Comprehensive Text Analysis begins with a fundamental question: how can we extract meaningful insights from vast amounts of text? In today's data-driven world, this analysis is not just a luxury but a necessity for organizations aiming to understand their audience and improve their strategies. By embracing various text analytics techniques, businesses can turn unstructured text into structured knowledge, paving the way for informed decision-making.
This section will explore the foundational aspects of Comprehensive Text Analysis, focusing on the key methodologies that drive effective text interpretation. From text classification that organizes data efficiently to sentiment analysis that decodes emotional tone, it is essential to grasp these pillars to harness the full potential of text analytics. Together, these methods create a robust framework for analyzing and deriving value from all forms of written communication.
Generate visualizations from your qualitative data. At Scale.
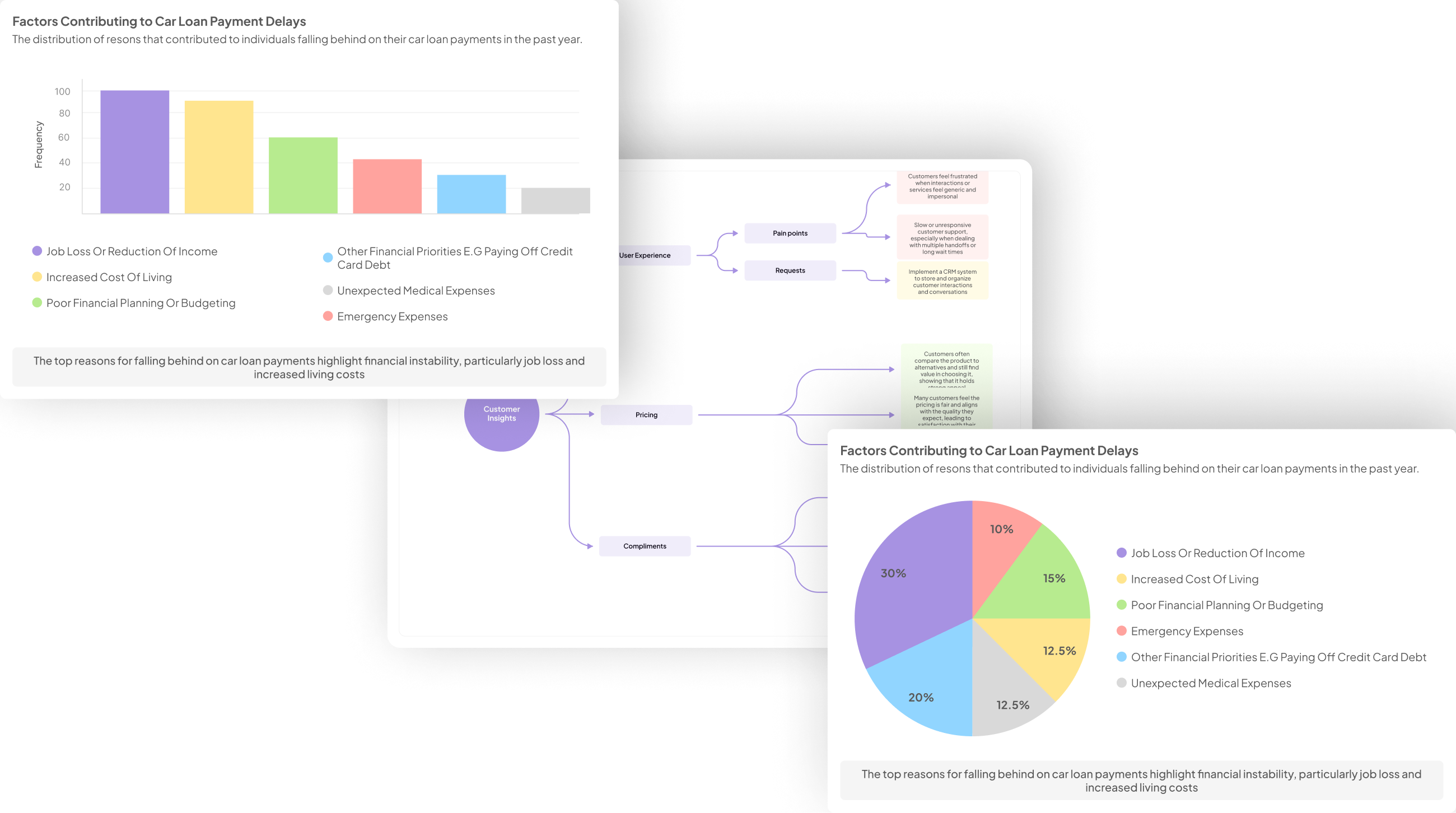
Understanding the Pillars of Comprehensive Text Analysis
Comprehensive Text Analysis lays its foundation on several key pillars that enable organizations to derive actionable insights from vast amounts of text data. One primary component is text classification, which organizes information into predefined categories. This process not only streamlines data handling but also enhances data retrieval, making it easier to analyze content effectively.
Another crucial aspect is sentiment analysis, which uncovers the emotional tone behind the text. By identifying sentiments such as joy, anger, or criticism, businesses can gain deeper insights into customer opinions and feedback. These two pillars—text classification and sentiment analysis—are essential for developing a robust framework in Comprehensive Text Analysis, guiding organizations in their quest for clarity and informed decision-making. Understanding these elements helps demystify the process and highlights their importance in extracting meaningful data from text sources.
Text Classification: Organizing Data Efficiently
Text classification plays a crucial role in organizing large volumes of data efficiently. By categorizing text into predefined classes or labels, organizations can streamline the process of data retrieval and analysis. This method ensures that relevant information can be easily accessed and interpreted, allowing for effective decision-making. Effective classification methods not only enhance data organization but also contribute significantly to comprehensive text analysis.
In practice, text classification may utilize various approaches such as supervised learning, unsupervised learning, and even deep learning techniques. Each method offers unique benefits, depending on the context and type of data being analyzed. For example, supervised learning requires labeled data for training, while unsupervised learning can identify patterns in unstructured data without prior labels. By deploying these techniques, businesses can derive meaningful insights from their textual data, fostering a better understanding of customer sentiments and market trends.
Sentiment Analysis: Decoding Emotional Tone
Sentiment analysis plays a crucial role in understanding the emotional tone embedded in text data. By analyzing customer feedback, social media interactions, and reviews, businesses can gain insights into public perception. This analysis allows organizations to gauge customer satisfaction and identify areas needing improvement or adjustment. It provides a lens through which sentiments can be decoded, helping to capture the nuances of customer feelings toward products or services.
To effectively conduct sentiment analysis, there are key steps to consider. First, data collection is essential; gathering textual data from various sources offers a broad view of customer opinions. Next, data preprocessing helps rid the text of noise, ensuring that the analysis focuses on relevant sentiments. Following this, sentiment scoring can assign positive, negative, or neutral tags to the text. Finally, interpreting these scores effectively provides actionable insights that guide strategic decision-making. By embracing sentiment analysis, businesses can enhance their understanding of customer emotions, leading to improved products and services.
Generate Journey maps, Mind maps, Bar charts and more from your data in Minutes
Advanced Techniques in Comprehensive Text Analysis
Comprehensive Text Analysis encompasses various advanced techniques that enhance the depth and accuracy of data interpretation. One key technique is Topic Modeling, which uncovers hidden patterns within large text corpora. This method identifies clusters of related words and phrases, allowing analysts to discern underlying themes without extensive manual review.
Another significant technique is Named Entity Recognition (NER), which automates the extraction of critical information from text. NER efficiently identifies entities such as names, organizations, and locations, transforming unstructured data into structured insights. By implementing these advanced methods, analysts can synthesize complex information quickly and effectively, leading to informed decision-making and strategic planning.
Utilizing these advanced techniques ensures that Comprehensive Text Analysis remains a vital tool, equipping users with the ability to navigate and interpret large volumes of data. As data-driven decision-making becomes increasingly important, mastering these methods is essential for deriving actionable insights in various fields.
Topic Modeling: Unveiling Hidden Patterns
Topic modeling serves as a powerful technique within the realm of comprehensive text analysis, designed to reveal hidden structures within vast datasets. By categorizing words and phrases into distinct themes, organizations can uncover insights that would otherwise remain obscured. This process often involves using algorithms that identify clusters of related terms, providing a clearer understanding of the underlying context and trends in the text data.
Several benefits emerge from implementing topic modeling. Firstly, it enhances the ability to identify customer pain points by analyzing large volumes of feedback. Secondly, it helps in summarizing information, allowing businesses to quickly grasp key themes without manually combing through every document. Finally, this technique can enhance report generation, offering a succinct overview that aids in decision-making. By employing topic modeling, organizations can deepen their understanding of customer sentiments, leading to more informed strategies and actions.
Named Entity Recognition (NER): Extracting Key Information
Named Entity Recognition (NER) is a critical component in comprehensive text analysis, focusing on identifying and classifying key entities within unstructured data. These entities often include names of people, organizations, locations, dates, and other specific terms relevant to the context. By automating the extraction of these elements, NER helps streamline the information-gathering process, making it easier for businesses to draw actionable insights from vast amounts of text.
For effective NER implementation, the process usually involves several steps. First, data preprocessing is essential for enhancing text clarity and structure. Next, machine learning algorithms are commonly employed to recognize patterns and predict entity classes based on context. Lastly, the extracted entities can be used for further analysis, such as improving customer service strategies or enhancing marketing campaigns. By leveraging NER technology, businesses can unlock valuable insights, thereby enhancing their decision-making processes and overall effectiveness in engaging their target audiences.
Conclusion: The Impact of Comprehensive Text Analysis on Modern Data Insights
Comprehensive Text Analysis plays a crucial role in transforming how organizations interpret vast amounts of textual data. By utilizing various methods, businesses can uncover insights that directly influence operational strategies. This process allows teams to identify trends, understand customer sentiments, and address pain points effectively.
Moreover, the implications of Comprehensive Text Analysis extend beyond immediate insights. They pave the way for informed decision-making, fostering a data-driven culture within organizations. As companies become adept at harnessing these analytical tools, they position themselves to innovate continually and adapt to market changes with agility, ensuring long-term success in today’s competitive landscape.