How to Analyze Consumer Sentiments
-
Hello Insight
- 10 min read
In the evolving realm of consumer feedback, understanding and interpreting sentiments can significantly shape business strategies. Introduction to Sentiment Analysis Techniques reveals how brands can decipher customer emotions underlying their feedback, whether expressed positively or negatively. By tapping into various methods, businesses gain insights that are crucial for enhancing products and services.
These techniques encompass a diverse range of approaches, including keyword spotting and statistical methods. Each technique holds unique advantages and limitations, making it essential for businesses to choose wisely. This informed selection can lead to deeper consumer insights, ultimately driving strategic decisions that align with user expectations and preferences.
Generate visualizations from your qualitative data. At Scale.
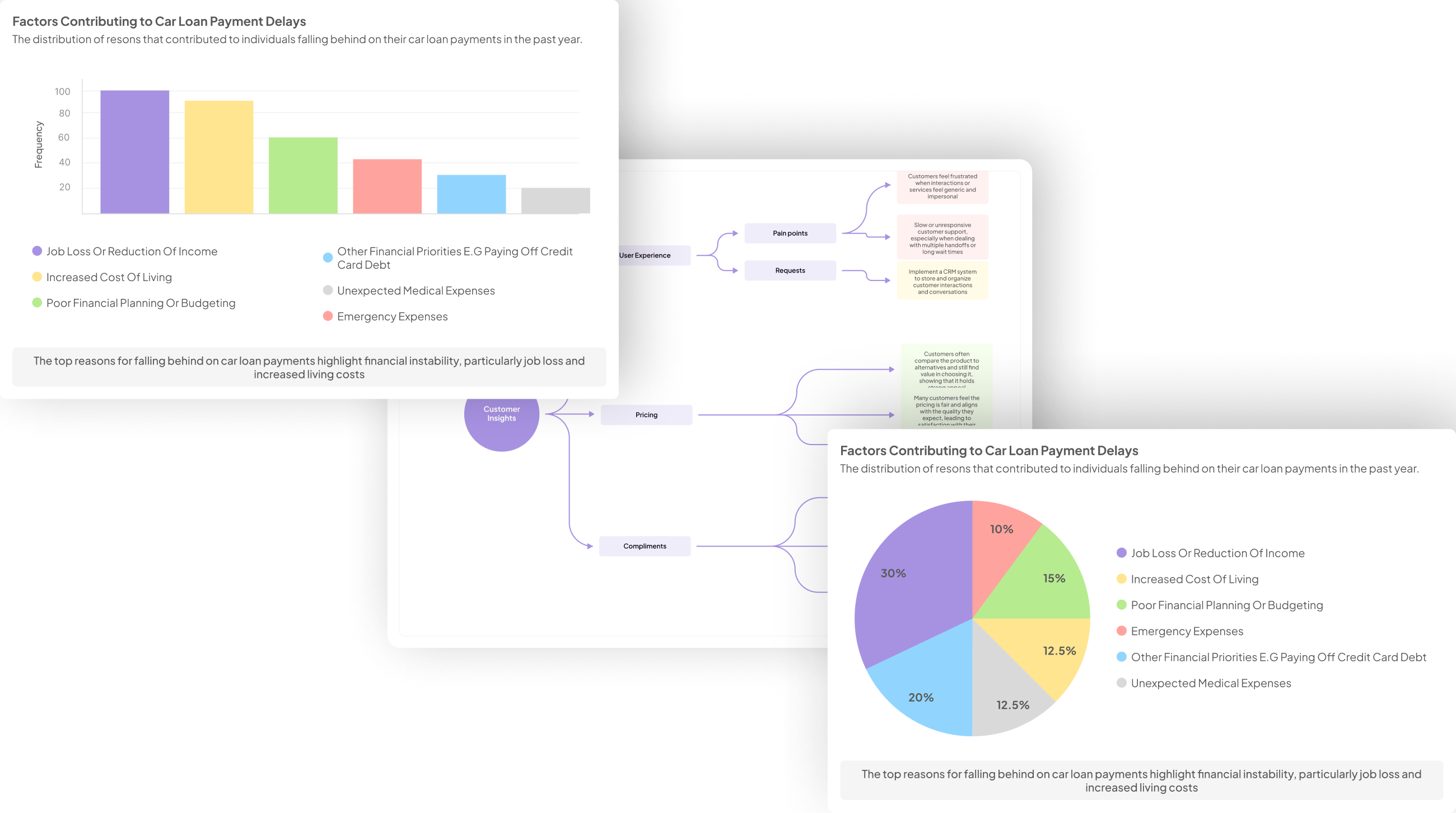
Understanding Sentiment Analysis Techniques
Sentiment analysis techniques encompass various methods used to interpret consumer emotions and opinions about products or services. Understanding these techniques is crucial for businesses seeking to enhance customer satisfaction and adapt their strategies accordingly. By extracting valuable insights from customer feedback, organizations can obtain a better understanding of market trends and consumer preferences.
Several key techniques are commonly employed in sentiment analysis. Keyword spotting involves identifying specific words or phrases that indicate sentiment, while statistical methods apply mathematical models to predict overall sentiment based on patterns in the data. Machine learning techniques can enhance accuracy by continually improving from new data inputs. Each technique has its advantages and limitations, influencing the choice of method based on organizational needs and data complexity. Exploring the right sentiment analysis techniques will empower businesses to make informed decisions and foster stronger connections with their customers.
The Basics of Sentiment Analysis Techniques
Sentiment analysis techniques are essential for understanding consumer feedback and enhancing business decisions. These techniques help identify emotional tones in textual data, enabling companies to gauge consumer sentiment about their products and services. By pinpointing positive or negative feelings, businesses can make informed adjustments and improve customer experiences.
There are several core components of sentiment analysis techniques, including data collection, data processing, and sentiment classification. First, gathering customer feedback from various sources, such as social media or surveys, lays the groundwork for meaningful analysis. Next, the data must be cleaned and organized to ensure accuracy in evaluation. Finally, sentiment classification applies different methods, such as keyword spotting or machine learning, to interpret the emotional context. Understanding these basics is crucial for any organization aiming to accurately analyze consumer sentiments and leverage insights effectively.
- Definition and importance in consumer sentiment analysis
Sentiment analysis techniques play a crucial role in understanding consumer sentiments. This process involves interpreting and quantifying emotions expressed in customer feedback, reviews, and social media interactions. Accurate sentiment analysis helps businesses gauge public opinion and identify areas for improvement, thus enhancing their products and services. By focusing on the underlying emotions of consumers, businesses can tailor their approaches to meet market demands and expectations effectively.
The importance of sentiment analysis extends beyond simple interpretation; it is a strategic tool that informs decision-making. Analyzing consumer sentiments allows businesses to capture both positive and negative feedback, informing product development and marketing strategies. Businesses can also identify trends over time, helping to predict future consumer behavior. Ultimately, utilizing sentiment analysis techniques is essential for creating a customer-centric environment that fosters loyalty and enhances overall satisfaction. Understanding this process can significantly impact a business's success in a competitive marketplace.
- Key components of sentiment analysis
In any exploration of sentiment analysis techniques, understanding the key components is essential. At its core, sentiment analysis focuses on extracting insights from consumer opinions, emotions, and feedback. This is typically achieved through various methods like natural language processing (NLP), which allows machines to interpret human language. Another significant component involves data mining, which helps in unearthing patterns and trends within vast datasets.
Additionally, the accuracy of sentiment analysis relies heavily on training datasets that are representative of the consumer demographic. Machine learning algorithms are employed to classify sentiments as positive, negative, or neutral, based on the context of the language used. Incorporating these elements ensures a robust analysis process, ultimately leading to a clearer understanding of consumer sentiments. Effective analysis enables businesses to make informed decisions based on genuine consumer feedback, thereby enhancing engagement and fostering loyalty.
Common Sentiment Analysis Techniques in Use
Sentiment analysis techniques are essential for understanding consumer feelings and opinions towards products or services. One common method is keyword spotting, which involves identifying specific terms that indicate sentiment. For instance, positive words like "excellent" can signal favorable attitudes, while negative terms such as "poor" highlight dissatisfaction. This technique is straightforward and easily implemented but might overlook contextual nuances.
Another approach is using statistical methods, which involve applying algorithms to analyze patterns in larger datasets. These techniques can capture trends and sentiments across vast amounts of feedback. However, they often require substantial data and can miss the subtleties of individual consumer emotions. On the advanced end, machine learning techniques utilize algorithms to learn from data and improve sentiments classification over time. While powerful, these methods necessitate technical expertise and large datasets, posing challenges for some businesses.
Understanding and choosing the appropriate sentiment analysis techniques can significantly impact the insights derived from consumer data, guiding effective strategies and decision-making.
- Overview of techniques such as keyword spotting, statistical methods, and machine learning
Analyzing consumer sentiments requires various methods, each contributing uniquely to understanding customer perspectives. One prominent technique is keyword spotting, which involves identifying specific words or phrases that reflect consumer opinions. This approach serves as a straightforward entry point into sentiment analysis, helping businesses gather insights from customer feedback. However, it is limited by its inability to understand context or emotion behind the words.
Statistical methods follow, employing quantitative data analysis to interpret sentiments on a larger scale. By using statistics, businesses can identify trends and correlations, providing a foundational understanding of consumer behavior. Meanwhile, machine learning stands out as a powerful tool; it can analyze vast amounts of data, recognize patterns, and adapt over time to improve accuracy. While machine learning offers advanced capabilities, it requires substantial data and expertise. Each of these sentiment analysis techniques has unique strengths and challenges, making it essential for businesses to choose an approach that best aligns with their specific needs.
- Advantages and limitations of each technique
When evaluating sentiment analysis techniques, it’s crucial to consider both their advantages and limitations. One widely used technique is keyword spotting, which is simple and effective for identifying specific sentiments based on targeted phrases. This method is quick to implement and doesn't require extensive training data, making it accessible for many businesses. However, its reliance on predetermined keywords can lead to missed nuances in consumer emotions, resulting in oversimplified conclusions.
On the other hand, statistical methods and machine learning offer more sophisticated approaches to sentiment analysis. They can capture complex sentiment patterns across large datasets, providing deeper insights into consumer feelings. Yet, these techniques often demand significant computational resources and a wealth of labeled training data, which may be challenging for smaller organizations to obtain. Understanding these advantages and limitations is vital for choosing the most suitable sentiment analysis technique that aligns with your analytical goals.
Evaluate Performance on Customer Calls for Quality Assurance.
Implementing Sentiment Analysis Techniques: Steps and Tools
Implementing sentiment analysis techniques involves several crucial steps, each designed to enhance understanding of consumer feedback. Initially, data collection is essential; it requires gathering various forms of consumer insights, such as reviews, surveys, or social media comments. Next comes data processing, which involves cleaning and preparing the collected data for analysis. This step ensures that noise and irrelevant information do not hinder your results.
Once data is ready, sentiment classification is the final analysis phase where sentiment analysis techniques are applied. This could include basic keyword spotting or more advanced machine learning methods. Equally important are the tools needed for implementation; popular options like IBM Watson or Google NLP can significantly streamline the process. Selecting the right tool hinges on your specific business needs and the complexity of the data involved. This systematic approach will empower you to interpret consumer emotions effectively and make informed decisions.
Step-by-Step Sentiment Analysis
To conduct effective sentiment analysis, it is essential to follow a step-by-step approach that clearly outlines the processes involved. The first step involves data collection, where you gather consumer feedback from various sources, such as surveys, reviews, and social media. This data serves as the foundation for your analysis, providing insights into consumer emotions and opinions.
Next, data processing is crucial. This step entails cleaning and preparing the data, which may involve removing noise, handling missing values, and organizing the information in a usable format. Once processed, the final step is sentiment classification. Here, you apply sentiment analysis techniques to categorize feedback into sentiments—positive, negative, or neutral. This structured approach not only enhances your analysis but also facilitates a clearer understanding of consumer sentiments.
- Data Collection: Gathering consumer feedback
Data collection is the foundation for meaningful consumer sentiment analysis. To gather effective feedback, it is crucial to employ various strategies that encourage open and honest responses from customers. This involves designing surveys, interviews, and feedback forms that are easy for consumers to navigate. Personalizing these interactions enhances the likelihood of obtaining valuable insights. Engaging with customers on social media platforms also provides organic feedback, reflecting their true sentiments.
Once feedback is collected, it is essential to analyze the data using sentiment analysis techniques. By categorizing comments as positive, negative, or neutral, businesses can identify trends and emerging issues that need addressing. Moreover, utilizing tools for data analysis streamlines the process and allows for deeper insights into customer perceptions. Integrating qualitative and quantitative feedback offers a comprehensive view and fosters informed decision-making, ultimately driving improvements in products and services.
- Data Processing: Cleaning and preparing the data
Data processing in sentiment analysis involves cleaning and preparing data to ensure accuracy and reliability. Initially, it requires removing irrelevant information and standardizing the format of the collected data. This step ensures uniformity across the dataset, making it easier to analyze sentiments effectively.
Next, normalization plays a crucial role, as it adjusts differing data scales to a common frame of reference. Techniques like tokenization, where text is broken down into individual words or phrases, help facilitate deeper analysis. Additionally, filtering out stop words—common words that hold little semantic value—can enhance the focus on keywords that genuinely reflect consumer sentiments. This meticulous approach to data preparation sets a robust foundation for sentiment classification, ultimately leading to more reliable insights for decision-making. By investing time in meticulous data processing, organizations can yield richer insights and foster improved customer engagement.
- Sentiment Classification: Applying the sentiment analysis techniques
Sentiment classification is a vital step in the broader process of applying sentiment analysis techniques. This phase involves categorizing textual data into predefined sentiment categories, such as positive, negative, or neutral. By effectively classifying sentiments, businesses can gain insights into customer opinions, preferences, and overall brand perception. It is crucial to develop a reliable system that accurately reflects consumer sentiment to inform strategic decision-making and improve customer satisfaction.
There are several techniques utilized in sentiment classification. First, keyword spotting analyzes specific words or phrases to determine sentiment. Second, statistical methods evaluate the frequency and distribution of words to ascertain overall sentiment trends. Finally, machine learning approaches use algorithms to learn from data, enabling more nuanced sentiment classification that adapts over time. Understanding these methods can enhance your ability to interpret consumer sentiments accurately, paving the way for more effective market strategies.
Tools for Sentiment Analysis Techniques
In the realm of consumer sentiment analysis, utilizing the right tools is crucial for effective data interpretation. There are various software options available that cater to different analysis needs, each offering unique capabilities for processing customer feedback. For instance, tools like IBM Watson and Google Natural Language Processing are widely recognized for their robust sentiment analysis techniques. They provide comprehensive features that streamline the analysis process, ensuring accurate insights are derived from raw data.
When selecting a sentiment analysis tool, consider factors like ease of use, scalability, and the specific requirements of your business. Begin with a tool that allows for straightforward data collection and processing, as these initial steps are foundational. Once you identify the insights your chosen tool reveals, you can effectively apply findings to enhance product development and customer engagement strategies. By carefully considering your options, you can leverage sentiment analysis techniques to transform consumer feedback into actionable knowledge.
- Overview of popular sentiment analysis tools (e.g., IBM Watson, Google NLP)
When exploring popular sentiment analysis tools, it becomes essential to understand the features that these platforms offer. Many solutions utilize advanced algorithms and machine learning models to analyze consumer sentiments efficiently. These tools are designed to sift through vast amounts of text data, identifying emotional undertones and trends within consumer feedback.
In the selection of sentiment analysis tools, consider the following points:
- Ease of Use: The user interface should be intuitive, allowing users to quickly navigate and access insights.
- Integration Capabilities: Ensure the tool can integrate seamlessly with your existing systems, enabling a more cohesive analysis process.
- Customization Options: Look for tools that offer customization, as this can help tailor the analysis to specific business needs.
- Scalability: Choose tools that can grow with your business, accommodating increasing data volumes without compromising performance.
Understanding these features will assist in selecting the best sentiment analysis techniques suited for your organization’s consumer insights goals.
- How to choose the right tool for your business needs
Choosing the right tool for your business needs is crucial in effectively analyzing consumer sentiments. Start by assessing what specific insights you want to gain, such as customer preferences, pain points, or trends. This clarity helps narrow down the options available to you. Consider the size of your business and the volume of data you handle, as some sentiment analysis techniques may work better for smaller datasets, while others are suited for larger volumes.
Evaluate various sentiment analysis tools based on their capabilities, ease of use, and whether they align with your goals. Explore options that integrate with your existing systems, which can improve efficiency. Additionally, factor in the level of support you might need, from onboarding to ongoing assistance. By carefully weighing your priorities against the available tools, you can make an informed choice that enhances your understanding of consumer sentiments and elevates your business strategy.
Conclusion: Mastering Sentiment Analysis Techniques for Consumer Insights
Mastering sentiment analysis techniques is crucial for gaining valuable consumer insights. By effectively analyzing consumer feedback, organizations can better understand customer emotions and preferences, guiding their product development and marketing strategies. Using a combination of qualitative and quantitative methodologies enhances the richness of the insights obtained, allowing businesses to make informed decisions.
As companies refine their sentiment analysis techniques, they discover patterns that directly influence consumer behavior. Understanding these sentiments empowers organizations to create tailored customer experiences, fostering loyalty and driving growth. By committing to a comprehensive approach, businesses can successfully translate consumer sentiments into actionable strategies that resonate with their target audiences.