Text Analytics vs NLP: Key Differences Explained
-
Hello Insight
- 10 min read
Text analytics focuses on extracting meaningful insights from unstructured text data. This involves methods such as sentiment analysis, which assesses emotional tone, or topic modeling, helping identify themes in large volumes of text. By processing data effectively, organizations can uncover trends and preferences that inform decisions. Additionally, text analytics is crucial for interpreting customer feedback, social media interactions, and survey responses, contributing to enhanced customer understanding.
In contrast, natural language processing (NLP) is about enabling machines to understand, interpret, and generate human language. It encompasses various techniques, such as speech recognition and language generation, allowing for more sophisticated interactions between humans and machines. NLP techniques support applications like chatbots and translation services, enhancing user experience. While both text analytics and NLP share the goal of improving communication through technology, their approaches and applications differ significantly in the broader context of language processing comparison.
Introduction: Language Processing Comparison in Text Analytics vs NLP
Language processing is a pivotal aspect of extracting insights from textual data, forming the backbone of both text analytics and natural language processing (NLP). As we explore this language processing comparison, it's essential to understand the distinct roles each domain plays in leveraging language for analytics. While text analytics focuses on the interpretation of structured and unstructured data, NLP provides the tools for understanding context, sentiment, and intent within that data.
In the ever-evolving world of data analysis, differentiating between text analytics and NLP can enhance decision-making. By recognizing how these two fields intersect yet diverge, businesses can better harness their potential. This understanding equips professionals with the knowledge to apply the most effective technique for their specific needs, leading to deeper insights and more informed strategies.
Generate visualizations from your qualitative data. At Scale.
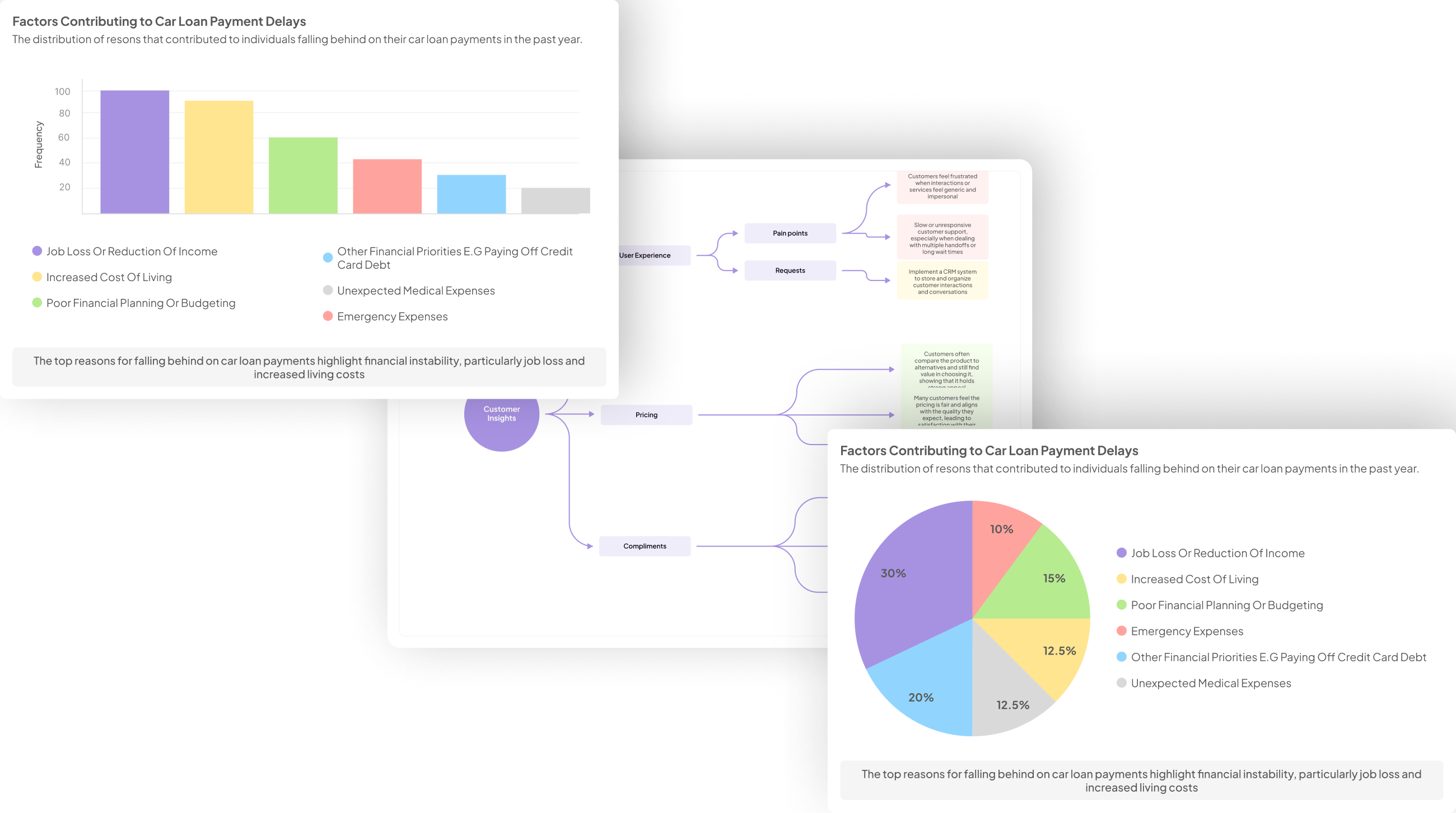
Key Concepts of Language Processing Comparison
Language processing comparison involves examining how various approaches, such as text analytics and natural language processing (NLP), handle linguistic data. Both frameworks utilize algorithms and data analysis to extract meaningful insights from text, yet they do so in distinct ways. This differentiation is essential for understanding how organizations can best meet their analytical needs.
Text analytics focuses on extracting structured information from unstructured data, often emphasizing quantitative measures. It is typically used for tasks like sentiment analysis and keyword extraction. On the other hand, NLP emphasizes understanding and interpreting language in a way that resembles human comprehension. It enables more complex interactions, such as language translation and conversational agents. By comparing these methods, organizations can understand which tools align best with their objectives, enhancing their decision-making and operational efficiencies.
Text Analytics in Language Processing
Text analytics plays a crucial role in language processing by extracting meaningful insights from text data. This process involves breaking down and analyzing large volumes of text to identify patterns, trends, and sentiments within the data. By employing various techniques, businesses can uncover valuable information that drives decision-making and enhances customer engagement.
In the comparison of text analytics and natural language processing (NLP), understanding how each contributes to language processing is essential. Text analytics focuses primarily on understanding and summarizing data, while NLP encompasses a broader scope by enabling machines to comprehend and interpret human language contextually. Both fields work in tandem to unlock the full potential of data-driven insights, showcasing the dynamic nature of language processing and its significance in analyzing information effectively.
Natural Language Processing (NLP) in Language Processing
Natural Language Processing (NLP) is a critical component within the broader scope of language processing. It focuses on the interaction between computers and humans through natural language, allowing computers to understand, interpret, and generate human languages. This process involves various techniques such as tokenization, sentiment analysis, and named entity recognition. NLP enables the extraction of meaning from text, making it indispensable for tasks like chatbots, translation services, and content analysis.
In contrast, traditional language processing may involve more superficial methods, primarily focused on text analytics. While text analytics is often concerned with quantifying data and identifying patterns in text, NLP dives deeper into contextual understanding and language nuances. This distinction is crucial for businesses aiming to utilize insights effectively from customer interactions. Understanding these differences can enhance decision-making and strengthen competitive strategies in today’s data-driven environment.
Evaluate Performance on Customer Calls for Quality Assurance.
Language Processing Comparison: Techniques and Applications
Language processing comparison delves into the various techniques and applications utilized in both text analytics and natural language processing (NLP). Text analytics focuses on extracting insights and themes from datasets, employing methods such as keyword extraction, sentiment analysis, and summarization. These techniques are powerful for businesses aiming to understand consumer behavior through structured datasets.
On the other hand, NLP encompasses a broader range of algorithms designed to understand and interpret human language in a more nuanced way. Techniques like machine translation, conversational agents, and named entity recognition are foundational to NLP applications. Companies can leverage these insights for customer support automation or enhancing user engagement. Ultimately, understanding the language processing comparison equips organizations with the knowledge to choose the right tools for their needs, ensuring more effective data utilization and strategic decision-making.
Techniques in Text Analytics
Text analytics employs various techniques that transform raw text into meaningful insights. These methods allow for the systematic extraction, analysis, and interpretation of data from textual content. By utilizing processes such as tokenization, sentiment analysis, and entity recognition, organizations can identify patterns and trends hidden within large volumes of data. This not only aids in understanding customer opinions but also informs strategic decision-making.
The effectiveness of text analytics stems from its structured approach. For instance, through text mining, practitioners can gather information from different source types, such as social media or customer feedback. Additionally, classification techniques can categorize text data into predefined groups, improving the ability to draw actionable conclusions. Each technique enhances the overall capability of text analytics, allowing businesses to convert simple language into valuable insights that drive growth and innovation.
Techniques in NLP
Natural Language Processing (NLP) encompasses a suite of techniques designed to help machines understand, interpret, and respond to human language. These techniques include tokenization, part-of-speech tagging, named entity recognition, and sentiment analysis. Each method serves to break down language into manageable components, enabling deeper analysis and understanding. Tokenization, for instance, splits text into words or phrases, while part-of-speech tagging identifies the grammatical roles of each word.
Additionally, NLP techniques are applied in various domains, enhancing services like customer support and content recommendation. By utilizing machine learning algorithms, NLP can refine its accuracy over time. This continuous improvement is vital for effective language processing comparison, as it allows NLP systems to learn from vast datasets, adapting to different linguistic patterns and nuances. Through these methodologies, NLP not only processes language but also unlocks insights that can significantly improve user experience.
Real-world Applications: Text Analytics vs NLP
Text Analytics and Natural Language Processing (NLP) have distinct real-world applications that cater to different needs. Text Analytics is primarily concerned with deriving insights from unstructured data. This includes customer feedback, surveys, and other textual sources. Companies utilize text analytics to identify trends, sentiment, and common themes in customer interactions. For instance, businesses can rapidly analyze customer reviews to gauge product reception and adapt marketing strategies accordingly.
Conversely, NLP focuses on understanding and generating human language. Its applications often include chatbots, voice assistants, and automated translation services. By employing NLP, organizations can develop systems that comprehend and respond to inquiries effectively. For example, customer service agents can enhance their efficiency through AI-powered chat interfaces that answer frequently asked questions. Overall, while both techniques fall under the language processing comparison umbrella, they serve unique roles that drive business growth and enhance customer experiences.
Conclusion: Language Processing Comparison Summary
In summary, the comparison between text analytics and natural language processing (NLP) highlights essential differences in their approaches to language processing. Text analytics primarily focuses on quantitative data analysis, emphasizing data extraction and pattern recognition. In contrast, NLP encompasses a broader scope, including language understanding and generation, aiming for more sophisticated interaction with human language.
Understanding this language processing comparison allows businesses to select the right tools based on their specific needs. By integrating the insights from text analytics and methods from NLP, organizations can enhance their decision-making processes and improve customer experiences effectively.
### Key Concepts of Language Processing Comparison
Understanding the nuances of text analytics and natural language processing (NLP) is essential in the realm of language processing comparison. Text analytics mainly focuses on extracting meaningful insights from text data, often utilizing statistical methods. It allows organizations to analyze trends, sentiments, and key themes present in large datasets. This capability is particularly useful for businesses aiming to enhance customer experiences and refine their products based on feedback.
On the other hand, NLP is more about enabling machines to understand, interpret, and respond to human language in a way that is valuable. This involves complex algorithms and machine learning techniques that empower applications like chatbots and virtual assistants. By contrasting these two fields—text analytics and NLP—organizations can better configure strategies that align with their goals, ensuring that they leverage the right technologies to process language effectively.