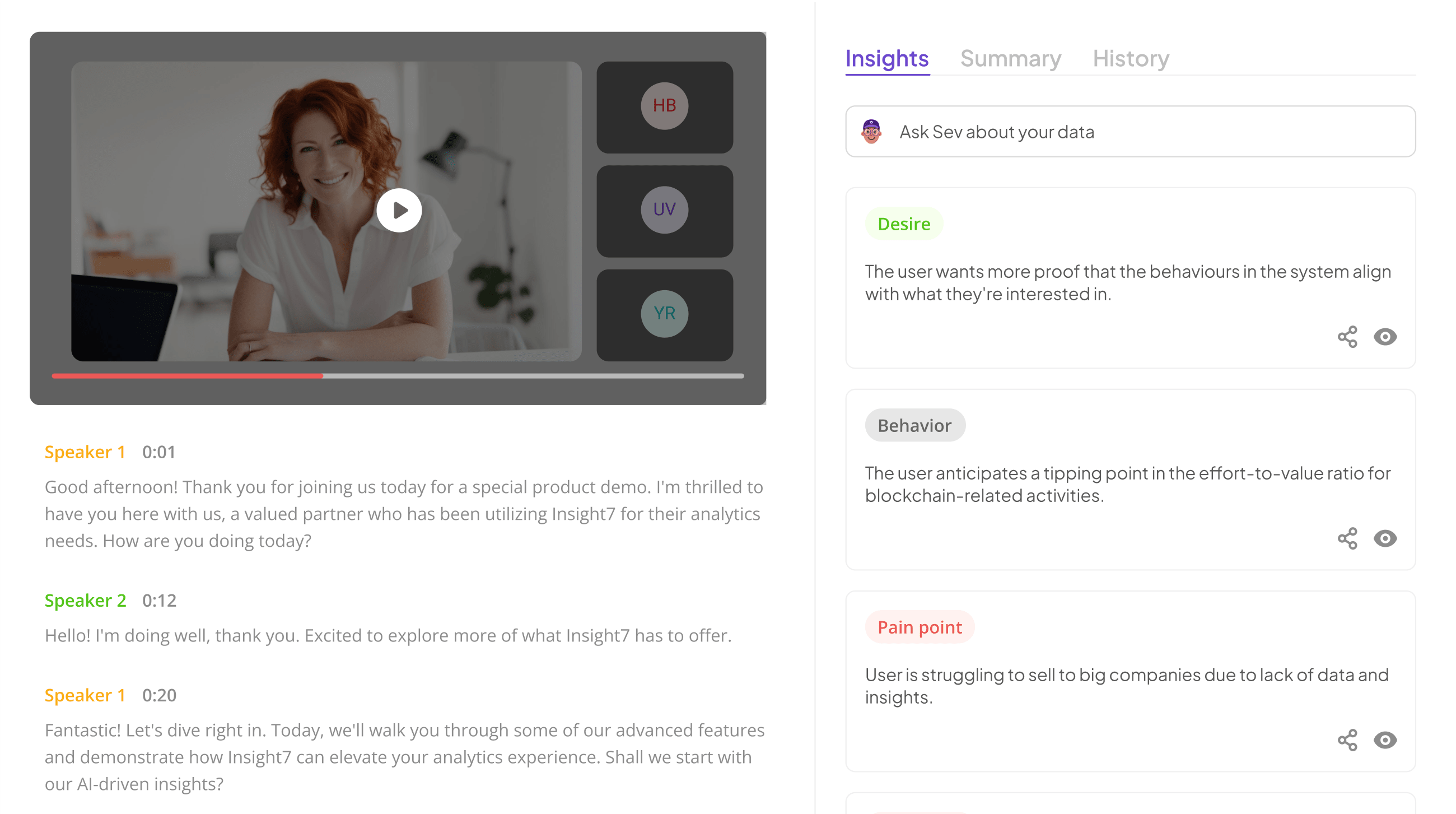
Exploring the Privacy-Personalization Balance
The Privacy-Personalization Balance is a critical aspect of today's data-driven environment. As organizations strive to deliver tailored experiences, they must also navigate the complexities of user privacy. This balancing act requires a nuanced understanding of both personalization strategies and the essential safeguards that protect user data.
To explore this balance effectively, it's vital to examine key components. First, personalization enhances user engagement by utilizing data to tailor services to individual needs. However, this practice often raises concerns about data misuse and privacy infringements. Organizations must prioritize ethical standards in data handling while striving for effective personalization. Transparency in data collection and a commitment to user privacy not only build trust but also pave the way for innovative and secure data practices. By recognizing the significance of the Privacy-Personalization Balance, organizations can achieve both user satisfaction and adherence to privacy standards.
Understanding Personalized Data
Understanding personalized data is essential for striking the right balance between privacy and personalization. Personalized data refers to the tailored information derived from individuals' preferences and behaviors, enabling organizations to deliver customized experiences. This can enhance user interactions by making them more relevant and engaging. For instance, e-commerce platforms analyze browsing behaviors to recommend products that align with a userโs interests, ultimately improving satisfaction and sales.
However, this approach raises significant privacy concerns. As organizations collect vast amounts of personal data, maintaining user trust becomes crucial. The Privacy-Personalization Balance is pivotal in ensuring that while companies create personalized experiences, they also safeguard individuals' privacy rights. Adopting ethical research practices, such as obtaining informed consent and minimizing data usage, can aid in achieving this balance. By implementing robust privacy measures, companies can foster an environment where innovation thrives alongside respect for personal data.
- Definition and examples of personalization in data-driven research.
Personalization in data-driven research refers to the tailored experiences created by analyzing user data to cater to individual preferences. This often involves collecting insights based on behaviors, demographics, and feedback, allowing organizations to deliver targeted content and services. For instance, a retail brand might analyze previous purchase patterns to suggest products that align with a customerโs unique tastes, demonstrating how tailored experiences can drive engagement.
However, achieving the right Privacy-Personalization Balance is crucial. The benefits of personalized data must be weighed against privacy concerns, where individuals may feel uncomfortable with their data being used extensively. Examples of effective personalization can be drawn from various sectors, including educational platforms offering customized learning paths and media streaming services curating recommendations based on viewing habits. These applications illustrate the potential of personalization, yet they must be pursued responsibly to ensure user trust and safety.
- Benefits and applications across different industries.
Personalization offers significant advantages across various industries, fundamentally changing how businesses interact with their customers. In retail, personalized marketing strategies enable companies to tailor recommendations based on individual preferences, enhancing user experience and boosting sales. The healthcare sector benefits from personalized treatment plans, which cater to the specific needs of individual patients, leading to improved health outcomes.
However, navigating this personalization journey requires a conscious approach to the Privacy-Personalization Balance. In the financial services industry, for example, companies can offer customized financial products while ensuring that sensitive customer data is protected. Additionally, personalizing educational content helps deliver targeted learning experiences for students, yet this must be done without compromising privacy. By adopting a thoughtful approach to personalization, organizations can maximize their benefits while respecting the essential aspects of data privacy.
Privacy Concerns in Data Usage
In the realm of data usage, understanding privacy concerns is essential for safeguarding individual rights. Personal data collection often includes sensitive information such as social security numbers and birth dates. Users frequently underestimate the potential risks associated with sharing their data, which can lead to unauthorized access and breaches. Consequently, maintaining a balance between privacy and personalization becomes a critical challenge for researchers and organizations.
To navigate this Privacy-Personalization Balance, it is crucial to implement effective strategies. First, organizations must prioritize transparency by informing users about what data is collected and how it will be used. Secondly, anonymization techniques can help minimize risks associated with personal data. By adopting these measures, organizations can create a more secure environment that respects individual privacy while still enabling beneficial personalization. This careful approach ensures that data-driven research can thrive without compromising the trust of the individuals it serves.
- Types of personal data typically collected.
Personal data collection is integral to data-driven research, as it enables personalization and tailored experiences. Common types of personal data collected include identifying details such as names, email addresses, and phone numbers. Additionally, demographic information, including age, gender, and location, often plays a crucial role in understanding user preferences and behaviors.
Beyond identifiable data, researchers often gather sensitive information like financial records, social security numbers, and personal interests. This data can optimize experiences but raises significant privacy concerns. The challenge lies in achieving a Privacy-Personalization Balanceโensuring that beneficial personalization does not compromise individual privacy. Establishing guidelines for ethical data collection and utilization is essential in mitigating risks while enhancing user experience. By focusing on responsible practices, researchers can respect user privacy and still harness the power of personalized insights.
- Risks associated with data sharing and breaches.
In the realm of data sharing, a critical challenge lies in striking a balance between personalization and privacy. Organizations often harness personal data to tailor experiences, but this can expose sensitive information to potential breaches. The risks associated with data sharing are multifaceted, including unauthorized access, identity theft, and reputational damage. These concerns necessitate robust security measures to protect personal information while still enabling personalized services.
Furthermore, data breaches can lead to significant legal repercussions and financial losses. When organizations fail to safeguard user data, trust diminishes, affecting user engagement and loyalty. Transparency about data use is essential, allowing individuals to understand how their information is utilized. By prioritizing this Privacy-Personalization Balance, organizations can foster trust while delivering tailored experiences, ultimately enhancing their relationship with consumers.
Strategies for Maintaining the Privacy-Personalization Balance
Balancing privacy and personalization in data-driven research is crucial for fostering trust and collaboration. One effective strategy involves implementing ethical research practices. Researchers should prioritize informed consent and transparency, ensuring participants understand how their data will be used. Clear communication not only builds trust but also empowers individuals to make informed decisions regarding their personal information.
Another vital approach is employing privacy-preserving technologies. Technologies such as differential privacy or data anonymization can help protect sensitive information while still enabling valuable insights. By integrating these techniques into research processes, organizations can mitigate risks associated with data breaches while maximizing the utility of personalized data. Ultimately, maintaining the privacy-personalization balance requires a thoughtful combination of ethical practices and innovative technologies, ensuring that both individual rights and data-driven insights are upheld.
Extract insights from interviews, calls, surveys and reviews for insights in minutes
Implementing Ethical Research Practices
Implementing ethical research practices is crucial for navigating the privacy-personalization balance in data-driven research. The first step involves securing informed consent and maintaining transparency throughout the research process. Participants should clearly understand what data is being collected, how it will be used, and their rights regarding that data. This fosters trust and encourages participants to engage more openly, ensuring that their contributions are meaningful and impactful.
Next, employing anonymization and data minimization techniques is vital. Researchers should only collect the information necessary for their study, which helps reduce the risks associated with data misuse. Anonymization techniques ensure that individual identities remain protected, even if the data is utilized for personalized insights. By thoughtfully applying these ethical practices, researchers can effectively strike a balance between personalization benefits and the need for privacy, ultimately enhancing the integrity and reliability of their findings.
- Step 1: Informed consent and transparency.
Effective informed consent is the cornerstone of ethical data-driven research. It ensures that participants are fully aware of how their data will be used, which leads to a trust-based relationship. Transparency in data practices empowers individuals by allowing them to make informed decisions about their participation. Without this crucial step, the delicate Privacy-Personalization Balance is jeopardized, risking the prospect of data misuse or breaches.
To facilitate informed consent, researchers can adopt specific practices. First, clearly articulate the purpose of data collection, explaining how personalized experiences will enhance user engagement and service offerings. Second, provide details on data handling procedures, including storage, access, and sharing protocols. Finally, ensure participants can opt out at any stage without repercussions. This multi-faceted approach fosters trust and respects users' privacy, making informed consent an essential element of ethical research practices.
- Step 2: Anonymization and data minimization techniques.
Anonymization and data minimization techniques are essential for achieving a delicate Privacy-Personalization Balance in data-driven research. Anonymization involves removing any identifiable information from datasets, making it impossible to trace data back to an individual. This method greatly reduces privacy risks, allowing researchers to analyze trends without compromising user identities. It involves techniques such as data masking and pseudonymization, which help protect personal details while still yielding valuable insights.
Data minimization complements anonymization by ensuring that only the necessary data for research purposes is collected. This means gathering the least amount of personal data needed to support specific objectives. By prioritizing essential data, researchers can mitigate privacy concerns and reinforce consumer trust, thereby fostering a more favorable environment for personalization efforts. Together, these techniques allow organizations to explore consumer preferences effectively while safeguarding individual privacy rights.
Utilizing Privacy-Preserving Technologies
The integration of privacy-preserving technologies plays a critical role in achieving the privacy-personalization balance. It enables researchers to utilize valuable data while ensuring that individual privacy is respected. These technologies include techniques such as differential privacy, federation learning, and secure multi-party computation, which allow for data analysis without compromising personal information.
To effectively implement these privacy-preserving technologies, researchers should follow key steps. First, they should assess the specific data needs while identifying the risks involved. Next, researchers can adopt anonymization techniques to eliminate identifiable information. Finally, regular audits and updates of technologies used are essential for maintaining compliance with privacy standards and regulations. By correctly utilizing these tools, researchers can create systems that enhance personalization without sacrificing user privacy. Balancing these aspects ultimately fosters trust and promotes responsible data-driven research practices.
- Overview of privacy-preserving technologies.
Privacy-preserving technologies play a crucial role in achieving the privacy-personalization balance. These technologies enable researchers to utilize personal data while protecting usersโ sensitive information. Techniques such as differential privacy and federated learning ensure that data remains secure, allowing for personalized insights without compromising individual privacy.
One of the key methods, differential privacy, adds random noise to datasets, making it difficult to identify individual contributors while still allowing for meaningful aggregate analysis. In contrast, federated learning allows algorithms to learn from decentralized data sources without ever collecting the data itself, thus safeguarding privacy. By integrating these technologies, organizations can pursue data-driven research ethically and responsibly, fostering trust while unlocking the benefits of personalization. Achieving this balance is essential in a world increasingly aware of privacy issues.
- Steps for integrating these technologies into research.
Integrating privacy-preserving technologies into research is essential for achieving the Privacy-Personalization Balance. First, researchers should conduct a needs assessment to identify which data personalizations are necessary for their objectives. This step clarifies the specific areas where personalization can enhance insights while ensuring that privacy concerns are addressed.
Next, implementing privacy-enhancing techniques such as data anonymization and encryption plays a critical role. Employing these methods helps safeguard participants' information and meets ethical standards. Additionally, leveraging consent management tools ensures that individuals are informed and comfortable with how their data is used. Finally, continuous monitoring and feedback loops after the initial integration allow researchers to adjust practices based on user feedback, ultimately fostering trust and adherence to ethical standards. This systematic approach aids in harmonizing the dual goals of personalization and privacy in data-driven research.
Tools for Achieving the Privacy-Personalization Balance
Achieving the Privacy-Personalization Balance requires an array of tools designed to manage data responsibly while enhancing user experiences. First, implementing data management platforms can streamline the data collection process, ensuring compliance with regulations while offering personalized insights. These platforms often include features for tracking user preferences, which can enhance personalization without compromising privacy.
Second, integrating privacy-preserving technologies, such as encryption and anonymization, can significantly bolster user trust. These technologies work by safeguarding individual data points while still providing valuable insights for research. Lastly, thorough training in ethical research practices empowers teams to prioritize transparency and informed consent, which are vital in maintaining user confidence.
By combining these tools, organizations can effectively navigate the complex landscape of personalization and privacy. This balanced approach leads to more ethical and impactful research outcomes, satisfying both user needs and regulatory standards.
insight7
In todayโs data-driven research environment, achieving a Privacy-Personalization Balance is essential. Organizations collect vast amounts of personal data to enhance user experiences and tailor services. However, this collection raises significant privacy concerns, often leading to distrust among users. Therefore, finding a way to effectively manage personalization without compromising individual privacy is crucial.
To strike this balance, researchers must adopt ethical practices. First, ensuring informed consent and transparency helps build trust with participants. They must know exactly what data is being collected and how it will be used. Additionally, employing anonymization and data minimization techniques can further protect user privacy while still allowing for valuable insights to be gleaned from the data. By implementing these strategies, organizations can not only foster a sense of security among users but also enhance the effectiveness of their personalization efforts. This dual approach is vital for the credibility and success of data-driven research.
- Key features and how it helps balance privacy and personalization.
Key features of effective data management tools play a crucial role in achieving a Privacy-Personalization Balance. These tools incorporate advanced technologies that ensure data collection respects user privacy while still delivering personalized experiences. One key feature is the implementation of anonymization processes, which protects individual identities while allowing organizations to derive meaningful insights. This method allows companies to analyze trends and preferences without compromising personal information.
Another essential feature is user-centered data practices. By prioritizing informed consent and transparency, organizations can ensure that users understand how their data will be utilized. This builds trust and encourages collaboration between users and service providers. Moreover, real-time data analytics capabilities allow for the dynamic adjustment of personalization strategies, aligning closely with user preferences while maintaining stringent privacy standards. Overall, these features contribute to a sustainable approach in balancing privacy and personalization effectively.
Additional Data Management Tools
In the quest for a Privacy-Personalization Balance, several additional data management tools can streamline research processes. These tools serve to enhance data organization while ensuring user privacy is upheld. Each tool uniquely contributes to managing and analyzing data more efficiently, allowing researchers to make informed decisions without compromising consumer trust.
Data Visualization Tools: These platforms transform complex datasets into understandable visuals. By highlighting trends and insights, they enable researchers to derive meaningful conclusions while minimizing privacy risks through aggregated data views.
Data Anonymization Software: This tool ensures that any identifiable information is removed from datasets. It allows researchers to analyze broad patterns without exposing individual privacy, preserving the integrity of the research process.
Consent Management Systems: These systems manage user consent into data usage, maintaining transparency. By empowering users, they help build trust and promote responsible data handling in personalized research efforts.
Adopting these tools fosters a responsible research environment that prioritizes user privacy without sacrificing personalization.
- Tool 1: Comprehensive features and benefits.
The initial exploration of the Privacy-Personalization Balance reveals various tools designed to enhance data-driven research. Tool 1 comprises comprehensive features that prioritize user empowerment while maintaining strict privacy standards. First, the tool offers robust data encryption methods, ensuring that sensitive information remains secure against unauthorized access. This feature allows researchers to personalize user experiences without compromising data integrity.
Additionally, the tool provides insightful analytics dashboards, facilitating the extraction of actionable insights from user data. Researchers can segment data without revealing personal identifiers, thereby respecting individual privacy preferences. Furthermore, the integration of customizable privacy settings empowers users to control how their data is utilized. This balance of personalization and data security not only enhances user trust but also fosters a more responsible approach to research. Ultimately, Tool 1 exemplifies a thoughtful design that acknowledges the critical importance of maintaining a Privacy-Personalization Balance in todayโs data landscape.
- Tool 2: Overview and use cases.
Data management tools play a vital role in navigating the complex landscape of the Privacy-Personalization Balance. The second tool offers a range of features that enable users to efficiently analyze and report data while preserving individual privacy. It is user-friendly, requiring no advanced technical skills, allowing everyone in an organization to access insights quickly. This accessibility democratizes data usage and fosters a culture of informed decision-making.
Case studies illustrate its diverse applications. For instance, researchers can analyze customer interviews to identify pain points while protecting sensitive information. Additionally, businesses can streamline reporting by generating summaries and extracting relevant insights from large datasets. Such use cases showcase how this tool contributes to balancing personalization and privacy, empowering organizations to harness data without compromising ethical standards. By leveraging its capabilities, teams can engage in responsible research that respects consumer trust while driving impactful insights.
- Tool 3: Application in various research settings.
In various research settings, the application of tools aimed at maintaining the Privacy-Personalization Balance is crucial. Researchers often grapple with the need to provide personalized experiences while safeguarding user privacy. By utilizing data-driven tools effectively, they can meet both objectives. For instance, researchers can collect specific data points while ensuring participants are aware of how their information is being used. This transparency fosters trust, which is essential for collecting rich, insightful data.
Additionally, the implementation of robust data management tools enables researchers to customize their methodologies. They can draw insights from extensive datasets while employing measures like aggregation or anonymization. This practice reduces the risk of revealing personal information and helps maintain ethical standards. Ultimately, tools that adapt to various research contexts will enable researchers to explore the nuanced interplay between personalization and privacy effectively. This balance not only enhances research quality but also empowers participants with a sense of security.
Conclusion: Finding the Right Privacy-Personalization Balance
Achieving the privacy-personalization balance is essential for effective data-driven research. As organizations strive to provide personalized experiences, they must also safeguard user privacy. To find the right equilibrium, researchers should prioritize transparency and informed consent, ensuring that participants understand how their data will be used.
Incorporating privacy-preserving technologies can further enhance this balance. By anonymizing data and limiting access, organizations can respect individual rights while still harnessing valuable insights. Ultimately, fostering a data culture that respects privacy will lead to more trustworthy and effective personalization efforts, benefiting both researchers and participants alike.