Churn Signal Detection begins with understanding the nuances embedded in customer conversations. Often, customers express their frustrations or dissatisfaction, which may signal potential churn. Recognizing these early indicators can significantly impact customer retention strategies and overall business health. By analyzing customer call transcripts, businesses can uncover valuable insights that reveal sentiment shifts and unmet expectations.
In this section, we will explore how to identify churn signals through linguistic cues and emotional tones present in transcripts. A structured approach to Churn Signal Detection enables proactive engagement, empowering teams to address concerns before they escalate. With the right tools and techniques, businesses can turn these conversations into actionable insights that foster loyalty and enhance customer experience.
Analyze qualitative data. At Scale.
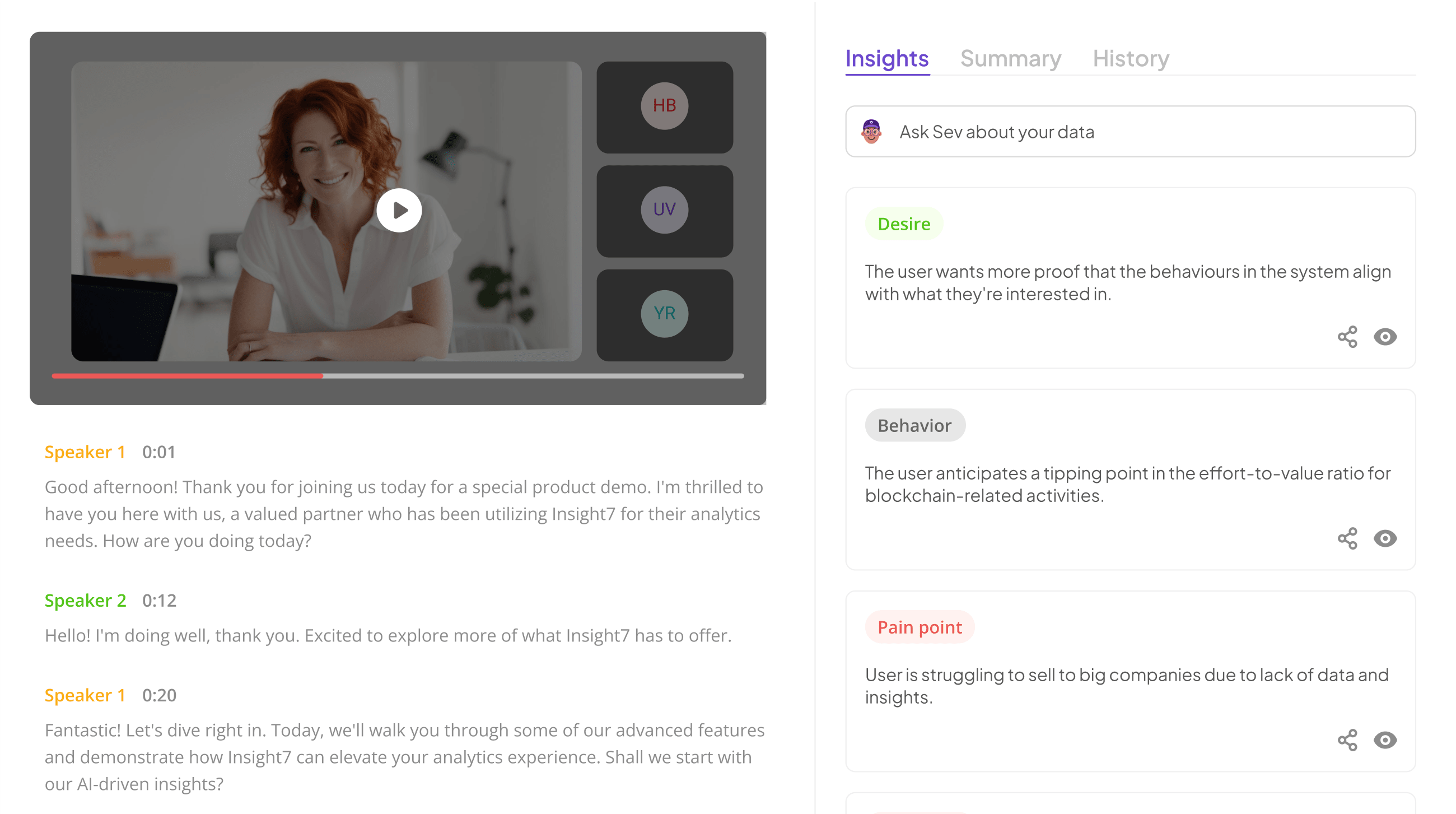
Understanding Key Indicators of Churn Signal Detection
Detecting churn signals in customer call transcripts is crucial for maintaining client relationships. Understanding key indicators helps organizations pinpoint potential issues before they escalate. Early detection allows teams to proactively address concerns and improve customer satisfaction, ultimately reducing churn rates.
Key indicators often include specific linguistic cues and emotional tones expressed during conversations. Words or phrases that reflect frustration or dissatisfaction can signal a risk of churn. Additionally, the overall emotional tone conveyed by customers, whether anxious, frustrated, or disengaged, can be significant. By analyzing these indicators, businesses can gain actionable insights from call transcripts, facilitating timely interventions and enhancing customer experiences. Developing a keen awareness of these indicators enables organizations to tailor strategies that address client needs, fostering loyalty and retention.
Identifying Linguistic Cues for Churn Signal Detection
Linguistic cues are essential for effective churn signal detection in customer interactions. These cues encompass specific words, phrases, and even the tone used by customers during discussions. By carefully analyzing call transcripts, businesses can pinpoint indicators of dissatisfaction or disengagement, which may signal a potential churn. Subtle language differences, such as informal or abrupt phrases, can signal frustration or confusion, while overly formal language may indicate disconnection or a lack of enthusiasm.
To enhance churn signal detection, pay attention to categories that include expressions of dissatisfaction, requests for clarification, or mentions of switching services. For instance, comments like "I'm thinking of leaving" or questions about renegotiating terms are strong signals of churn risks. Additionally, examining emotional tone can further refine detection efforts. By training teams to identify these linguistic patterns, organizations can proactively address customer concerns before they escalate, ultimately improving retention strategies.
Evaluating Emotional Tone for Churn Signal Detection
Understanding emotional tone is crucial for effective churn signal detection. Customers express their satisfaction and dissatisfaction through their tone, providing valuable insights. By analyzing the emotional undertones in customer call transcripts, you can identify potential distress signals. Look for shifts in wording and emotional intensity; these may indicate a customer is unhappy or contemplating leaving.
To enhance your analysis, focus on these aspects:
- Sentiment Variation: Examine changes in positive and negative sentiments over time.
- Expressive Language: Note the use of emotionally charged words that may signify frustration or disappointment.
- Pacing and Tone: Listen for variations in how quickly or slowly a customer speaks, as pacing can reveal their comfort or discomfort.
By evaluating these elements, you can develop a more nuanced understanding of customer emotions, ultimately improving your churn signal detection strategies. Tracking these emotional cues can guide proactive interventions, helping retain customers before they consider leaving.
Extract insights from interviews, calls, surveys and reviews for insights in minutes
Utilizing Tools for Efficient Churn Signal Detection
To effectively address churn signal detection, utilizing the right tools can significantly enhance the accuracy and efficiency of your analyses. By employing advanced software, businesses can streamline the process of identifying early signs of customer dissatisfaction in call transcripts. Tools such as Insight7 can provide valuable quantitative insights, transforming raw data into actionable information.
In addition to Insight7, other tools like Sentiment Analysis APIs, CallMiner, IBM Watson Tone Analyzer, and MonkeyLearn offer diverse functionalities. These platforms analyze not just the spoken words, but also the emotional tones conveyed during customer interactions. By integrating these technologies, companies can parse through large volumes of transcripts swiftly, allowing for timely interventions when churn signals are detected. This proactive approach not only aids in retaining customers but also fosters a deeper understanding of their needs and preferences, ultimately leading to enhanced customer satisfaction.
insight7: A Comprehensive Tool for Churn Signal Detection
In the realm of churn signal detection, insight7 offers a robust solution that goes beyond mere analysis. This comprehensive tool effectively scans customer call transcripts to identify linguistic cues and emotional tones indicative of potential churn. By harnessing advanced algorithms, insight7 can spotlight key interactions where customers express dissatisfaction or disengagement, thereby enabling companies to act before valuable clients decide to leave.
Additionally, insight7's intuitive interface facilitates easy integration into existing workflows. Users can swiftly analyze vast amounts of data, making it easier to uncover patterns and trends related to churn. The ability to detect these early warning signs is crucial for implementing proactive strategies that enhance customer retention. With insight7 in their toolkit, organizations can transform potentially negative experiences into opportunities for improvement, ultimately fostering stronger relationships with their customers.
Other Essential Tools for Analyzing Call Transcripts
To effectively analyze call transcripts, using the right tools is crucial. These tools facilitate deeper insights into customer sentiments and behavioral patterns, essential for churn signal detection. For example, sentiment analysis APIs examine the emotional tone of customer interactions, helping identify potential churn signals more accurately. This technology allows businesses to surface indicators that may not be immediately apparent in raw transcripts.
Additionally, tools like CallMiner and IBM Watson Tone Analyzer provide sophisticated analytics to interpret customer emotions and engagement levels. CallMiner specializes in extracting actionable intelligence from conversations. In contrast, the IBM Watson Tone Analyzer assesses various emotional tones, such as anger or frustration, aiding in pinpointing at-risk customers. Lastly, MonkeyLearn offers an intuitive platform for building custom models, enabling tailored analysis of call transcripts that align with specific churn metrics. Utilizing these essential tools enriches your capability to identify early churn signals effectively.
- Sentiment Analysis API
Integrating a Sentiment Analysis API into your analysis workflow can be a game-changer for detecting early churn signals. This technology leverages natural language processing to evaluate customer emotions expressed during calls. By analyzing the tone and sentiment of conversations, businesses can uncover lingering concerns or dissatisfaction that may lead to churn. Efforts to understand customer sentiment better can proactively address issues before they escalate.
Employing such an API can also streamline the feedback collection process. It identifies patterns in customer sentiments across various touchpoints, providing insights into how customers perceive your product or service. You can utilize specific tools, like CallMiner or IBM Watson Tone Analyzer, to enhance your analysis. This approach not only helps in timely intervention but also aids in aligning services with customer expectations, ultimately driving retention and satisfaction.
- CallMiner
In the realm of churn signal detection, harnessing advanced tools can be a significant game-changer. A well-designed system can analyze customer call transcripts, identifying subtle linguistic cues and emotional tones that hint at potential churn. This is precisely where powerful tools like CallMiner come into play. These tools employ sophisticated algorithms to assess conversations, extracting meaningful patterns that reflect customer sentiments and concerns.
By scrutinizing customer interactions, such platforms can pinpoint specific phrases or emotional dips that often precede cancellations. This proactive approach enables businesses to react early, addressing potential dissatisfaction before it escalates. Companies can monitor customer feedback in real-time, ensuring that they stay ahead of churn cases. Ultimately, using tools like CallMiner enhances a business's ability to refine engagement strategies, improve customer satisfaction, and reduce the risk of losing valued clients.
- IBM Watson Tone Analyzer
The IBM Watson Tone Analyzer offers a powerful solution for identifying emotional tones in customer call transcripts. This tool uses advanced machine learning algorithms to analyze text and extract nuanced data related to emotional expressions. By assessing the emotional tone conveyed in customer interactions, businesses can uncover underlying issues that may signal potential churn. A thorough examination of these emotional cues allows organizations to proactively address customer concerns before they escalate into lost business.
Employing the Tone Analyzer as part of churn signal detection enables companies to recognize distress signals. Understanding the emotional context of conversations can help identify dissatisfaction and disengagement, allowing companies to tailor their responses effectively. This proactive approach to customer care can ultimately strengthen relationships, enhance satisfaction, and improve retention rates. Overall, utilizing innovative tools like the IBM Watson Tone Analyzer contributes significantly to informed strategies for enhancing customer loyalty.
- MonkeyLearn
MonkeyLearn serves as a valuable resource in the realm of churn signal detection, particularly when analyzing customer call transcripts. With its powerful capabilities in natural language processing, this tool transforms vast amounts of unstructured data into insights that reveal potential churn indicators. By harnessing machine learning algorithms, businesses can decode customer sentiment and identify linguistic cues signaling dissatisfaction or disengagement.
Taking advantage of MonkeyLearn enables organizations to streamline their analysis. It allows businesses to extract key phrases that may suggest a higher risk of churn, such as expressions of frustration or repeated requests for assistance. The toolโs ability to categorize and visualize data helps teams prioritize follow-up actions. Ultimately, leveraging such functionalities helps teams proactively address customer needs, fostering a better overall experience while minimizing the likelihood of churn.
Conclusion: Mastering Churn Signal Detection to Prevent Customer Loss
To effectively combat customer churn, mastering churn signal detection is essential. By analyzing customer call transcripts, businesses can identify the subtle cues that indicate a potential loss. Recognizing linguistic patterns and emotional tones in conversations allows teams to intervene before a customer decides to leave. Instead of waiting to react after a cancellation occurs, proactive measures can be implemented by addressing the root issues identified through these signals.
Preventing customer loss is not just about responding to complaints; it involves understanding the underlying challenges customers face. By utilizing comprehensive tools for churn signal detection, organizations can create strategies that resonate with their customersโ needs. Ultimately, honing these skills not only secures customer loyalty but also fosters a deeper relationship with them, ensuring long-term success.