Text-Analytics: Comprehensive Overview for Beginners
-
Hello Insight
- 10 min read
In the modern digital age, the ability to glean insights from vast amounts of text is invaluable. As information surrounds us, Introductory Text Analytics emerges as a powerful tool for understanding and interpreting written content. This approach not only helps businesses analyze customer feedback but also aids in deciphering social media conversations. By harnessing text analytics, users can turn raw data into meaningful insights, paving the way for informed decision-making.
To embark on this journey, one must familiarize themselves with the underlying principles of text analytics. This section serves as your starting point, illuminating the essential concepts and techniques that form the foundation of this field. From understanding natural language processing to preparing for an effective analysis, mastering Introductory Text Analytics offers a pathway to unlocking the potential within textual data.
Generate visualizations from your qualitative data. At Scale.
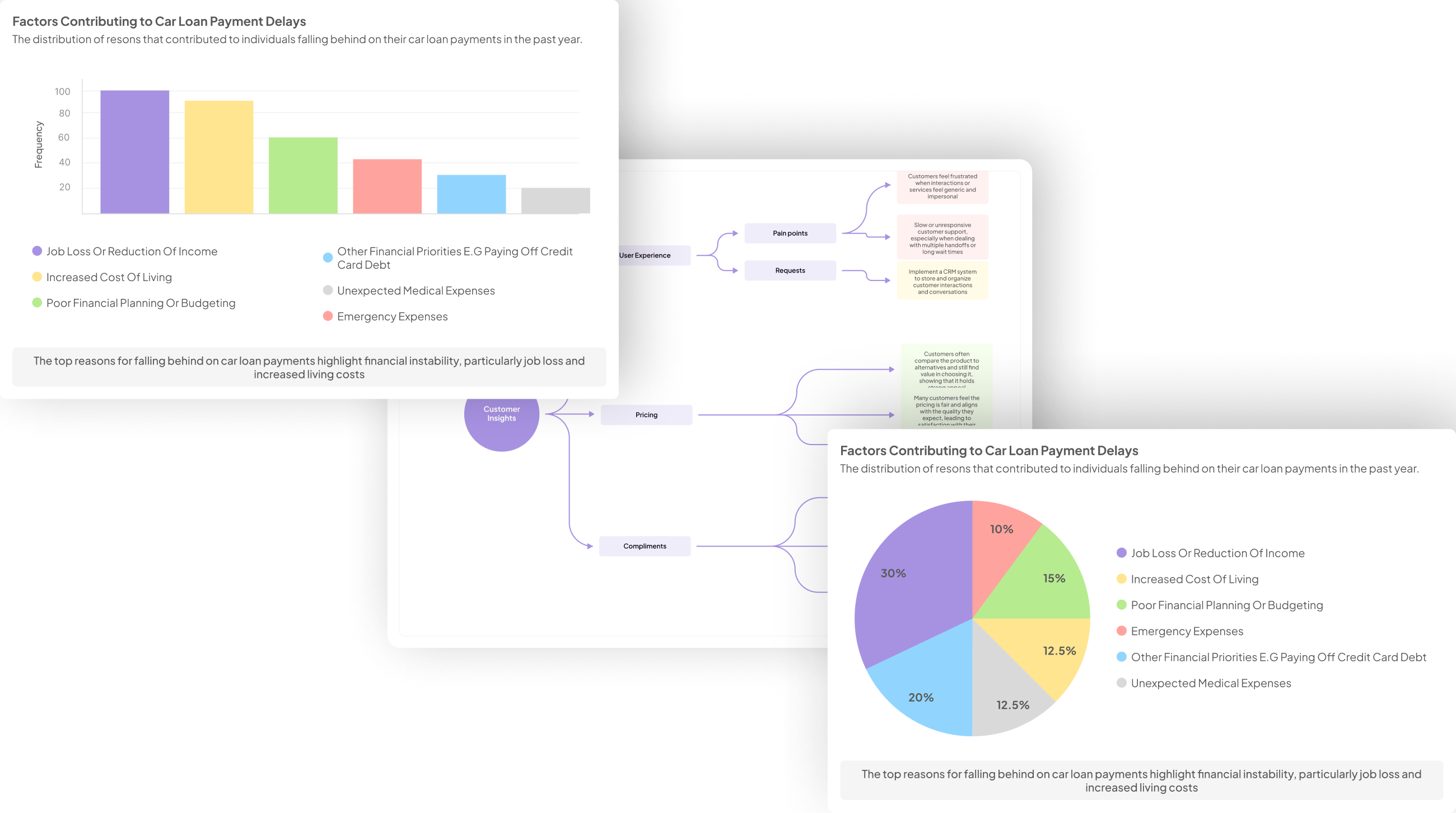
Key Concepts in Introductory Text Analytics
Introductory Text Analytics encompasses several key concepts that beginners need to grasp. Understanding these concepts is crucial for successfully applying text analytics techniques. At its core, text analytics involves the extraction and analysis of insights from unstructured text data. This type of data can come from various sources, including emails, social media, and customer feedback.
Several fundamental components facilitate effective text analytics. First, Natural Language Processing (NLP) plays a pivotal role in enabling computers to understand human language nuances. Techniques such as tokenization and stemming help break down text into manageable pieces for analysis. Second, sentiment analysis enables you to gauge opinions expressed in text, giving valuable insights into customer emotions and perceptions. Lastly, familiarizing yourself with data preprocessing techniques ensures your analysis yields accurate results. By mastering these concepts, you position yourself to utilize text analytics effectively in various applications.
Understanding Natural Language Processing (NLP)
Natural Language Processing (NLP) is an essential component of introductory text analytics, enabling computers to understand human language. It transforms unstructured text into structured data, allowing for more straightforward analysis. This technology is crucial for various applications, such as chatbots, sentiment analysis, and information extraction, significantly enhancing how users interact with digital content.
To grasp NLP effectively, consider the following concepts:
Tokenization: This process breaks down text into individual components, such as words or phrases, making it easier to analyze.
POS Tagging: Part-of-speech tagging assigns grammatical categories to each token, aiding in understanding the text's structure and meaning.
Named Entity Recognition (NER): NER identifies and categorizes key elements in the text, like names, organizations, and dates, which is vital for information retrieval.
Sentiment Analysis: This technique evaluates the emotional tone behind words, helping organizations understand customer opinions and feelings.
Understanding these concepts lays the foundation for utilizing NLP in introductory text analytics, allowing you to extract valuable insights from textual data effectively.
Why Text Analytics Matters
Text analytics plays a crucial role in today’s data-driven world. It enables organizations to transform vast amounts of unstructured text into meaningful insights. By capturing customer conversations, feedback, and social media interactions, businesses can uncover trends, sentiments, and potential areas for improvement.
One of the primary benefits of text analytics is its capacity to enhance decision-making. With timely insights, organizations can respond proactively to customer needs and market changes. Moreover, it facilitates efficient collaboration by consolidating insights that would typically remain scattered across various platforms. Understanding why text analytics matters is essential for any business aiming to thrive in a competitive environment. As we explore introductory text analytics, it becomes evident that organizations equipped with these tools can translate data into action, positioning themselves ahead of the competition. Embracing this technology not only streamlines operations but also fosters a customer-centric approach that drives growth and innovation.
Evaluate Performance on Customer Calls for Quality Assurance.
Introductory Text Analytics Tools and Techniques
Introductory Text Analytics encompasses various tools and techniques essential for transforming raw text into meaningful insights. To embark on this journey, beginners should familiarize themselves with common tools designed to simplify the analysis process. Popular software programs offer user-friendly interfaces, enabling users to easily upload files and retrieve valuable information. The initial step is crucial; selecting the appropriate software tailored to the specific needs of your analytics project is vital for success.
Once the right tool is chosen, the next step is setting up your analysis framework. This includes text preprocessing, which prepares data by cleaning it, removing noise, and normalizing it for accurate results. Another critical technique is sentiment analysis, aimed at determining the emotional tone behind textual data. Together, these introductory techniques lay a solid foundation for successful text analytics and provide actionable insights for decision-making processes.
Common Tools for Beginners
When diving into Introductory Text Analytics, it's essential to familiarize yourself with common tools that make the process more accessible. This exploration empowers beginners to uncover valuable insights without requiring extensive expertise. First, there are user-friendly platforms designed specifically for analyzing text data. These tools often come with intuitive interfaces that allow for seamless interaction, making it easy to conduct analyses right from the homepage.
Next, a critical aspect is setting up your analysis effectively. An ideal tool should offer features like the ability to transcribe calls, visualize conversations, and pull out key insights such as customer pain points and behaviors. Such functions enable users to understand the voice of the customer better, facilitating data-driven decisions. Lastly, consider tools that support project-level analyses, allowing you to group multiple files for broader insights. Engaging with these tools can dramatically enhance your learning experience and help solidify your foundation in text analytics.
Step 1: Choosing the Right Software
Selecting appropriate software is crucial for your journey into Introductory Text Analytics. The right software can enhance your data analysis and streamline your workflow. When choosing software, consider the specific features you need. Look for user-friendliness, integrations with other tools, and scalability. It’s essential to clarify your objectives, which will guide you in identifying software that fits your requirements.
Next, evaluate your budget. Various options are available, ranging from free tools to professional-grade software. Explore user reviews to gauge effectiveness and customer support services. Also, consider tools that offer tutorials or guided assistance, especially if you are new to text analytics. Familiarity with the basics will not only simplify your process but also empower you as you grow in your analytical skills. These steps will pave the way for a successful analysis experience, boosting your confidence and understanding of this fascinating field.
Step 2: Setting up Your Analysis
Setting up your analysis is a crucial part of introductory text analytics. To begin, you should gather relevant data sources that contain the text you want to analyze. This may include customer feedback, social media posts, or product reviews, for instance. Organizing your data will help streamline your analysis, as it allows you to identify themes and insights more easily.
Once your data is organized, it's important to define your objectives. What specific questions do you want to answer? Clarifying your goals with the analysis can guide you in selecting the right tools and techniques. For example, if you're interested in sentiment analysis, proper labeling of your data can enhance the accuracy of your results. Additionally, consider the methods you will use to visualize your data. Visual tools can provide a clear representation of trends and patterns, making your findings more interpretable. By meticulously setting up your analysis, you lay a solid foundation for extracting actionable insights from your text data.
Basic Techniques in Text Analytics
In the realm of introductory text analytics, mastering basic techniques is crucial for effective data interpretation. Two essential techniques stand out: text preprocessing and sentiment analysis. Text preprocessing lays the groundwork for any analysis, ensuring that the raw text data is cleaned and formatted appropriately. This process involves removing irrelevant elements, standardizing formats, and tokenizing text into manageable chunks. By filtering out noise, we can focus on the core messages within the text.
Once the data is prepared, sentiment analysis delves into understanding the emotional tone behind the words. This technique helps identify sentiments such as positivity, negativity, or neutrality, providing a richer context for interpretation. By combining these techniques, beginners can extract valuable insights from textual data while also honing their overall analytical skills. This foundational knowledge sets the stage for deeper exploration into more advanced text analytics systems.
Step 1: Text Preprocessing
Text preprocessing is the foundational step in introductory text analytics that prepares raw text for analysis. This process involves cleaning and structuring the text data to derive meaningful insights. The goal is to transform the text into a format that can be easily understood by analytical algorithms. Without proper preprocessing, subsequent analysis such as sentiment detection or topic modeling may yield unreliable results.
Key aspects of text preprocessing include tokenization, where text is split into smaller units like words or phrases, and removing irrelevant information such as stop words, which do not contribute to the text's meaning. Additionally, normalizing text through techniques like stemming or lemmatization helps in reducing different word forms to their base forms. By following these essential steps, analysts can ensure that their text analytics efforts produce accurate and valuable insights.
Step 2: Sentiment Analysis
Sentiment analysis is a fundamental step in introductory text analytics, enabling users to gauge the emotions behind textual data. This technique examines words and phrases to categorize them into positive, negative, or neutral sentiments. By applying sentiment analysis, businesses can understand customer opinions and emotional reactions to their products or services.
The process begins with gathering the text data, which could range from customer reviews to social media comments. Then, automated tools analyze the language patterns, helping to identify trends and overall sentiment. This provides valuable insights into customer satisfaction and areas for improvement. Understanding sentiment is crucial as it directly influences decision-making and enhances customer engagement. By effectively utilizing sentiment analysis, organizations can tailor their strategies to meet consumer needs and expectations, ultimately driving success.
Conclusion: The Future of Introductory Text Analytics
As we look towards the future of Introductory Text Analytics, the landscape is becoming increasingly accessible and user-friendly. New tools aim to democratize data insights, empowering individuals without technical backgrounds to analyze text data efficiently. This shift will foster greater understanding and utilization of text analytics, making it integral within various sectors.
Moreover, advancements in artificial intelligence and machine learning will simplify processes like sentiment analysis and text preprocessing. These developments will enable businesses to uncover valuable insights swiftly, optimizing decision-making. As more organizations recognize the transformative potential of Introductory Text Analytics, we can anticipate a surge in innovative applications and increased emphasis on data-driven strategies.