In today’s fast-paced service environments, ensuring compliance with Service Level Agreements (SLAs) can be a daunting challenge. Support call data emerges as an invaluable asset in this context, providing actionable insights into service performance and customer satisfaction. By effectively analyzing these interactions, organizations can uncover trends and patterns that reveal opportunities for SLA optimization and overall service enhancement.
SLA optimization insights derived from call data empower decision-makers to identify key performance metrics that directly influence compliance. Implementing a structured approach to data collection not only streamlines this process but also minimizes potential errors, creating a reliable foundation for effective analysis. Embracing these insights establishes a pathway for continuous improvement, allowing businesses to meet and exceed SLA expectations while fostering exceptional customer experiences.
Analyze qualitative data. At Scale.
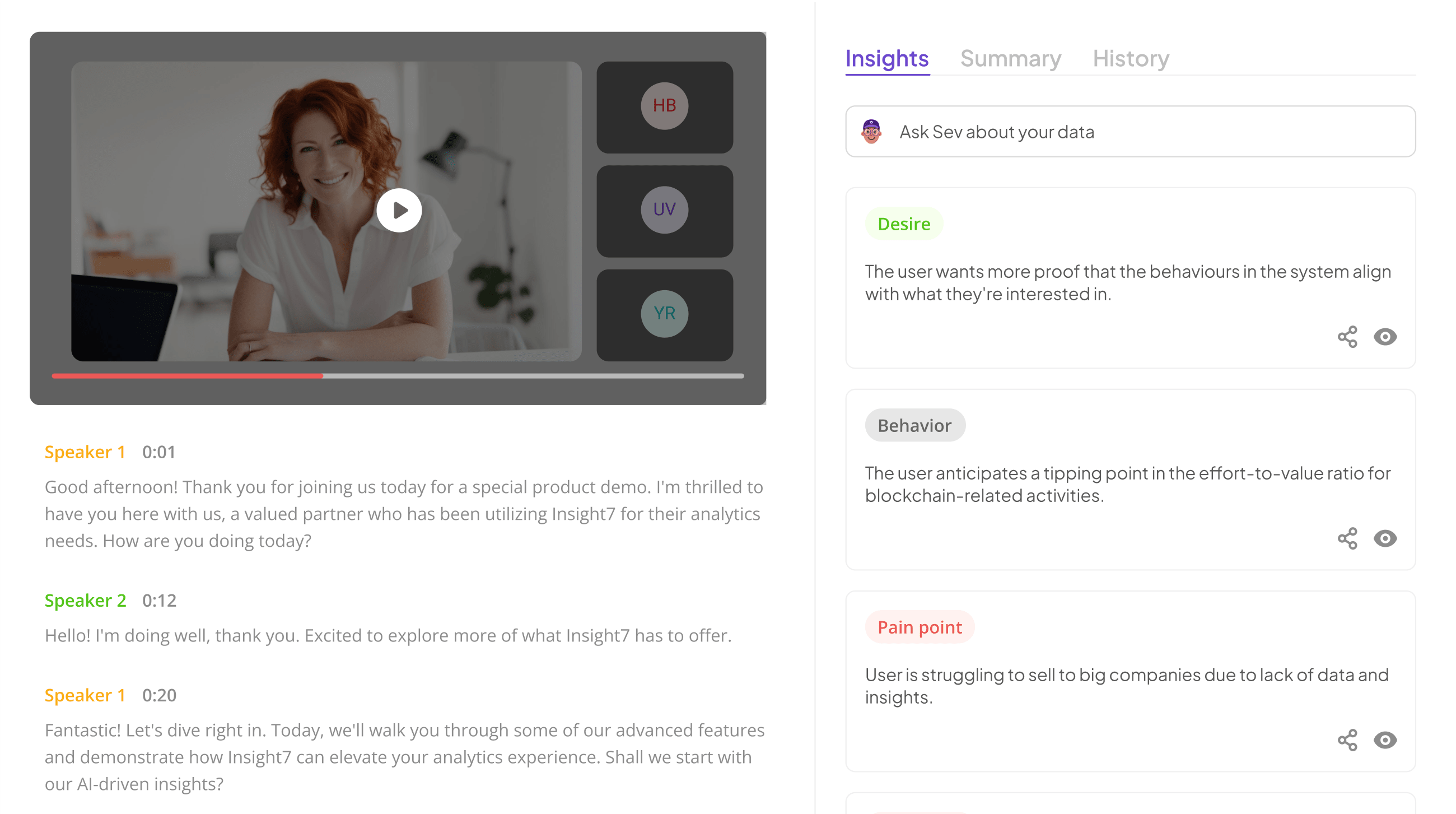
Leveraging SLA Optimization Insights for Data Collection
Effective data collection is essential for meeting service level agreements (SLAs) and improving overall compliance. To harness SLA optimization insights, organizations must start by identifying key performance metrics that directly influence SLA achievements. By carefully selecting these metrics, teams can better capture relevant support call data and measure compliance effectively.
Next, implementing a structured data collection process is crucial for accuracy and reliability. Begin by establishing clear procedures for gathering data, ensuring everyone understands their roles. Utilizing automated systems can minimize errors, allowing for a smooth flow of information. Regularly reviewing data collection methods also helps refine processes, ensuring the information gathered remains relevant. This strategic approach to data collection will ultimately lead to better insights, enabling teams to enhance SLA performance and customer satisfaction. By turning these insights into actionable strategies, organizations can consistently improve their SLA compliance over time.
Identifying Key Metrics for SLA Optimization Insights
Identifying key metrics is essential for gaining actionable SLA optimization insights. Start by defining primary metrics that directly influence SLA compliance. Metrics such as response times, resolution rates, and customer satisfaction scores not only highlight performance trends but also reveal pain points within support processes. Understanding these metrics helps illuminate which areas demand immediate attention and continuous improvement.
Next, focus on capturing call data that is relevant to SLA performance. Implementing tools to systematically track this data can enhance your understanding of adherence to SLAs. By organizing call data effectively, support teams can identify recurring issues, allowing for targeted interventions. Ultimately, having clear visibility into these key metrics equips organizations with the knowledge necessary for informed decision-making and proactive SLA management. These insights are crucial for fostering a streamlined support experience that benefits both customers and the business.
- Define primary metrics influencing SLA compliance.
Understanding the primary metrics that influence SLA compliance is essential for effective service management. These metrics provide critical insights into how well service level agreements are being met and where improvements can be made. Key metrics typically include response time, resolution time, first-contact resolution rate, and customer satisfaction scores. Each of these metrics offers valuable information that can guide strategies for enhancing overall service performance.
Response time measures how quickly support teams acknowledge requests, while resolution time tracks the duration taken to resolve issues. The first-contact resolution rate indicates the proportion of cases resolved during the initial interaction, significantly affecting customer satisfaction. By closely monitoring these metrics, companies can identify trends, pinpoint problem areas, and prioritize resources accordingly. Ultimately, focusing on these primary metrics enables businesses to glean SLA optimization insights, resulting in improved compliance and enhanced customer loyalty.
- Capture call data relevant to SLA performance.
Capturing call data relevant to SLA performance is essential for understanding areas requiring improvement in service levels. First, it’s important to identify the metrics that significantly impact SLA compliance. Track aspects such as response time, resolution time, and customer satisfaction ratings to gather comprehensive insights. These metrics will help you pinpoint areas where enhancements may lead to better SLA performance.
Next, establish a robust system for collecting this data consistently. Use automated tools, such as Insight7, for efficient data transcription and evaluation. This strategy not only reduces the chances of human error but also ensures that the data gathered is accurate and relevant. Furthermore, continuously review and refine your data collection processes to stay aligned with changing customer needs and technological advancements. By accurately capturing relevant call data, you gain powerful SLA Optimization Insights that can guide strategic decisions and bolster service delivery.
Implementing Structured Data Collection Processes
Implementing structured data collection processes is essential for gaining SLA optimization insights from support call data. First, it’s crucial to establish clear and systematic procedures for data collection. This ensures that every piece of information collected contributes meaningfully to SLA compliance objectives. With defined procedures, your team can minimize confusion and enhance the data's reliability.
Next, utilizing automated systems to capture data reduces manual errors and boosts efficiency. Automating data entry and aggregation can significantly streamline the process, allowing your team to focus on analyzing the data rather than collecting it. Finally, regularly reviewing and adjusting your data collection methods is vital. By assessing the effectiveness of your current strategies, you can identify what works best and refine your approach to maximize SLA optimization insights. This cyclical process not only ensures consistent improvements but also fosters a culture of data-driven decision-making within your organization.
- Step-by-step guide to organizing data collection.
To organize data collection effectively, begin by establishing clear procedures tailored to your specific needs. Clearly defined steps help streamline the process and ensure data consistency. Document each procedure meticulously, so all team members understand their roles in data collection. This structured approach enables better tracking and fosters accountability.
Next, incorporate automated systems to minimize human errors. Leveraging technology can enhance the efficiency of your operations while allowing your team to focus on interpreting data rather than manually collecting it. Regularly reviewing and adjusting your data collection processes is essential to adapt to evolving needs. Continually seek feedback on the procedures and implement changes to ensure they remain effective and relevant. By following these steps, you can lay a solid foundation for gathering SLA optimization insights that will drive better compliance and improve overall service quality.
- Step 1: Establish clear data collection procedures.
Establishing clear data collection procedures is essential for effectively utilizing support call data to enhance SLA compliance. Start by defining the data points you need, such as call resolution times, customer feedback, and service level agreement fulfillment. Having a focused intention will help streamline the collection process and enable you to gather relevant SLA optimization insights.
Next, integrate a structured approach to data collection. Create standardized templates and ensure that all team members are trained to input data consistently. This reduces the chance of errors and keeps the information uniform, making it easier to analyze later. Additionally, consider automated systems to facilitate data entry and minimize manual labor. Regularly reviewing these processes will allow you to adapt procedures based on what you learn, ensuring continuous improvement in meeting SLA goals. By establishing strong data collection foundations, you pave the way for impactful analysis and actionable insights.
- Step 2: Utilize automated systems to minimize errors.
To effectively utilize automated systems for minimizing errors in your support call data, it is essential to implement robust technology solutions. Automated systems can streamline data collection, ensuring accurate capture and reducing human error. By automating repetitive tasks, you allow team members to focus on more critical aspects of SLA compliance, such as analyzing the data for insights that truly matter.
Next, consider integrating tools that provide real-time monitoring and analytics. These tools can help identify trends and potential issues before they impact SLA compliance. Additionally, adopting a consistent data entry method through automated systems can improve reliability. This structured approach leads to more actionable SLA optimization insights, enabling your team to respond more efficiently when issues arise. Embracing these automated solutions not only enhances operational efficiency but also fosters a proactive approach to managing service level agreements effectively.
- Step 3: Regularly review and adjust collection processes.
Regular reviews of data collection processes are essential for optimizing Service Level Agreements (SLAs) effectively. Initially, gather insights from support call data to identify any gaps or inefficiencies in your current collection methods. Evaluate the frequency and nature of support requests to understand customer needs better. This understanding will help you adapt your collection mechanisms to enhance SLA compliance.
Adjustments based on this analysis are crucial. For example, if certain metrics consistently fall short, consider refining the related data collection procedures. Regularly schedule review sessions to assess these processes, ensuring they evolve with changing customer dynamics. Moreover, integrate technology that enables real-time monitoring, which can lead to timely adjustments. By continuously revisiting and refining your data collection strategies, you unlock valuable SLA Optimization Insights, ultimately driving customer satisfaction and compliance performance.
Extract insights from interviews, calls, surveys and reviews for insights in minutes
Analyzing Support Call Data for SLA Optimization Insights
Analyzing support call data is essential for uncovering SLA optimization insights. By examining the nuances of call interactions, businesses can identify patterns that directly affect compliance rates. This analysis helps highlight the root causes behind missed SLAs and informs strategic adjustments. Incorporating real-time monitoring techniques allows organizations to act swiftly on actionable insights, enabling proactive compliance management.
Utilizing predictive analytics can significantly enhance understanding of future SLA performance. Developing predictive models with historical call data allows for precise forecasting of potential challenges. Once these models are tested and validated, businesses can apply findings to anticipate SLA risks and opportunities effectively. These combined analytical strategies foster a deeper understanding of customer interactions and ultimately drive higher adherence to SLAs.
Real-Time Data Analysis Techniques
Real-time data analysis techniques are essential for achieving SLA optimization insights from support call data. By implementing real-time monitoring, organizations can track SLA performance as it unfolds. This approach enables the prompt identification of trends and potential issues, allowing for timely intervention. For example, if call wait times begin to spike, teams can quickly deploy additional resources to address the situation.
Integrating real-time data analysis tools further enhances this capability. Utilizing automated systems for data collection and reporting minimizes human error and provides consistent updates. Techniques such as dashboard analytics allow decision-makers to visualize performance metrics instantly. This immediacy empowers teams to make informed decisions that drive SLA compliance continuously. By marrying real-time analysis with effective communication, organizations can effectively manage customer expectations and improve overall service delivery.
- Use real-time monitoring to gain immediate insights.
Real-time monitoring is crucial for gaining immediate insights into SLA compliance. By continuously tracking support call data, teams can quickly identify trends and issues as they arise, allowing for timely interventions. When issues are detected early, organizations can adjust their strategies to bolster SLA compliance and enhance customer satisfaction.
To effectively utilize real-time monitoring, consider the following methods. First, establish key performance indicators (KPIs) that directly relate to SLA metrics. These indicators can include response times, resolution rates, and customer feedback scores. Next, integrate real-time data analysis tools that provide dashboards and alerts to highlight performance issues. Lastly, ensure regular team reviews based on real-time data insights. This practice encourages proactive engagement, enabling the team to address potential SLA breaches before they impact customer experience. By adopting these practices, organizations can harness SLA Optimization Insights to drive continuous improvement in support services.
- Techniques for integrating real-time data analysis tools.
Integrating real-time data analysis tools is crucial for optimizing service level agreements (SLAs) through support call data. To effectively implement these tools, begin by identifying the specific data sources that directly impact SLA compliance. Monitor call data continuously to capture trends and detect anomalies, enabling prompt responses to potential issues.
Next, integrate robust real-time analytics tools into your existing systems. These tools should provide dashboards showcasing key performance indicators, helping stakeholders easily visualize areas needing attention. Regularly adjust algorithms based on new data inputs to enhance accuracy in insights. Furthermore, consider implementing automated alerts for workflow disruptions to facilitate quick resolutions. By utilizing these techniques, organizations can transform raw support call data into actionable SLA optimization insights, driving improvements and ultimately enhancing customer satisfaction.
Implementing Predictive Analytics for SLA Optimization
Implementing predictive analytics for SLA optimization involves using historical support call data to foresee potential compliance issues. By developing predictive models, you gain valuable SLA optimization insights that can enhance operational effectiveness. Start by analyzing past performance metrics to identify patterns and trends within your support calls. This initial analysis sets the stage for creating models that can accurately forecast future SLA outcomes.
Once you establish your predictive models, the next step is testing and validating them to ensure reliability. This process allows you to fine-tune your predictors, improving their accuracy in identifying risk areas. By routinely applying these insights, your organization can proactively address potential SLA violations before they affect service quality. Overall, predictive analytics empowers teams to make informed decisions, driving continuous improvement in SLA compliance and enriching customer satisfaction along the way.
- Step-by-step guide to predictive analytics.
To effectively implement predictive analytics for SLA optimization, begin by collecting historical support call data. This data serves as the foundation for developing predictive models, which can reveal trends and potential risks related to SLA compliance. Start this process by analyzing existing call patterns, response times, and resolution rates. Each element offers crucial insights into areas that require improvement.
Next, it's important to validate your predictive models against real-world scenarios. This can involve testing your models with a subset of new data and refining them as necessary. Finally, apply your findings to predict future SLA performance. This process will allow for proactive decision-making, ultimately leading to enhanced SLA compliance. By tailoring these insights to your specific support strategies, organizations can better allocate resources and enhance customer satisfaction. Employing a systematic approach to predictive analytics will lead to ongoing enhancements in SLA performance and create more effective support frameworks.
- Step 1: Develop predictive models using historical data.
Developing predictive models using historical data is essential for understanding patterns and trends that influence SLA compliance. This step involves analyzing past support call data to identify factors that predict SLA performance. By understanding these elements, organizations can forecast potential compliance risks and opportunities for improvement.
First, gather relevant historical data, including call volumes, response times, and resolution rates. Next, utilize statistical tools to create models that analyze these variables. It’s crucial to evaluate the models' effectiveness through rigorous testing and validation, ensuring they accurately predict SLA indicators. Ultimately, applying these predictive models enhances decision-making, providing SLA optimization insights that guide strategic interventions to improve service levels while effectively managing customer expectations.
- Step 2: Test and validate predictive models.
To effectively test and validate predictive models, one must first ensure that the models are built on robust historical data. This process includes analyzing call data to refine the models, allowing for greater accuracy in predictions. Once the analysis is thorough, testing the models against existing service-level agreements (SLAs) can reveal their strengths and weaknesses. Validation involves comparing the model's predictions with actual outcomes to assess performance and improve its reliability over time.
Next, it is essential to continuously monitor the models as new data comes in. This ongoing evaluation helps identify shifts in customer behavior and possible changes in SLA compliance. By doing so, organizations can proactively adjust their strategies and enhance SLA Optimization Insights. Ultimately, a strategic approach to testing and validating predictive models not only improves accuracy but also drives better customer satisfaction and service quality.
- Step 3: Apply findings to forecast SLA risks and opportunities.
To effectively forecast SLA risks and opportunities, the findings from your support call data must be applied comprehensively. Start by identifying patterns from historical data that indicate potential vulnerabilities in your SLA. Evaluating these patterns will allow you to anticipate problem areas before they escalate, giving you the ability to implement proactive measures. This approach not only mitigates risks but can also unveil opportunities for service improvements.
Next, distill your insights into actionable strategies for optimization. Consider adjusting your response times or refining communication protocols to enhance customer training on expected service levels. By mapping out potential risks and opportunities directly linked to SLA performance, you create a framework that prioritizes continuous improvement. With a clear understanding of these dynamics, your organization can enhance SLA compliance and foster better customer relationships, ultimately driving greater operational efficiency.
Conclusion: Harnessing SLA Optimization Insights for Continuous Improvement
The journey towards continuous improvement through SLA Optimization Insights begins with a thorough analysis of support call data. By understanding trends and patterns in this data, organizations can identify strengths and areas for enhancement. This systematic approach allows teams to make informed decisions that lead to better SLA compliance and customer satisfaction.
As insights are harnessed, it becomes essential to develop a robust framework for ongoing assessment and adaptation. Regularly revisiting these insights ensures that teams remain agile in their strategies, continuously refining processes based on actionable data. Thus, leveraging SLA Optimization Insights fosters a culture of improvement, empowering organizations to exceed expectations and drive customer loyalty.