AI Feedback Analysis in customer calls is transforming how businesses understand their clients. Imagine a world where every conversation is a goldmine of insights, revealing customer sentiments and preferences instantly. This is the promise of AI-powered analysis, which streamlines the process of turning spoken feedback into actionable data. By utilizing advanced algorithms, organizations can sift through vast amounts of call data, identifying key trends and concerns that traditional methods may overlook.
With AI Feedback Analysis, businesses gain a competitive edge through real-time sentiment detection and deeper contextual understanding. The technology enables teams to analyze feedback quickly, addressing customer needs proactively. By integrating AI into feedback processes, organizations can elevate their customer experience, foster loyalty, and continuously enhance their products with relevant insights.
Analyze qualitative data. At Scale.
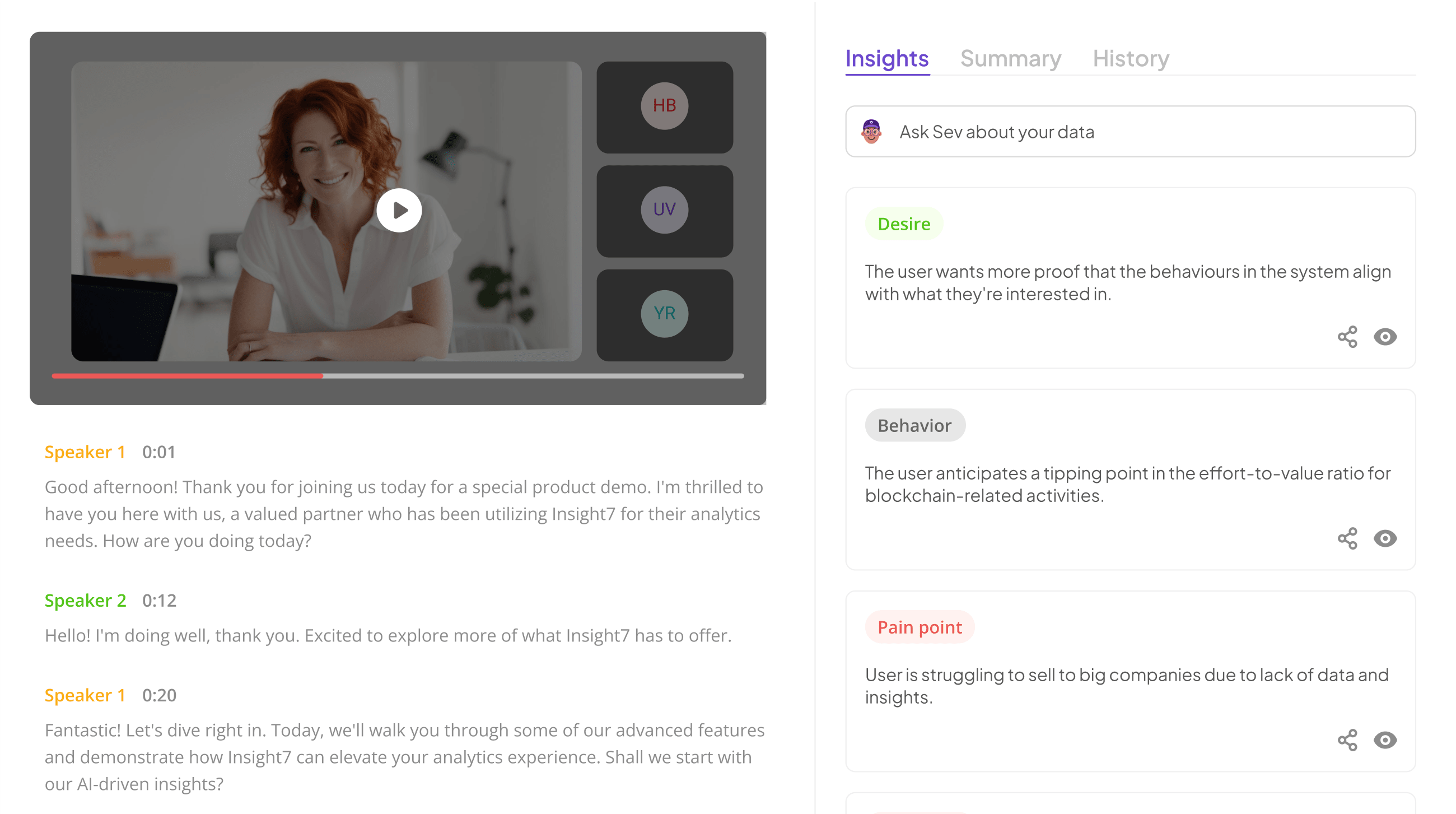
AI Feedback Analysis in Customer Calls represents a transformative approach to understanding customer experiences. Traditional feedback methods often struggle to capture the full scope of customer sentiment, leading to lost opportunities for growth. AI-powered techniques, however, excel in processing large volumes of data in real-time, allowing companies to gain immediate insights. This shift not only enhances efficiency but also delivers a more nuanced understanding of customer needs and expectations.
Implementing AI Feedback Analysis involves several key steps. Initially, companies must focus on effective data collection from customer calls through systematic techniques, ensuring that all relevant information is captured. Next, selecting the appropriate AI model is crucial, as various machine learning approaches can differently impact data interpretation. Finally, identifying patterns and trends in feedback can lead to actionable insights, paving the way for product improvements that resonate with consumers. Ultimately, embracing these advanced analysis methods equips businesses to stay competitive in a rapidly evolving market.
Understanding AI Feedback Analysis: The Basics
AI Feedback Analysis is a revolutionary approach that employs advanced technology to derive meaningful insights from customer feedback. Understanding the basics of this analysis reveals its significance in modern business practices. At its core, AI Feedback Analysis leverages algorithms to process and evaluate data collected from customer interactions, especially calls. This method stands out because it can handle large volumes of data, allowing for a comprehensive evaluation that traditional feedback methods often cannot match.
To grasp the fundamentals, consider two essential components: data processing and sentiment analysis. Data processing enables organizations to manage feedback in real-time, translating calls into actionable information. Meanwhile, sentiment analysis identifies customer emotions and attitudes, providing a deeper understanding of the feedback given. By embracing these innovations, companies can make informed adjustments that resonate with customer needs and enhance their offerings.
The Importance of AI in Customer Feedback
AI Feedback Analysis plays a pivotal role in understanding customer sentiments and improving products. Traditional methods of gathering feedback often fail to capture the depth and nuance of customer experiences. They are time-consuming and may miss critical insights hidden within the data. With the integration of AI, businesses can process large volumes of feedback quickly, making it both efficient and effective.
The key benefits of AI analysis are manifold. First, it offers real-time processing of feedback, allowing companies to respond promptly to customer needs. Second, sentiment analysis powered by AI can uncover underlying emotions in conversations, revealing areas for improvement. Lastly, AI can identify recurring patterns and trends, helping organizations make data-driven decisions that enhance customer satisfaction. By embracing AI-powered methods, companies can bridge the gap between customer expectations and product offerings, fostering stronger relationships with their audience.
- Why traditional methods fall short
Traditional methods of analyzing customer feedback from calls often rely on manual data processing, which can lead to inefficiencies and oversights. These outdated techniques typically involve human transcription, interpretation, and categorization, placing significant burdens on teams trying to derive actionable insights. Issues like bias in interpretation and difficulties in capturing emotions can further hinder accurate analysis, ultimately affecting decision-making.
Moreover, the sheer volume of data generated from customer interactions can overwhelm conventional approaches. As organizations strive to enhance customer satisfaction, their existing methods frequently fall short in processing feedback quickly and accurately. This is where AI feedback analysis offers a significant advantage, enabling real-time data processing and sophisticated sentiment analysis. By employing AI, businesses can not only scale their feedback analysis efforts but also unlock deeper insights that drive meaningful product improvements.
- Key benefits of AI analysis
AI Feedback Analysis offers transformative advantages for businesses seeking to refine their product offerings based on customer insights. One significant benefit is the ability to process vast amounts of data quickly and accurately. Traditional methods struggle with volume and speed, while AI tools can analyze large datasets from customer calls in real-time, ensuring timely insights.
Another key advantage is the nuanced sentiment analysis capabilities of AI. Unlike standard feedback mechanisms, AI can discern emotions and underlying sentiments, providing a deeper understanding of customer perceptions. This contextual analysis helps companies address pain points more effectively, leading to targeted improvements in products and services. Overall, AI Feedback Analysis not only enhances efficiency in processing customer data but also enables a richer understanding of customer needs, fostering innovation and growth.
How AI Transforms Feedback into Insights
AI transforms feedback into insights by utilizing advanced technologies that process customer interactions rapidly and accurately. Traditional feedback methods often struggle with scalability and the ability to extract meaningful information from large volumes of data. In contrast, AI feedback analysis employs real-time data processing, enabling businesses to respond to customer needs promptly and effectively.
Moreover, through sentiment analysis, AI interprets emotional tone and intent behind customer comments. This goes beyond simple keyword recognition, allowing for a nuanced understanding of customer perceptions and experiences. By identifying patterns and trends in feedback, AI systems help organizations discern actionable insights that can drive product improvement and enhance customer satisfaction. Ultimately, the integration of AI in feedback analysis represents a significant leap forward, turning passive data into active strategies for success.
- Real-time data processing
Real-time data processing is crucial in AI Feedback Analysis, enabling organizations to react swiftly to customer insights derived from calls. This capability allows businesses to capture data as it emerges, fostering a responsive approach to customer feedback. By analyzing comments immediately, companies can identify patterns and trends that reflect customer sentiments about products and services.
Moreover, the need for speed in data processing complements the rapidly changing expectations of consumers. Traditional feedback methods often lag behind, leaving gaps in understanding customer needs. Real-time processing not only enhances accuracy but also empowers teams to make timely decisions based on reliable insights. Implementing effective AI tools like Insight7 can facilitate this process, automating the transcription and evaluation of calls to deliver valuable feedback, leading to enhanced customer satisfaction and product development.
- Sentiment analysis and beyond
Sentiment analysis serves as a foundation for understanding customer feelings derived from interactions during calls. It provides businesses with pivotal insights, enabling them to gauge consumer satisfaction and identify areas needing improvement. By utilizing AI feedback analysis, companies can process large volumes of data quickly and accurately, transforming raw feedback into actionable insights.
However, the journey of feedback analysis extends beyond sentiment. Advanced tools can uncover deeper insights by recognizing patterns, detecting trends, and assessing contextual nuances in conversations. This holistic approach allows organizations to not only respond to customer concerns but also anticipate their future needs. Embracing AI feedback analysis opens avenues for data-driven decision-making and fosters enhanced customer relationships, positioning businesses for long-term success. By understanding sentiment and the broader context, companies can develop strategies that resonate with consumer desires, ultimately leading to improved product offerings and higher customer loyalty.
AI Feedback Analysis Tools: Leading the Change
AI Feedback Analysis Tools are fundamentally reshaping how organizations interpret customer calls. These advanced tools harness artificial intelligence to sift through vast amounts of feedback, extracting vital insights that traditional methods often miss. The efficacy of AI in feedback analysis lies in its ability to process data in real time and discern patterns, ultimately enabling businesses to respond meaningfully to customer needs.
Key tools leading this change include Insight7, known for its quantitative analysis capabilities, and others like MonkeyLearn and Qualtrics, which streamline data interpretation and sentiment analysis. When selecting an AI tool, factors such as integration capacity, pricing, and scalability are crucial. By adopting these advanced AI Feedback Analysis tools, businesses can unlock the potential for deeper customer understanding, leading to innovations that are truly aligned with market demands. Ultimately, the shift towards AI-driven feedback analysis is not just a trend; it's a necessary evolution for companies aiming to stay competitive.
Extract insights from interviews, calls, surveys and reviews for insights in minutes
Top Tools for AI Feedback Analysis
In the realm of AI feedback analysis, selecting the right tools is crucial for extracting valuable insights from customer calls. Several standout options can streamline this process. Firstly, Insight7 offers advanced capabilities for quantitative data analysis, making it easier to interpret large volumes of feedback. Additionally, MonkeyLearn is known for its intuitive interface that supports a variety of machine learning applications, enabling businesses to perform sentiment analysis efficiently.
Qualtrics stands out by providing comprehensive survey tools alongside robust feedback analysis features. Its ability to integrate various data sources ensures a holistic view of customer sentiments. Finally, IBM Watson distinguishes itself with powerful natural language processing, helping businesses understand complex feedback nuances. Choosing the best tools involves considering factors such as integration capabilities and pricing, aligning them with your specific needs for optimal results. Embracing these AI-driven tools unlocks a new level of understanding, ensuring your products evolve according to customer expectations.
Insight7
AI Feedback Analysis is revolutionizing how businesses interpret insights from customer calls. The paramount objective of understanding this process lies in transforming raw feedback into strategic advantages. By harnessing advanced algorithms and machine learning techniques, organizations can swiftly process extensive amounts of data, giving them a clearer picture of customer sentiments and experiences.
In practical terms, the process begins with data collection, where calls are intelligently transcribed and analyzed for key themes. Next, organizations leverage AI to conduct sentiment analysis, which uncovers valuable insights about customer satisfaction and areas for improvement. Armed with these findings, businesses can adapt their strategies, enhancing product offerings and tailoring customer service to meet evolving needs. This comprehensive approach is not just about gathering data; itโs about using insights efficiently to foster meaningful improvements in customer engagement. Embracing AI Feedback Analysis is thus crucial for thriving in a competitive landscape.
- Overview and key features
AI Feedback Analysis harnesses advanced algorithms to transform customer call data into actionable insights. This approach enables businesses to gain a deeper understanding of customer sentiment and feedback, which is crucial in todayโs competitive marketplace. By employing natural language processing and machine learning techniques, organizations can quickly analyze large volumes of customer interactions. This not only improves response times but also enhances the overall quality of customer service.
Key features of AI Feedback Analysis include real-time data processing, comprehensive sentiment evaluation, and the identification of recurring themes within feedback. For instance, AI can categorize customer emotions, helping businesses address issues proactively. Additionally, these tools often integrate seamlessly with existing customer relationship management systems, ensuring smooth information flow. The combination of these features provides organizations with the capability to respond swiftly to customer needs and continuously improve products based on direct feedback. Embracing this technology ultimately drives customer satisfaction and loyalty.
MonkeyLearn
MonkeyLearn is a powerful tool designed to assist in the analysis of customer feedback, especially through product conversations. With its capabilities, it empowers businesses to extract meaningful insights from customer calls, combining natural language processing with machine learning techniques. This approach enables organizations to decode complex sentiments and opinions expressed by their customers, which traditional methods often miss.
One of the standout features is its ability to automate sentiment analysis, providing immediate feedback on customer emotions. As a result, businesses can quickly grasp how their products are viewed, allowing for agile responses to feedback. Additionally, the tool excels in optimizing data categorization and text extraction, which is crucial in organizing feedback for actionable insights. By harnessing AI feedback analysis, companies can foster a deeper connection with customers, ensuring products align closely with their needs and expectations.
- Features and capabilities
AI Feedback Analysis provides a range of features and capabilities that are crucial for effectively interpreting customer calls. First, it enables real-time data processing, which significantly accelerates the feedback review process. This allows businesses to respond promptly to customer concerns and adapt their strategies accordingly. Furthermore, sentiment analysis within AI systems identifies not just what customers are saying, but also how they feel, allowing companies to understand their audience more deeply.
Another important feature is the ability to categorize and analyze large volumes of unstructured data effortlessly. AI tools can pinpoint recurring themes and trends, converting raw feedback into actionable insights. Advanced integration capabilities with existing systems ensure a seamless transition to AI-powered methods. By embracing these capabilities, organizations can enhance their product offerings and foster stronger customer relationships, ultimately driving growth and success in a competitive market.
Qualtrics
Qualtrics plays a pivotal role in enabling businesses to conduct AI Feedback Analysis effectively. By utilizing its sophisticated features, organizations can gather, analyze, and interpret product feedback from customer calls more efficiently. This platform empowers companies to transition from traditional feedback collection methods to advanced AI-driven systems, ultimately enhancing customer experiences.
The tool offers unparalleled capabilities like real-time feedback analysis and sentiment assessment, which illuminate key customer insights. Businesses can identify trends and address concerns faster, allowing for timely adjustments to product offerings. Moreover, its user-friendly interface ensures that teams without extensive technical backgrounds can harness the power of AI for actionable insights. By integrating Qualtrics into their feedback analysis processes, organizations can not only improve their products but also foster deeper relationships with customers, paving the way for future success.
- How it assists in feedback analysis
AI Feedback Analysis plays a pivotal role in enhancing the understanding of customer sentiments and experiences through their calls. By using advanced algorithms, AI can sift through large volumes of conversations to identify key themes, emotions, and areas for improvement. This process allows organizations to capture nuanced feedback that might be overlooked by traditional methods.
Additionally, AI tools enable real-time analysis, providing immediate insights that can inform decision-making. For instance, identifying recurring issues about a product can lead to timely adjustments. By enabling thorough examination of feedback patterns, AI helps teams transform customer calls into actionable strategies. This not only streamlines product development but also enhances overall customer satisfaction. Ultimately, AI feedback analysis fosters a more nuanced understanding of customer needs, paving the way for continuous improvement and innovation.
IBM Watson
In the realm of AI feedback analysis, certain tools stand out due to their advanced capabilities and unique features. One such notable tool excels in transforming raw customer call data into actionable insights. Its strength lies in its ability to process large volumes of data efficiently while utilizing sophisticated algorithms for sentiment analysis. This enables businesses to gain a deep understanding of customer sentiments and preferences through feedback analysis.
Furthermore, the tool offers rich capabilities for natural language processing. This aspect is crucial as it allows organizations to analyze customer emotions comprehensively. By identifying trends and patterns within the feedback, businesses can make informed decisions to enhance product offerings. Ultimately, leveraging AI-powered methods for feedback analysis empowers organizations to respond proactively to customer needs, ensuring continuous improvement and satisfaction.
- Key differentiators in AI feedback
In the realm of AI feedback analysis, several key differentiators set apart effective tools from the competition. One crucial factor is the ability to process data in real-time. This instant processing capability enables companies to promptly respond to customer inputs, allowing for swift adjustments in products or services based on immediate feedback. Moreover, sophisticated sentiment analysis features enhance understanding of customer emotions, capturing nuances that traditional methods often overlook.
Another important differentiator is the adaptability of AI systems to diverse feedback sources. Whether it's structured feedback from surveys or unstructured data from customer calls, quality AI feedback analysis tools can assimilate and interpret this variety seamlessly. Additionally, machine learning algorithms continuously refine their analytical capabilities, increasing accuracy over time. Ultimately, these distinguishing features empower businesses to derive actionable insights that inform decision-making and drive customer satisfaction.
Factors to Consider When Choosing an AI Tool
When selecting an AI tool for effective feedback analysis, several factors come into play that can significantly impact the overall performance. Firstly, assess the integration capabilities. An effective AI tool should seamlessly connect with your existing systems, ensuring smooth data flow and accessibility. This integration facilitates real-time analysis, allowing you to gather insights from customer calls promptly.
Next, consider pricing and scalability. Different tools come with varying costs, and itโs essential to find one that aligns with your budget while providing the features you need. Additionally, the tool should be scalable, accommodating your organizationโs growth without necessitating frequent changes.
Finally, evaluate user-friendliness. A tool that is easy to navigate will encourage efficient utilization among your team, ultimately enhancing the effectiveness of your feedback analysis efforts. Balancing these considerations will lead to informed decisions and more valuable insights from your AI feedback analysis initiatives.
- Integration capabilities
Integration capabilities play a pivotal role in enhancing AI feedback analysis from customer calls. A seamless integration ensures that the AI tool can effortlessly connect with existing systems such as customer relationship management (CRM) platforms and data analytics tools. This connection allows companies to analyze feedback more effectively, utilizing data from various sources for a comprehensive view of customer sentiments.
Several key factors influence the integration experience. Firstly, compatibility with prevailing software is essential, guaranteeing that AI tools like Insight7 can smoothly share and process data. Secondly, scalability must be considered to accommodate future growth, ensuring that as business needs change, the integration can adapt. Lastly, user-friendliness is important; a straightforward integration process minimizes team training and accelerates implementation. In summary, robust integration capabilities are fundamental to maximizing the benefits of AI feedback analysis, ultimately leading to enhanced product development and customer satisfaction.
- Pricing and scalability
Pricing and scalability play a crucial role in the adoption of AI feedback analysis tools. Understanding the cost structure of these solutions is essential for businesses seeking to optimize their feedback processes. Different platforms will offer varied pricing models, from subscription-based fees to pay-per-use options. This variety allows organizations to select a plan that aligns with their budget and operational demands.
Scalability is equally important, as companies grow and their feedback requirements evolve. An ideal AI feedback analysis tool should seamlessly adapt to increased volumes of customer data without compromising performance. This adaptability ensures that businesses can continue to derive valuable insights from customer calls, regardless of their size or scope. By investing in a flexible AI feedback analysis solution, companies can future-proof their processes and maintain a competitive edge in the market.
Steps to Implement AI Feedback Analysis Effectively
To implement AI feedback analysis effectively, begin by laying a robust foundation for data collection from customer calls. This involves establishing a streamlined method to capture both recorded conversations and written transcripts. Employ tools like Insight7 to assist in accurate data transcription and management. Consistent and comprehensive data collection prepares your system for the next steps in analysis.
Next, choose the right AI model to suit your specific feedback analysis needs. Consider factors such as the complexity of the data and the insights you hope to gain. Once your AI model is in place, begin identifying patterns and trends through various analytical techniques. Focus on spotting recurring themes in customer sentiments that can impact product improvements. Finally, convert these insights into actionable strategies to enhance customer experience, ensuring your product continues to meet market demands effectively.
Setting Up an AI System for Feedback
To set up an AI system for feedback, begin by efficiently collecting data from customer calls. This can be accomplished through automated transcription tools that convert voice conversations into text, allowing deeper analysis of customer sentiments. Focus on gathering a broad range of calls to ensure the dataset is comprehensive and representative of your customer base.
Next, selecting the right AI model is crucial for effective AI Feedback Analysis. You'll want to consider options such as supervised or unsupervised learning models tailored to your specific feedback objectives. Each model has advantages depending on the nature of the feedback you're analyzing. Additionally, ensure the chosen system can integrate seamlessly with existing tools for a smoother operational workflow and scalable growth in insights generation. The successful setup of your AI system will lead to enhanced understanding and actionability of customer feedback, ultimately driving product improvements.
Step 1: Data Collection from Customer Calls
To effectively implement AI feedback analysis, the initial step is the systematic collection of data from customer calls. This is crucial, as the insights gathered directly influence the quality of the analysis. Various techniques can be employed for efficient data capture, including call recording, transcription services, and note-taking during calls. Each of these methods aims to ensure that all essential customer feedback is documented accurately for further analysis.
Once the data is captured, it can be processed to extract valuable insights. Transcription tools can play a significant role here, converting audio data into text format, making it easier for AI systems to analyze. The richness of the language used in customer calls can reveal important sentiments and themes, all contributing to a comprehensive understanding of customer feedback. Investing time and resources in this initial data collection phase sets a solid foundation for successful AI feedback analysis and ultimately enhances the customer experience.
- Techniques for efficient data capture
Efficient data capture is essential for the success of AI feedback analysis, especially when analyzing product feedback from customer calls. To begin with, organizations must develop a structured process for collecting data directly from calls. This involves using advanced transcription software to convert spoken feedback into text, making the information easier to analyze and categorize.
Next, incorporating real-time data collection mechanisms, such as automatic sentiment scoring, can significantly enhance the analysis process. By integrating tools like Insight7, organizations can streamline data capture and ensure that the key points from customer conversations are not overlooked. Additionally, employing standardized templates for feedback categories can facilitate an organized approach to capturing nuanced customer insights, ultimately leading to more effective AI-driven analysis and better decision-making. Focusing on these techniques ensures that valuable customer feedback is translated into actionable strategies, improving product offerings and enhancing customer satisfaction.
Step 2: Choosing the Right AI Model
Selecting the appropriate AI model is a critical step in the process of AI feedback analysis. The effectiveness of this analysis hinges on the right model, which will dictate the quality of insights extracted from customer calls. Different AI modelsโsuch as supervised learning, unsupervised learning, and natural language processingโoffer various strengths and are suitable for different types of feedback data.
When determining the right model, several factors should be evaluated. Consider the nature of the feedback: Is it structured or unstructured? The model choice can depend on whether you're dealing with sentiment analysis or classifying feedback themes. Additionally, assess the modelโs ability to integrate with existing systems, its adaptability to scalability and evolving data needs, and the specific business goals you aim to achieve through AI feedback analysis. By carefully weighing these criteria, you can ensure that the AI model selected will effectively meet your organization's feedback analysis needs.
- Overview of machine learning models
Machine learning models play a critical role in AI feedback analysis, transforming how businesses analyze and interpret customer feedback. Various models can be employed, each suiting different types of data and analytical requirements. For instance, supervised learning models are often preferred for structured data, as they learn from labeled datasets. Conversely, unsupervised learning models can identify hidden patterns in unstructured data, making them ideal for extracting sentiments from customer calls.
To effectively implement machine learning in AI feedback analysis, organizations should consider key factors. First, they must evaluate the volume and type of feedback data they receive. Next, understanding the business goals will help in selecting the most suitable model. Finally, the computational resources available can significantly influence the choice of the model, especially for complex algorithms. By strategically choosing the right model, businesses can harness customer insights to drive improvements and innovation.
- Factors influencing model choice
Choosing the right AI model for analyzing product feedback is a crucial step in leveraging AI Feedback Analysis effectively. Various factors influence this decision, including the nature of the data and the specific goals of the analysis. Models may vary significantly in their approach, making it essential to align the choice with the intended outcomes, such as sentiment detection or trend identification.
One vital consideration is the complexity of the data being analyzed. Simple feedback might be effectively addressed with basic models, while intricate data may necessitate more sophisticated techniques. Additionally, the scalability of the model is essential; it should be able to adapt to varying volumes of feedback as the business grows. Lastly, the model's integration capabilities with existing systems should not be overlooked, ensuring a cohesive workflow. Each of these factors plays a pivotal role in optimizing AI Feedback Analysis for actionable insights.
Analyzing Feedback for Actionable Insights
To transform customer feedback into actionable insights, it is essential to employ AI feedback analysis. The process begins with identifying patterns and trends within the data collected from customer calls. This involves examining recurring themes and sentiments that emerge, thereby revealing areas of concern or satisfaction for your product. Using advanced algorithms, AI systems can process large volumes of data quickly, making it possible to pinpoint critical feedback points.
Once these patterns are recognized, it's crucial to translate them into actionable insights. This step encourages stakeholders to prioritize updates or changes based on customer input, ultimately leading to enhanced product offerings. By integrating customer perspectives into development strategies, organizations can ensure they are meeting true market needs and expectations. The effective utilization of AI in this analysis streamlines feedback interpretation and fosters continuous product improvement, creating a more responsive and customer-centric business model.
Step 3: Identifying Patterns and Trends
In this pivotal step, the focus turns to identifying patterns and trends within customer feedback. Analyzing product feedback from customer calls becomes a structured process aimed at discovering recurring themes that can guide future actions. By utilizing AI feedback analysis, organizations can sift through vast amounts of data efficiently, extracting meaningful insights that may otherwise remain hidden.
Next, establish a systematic approach to recognize these trends. Start by categorizing feedback into common themes, such as product features, customer service experiences, or pain points. Analyze sentiment to determine customer perceptions toward specific aspects. Lastly, monitor changes over time to gauge shifts in customer sentiment. This method not only facilitates deeper understanding but also helps in aligning product development with customer needs. By recognizing these patterns, businesses can implement strategic improvements, ultimately enhancing customer satisfaction and loyalty.
- Methods for spotting recurring themes
Spotting recurring themes is a crucial step in the AI feedback analysis process. To effectively identify these themes within product feedback from customer calls, one can utilize various analytical techniques. Initially, text mining methods can dissect the raw feedback data, extracting significant phrases and sentiments that frequently occur. This systematic extraction allows businesses to unveil common concerns, desires, or suggestions shared by customers.
Another powerful approach involves employing natural language processing (NLP) algorithms, which enhance the ability to recognize patterns in unstructured data. By classifying comments based on sentiment polarity and subject matter, organizations can categorize feedback into manageable insights. Additionally, incorporating visual data representation tools can aid in recognizing these themes quickly. It helps stakeholders grasp the underlying trends and evolve their products accordingly, ensuring that customer perspectives drive innovations and enhancements.
Step 4: Actionable Insights and Their Impact
After analyzing customer feedback, the next critical phase involves translating insights into concrete actions. Actionable insights are findings derived from AI feedback analysis that directly inform product enhancements and service improvements. Identifying these insights can lead to strategic decisions that resonate with customer needs, ultimately fostering brand loyalty and increasing sales.
To maximize the impact of actionable insights, businesses should focus on three key areas. First, prioritize customer-centric changes that align with recurring themes found in the analyzed feedback. Second, implement a feedback loop, where customers see their input reflected in product updates, bridging the gap between them and the brand. Finally, monitor how these changes influence customer satisfaction and adjust strategies accordingly, ensuring continuous improvement. By fully embracing this approach, companies not only enhance their offerings but also strengthen their relationship with customers, creating a cycle of trust and engagement.
- Leveraging insights to improve products
Understanding customer feedback is crucial for any organization aiming to enhance its products. By utilizing AI Feedback Analysis, businesses can transform raw data from customer calls into actionable insights. This process involves identifying key patterns and trends that emerge from customer sentiments, enabling companies to tailor their offerings according to customer needs and preferences.
The next step is to interpret these insights effectively. For instance, by pinpointing recurring themes in feedback, companies can prioritize their development efforts. This may involve tweaking existing features, resolving pain points, or even introducing entirely new functionalities. Over time, employing this strategy not only improves product quality but fosters long-term customer loyalty and satisfaction. By embracing a systematic approach to analyzing feedback, organizations can stay ahead of market demands and ensure that their offerings consistently resonate with users.
Conclusion: Embracing AI Feedback Analysis for Future Success
As businesses navigate the complexities of customer feedback, embracing AI Feedback Analysis becomes essential for future success. By employing AI-powered methods, companies can efficiently process vast amounts of data collected from customer calls. This not only streamlines the feedback analysis but also uncovers actionable insights that can significantly enhance product development and customer satisfaction.
Adopting AI feedback tools paves the way for businesses to identify patterns and trends in customer sentiments. By integrating these insights into their strategies, organizations can better align their offerings with customer expectations. As technology continues to evolve, leveraging AI will be crucial in staying ahead of the competition and ensuring long-term growth and adaptability.