AI Pattern Analysis is revolutionizing the way we understand transcribed interviews. Imagine pouring over hours of interview transcripts, searching for common threads and insights while feeling overwhelmed by the sheer volume of data. Now, with the power of AI, this exhaustive task becomes more manageable, allowing researchers to focus on interpretation rather than manual data processing.
This section will explore how AI Pattern Analysis can streamline the analysis of transcribed interviews, providing tools to uncover hidden themes and significant observations. By automating parts of the analysis, this approach not only saves time but also ensures a more objective examination of patterns and sentiments expressed during interviews. As we delve deeper, you'll discover strategies to implement AI effectively in your research processes.
Analyze qualitative data. At Scale.
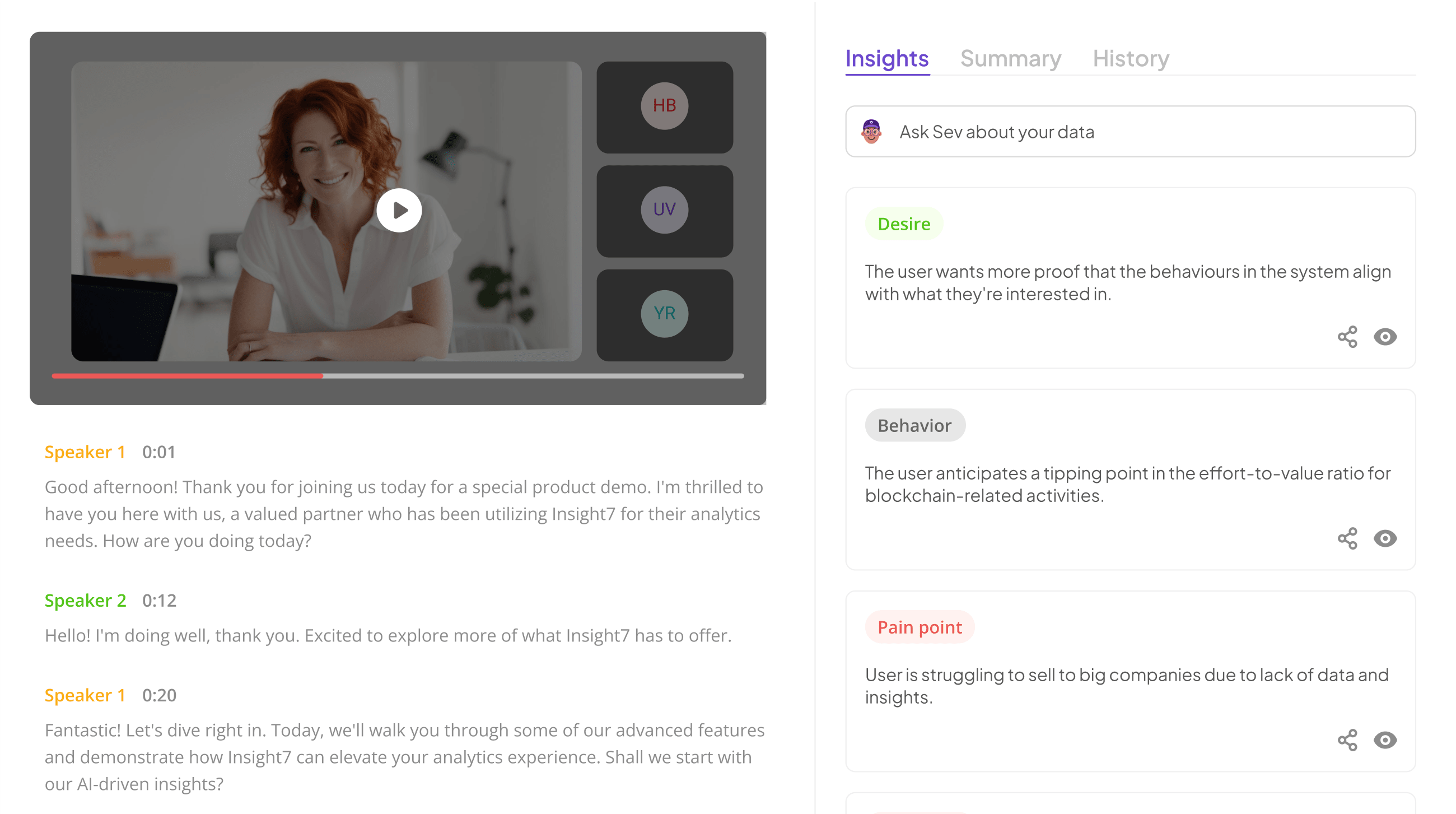
Understanding AI Pattern Analysis
AI Pattern Analysis is a transformative approach to dissecting and understanding qualitative data, particularly from transcribed interviews. This method harnesses machine learning algorithms to identify trends, themes, and insights that might be overlooked through manual analysis. By utilizing AI, researchers can efficiently process vast amounts of interview data, drastically reducing the time and effort required for traditional qualitative analysis.
The benefits of AI Pattern Analysis extend beyond speed; it enhances consistency and accuracy. Human analysts may introduce bias or miss critical patterns due to subjective interpretations. AI, on the other hand, provides a more objective analysis framework, enabling researchers to uncover hidden insights in the data. Overall, embracing AI Pattern Analysis can lead to richer insights and more informed decision-making in research practices. With this understanding, researchers can tap into AI's potential to streamline their workflows and enhance the quality of their findings.
What is AI Pattern Analysis?
AI Pattern Analysis refers to the process of identifying trends, themes, and patterns within data through artificial intelligence techniques. Most significantly, this approach focuses on analyzing qualitative data from sources like transcribed interviews, allowing researchers to extract meaningful insights efficiently. By harnessing AI algorithms, professionals can quickly examine large volumes of interview transcripts, highlighting recurring topics and sentiments.
The advantages of AI Pattern Analysis extend beyond speed and efficiency. It significantly reduces human bias, promoting consistency in how data is interpreted. This capability allows teams to streamline their workflows, transforming time-consuming analysis into straightforward, actionable insights. Overall, understanding AI Pattern Analysis empowers researchers to leverage technology effectively, enhancing their ability to derive value from qualitative data and making informed decisions based on robust findings.
The Importance of Pattern Analysis in Interviews
Pattern analysis plays a pivotal role in maximizing the value derived from transcribed interviews. By identifying recurring themes, phrases, or sentiments, its significance becomes evident in drawing actionable insights from qualitative data. These patterns can unveil underlying preferences, concerns, and motivations, enabling researchers and businesses to tailor their strategies for greater effectiveness.
AI pattern analysis enhances this process by automating the identification of these trends, which can often be overwhelming when conducted manually. As researchers sift through interviews, AI tools can rapidly highlight significant patterns, diminishing the risk of overlooking crucial insights. This not only bolsters efficiency but also fosters collaboration across teams, as findings are neatly organized and easily accessible. Thus, leveraging AI pattern analysis transforms interviews from mere data points into a rich narrative that guides decision-making and strategic planning.
Extract insights from interviews, calls, surveys and reviews for insights in minutes
Steps to Implement AI Pattern Analysis in Transcribed Interviews
To implement AI Pattern Analysis in transcribed interviews, start with preparing and preprocessing your data. Ensure all transcriptions are clear and organized, as the quality of input directly impacts analysis accuracy. Clean your transcripts by removing irrelevant information, such as filler words and non-verbal cues. This step creates a solid foundation for subsequent analysis.
Next, choosing the right AI tools is crucial. Research various options based on your requirements, such as ease of use, features, and integration capabilities. Tools should enable seamless data upload and support robust analytical functions like theme extraction and sentiment analysis. Finally, analyze and interpret the data by applying AI techniques to identify patterns and generate insights. Visualizations and reports can help distill these patterns into actionable findings, allowing for informed decision-making based on interview insights.
Step 1: Preparing and Preprocessing Data
To successfully implement AI pattern analysis, the first step is preparing and preprocessing the data collected from transcribed interviews. Start by organizing your audio or video recordings into manageable clips, focusing on significant ideas and key data points. Once you have your material segmented, transcribe audio files directly into your project environment. This will streamline the input process, allowing for easy access to your data.
Next, ensure your transcriptions are clean and structured. Remove any irrelevant content and standardize the format for consistency. You may want to identify themes relevant to your objectives, like employee engagement or performance management. This is crucial, as it lays the groundwork for the AI tools to analyze and extract valuable insights efficiently. With neatly organized and properly preprocessed data, the subsequent steps in AI pattern analysis will yield more precise and actionable findings.
Step 2: Choosing the Right AI Tools for Pattern Analysis
Selecting the right AI tools for pattern analysis is crucial in extracting meaningful insights from transcribed interviews. The effectiveness of AI pattern analysis largely depends on the tools you choose, as they can significantly enhance the efficiency and accuracy of data interpretation. The right tools should offer features like advanced natural language processing, sentiment analysis, and theme detection, which are vital in identifying patterns across large datasets.
To find the most suitable AI tools, consider factors such as ease of use, integration capabilities, and the specific needs of your analysis. For instance, tools with robust customization options can help adapt to the unique requirements of your interview data. Furthermore, ensure the tools provide actionable insights rather than just data points, enabling your team to make informed decisions quickly. By carefully evaluating these aspects, you can identify the right technology to streamline your qualitative analysis and deliver faster, more consistent results.
Step 3: Analyzing and Interpreting the Data
Once the data from transcribed interviews has been gathered, it is time for Step 3: Analyzing and Interpreting the Data. This crucial stage focuses on identifying patterns and extracting meaningful insights through AI Pattern Analysis. Begin by categorizing the data into themes or codes that resonate with your research goals. This helps in organizing disparate pieces of information into coherent groups for easier analysis.
Next, utilize AI tools to perform sentiment analysis on these identified themes. This process reveals the emotional undertones in responses, assisting in understanding participant attitudes and perceptions. Important insights can be derived from examining how often certain themes appear and what sentiments accompany them. By critically analyzing this data, you will establish a clearer picture of the overarching trends and patterns that emerge from participant interviews. Ultimately, this analysis serves as the foundation for actionable recommendations and strategic decision-making.
Top AI Tools for Pattern Analysis
When engaging in AI Pattern Analysis, selecting the right tools is essential for extracting meaningful insights from transcribed interviews. Among the top AI tools available today, several stand out for their unique features and capabilities.
First, at the forefront is insight7, offering a comprehensive solution tailored for qualitative analysis, including interviews. It streamlines data processing and delivers insights quickly, addressing the urgency in research reporting. Next, IBM Watson provides advanced text analytics, capable of understanding context and sentiment, which can enrich qualitative data interpretation.
Google Cloud Natural Language is another robust choice, recommended for its powerful AI capabilities that help analyze content effectively. Additionally, MonkeyLearn offers user-friendly text analysis, making it accessible for teams with varying levels of technical expertise. Lastly, Lexalytics allows easy integration into existing workflows, enhancing the efficiency of analysis. By utilizing these tools, researchers can transform their approach to data, achieving faster and more accurate results in understanding patterns from interviews.
insight7: A Comprehensive Solution
A comprehensive solution for AI Pattern Analysis can transform how transcribed interviews are utilized. By integrating advanced algorithms with your data, you unlock unprecedented insights that were previously difficult to obtain. This approach not only enhances the accuracy of pattern detection but also saves time, allowing analysts to focus on deeper interpretations and strategic decisions.
Utilizing a robust AI framework, this solution automatically processes large volumes of transcripts, detecting key themes, sentiments, and correlations. It provides actionable insights by grouping related concepts, thus facilitating better understanding and informed decision-making. By streamlining the analysis phase, users can dedicate more time to refining their strategies and deriving substantial value from qualitative data. Ultimately, embracing AI Pattern Analysis leads to a more efficient and effective approach to understanding the nuances in transcribed interviews.
IBM Watson: Advanced Text Analytics
Advanced text analytics plays a pivotal role in uncovering insights from transcribed interviews. By utilizing AI pattern analysis, organizations can sift through vast amounts of conversational data, revealing key themes and sentiments that may have gone unnoticed. This technology harnesses natural language processing to transcribe and analyze interviews at an unprecedented scale, transforming raw data into structured insights.
One significant advantage of advanced text analytics is its ability to perform sentiment analysis, identifying positive, negative, and neutral sentiments within the text. This helps in understanding not only what was said but also how it was perceived. Moreover, the technology can automatically generate reports and presentations from the analyzed data, facilitating easier decision-making. Harnessing these advanced capabilities allows businesses to derive meaningful patterns and insights that drive strategic initiatives, bridging the gap between data and actionable business strategies.
Google Cloud Natural Language: Robust AI Capabilities
Google Cloud Natural Language offers powerful AI capabilities that transform how we analyze transcribed interviews. By processing textual data, this tool helps identify patterns that may not be immediately apparent. Its ability to parse complex language allows for nuanced insights into customer sentiments and themes within conversations, which is crucial for organizations seeking to understand their audiences better.
One of the key features of this tool includes entity recognition, which identifies relevant keywords and phrases. Additionally, sentiment analysis provides valuable context regarding emotions expressed during interviews. These capabilities equip analysts with a robust framework for AI Pattern Analysis, enabling them to extract meaningful data efficiently. By utilizing these features, you can enhance your understanding of customer needs and adapt your strategies accordingly. Ultimately, integrating AI capabilities into your analysis process allows for more effective data-driven decision-making in any business context.
MonkeyLearn: Simple Text Analysis
The process of simple text analysis offers valuable insights by transforming raw data into understandable formats. For those engaged in AI pattern analysis of transcribed interviews, the accessibility of tools plays a critical role. Users can effortlessly upload transcriptions, which are then analyzed to extract key themes, quotes, and notable sentiments.
First, the transcriptions can be processed in bulk, making it easy to handle multiple files at once. Once uploaded, the platform takes care of the analysis, enabling users to visualize patterns and trends in the responses. This streamlined process empowers researchers to ask relevant questions based on the gathered insights, leading to informed decision-making.
Additionally, strong data privacy measures ensure that all information remains secure throughout this analysis journey. By simplifying the text analysis process, researchers can focus more on deriving actionable insights from interviews, which is the core goal of utilizing AI for pattern analysis.
Lexalytics: Easy Integration
Integrating advanced tools for AI pattern analysis can significantly streamline your process in deriving insights from transcribed interviews. First, effortless integration allows you to easily upload media and leverage built-in transcription capabilities. By eliminating the need for third-party tools, you can focus on analysis rather than managing multiple platforms. This simplicity fosters efficiency, enabling users to quickly generate and analyze transcripts in bulk or individually.
Next, user-friendly interfaces and organized project structures enhance the experience. Once your data is transcribed, it is stored in a library for easy access, where you can extract themes, quotes, and insights with just a few clicks. This accessibility simplifies the process of identifying patterns within narratives, allowing you to focus on questions and projects that matter most. With easy integration, you'll find that creating detailed reports from these interviews becomes a straightforward task, setting the stage for informed decision-making.
Conclusion: Embracing AI Pattern Analysis for Enhanced Insights
In conclusion, embracing AI pattern analysis can significantly transform how we derive insights from transcribed interviews. By employing advanced tools to sift through vast amounts of qualitative data, researchers can uncover deeper meanings and trends that might otherwise remain hidden. This approach not only streamlines the analysis process but also enhances the accuracy of insights drawn from conversations.
AI pattern analysis empowers users to filter and organize data in meaningful ways, enabling targeted insights that align with specific research goals. As we fully integrate AI into our analytical workflows, we can expect our findings to be not only quicker but also richer in depth and quality, making us better equipped to inform decision-making in various fields.