Bias Detection AI is transforming qualitative research coding by addressing the critical issue of bias in data analysis. In qualitative research, the risk of bias can obscure insights and mislead conclusions. This challenge becomes particularly pronounced when multiple researchers interpret data differently, leading to inconsistencies in findings. Enter Bias Detection AI, which offers a solution to enhance the objectivity and reliability of research outcomes.
Implementing AI in qualitative coding not only streamlines workflows but also identifies hidden biases that may not be evident through traditional methods. By analyzing patterns in language and sentiment, Bias Detection AI provides insights into potential biases and enhances consistency across coding processes. As researchers increasingly seek to deliver timely and accurate reports, AI presents an invaluable tool for elevating the quality and efficiency of qualitative research.
Analyze qualitative data. At Scale.
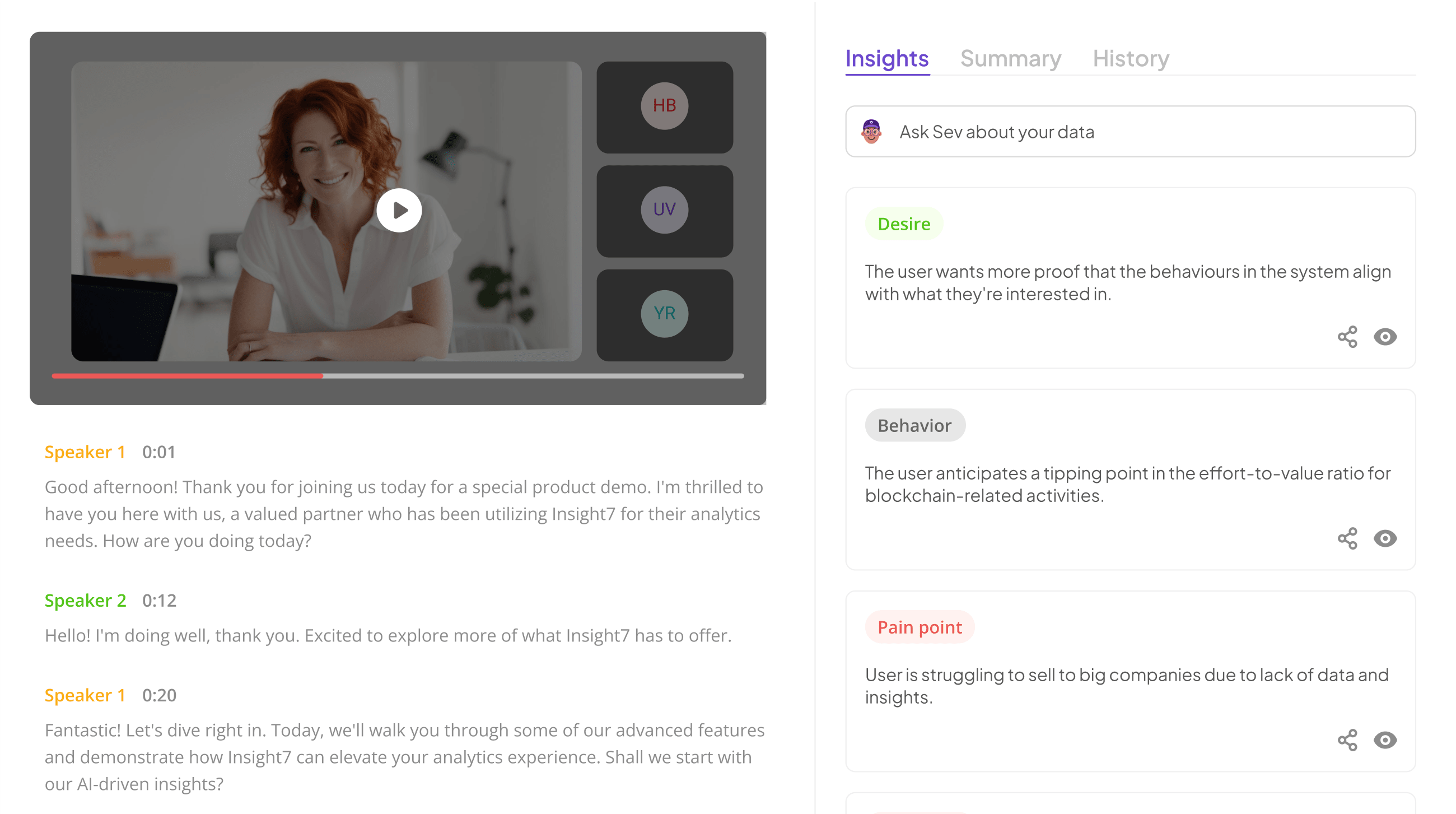
Understanding the Role of AI in Bias Detection
Artificial Intelligence plays a crucial role in bias detection, particularly in qualitative research. Bias Detection AI systems are designed to analyze qualitative data with a level of precision that human coders may struggle to achieve. By employing advanced algorithms, these systems can identify patterns or inconsistencies that may indicate bias in data, allowing researchers to address these issues early in the research process.
Understanding how Bias Detection AI operates revolves around two main functions: increasing consistency and enhancing objectivity. The ability to maintain consistent coding across different datasets minimizes the potential for human error. Moreover, AI systems can offer a more objective analysis, free from personal prejudices or emotions that might influence human coders. In this way, leveraging AI technology significantly contributes to producing reliable results in qualitative research, ultimately improving data integrity and paving the way for more trustworthy insights.
Benefits of Using AI for Bias Detection in Qualitative Data
Incorporating Bias Detection AI into qualitative research offers significant advantages that can transform how data is interpreted and reported. One key benefit is increased consistency in coding. Traditional manual coding often results in varied interpretations among researchers, leading to discrepancies in results. However, Bias Detection AI standardizes this process, ensuring that insights are consistently derived across different projects or teams.
Another benefit is enhanced objectivity. Human biases can unintentionally influence data analysis, potentially skewing findings. By utilizing AI, researchers can mitigate these biases, relying on algorithms that interpret data without emotional or cognitive biases. This not only leads to more reliable insights but also fosters greater confidence in the reported outcomes. Implementing Bias Detection AI ultimately streamlines qualitative research, reduces analysis time, and enhances the quality of insights derived from data.
Overall, these benefits underline the critical role of AI in providing unbiased, clear analysis within qualitative research.
- Increased Consistency
Increased Consistency in qualitative research is essential to achieving reliable and valid results. Bias Detection AI plays a crucial role in maintaining this consistency by automating coding processes that can often be influenced by human subjectivity. By applying advanced algorithms, AI can ensure that the same coding criteria apply uniformly across different data samples. This consistency minimizes discrepancies, leading to more robust analysis and interpretation of qualitative data.
Moreover, the use of Bias Detection AI enables researchers to identify and address any biases embedded within the data coding processes. For instance, when analyzing responses, the AI can highlight patterns that may reflect underlying biases, allowing researchers to adjust their approaches accordingly. This iterative feedback loop not only strengthens the research outcomes but also fosters a more transparent environment, where findings are trusted and reproducible. By integrating Bias Detection AI into qualitative research, researchers take a significant step toward greater objectivity and integrity in their work.
- Enhanced Objectivity
Enhanced objectivity is a crucial aspect of utilizing Bias Detection AI in qualitative research coding. By minimizing personal interpretations and judgments, we can promote more reliable and consistent findings. AI enhances objectivity by analyzing large datasets without the influence of human emotions or preconceived notions. This capability allows researchers to uncover patterns and insights that may otherwise go unnoticed, thereby improving the overall quality of analysis.
AI-driven tools can systematically evaluate data to identify biases inherent in coding processes. This leads to more data-driven conclusions, ensuring that the results reflect genuine patterns rather than subjective preferences. As researchers increasingly incorporate AI into their methodologies, enhanced objectivity becomes a transformative factor, allowing for a more robust understanding of qualitative data. Ultimately, embracing AI's strengths not only clarifies findings but also democratizes insights, making research more accessible and credible.
Techniques for Bias Detection AI in Qualitative Research
Bias Detection AI plays a crucial role in enhancing the quality and reliability of qualitative research. By utilizing advanced machine learning techniques, researchers can effectively identify patterns and potential biases in coding. One of the primary methods involves training AI models on diverse datasets, which helps the algorithms recognize various nuances in data. This process not only ensures a broader understanding of different perspectives but also minimizes the chance of overlooking biased data interpretations.
Another vital technique includes establishing robust validation processes. Regular updates and calibrations of AI systems maintain their reliability over time. Identifying bias indicators through systematic analysis empowers researchers to automate the coding process. This automation not only speeds up data analysis but also creates a more consistent framework for comparing results across different studies. Employing Bias Detection AI ultimately leads to more accurate insights, essential for informed decision-making in qualitative research.
Leveraging Machine Learning Models
Machine learning models present powerful tools for enhancing the accuracy of bias detection in qualitative research. By training these models on diverse datasets, researchers can significantly improve the detection of nuanced biases that may not be readily apparent through manual coding. Such training helps the machine learn to recognize patterns in the data, allowing it to flag inconsistencies or biases effectively.
Once trained, validating AI models for reliability is crucial. This involves assessing their performance against known benchmarks to ensure they maintain accuracy over time. By continuously refining these models and incorporating new data, researchers can enhance their ability to detect bias throughout their coding process. Implementing these machine learning models can lead to more objective and consistent research outcomes, ultimately fostering better decision-making based on reliable data insights.
- Training AI on Diverse Datasets
Training AI on diverse datasets is essential for creating effective Bias Detection AI. Diverse datasets ensure that the AI system encounters a wide array of perspectives, experiences, and contexts. This exposure helps the AI learn to recognize patterns and identify bias more accurately across different qualitative research settings. The more varied the training data, the better equipped the AI becomes to understand the nuances that come with subjective interpretation.
To ensure that Bias Detection AI performs reliably, it's crucial to include data from different demographic, cultural, and socio-economic backgrounds. This inclusion minimizes the risk of echo chambers, where the AI only reflects a narrow viewpoint. When training AI on diverse datasets, it also allows for the detection of subtle biases that may emerge in qualitative research. By actively integrating multiple viewpoints, researchers can create a more robust AI toolkit that enhances the reliability of qualitative coding and ultimately leads to fairer insights.
- Validating AI Models for Reliability
Validating AI models for reliability is crucial in ensuring accurate bias detection in qualitative research. The process involves several essential steps to guarantee that the AI system consistently interprets data without imparting bias. First, it's necessary to assess the training datasets for diversity. A diverse dataset helps the model learn from varied perspectives, reducing the risk of skewed results. Regular testing against known benchmarks also plays a vital role in this validation process.
Second, calibration of the AI model is imperative. This might involve ongoing adjustments based on feedback and results, ensuring the model evolves and improves over time. Lastly, employing cross-validation techniques can enhance reliability, as they help identify potential blind spots in the model’s capabilities. Overall, a structured approach to testing and validating bias detection AI will lead to more reliable and trustworthy outcomes in qualitative research coding, ultimately paving the way for innovations in data analysis.
Step-by-Step Guide to Integrating AI
Integrating AI into qualitative research coding entails a systematic approach that enhances bias detection capabilities. The first step is identifying bias indicators, such as patterns of language that may differ significantly across demographic groups. Understanding these indicators is essential for effectively employing Bias Detection AI in your research. Observing language nuances can pave the way for designing more equitable research methodologies.
Next, automating coding with AI can streamline the analysis process, reducing the time researchers spend on manual coding while increasing accuracy. This integration allows for the identification of bias patterns that might otherwise go unnoticed. Regular updates and calibration of the AI systems are crucial to maintain their reliability and responsiveness to new data. By committing to these steps, researchers can significantly improve the quality of their analyses, ultimately leading to more trustworthy and comprehensive findings.
- Identifying Bias Indicators
Identifying bias indicators is crucial in ensuring that qualitative research coding remains reliable and accurate. First, one must recognize potential sources of bias, including researcher perspective, question framing, and participant selection. These factors can skew results, leading to conclusions that may not represent the broader population. By systematically analyzing these aspects, researchers can better pinpoint where bias may infiltrate their findings.
Another important step involves leveraging Bias Detection AI to analyze coded data for inconsistencies and patterns that hint at bias. This technology can enhance the detection of human error and subjectivity, enabling a more objective assessment. For instance, discrepancies in coding across different team members often signal underlying bias or misinterpretation. Employing AI can also streamline the process, ensuring that researchers focus on interpretation and insight rather than manual coding labor. Ultimately, identifying and addressing bias indicators not only refines the research process but also enhances the validity of the insights gained.
- Automating Coding with AI
Automating coding with AI can significantly enhance the efficiency and accuracy of qualitative research. By utilizing Bias Detection AI, researchers can ensure that their coding processes remain consistent and free from human biases. This automation allows for a more objective analysis of qualitative data, enabling researchers to focus on interpreting findings rather than getting bogged down in manual coding tasks.
Incorporating AI into the coding process involves several essential steps. Initially, researchers must identify the specific indicators of bias that they seek to examine. Once these indicators are determined, researchers can automate the coding process to efficiently categorize qualitative data. Regular updates and calibration of the Bias Detection AI tools will further improve their performance, allowing for continuous refinement and adjustment as new data emerges. These strategies not only streamline the research process but also foster more reliable insights in qualitative studies.
- Regular Updates and Calibration
To maintain the effectiveness of Bias Detection AI, regular updates and calibration are vital components of the process. Continuous refinement ensures that the AI system adapts to new information and evolving contexts, thereby enhancing its bias detection capabilities. By routinely updating the AI with fresh training data and feedback from qualitative research, researchers can improve the model's accuracy and relevance. This is critical, as biases may shift over time and require adjustments in how the AI interprets and codes qualitative data.
Calibration involves verifying that the AI's assessments align with human experts' evaluations. Frequent calibration sessions not only confirm the consistency of the AI's performance but also allow teams to identify areas for improvement. This proactive approach fosters trust in the AI system, helping mitigate concerns about potential biases that may emerge in qualitative coding. Implementing these practices ensures that Bias Detection AI remains a reliable tool in the quest for unbiased insights in research.
Extract insights from interviews, calls, surveys and reviews for insights in minutes
Top Tools for AI-Driven Bias Detection
In today's research environment, selecting the right tools for AI-driven bias detection is paramount. Advanced software options streamline qualitative data analysis, enabling researchers to uncover biases hidden within their data. These tools utilize machine learning algorithms to enhance reliability and provide insights faster than traditional manual coding methods. By automating the coding process, researchers can focus on interpreting nuanced findings rather than getting lost in data management.
Several notable tools are essential for bias detection AI. Popular options include NVivo, which excels in organizing unstructured data and identifying biases effectively. Atlas.ti offers powerful qualitative analysis features that help researchers reveal patterns in their data. Meanwhile, Dedoose is known for its user-friendly interface, allowing easy integration of various data types while ensuring robust bias detection capabilities. Overall, embracing these tools enhances the research process, ensuring more reliable and objective outcomes.
insight7
Detecting bias in qualitative research coding is vital for maintaining the integrity of insights derived from data. Insight7 highlights the necessity of implementing Bias Detection AI to ensure objective analysis. This AI-driven approach enhances the consistency of qualitative coding by minimizing human error and preconceived notions, which are often sources of bias. By employing advanced algorithms, researchers can scrutinize data for inherent biases that may skew findings, ultimately leading to questionable conclusions.
Moreover, Bias Detection AI offers a structured pathway for identifying recurring patterns and themes within qualitative data. As organizations strive for more accurate insights, integrating AI helps automate and refine the coding process. Regular calibration and updates of AI systems are crucial in maintaining their accuracy over time. This ensures that coding reflects the most current understanding of the data, thus providing a more reliable foundation for decision-making. Embracing Bias Detection AI is not just beneficial; it's essential for producing trustworthy qualitative research outcomes.
- Overview and Features
In the realm of qualitative research, recognizing and addressing bias is essential for accurate data interpretation. The "Overview and Features" section delves into how Bias Detection AI transforms traditional coding methods. This advanced technology offers researchers the tools to systematically identify biases, ensuring their analyses are grounded in objective insights rather than subjective interpretations. Through automated processes, Bias Detection AI enhances both the reliability and validity of the research findings.
Key features of Bias Detection AI include customizable themes, robust reporting capabilities, and user-friendly interfaces. Researchers can effortlessly navigate through their data, extracting insights that directly inform decision-making. Furthermore, the ability to edit and refine themes means that the results are not just accurate; they are also meaningful. This technological integration ultimately empowers researchers to deliver richer, more trustworthy insights that drive impactful conclusions and recommendations in their work.
NVivo
NVivo stands out as a robust tool for qualitative researchers seeking to enhance their coding processes through advanced capabilities. With its focus on integrating human insight and AI, NVivo helps users efficiently navigate through complex datasets while detecting potential biases. This platform enables researchers to systematically analyze text, audio, and video content, allowing them to uncover patterns that may reflect underlying biases.
The strength of NVivo lies in its comprehensive coding framework. Researchers can easily tag and organize data segments, which facilitates the identification of bias indicators within qualitative research. By applying Bias Detection AI, users gain enhanced objectivity and consistency in their analyses, paving the way for more reliable findings. Continuous updates and training ensure that NVivo evolves alongside best practices in qualitative research, making it a vital resource for anyone focused on accurate and honest data interpretation.
- Capabilities in Bias Detection
Bias Detection AI plays a critical role in enhancing the integrity of qualitative research coding. Its capabilities allow researchers to identify and mitigate biases in data interpretation, ensuring that insights drawn from the research are fair and accurate. One of its primary strengths lies in the ability to analyze large volumes of qualitative data quickly, which can often overwhelm human coders. By employing advanced algorithms, Bias Detection AI can flag inconsistencies and patterns that may indicate bias, enabling researchers to focus on more nuanced analysis.
Furthermore, the capability of Bias Detection AI extends to the continuous improvement of coding processes. This tool learns from diverse datasets, which helps in recognizing various bias indicators specific to different contexts. Regular updates and calibrations ensure that the AI remains effective as new biases may emerge with changing societal standards. Ultimately, the integration of Bias Detection AI not only strengthens the validity of research findings but also fosters a more objective understanding of qualitative information.
Atlas.ti
When considering tools for qualitative research coding, understanding how various applications perform can significantly aid in bias detectionAI efforts. One such tool stands out for its comprehensive capabilities in this domain. With robust features that allow users to organize and analyze qualitative data efficiently, the tool facilitates an in-depth exploration of themes, codes, and categories.
Key strengths include its intuitive interface, which streamlines the coding process. By allowing researchers to easily tag and categorize data, it ensures better tracking of potential biases. Additionally, this tool supports collaborative work environments where multiple users can contribute to coding efforts. Ultimately, when integrated with AI capabilities, it enhances the overall quality and objectivity of qualitative research, contributing to more reliable insights and findings.
- Strengths in Qualitative Research Coding
Qualitative research coding possesses distinct strengths that enhance its effectiveness in uncovering nuanced insights. The use of advanced techniques, such as coding frameworks and thematic analysis, allows researchers to structure qualitative data meaningfully. This structured approach enables analysts to identify patterns, relationships, and trends that might otherwise remain hidden. Additionally, employing Bias Detection AI can further streamline this process, as AI tools assist in efficiently categorizing large datasets and revealing nuanced findings.
Another key strength of qualitative research coding lies in its flexibility. Researchers can adapt coding schemes as new themes emerge from the data, allowing for a responsive analysis process. By harnessing Bias Detection AI, researchers can rapidly assess the objectivity of their coding, reducing the risk of human bias. This fosters a more accurate interpretation of qualitative findings and enhances the overall reliability of the research outcomes, ensuring that valuable insights are not overshadowed by subjective perspectives.
Dedoose
Dedoose offers a streamlined platform tailored for qualitative research, making the coding process more efficient and manageable. Its user-friendly interface allows researchers to easily sort and analyze vast amounts of data, pinpointing potential biases throughout the coding journey. By integrating visualizations and collaboration features, it fosters a more comprehensive understanding of the research findings, allowing diverse team members to contribute insights. This tool also supports compliance with data privacy regulations, ensuring that sensitive information remains protected during the research process.
The usability of Dedoose is significant when addressing bias detection AI. Researchers can upload various data formats, including interviews, while leveraging built-in transcription services to improve accuracy. This ensures a more thorough analysis as AI identifies patterns and potential biases in qualitative research. With its capabilities, Dedoose not only enhances the research process but also contributes to generating reliable insights by continuously adapting to the project's needs.
- Usability and Integration Features
To ensure a user-friendly experience in bias detection, tools must prioritize simplicity without sacrificing capability. Usability and integration features are essential for seamless interaction with Bias Detection AI. An intuitive interface allows researchers to navigate complex datasets effortlessly. When tools focus on user-centric design, they empower researchers to identify biases more effectively. Moreover, integrating with existing qualitative research software enhances the overall experience, streamlining workflows.
Integration features play a vital role as well. These allow Bias Detection AI to communicate smoothly with various data sources and analysis platforms. By supporting multiple formats and fostering compatibility, users can harness the full potential of their data. This synergy not only saves time but also enhances the accuracy of bias detection, resulting in more reliable insights for qualitative research. Ultimately, the combination of usability and integration establishes a foundation for effective bias analysis in research practices.
Conclusion: The Future of Bias Detection AI in Qualitative Research
The future of bias detection AI in qualitative research promises to reshape how researchers approach data interpretation. As the demand for rapid and trustworthy insights grows, integrating advanced AI capabilities will enhance both the speed and accuracy of coding processes. Researchers can expect AI tools to provide more consistent analysis, minimizing human error, and addressing varying interpretations among team members.
Looking ahead, the evolution of bias detection AI will likely focus on sophisticated algorithms that learn from diverse data sets. This ongoing learning will empower researchers to identify emerging biases more effectively, fostering a culture of objectivity in qualitative analysis. By embracing these innovations, qualitative researchers can ensure that their findings are not only timely but also reliable, paving the way for informed decision-making across industries.