In today’s fast-paced environment, optimizing call analytics in call center phone data is more crucial than ever. Businesses strive to enhance customer service and operational efficiency, needing reliable insights to transform interactions into actionable strategies. Call Analytics Optimization serves as a key component for identifying trends, evaluating performance, and improving training effectiveness within your team.
By implementing advanced analytics techniques, organizations can analyze caller behavior and issues in real-time. This enables managers to tailor training programs effectively and streamline processes. Ultimately, focusing on Call Analytics Optimization equips call centers with the tools needed to enhance customer satisfaction and drive operational improvements.
Generate visualizations from your qualitative data. At Scale.
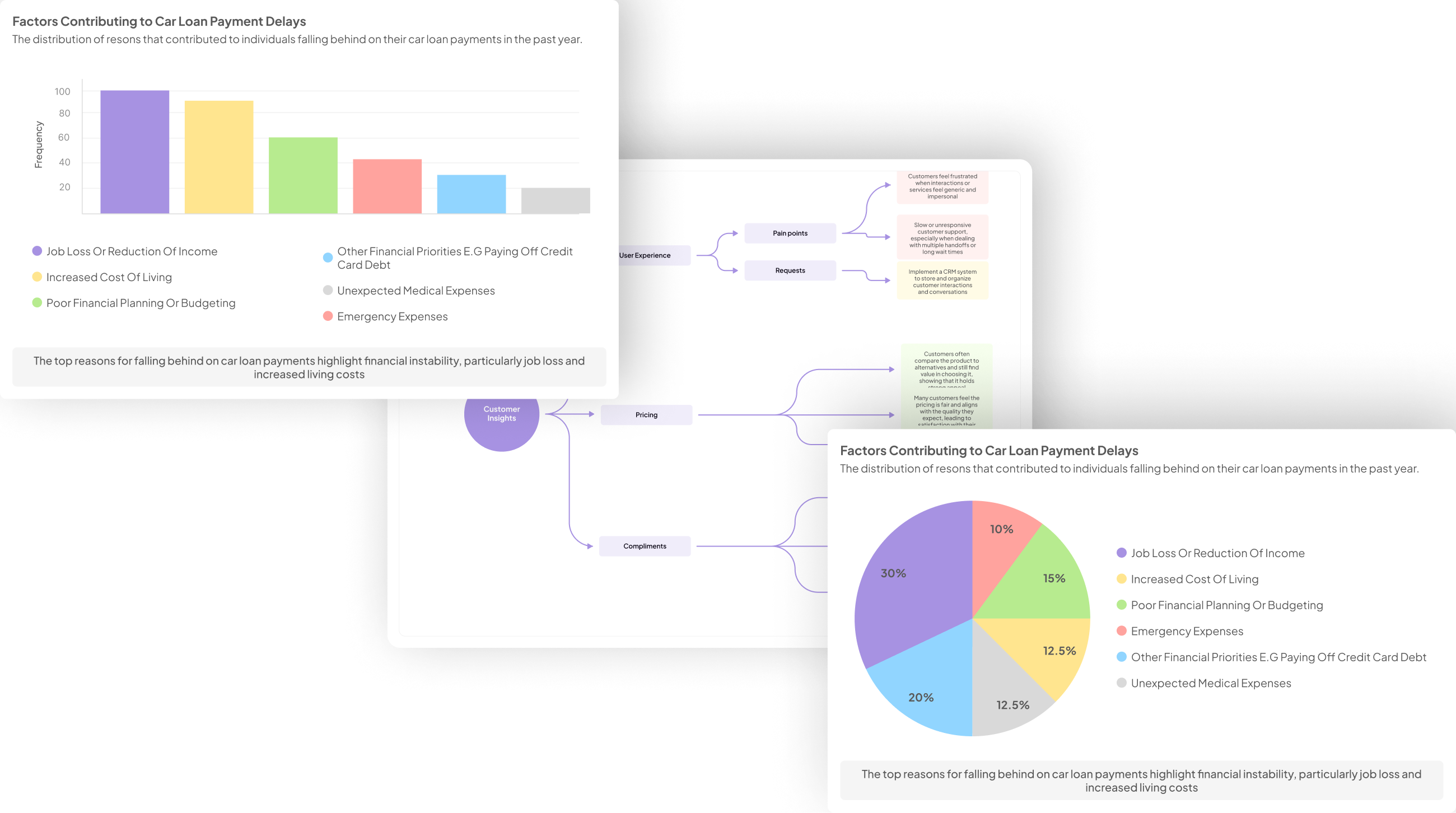
Understanding Call Analytics Optimization Metrics
Understanding Call Analytics Optimization Metrics is crucial for enhancing the effectiveness of call center operations. It involves assessing various metrics that inform strategies for training and customer interaction. By focusing on Call Analytics Optimization, organizations can derive key insights from call data, leading to improved customer service representative (CSR) performance and overall customer satisfaction.
Key metrics include call duration, resolution rates, and customer feedback scores. By regularly evaluating these indicators, managers can identify trends and areas needing improvement. For instance, if an analysis reveals longer call durations with certain CSRs, it may signify a need for additional training. Additionally, understanding common customer queries allows for better alignment of training programs to meet evolving needs. The ultimate goal of Call Analytics Optimization is to utilize data to enhance the overall customer experience while streamlining call center efficiency.
The Role of Key Performance Indicators (KPIs)
Key Performance Indicators (KPIs) are essential for measuring the effectiveness of call center operations. They serve as quantifiable metrics that allow teams to assess their performance and identify areas for improvement. An effective implementation of KPIs can enhance Call Analytics Optimization, enabling call centers to understand customer interactions and streamline processes more efficiently.
There are several critical KPIs that call centers should monitor. These include Average Handle Time (AHT), first call resolution (FCR) rate, customer satisfaction score (CSAT), and call volume. Average Handle Time helps assess the efficiency of agents, while FCR helps gauge how effectively issues are resolved on the first contact. Customer satisfaction scores reflect client perceptions and highlight areas needing attention, and call volume metrics provide insight into staffing needs. By regularly analyzing these KPIs, call centers can make informed decisions, ultimately improving overall performance and customer experiences.
Analyzing Call Duration and Volume
Analyzing call duration and volume is crucial for understanding call center performance. By examining these metrics, organizations can identify trends, efficiency gaps, and customer behavior patterns over time. A clear analysis of call duration helps determine if calls are resolved promptly, while call volume metrics indicate busy periods and workforce requirements. Both elements are integral for ensuring that the call center operates smoothly.
To effectively analyze these metrics, consider the following steps. First, collect data consistently on call lengths and the number of calls received. This foundational step allows you to build a comprehensive database for evaluation. Second, analyze the data to spot patterns and anomalies. For example, longer calls may suggest complex customer issues or inadequate training for staff. Finally, use insights gained from the data to optimize workflows and improve service quality. By focusing on call analytics optimization, organizations can enhance customer satisfaction and operational effectiveness.
Generate Journey maps, Mind maps, Bar charts and more from your data in Minutes
Optimizing Call Center Processes with Phone Data Analytics
Optimizing call center processes with phone data analytics transforms how customer interactions are managed. By focusing on Call Analytics Optimization, centers can efficiently evaluate employee performance and customer satisfaction. One key area of focus involves employing advanced algorithms to analyze call patterns, which can reveal insights into frequently asked questions and recurring issues. This data not only aids in improving training programs for customer service representatives but also enhances the overall customer experience.
Additionally, optimizing processes entails systematic data collection and analysis. Gather information from various channels, such as phone calls and customer feedback, to ensure a comprehensive understanding of performance metrics. Once the data is collected, it should be processed and cleaned to eliminate inconsistencies. Finally, applying advanced data analysis methods can lead to actionable insights, enabling call centers to continuously refine their operations. By embracing these strategies, organizations can create a more efficient and responsive customer service environment.
Step 1: Data Collection Techniques
Step 1 involves gathering all relevant data to set the foundation for effective call analytics optimization. Begin by identifying the channels through which customer interactions occur, such as phone calls, emails, and chat. Each channel presents unique data types that can enhance understanding of customer behavior and service quality. Documenting these interactions is crucial for building a comprehensive dataset that covers various metrics, including call duration, customer inquiries, and agent responses.
In the next phase, employ tools to record and store this data securely. Utilizing software specifically designed for call center analytics can streamline data collection and ensure accuracy. Automated systems help minimize human errors while capturing essential details, such as call recordings and transcripts. As you compile this data, consider various methods of analysis that will inform your strategies moving forward. By establishing robust data collection techniques, organizations can significantly improve their call analytics optimization efforts.
Step 2: Data Processing and Cleaning
Data processing and cleaning is a critical step in optimizing call analytics. Initially, this phase involves the careful examination of raw call data to identify inconsistencies and errors. When data is gathered from various sources, discrepancies can arise, leading to misleading conclusions. A thorough cleaning process helps in rectifying these issues, ensuring that only accurate and relevant information is retained for analysis.
Furthermore, standardizing the data format is essential for effective analysis. This means converting different data types into a uniform structure. For example, time stamps must be consistent, and duplicate entries should be removed. By ensuring that the data is clean and well-organized, call analytics optimization can deliver actionable insights. Proper processing not only enhances data reliability but also facilitates deeper understanding of call patterns, customer feedback, and operational efficiencies.
Step 3: Advanced Data Analysis Methods
In Step 3: Advanced Data Analysis Methods, the focus of the discussion centers on employing sophisticated analytical techniques to optimize call analytics. This phase is vital, as it allows organizations to dig deeper into call data and extract actionable insights. Advanced methodologies enable analysts to identify patterns, prioritize customer feedback, and compare performance metrics across different datasets. For instance, leveraging AI tools can enhance data interpretation, enabling teams to visualize positive and negative sentiment in calls effectively.
To harness the full potential of these methods, consider the following techniques:
- Sentiment Analysis: Utilize natural language processing to gauge customer emotions.
- Comparative Analysis: Analyze calls across different locations or timeframes to identify trends.
- Volume Analysis: Break down call data by categories such as issues or customer demographics to detect patterns.
- Predictive Modeling: Forecast future call trends or customer behavior based on historical data.
These advanced data analysis approaches enhance call analytics optimization by transforming raw data into strategic insights, ultimately driving improved customer satisfaction and operational efficiency.
Conclusion: Mastering Call Analytics Optimization
Mastering call analytics optimization centralizes the effectiveness of your call center. By harnessing data-driven insights, teams can discern patterns, improve training processes, and enhance customer service. Understanding the analytics not only showcases frequent customer inquiries but also aligns staff training to meet those specific needs. This proactive approach paves the way for improved performance within your organization.
Furthermore, optimizing these analytics leads to a deeper comprehension of customer interactions. By analyzing call duration, volume, and caller sentiment, your team can implement strategic improvements. Embracing call analytics optimization transforms raw data into actionable insights, ultimately driving your call center towards enhanced productivity and customer satisfaction.
Generate visualizations from your qualitative data. At Scale.
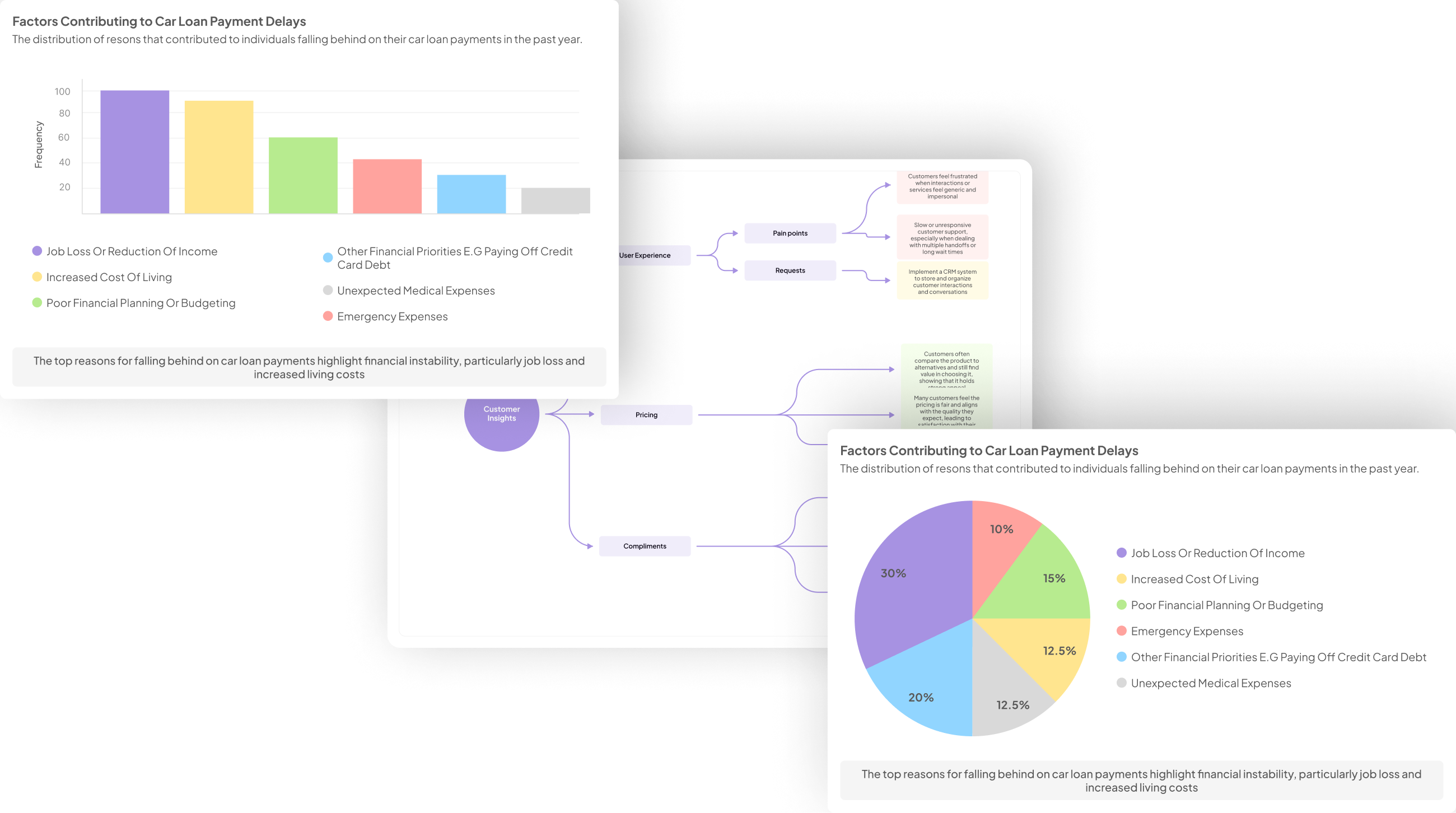