Automated Insight Tagging is revolutionizing qualitative research by addressing the inefficiencies associated with manual analysis. Traditionally, researchers would devote significant time to sifting through interviews and open-ended survey responses, often leading to inconsistencies and biases in the insights gathered. With the rising demand for quicker and more accurate reporting, researchers are now turning to automation solutions that enhance their workflow and allow them to focus on deeper analysis.
This introduction highlights the transformative potential of Automated Insight Tagging in qualitative research archives. By streamlining the tagging process, researchers can achieve a more cohesive understanding of data, ensuring that insights align with client expectations and deadlines. Embracing these advanced tools not only improves accuracy but also enhances collaboration across research teams, ultimately leading to richer findings and actionable recommendations.
Analyze qualitative data. At Scale.
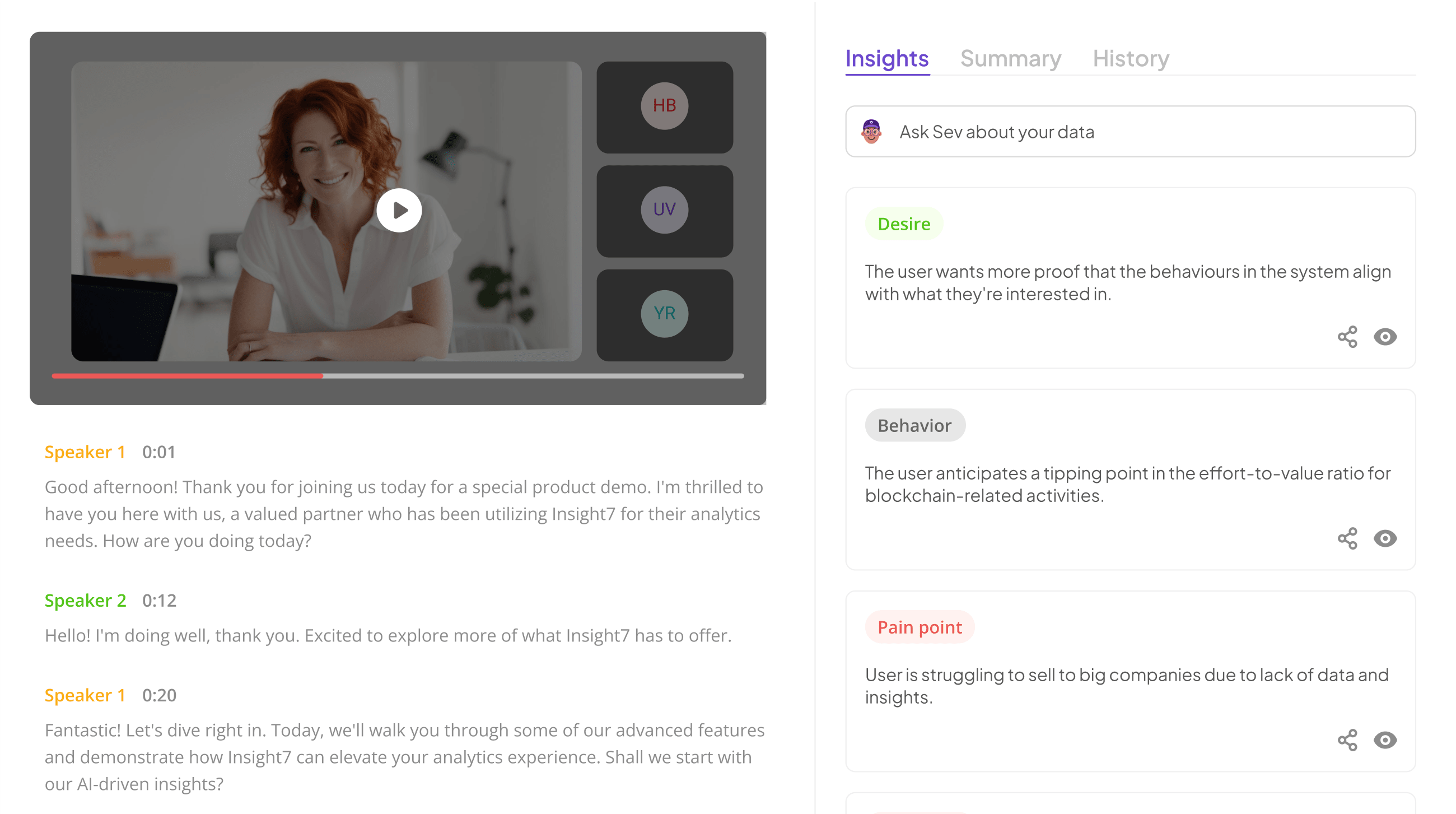
The Role of Automated Insight Tagging in Streamlining Research Processes
Automated Insight Tagging significantly enhances the efficiency of research processes by transforming qualitative data into structured insights. This technology streamlines the analysis phase, allowing researchers to categorize and summarize information quickly. As qualitative data often contains vast amounts of nuanced information, automated insight tagging aids in filtering key sentiments and themes. Consequently, researchers can focus more on interpretation rather than manual coding, saving time and minimizing human error.
Moreover, having visibility over insights allows researchers to easily reference the origin of data. They can filter insights by speaker or theme, which enhances the reliability of conclusions drawn. Additionally, the ability to export tagged insights directly into reports fosters seamless integration into existing methodologies. Overall, automated insight tagging empowers researchers to deliver high-quality analyses with speed and accuracy, revolutionizing how qualitative research archives are utilized.
Benefits of Using Automated Insight Tagging
Automated Insight Tagging offers numerous advantages that revolutionize how qualitative research is conducted. One significant benefit is the enhanced efficiency in data processing. By automating the tagging process, researchers can quickly categorize and analyze vast amounts of qualitative data, saving valuable time and resources. This speed not only facilitates faster reporting but also enables researchers to focus on interpretation and strategic decision-making.
Another key advantage is the consistency and accuracy of insights produced. Automated systems reduce human error and ensure that insights are tagged systematically, allowing for greater reliability in data interpretation. Furthermore, it enhances transparency, enabling researchers to track the sources of insights easily. This systematic approach not only reinforces trust in the findings but also allows for more refined data presentations tailored to specific themes or topics of interest, leading to deeper insights and actionable outcomes.
In combining these benefits, Automated Insight Tagging emerges as an essential tool for today's qualitative research, streamlining workflows while bolstering the quality and reliability of insights.
Challenges Addressed by Automating Tagging
Automating tagging addresses several critical challenges faced by qualitative research teams. One significant issue is the time-consuming nature of manual analysis. Researchers spend countless hours sifting through interviews and surveys, delaying the delivery of insights to clients. By automating this process, teams can significantly speed up their workflows, allowing them to respond more quickly to client needs.
Another challenge is the inconsistency that arises when multiple researchers analyze data. Each team member may interpret results differently, leading to potential biases and fragmented conclusions. Automated insight tagging provides a standardized framework, ensuring that insights are uniformly categorized and reducing variations in interpretation. This not only enhances the reliability of the insights produced but also fosters team collaboration and cohesiveness. By addressing these challenges, automated insight tagging ensures that qualitative research remains efficient, consistent, and impactful.
Extract insights from interviews, calls, surveys and reviews for insights in minutes
Implementing Automated Insight Tagging: A Step-by-Step Guide
Implementing Automated Insight Tagging is essential for enhancing the efficiency of qualitative research archives. The first step involves identifying suitable tools and technologies that align with your research goals. It’s important to evaluate platforms like insights7, which offers streamlined tagging processes and robust data integration capabilities. Exploring additional tools can also provide diverse options tailored to specific needs, enhancing the overall workflow.
Once the right tools are in place, begin the integration process into existing research workflows. This ensures that new tagging systems complement traditional methods without overwhelming researchers. The final step focuses on training and fine-tuning the tagging system. Continuous learning and adaptation will help in accurately tagging insights as new data comes in. By following these steps, teams can transition to an automated tagging system that significantly improves data organization and accessibility in qualitative research archives.
Step 1: Identifying Suitable Tools and Technologies
Finding the right tools and technologies is essential for effective automated insight tagging. This initial step sets the foundation for a smooth research process. Begin by assessing the specific needs of your project. Analyze the type of data you’ll be working with and the complexity of insights required. Ensuring compatibility with existing systems can greatly enhance productivity.
Next, explore various platforms tailored for qualitative research automation. Look for solutions that not only transcribe data accurately but also allow the extraction of themes and generate reports. Prioritize tools that offer robust data management and ensure compliance with privacy regulations. For instance, certain tools enable bulk uploads and feature built-in transcription, enhancing efficiency. Ultimately, the right selection of tools will streamline your tagging process, allowing for deeper insights and improved analysis in your qualitative research archives.
- 1.1 Using insight7 for Insight Tagging
Using insight7 for insight tagging simplifies the process of extracting valuable insights from qualitative research archives. Users can upload different types of data, including audio files, directly into the platform. There, audio is transcribed, and insights are automatically generated, allowing for the identification of themes and key concepts without extensive manual intervention.
The platform supports functionalities that allow researchers to cluster data, analyze interviews, and generate comprehensive reports. Users can easily ask questions of their data, enabling nuanced insights that inform decision-making. By utilizing automated insight tagging, researchers can expedite their workflows, reduce manual effort, and focus on more strategic tasks. This tool exemplifies how technology can enhance the research experience, making it accessible for individuals without technical backgrounds. Ultimately, the integration of automated insight tagging promotes efficiency and clarity in analyzing qualitative data.
- 1.2 Exploring Other Automation Tools
In this section, we will delve into various automation tools that complement automated insight tagging for qualitative research archives. Each tool presents unique functionalities that can enhance the tagging process and ultimately streamline your research efforts. By exploring these alternatives, researchers can make informed decisions to further optimize their workflows.
Consider the following tools that provide valuable features for automated insight tagging:
Tool A: This tool offers advanced machine learning algorithms to identify patterns in qualitative data, making tagging more accurate and efficient.
Tool B: Designed with user-friendliness in mind, Tool B allows researchers to customize tags based on specific themes relevant to their projects, enhancing relevance.
Tool C: Aimed at enhancing collaboration, this tool provides real-time updates on tagging, allowing teams to work seamlessly together.
Tool D: This automation tool integrates with existing software, enabling smoother processes and reduced manual input for researchers.
By understanding the strengths of each tool, researchers can significantly enhance their automated insight tagging capabilities, leading to more focused and actionable outcomes.
- Tool A
In the journey of qualitative research, Tool A emerges as a pivotal solution for implementing automated insight tagging. This tool is designed to streamline the initial analysis phase, allowing researchers to efficiently process and tag interview transcripts. By automatically scanning through extensive qualitative data, Tool A provides a solid foundation for identifying key themes and insights, significantly reducing the time traditionally spent on manual reviews.
Utilizing Tool A enables researchers to focus on deeper analysis and interpretation of the data, rather than getting bogged down by the initial tagging process. Its user-friendly interface and robust features facilitate seamless integration into existing workflows, ensuring a smoother transition to automated processes. With this tool, researchers can effectively manage multiple projects, enhancing overall productivity while maintaining the quality of their insights. In essence, Tool A is an essential asset in harnessing the full potential of automated insight tagging, elevating qualitative research efforts to new heights.
- Tool B
Tool B offers an innovative approach to Automated Insight Tagging, catering specifically to qualitative research archives. This tool seamlessly integrates with existing research workflows, allowing researchers to efficiently tag insights without the manual effort traditionally associated with this process. Its design prioritizes user experience, ensuring that qualitative data is analyzed quickly and accurately.
Using Tool B, researchers can set criteria that the system applies to identify themes and patterns from the vast amount of qualitative data. As a result, users can swiftly locate relevant insights, enhancing the analytical process. The tool learns from previous tagging, continuously improving its accuracy over time. Its user-friendly interface minimizes the learning curve, enabling researchers to focus on the insights rather than the tagging mechanics. Overall, Tool B significantly streamlines the research process, making it an indispensable resource for anyone looking to automate their insight tagging efficiently.
- Tool C
Tool C offers a unique perspective on automated insight tagging, specifically designed to enhance your qualitative research archives. It serves as a bridge, connecting raw data to actionable insights efficiently. By utilizing this tool, you can easily define specific tags and themes relevant to your research goals. This allows for a streamlined analysis of data, ensuring that important themes are not overlooked.
One essential feature of Tool C is its capacity for sentiment analysis alongside insight clustering. This functionality helps researchers gain a deeper understanding of participant sentiments and interactions within the data. For example, insights related to collaboration and knowledge sharing become more structured, allowing you to target areas like member experiences directly. This method not only saves time in data processing but also generates quality insights, making your research efforts more effective and impactful.
- Tool D
Tool D emerges as a vital component in the quest for efficiency in qualitative research archives. This tool focuses on enhanced automated insight tagging, which facilitates quicker analysis and organization of qualitative data. By utilizing advanced algorithms, Tool D streamlines the identification of key themes and insights from extensive datasets, thus saving valuable time for researchers.
One of the standout features of Tool D is the ability to customize tagging based on specific research goals. Users can initiate predefined themes, such as risks or challenges, or create their unique tags that align with various analysis focuses. Furthermore, Tool D provides sentiment analysis, offering a quantifiable measure of participant emotions linked to different themes. This analytical depth not only supports informed decision-making but also enhances the overall quality of insights derived from qualitative research archives, making Tool D indispensable in modern research practices.
Step 2: Integrating Tools Into Existing Research Workflows
To successfully integrate tools into existing research workflows, begin by assessing your current processes and identifying where automated insight tagging can bring the most value. Start by mapping out your qualitative research stages, noting any repetitive or time-consuming tasks. This insight will guide you in selecting tools that complement your workflow rather than disrupt it. Ensuring that automation seamlessly fits into how your team operates is essential for effective implementation.
Next, involve your team in the integration process. Gather feedback on chosen tools and provide training on their functionalities. Focus on demonstrating how automated insight tagging can enhance efficiency and data organization. Encourage an iterative approach where users can suggest modifications based on their experiences, aligning the tools with research objectives. As you refine your automated systems, take advantage of insights gained to foster collaboration and enhance the overall research output.
Step 3: Training and Fine-Tuning the Tagging System
Training and fine-tuning the tagging system is critical for effective automated insight tagging. This process involves adjusting the algorithms and rules that enable the tagging system to classify and label data accurately. Initially, it’s essential to train the system using a diverse set of qualitative research data to provide a solid foundation. This data should include various themes, terminologies, and context specific to your research focus to enhance the training experience.
Once the initial training is complete, continuous fine-tuning is necessary. This can involve monitoring the tagging accuracy and making adjustments to the algorithms based on user feedback or real-world application results. Regular updates to the system ensure it evolves alongside your research needs, improving its capability to capture nuanced insights accurately. Ultimately, a well-trained tagging system streamlines data management, enabling researchers to derive meaningful insights quickly and efficiently.
Conclusion on Automated Insight Tagging for Qualitative Research Archives
In conclusion, Automated Insight Tagging offers a transformative solution for managing qualitative research archives. By using advanced algorithms, researchers can significantly reduce the time needed for data analysis and eliminate inconsistencies often found in manual tagging. This not only streamlines the research process but also enhances the quality of insights derived from qualitative data.
Furthermore, the implementation of automated systems contributes to quicker reporting, a critical requirement in today's fast-paced research environment. As teams experience improved efficiency, they can focus more on interpretation and strategic decision-making, ultimately providing more value to their clients. Embracing this technology signifies a shift towards smarter qualitative research practices, fostering better understanding and insights.