Text Analytics AI: Best Practices for Businesses
-
Hello Insight
- 10 min read
In today’s data-driven world, businesses continuously seek new ways to harness information. Optimizing text insights not only unlocks customer sentiments but also reveals underlying trends that drive strategic decisions. As companies grapple with vast amounts of text data from surveys, interviews, and feedback, the need for effective analysis becomes critical. Understanding how to extract meaningful insights can be the difference between staying competitive and falling behind.
The journey to optimizing text insights begins with understanding the significance of text analytics. Utilizing AI in this field enables businesses to turn unstructured data into structured formats, facilitating easier comprehension. With the right tools, organizations can identify key pain points and align their offerings with customer needs. By prioritizing data quality and implementing best practices, businesses can transform their approach to understanding market dynamics and customer expectations.
Generate visualizations from your qualitative data. At Scale.
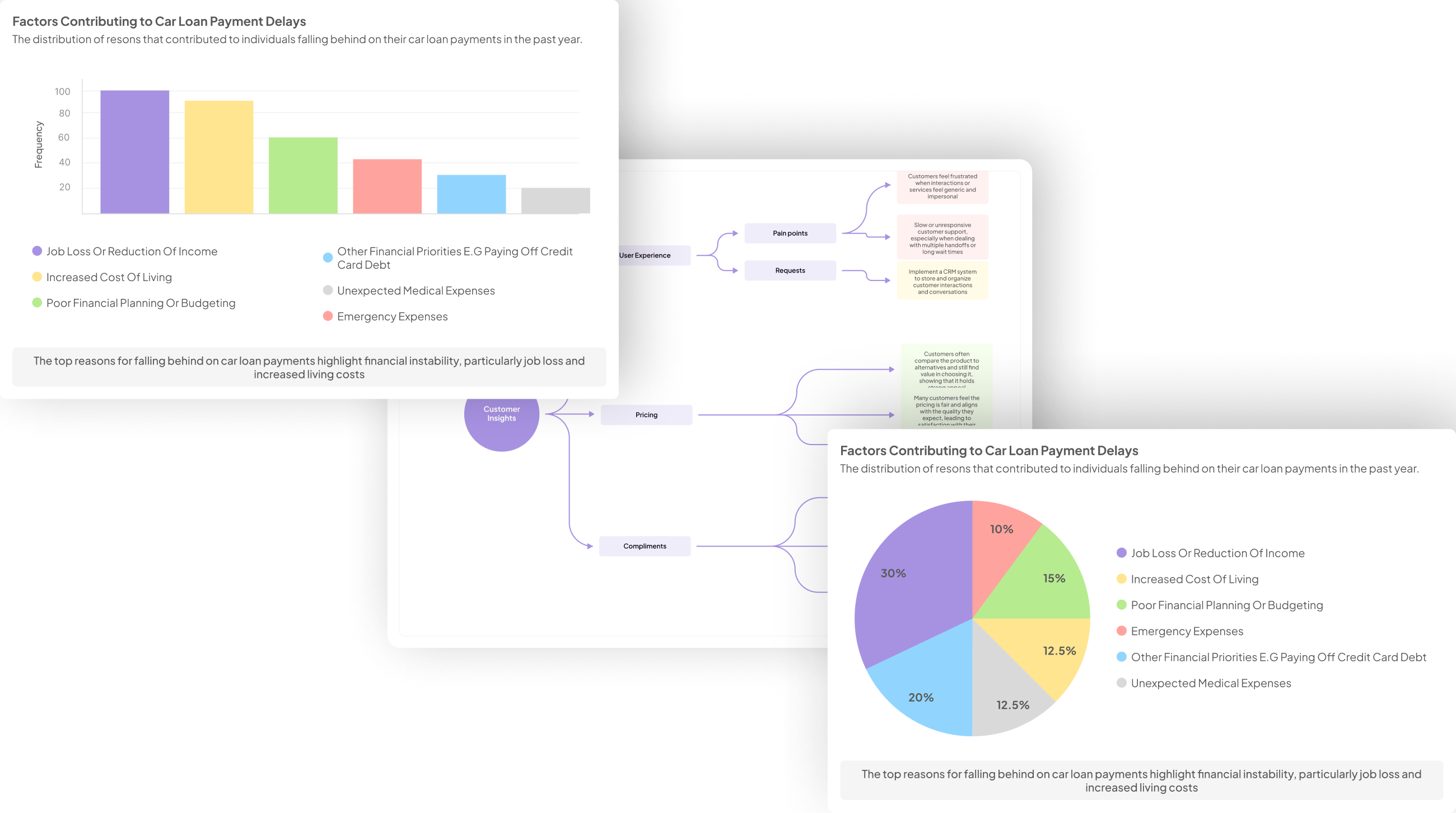
Understanding Text Analytics AI: A Foundation for Optimizing Text Insights
Text Analytics AI serves as a critical foundation for optimizing text insights in today’s data-driven business world. By transforming unstructured text into structured insights, businesses can uncover valuable trends and sentiments that drive decision-making. Organizations can utilize these insights to enhance customer engagement, minimize operational inefficiencies, and inform strategic planning.
To effectively optimize text insights, it is essential to understand the various components of Text Analytics AI. This includes familiarizing oneself with key technologies and methodologies, such as natural language processing and machine learning algorithms. Moreover, organizations should prioritize the organization and storage of textual data to facilitate effective analysis. By implementing best practices in text analytics, businesses can not only extract relevant insights but also ensure they are actionable, paving the way for informed decisions and increased competitiveness in the market.
The Role of Text Analytics AI in Modern Business
Text Analytics AI plays a pivotal role in modern business by transforming vast amounts of unstructured text data into valuable insights. Companies today generate more customer feedback and interactions than ever, and understanding this data has become a competitive advantage. Text Analytics AI helps businesses identify patterns and trends from customer conversations, enabling them to make informed decisions quickly.
To optimize text insights, organizations should focus on two main areas. First, they need to streamline their data gathering processes, ensuring they capture all relevant text inputs. This can involve using advanced tools that facilitate the collection and categorization of customer feedback. Second, businesses must prioritize turning these insights into actionable strategies. This means developing a framework that translates analytical findings into concrete business actions, rather than merely gathering data for the sake of it. By implementing these practices, businesses can remain agile and responsive in a fast-evolving market.
- Leveraging Text Data: Essential Business Intelligence
Text data is increasingly recognized as a crucial asset for gaining business intelligence. By understanding customer feedback, interactions, and sentiments, organizations can draw meaningful conclusions that guide decision-making. Optimizing text insights allows businesses to transform raw text data into actionable intelligence. This process entails analyzing customer communications, feedback surveys, and other textual interactions to uncover patterns and trends reflective of customer preferences.
To effectively harness text data, companies should adopt a systematic approach. First, they need to ensure that the text data collected is relevant and comprehensive. Next, using advanced text analytics tools can automate the extraction of significant insights from these data sets. By doing so, organizations can identify pain points, understand consumer desires, and enhance overall customer experiences. Through these efforts, businesses can create strategic initiatives that lead to improved outcomes and stronger customer relationships.
- Transforming Text to Actionable Insights
Transforming raw text into actionable insights is crucial for businesses aiming to thrive in a data-driven world. By employing effective text analytics, organizations can uncover hidden trends and patterns that inform strategic decision-making. This process begins with data collection, where capturing diverse textual data—such as customer feedback and interviews—serves as the foundation for generating insights.
To effectively transform text into insights, consider these key steps: First, leverage advanced analytics tools to analyze conversations and documents. This allows for the identification of common pain points and customer desires. Next, employ visualization techniques to present insights clearly, making it easier for teams to grasp the findings. Lastly, integrate these actionable insights into business strategies to foster innovation and improve customer experiences. By optimizing text insights, businesses not only enhance their understanding of customer needs but can also make informed decisions that drive growth.
Optimizing Text Insights: Strategies and Best Practices
Optimizing text insights begins with understanding your business's specific needs and the types of insights you want to extract. Start by clearly defining key business objectives, such as improving customer satisfaction or increasing operational efficiency. Once these objectives are established, ensure that you choose the appropriate tools and technologies that align with your goals. This targeted approach will provide a stronger foundation for analyzing text data effectively.
Next, focus on data quality to enhance the insights you extract. Clean, reliable data is critical for meaningful analysis. Implement robust data validation and cleaning techniques to ensure accuracy in your results. By prioritizing data integrity, you can significantly improve the outcomes of your text analytics initiatives. Ultimately, applying these strategies and best practices will help your business transform text analytics into actionable insights, paving the way for informed decision-making and strategic growth.
Evaluate Performance on Customer Calls for Quality Assurance.
Step-by-Step Guide to Implementing Text Analytics AI
Implementing Text Analytics AI requires a structured approach to maximize its effectiveness. The first step is to identify key business objectives that you want to achieve. Understand what specific insights you seek, whether it’s enhancing customer experiences, insights into market trends, or improving operational efficiencies. Ensure that these objectives align with your overarching business goals.
💬 Questions about Text Analytics AI: Best Practices for Businesses?
Our team typically responds within minutes
Next, selecting the right tools and technologies is crucial. Explore various text analytics platforms that cater to your unique requirements. Look for features such as natural language processing, sentiment analysis, and integration capabilities. Additionally, considering user-friendliness will ensure that your team can easily utilize the tools. As you implement these technologies, continuously assess their performance against your identified objectives to ensure you are indeed optimizing text insights effectively. By following these steps, businesses can harness the full potential of text analytics for better decision-making.
- Step 1: Identify Key Business Objectives
Identifying key business objectives is the cornerstone of optimizing text insights. Businesses must begin by clarifying their goals, ensuring each objective aligns with the overall vision and mission. This clarity will not only guide the implementation of text analytics but also ensure that the insights generated are relevant and actionable.
Start by evaluating the specific areas where text analytics can bring value, such as customer feedback, market trends, or operational efficiency. Establish clear metrics for success, considering aspects like increased engagement, improved customer satisfaction, or enhanced decision-making. By systematically identifying these objectives, businesses can effectively channel their efforts toward meaningful insights that drive growth and innovation. This foundational step is essential for harnessing the full potential of text analytics AI in a competitive landscape.
- Step 2: Select the Right Tools and Technologies
The selection of appropriate tools and technologies is a pivotal step in optimizing text insights. When it comes to text analytics AI, not all tools are created equal. You must evaluate your specific needs, considering factors like scalability, user-friendliness, and integration capabilities. A robust analytics platform can effectively process large volumes of text data and deliver actionable insights. This ability is critical for businesses looking to enhance customer understanding and drive decision-making.
Additionally, your choice of technology should align with your business objectives. You might consider tools that offer advanced features such as sentiment analysis, topic modeling, and natural language processing. These technologies can significantly improve your data analysis capabilities. By ensuring that you select the right tools, you set the groundwork for a successful text analytics implementation, ultimately positioning your business to leverage the full potential of its text data.
How to Ensure Data Quality and Reliability
Ensuring data quality and reliability is critical when optimizing text insights for decision-making. Clean and accurate data serves as the foundation for effective text analytics, allowing organizations to derive valuable insights. One effective strategy is to establish robust data validation processes. These processes should include regular audits of data sources, ensuring that inputs are correct before analysis begins. A thorough understanding of data formats and structures also aids in avoiding discrepancies, which can lead to flawed insights.
Additionally, implementing data cleansing techniques is vital for maintaining data integrity. This involves identifying and correcting errors, such as duplicates and inconsistencies. Utilizing automated tools for these tasks can enhance efficiency and accuracy. Businesses must prioritize these quality assurance practices to ensure the information derived from text analytics is reliable and actionable. Ultimately, investing time and resources in data quality significantly improves the impact of decision-making processes within the organization.
- Importance of Clean Data in Text Analytics
Clean data is fundamental for achieving reliable outcomes in text analytics. When businesses utilize text data without ensuring its quality, the insights generated can be misleading, leading to poor decision-making. Inaccurate or corrupted data can distort trends and analytics, making it challenging to optimize text insights effectively. Organizations must prioritize data cleanliness to enhance their understanding of customer sentiments and market trends.
To maintain data integrity in text analytics, consider implementing several key practices. First, establish thorough data validation protocols to identify inconsistencies. Regular cleaning processes, such as removing duplicates and correcting errors, play a vital role in maintaining high-quality datasets. Additionally, training teams on best practices for data entry can significantly reduce human errors. By focusing on these techniques, businesses can optimize text insights and make informed strategic decisions based on accurate, actionable information.
- Techniques for Data Validation and Cleaning
Data validation and cleaning are critical processes in optimizing text insights. For businesses to fully harness the power of text analytics AI, ensuring the quality of the data used is essential. This involves systematically identifying any inconsistencies, errors, or redundancies within the text data. Utilizing techniques such as regular expressions can help detect patterns and anomalies in the data, making it easier to standardize inputs across datasets.
Another technique for data cleaning is deduplication, which removes duplicate records that can skew insights. Additionally, employing natural language processing tools can enhance data validation by automatically correcting spelling and formatting issues. Ultimately, these practices not only safeguard the integrity of your datasets but also enhance the accuracy of the insights generated. By actively engaging in these techniques, businesses can leverage text analytics AI to gain actionable insights and make informed decisions based on reliable data.
Conclusion: Embracing Text Analytics AI for Optimized Business Insights
The integration of Text Analytics AI into business practices marks a significant step toward optimizing text insights for enhanced decision-making. Businesses can extract meaningful patterns and sentiments from raw data, leading to improved strategies and customer experiences. This technology empowers teams to quickly analyze vast amounts of text information, transforming previously opaque insights into clear, actionable outcomes.
Embracing Text Analytics AI not only streamlines operations but also fosters an environment where insights drive innovation. As companies increasingly rely on data to guide their workflows, understanding how to effectively utilize AI tools will be crucial. By prioritizing optimized text insights, organizations can remain competitive and responsive in an ever-evolving marketplace.
💬 Questions about Text Analytics AI: Best Practices for Businesses?
Our team typically responds within minutes