Sentiment Analysis Evaluation Metrics: Key Points
-
Bella Williams
- 10 min read
Sentiment Evaluation Metrics play a crucial role in understanding how effectively a message connects with its audience. From product feedback to social media interactions, the ability to gauge sentiment allows businesses to adjust strategies accordingly. By employing varied evaluation methods, organizations can explore emotions behind opinions, enabling them to foster better customer engagement and satisfaction.
In this section, we will delve into essential aspects of sentiment evaluation. We will explore the different metrics used to assess and interpret sentiment data. Understanding these metrics can help businesses make informed decisions, enhancing their approach to customer relationships and overall brand perception.
Generate visualizations from your qualitative data. At Scale.
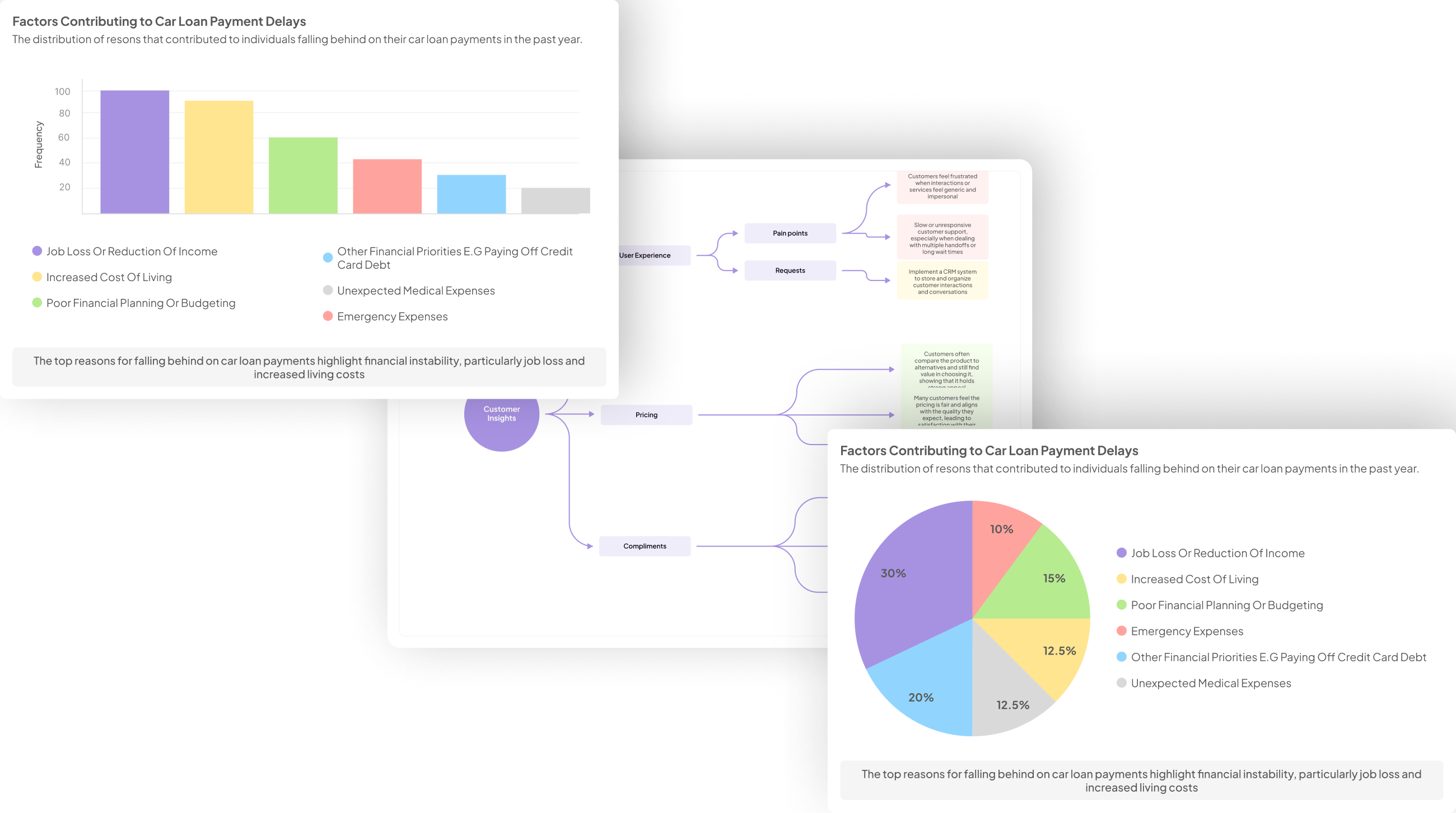
The Importance of Sentiment Evaluation Metrics in NLP
Sentiment Evaluation Metrics are crucial in Natural Language Processing (NLP) as they help gauge the emotional tone behind words. Accurate sentiment analysis is increasingly important for businesses striving to understand customer opinions. By measuring sentiments effectively, organizations can make informed decisions and tailor their strategies to enhance customer experiences.
Incorporating metrics like precision, recall, and F1-score allows researchers to evaluate the performance of sentiment analysis models. Precision measures the accuracy of positive predictions, while recall assesses the ability to identify actual positive cases. The F1-score serves as a balanced metric that combines both precision and recall, offering a more comprehensive view of model effectiveness. These metrics not only facilitate better models but also foster greater trust in automated sentiment analysis systems, empowering organizations to act on insights with confidence.
Key Metrics for Sentiment Analysis
Key Metrics for sentiment analysis are essential for evaluating public opinion, customer satisfaction, and brand perception. To effectively measure sentiment, several critical metrics can be employed. These include accuracy, precision, recall, and F1 score. Each metric serves a unique purpose in sentiment analysis evaluation metrics, providing insights into the performance of the analytical model used.
Accuracy measures the proportion of correct predictions made by the model. Precision indicates how many of the positive predictions were genuinely positive. Recall assesses the model’s ability to identify all relevant instances. Finally, the F1 score balances precision and recall, delivering a single score that captures the model’s overall effectiveness. By analyzing these key metrics, businesses can refine their sentiment analysis processes and gain deeper insights into customer attitudes and feedback. Understanding these metrics promotes better decision-making and enhances strategic marketing efforts.
Sentiment Evaluation Metrics: Precision, Recall, and F1-Score
In sentiment evaluation metrics, precision, recall, and F1-score play critical roles in assessing the effectiveness of sentiment analysis models. Precision measures the accuracy of positive predictions, indicating how often positive sentiment predictions are correct. A high precision score means that when the model predicts positive sentiment, it is usually correct, reducing false positives in evaluation.
Recall, on the other hand, gauges the model’s ability to identify all relevant instances of positive sentiment. A high recall indicates that the model captures most of the actual positive sentiments, minimizing false negatives. However, achieving high precision and recall can be challenging; thus, the F1-score serves as a harmonic mean of both metrics. It provides a balanced assessment, particularly useful when there is an uneven distribution of classes. Overall, understanding these key metrics is essential for optimizing sentiment analysis systems and ensuring reliable outcomes.
Precision: Measuring Accuracy of Positive Sentiments
Precision is crucial in evaluating the accuracy of positive sentiments through sentiment analysis. It focuses on the proportion of true positive sentiments identified by the model out of all sentiments labeled as positive. This evaluation provides insights into how well a system distinguishes positive information from other sentiments. By tracking precision, organizations can gauge the effectiveness of their sentiment analysis tools, ensuring that they capture genuine enthusiasm or favorable reviews without false positives.
To measure precision effectively, consider the following aspects. First, the True Positives (TP) represent the number of correctly identified positive sentiments. Second, False Positives (FP) reflect incorrect classifications where negative or neutral sentiments are labeled as positive. The formula for precision is straightforward: Precision = TP / (TP + FP). Maintaining high precision is essential as it not only enhances customer satisfaction but also drives more accurate decision-making based on user feedback. Thus, understanding precision as part of sentiment evaluation metrics is integral to developing reliable insights.
Recall: Capturing All Relevant Sentiments
Recall plays a crucial role in sentiment analysis, as it measures the toolโs effectiveness in capturing all relevant sentiments present in a dataset. A high recall indicates that the analysis accurately identifies most of the positive or negative sentiments expressed, ensuring that important insights are not overlooked. This is especially vital for businesses aiming to understand customer feedback and pinpoint issues, enhancing their decision-making processes.
๐ฌ Questions about Sentiment Analysis Evaluation Metrics: Key Points?
Our team typically responds within minutes
To improve recall, there are a few key strategies one can implement. First, using a broader set of keywords and phrases can help identify varying expressions of sentiment. Second, incorporating advanced natural language processing techniques allows for a more thorough understanding of context and nuances. Finally, continuous updating of sentiment analysis models based on new data ensures they remain effective. Ultimately, maximizing recall in sentiment evaluation metrics leads to more reliable and actionable insights.
Generate Journey maps, Mind maps, Bar charts and more from your data in Minutes
Sentiment Evaluation Metrics: Additional Considerations
In sentiment evaluation metrics, several important factors influence the effectiveness of your analysis. First and foremost, consider the context of the text being analyzed. Sentiment can shift based on cultural and situational factors, making contextual understanding crucial for accurate evaluation. Additionally, the nuances of language, such as sarcasm or idiomatic expressions, can significantly affect sentiment detection.
Another critical consideration is the balance between precision and recall. While high precision ensures the correct classification of positive and negative sentiments, achieving adequate recall ensures that sentiments are not overlooked. Moreover, the choice of evaluation metrics used, such as F1 score or area under the curve (AUC), plays a pivotal role in assessing overall model performance. By addressing these considerations, you can enhance the reliability of sentiment evaluation metrics and the insights they provide.
Accuracy: Overall Effectiveness of the Model
Accuracy serves as a pivotal metric for evaluating the overall effectiveness of sentiment analysis models. High accuracy indicates that the model correctly identifies the sentiment behind textual data, ensuring reliable insights for decision-making. Effective sentiment evaluation metrics not only assess the proportion of correct predictions but also highlight areas for model improvement. For practitioners, understanding accuracy involves examining how well a model performs in diverse contexts, yielding insights that contribute to more informed marketing strategies.
Additionally, while accuracy is significant, it is essential to consider other metrics like precision, recall, and F1 score. These metrics provide a comprehensive view of the modelโs performance, as they capture different perspectives on prediction effectiveness. A well-rounded evaluation strategy enhances trust in the modelโs predictions and allows businesses to make strategic decisions based on robust data. Ultimately, the interplay of these factors results in a clearer picture of a model’s effectiveness in capturing sentiment nuances within text data.
AUC-ROC: Balancing Sensitivity and Specificity
The AUC-ROC curve is crucial for assessing models in sentiment analysis, as it effectively balances sensitivity and specificity. Sensitivity, or true positive rate, represents the model’s ability to correctly identify positive sentiments. Conversely, specificity measures the correct identification of negative sentiments, or true negatives. An ideal model achieves a high AUC score, as this indicates superior performance in distinguishing between positive and negative sentiments.
Understanding the AUC-ROC curve allows researchers and analysts to visualize trade-offs between sensitivity and specificity. A model that favors sensitivity may misclassify negative sentiments, while one focused on specificity might overlook many positive sentiments. By analyzing the AUC-ROC, practitioners can select models that align with their evaluation metrics priorities. This insight is invaluable for ensuring that sentiment analysis solutions provide reliable and actionable results in various applications.
Conclusion: The Role of Sentiment Evaluation Metrics in Model Improvement
Sentiment evaluation metrics play a crucial role in enhancing model performance. By providing valuable feedback, these metrics help identify areas where models may falter, guiding iterative improvements. Continuous assessment allows for better understanding of how well a model captures and interprets the nuances of sentiment in data.
Moreover, the refinement process hinges on effectively utilizing sentiment evaluation metrics. Accurate metrics drive strategic adjustments, ensuring that the model alignment enhances both its predictive capability and reliability. Ultimately, this ongoing evaluation fosters a more robust sentiment analysis framework that can adapt to evolving data landscapes and user expectations.
Generate visualizations from your qualitative data. At Scale.
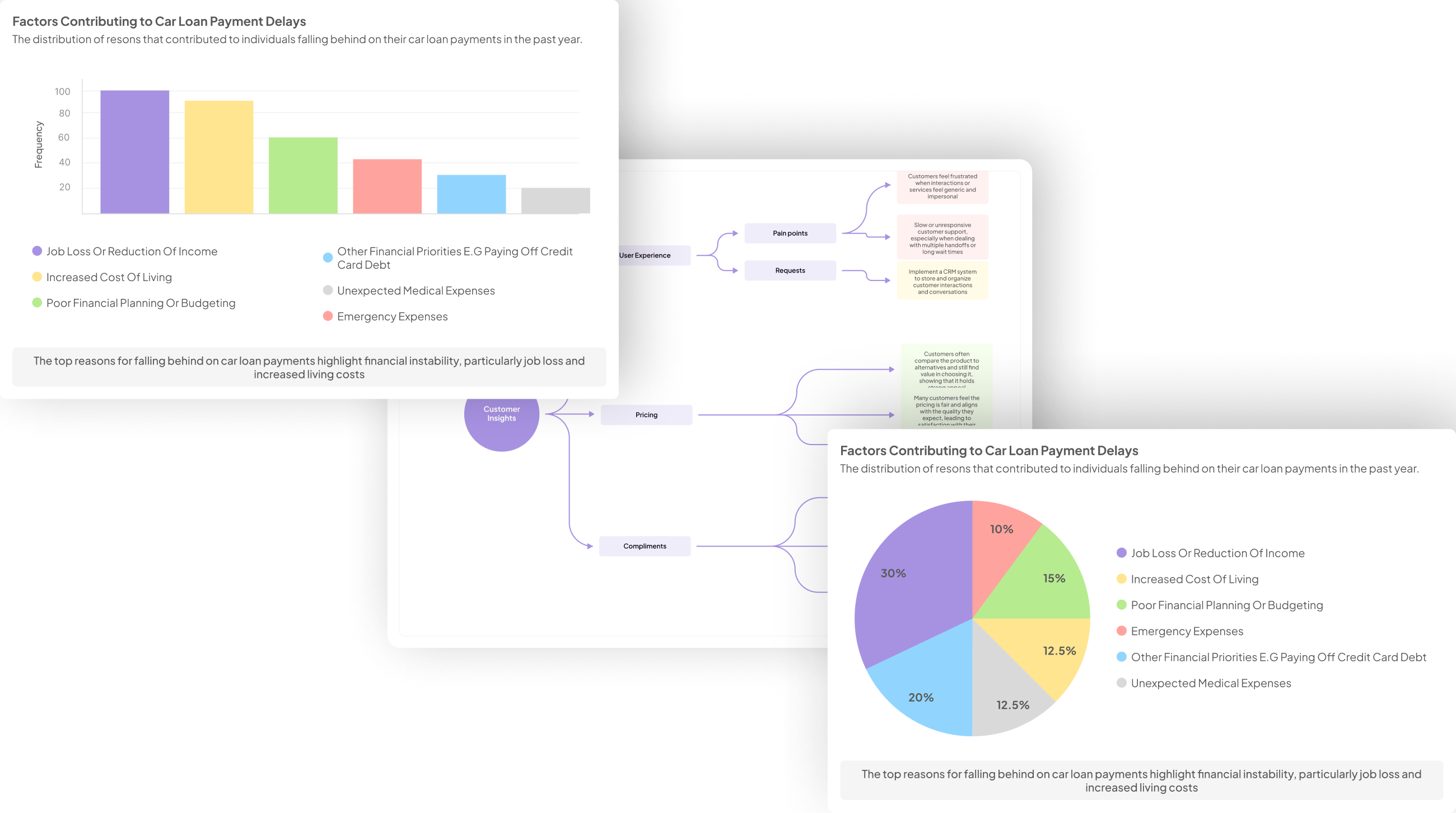
Moving Forward with Sentiment Evaluation Metrics
To effectively progress with sentiment evaluation metrics, it is essential to establish a clear framework and understanding of available tools. Organizations can enhance their analysis by implementing systematic metrics that capture not only quantitative data but also qualitative insights. This dual approach allows for a more comprehensive picture of customer sentiment and satisfaction over time.
Key components to consider include accuracy, precision, and recall. Accuracy measures the overall correctness of sentiment predictions, while precision focuses on the proportion of true positives among predicted positives. Recall, on the other hand, evaluates the ability to identify actual positives within the dataset. Regularly engaging with these metrics not only ensures alignment with business goals but also facilitates timely adjustments to strategies as needed. Fostering this continuous evaluation will ultimately contribute to more sophisticated sentiment analysis practices.
๐ฌ Questions about Sentiment Analysis Evaluation Metrics: Key Points?
Our team typically responds within minutes