How to Detect Voice of Customer Patterns Using Qualitative Data AI
-
Bella Williams
- 10 min read
Introduction to Qualitative Insights AI in Voice of Customer Analysis delves into the evolving role of technology in understanding consumer sentiments. Companies today gather immense amounts of feedback, yet deciphering valuable insights can be overwhelming. Qualitative Insights AI emerges as a transformative tool, allowing organizations to unveil underlying patterns in customer feedback efficiently.
This section will explore how qualitative data can provide deep understanding into customer experiences. By utilizing AI to analyze unstructured data, businesses can identify trends and sentiments that drive customer behavior. This process not only enhances decision-making but also opens avenues for more personalized customer engagement strategies. Understanding these insights is crucial for any organization striving to meet and exceed customer expectations.
Analyze & Evaluate Calls. At Scale.
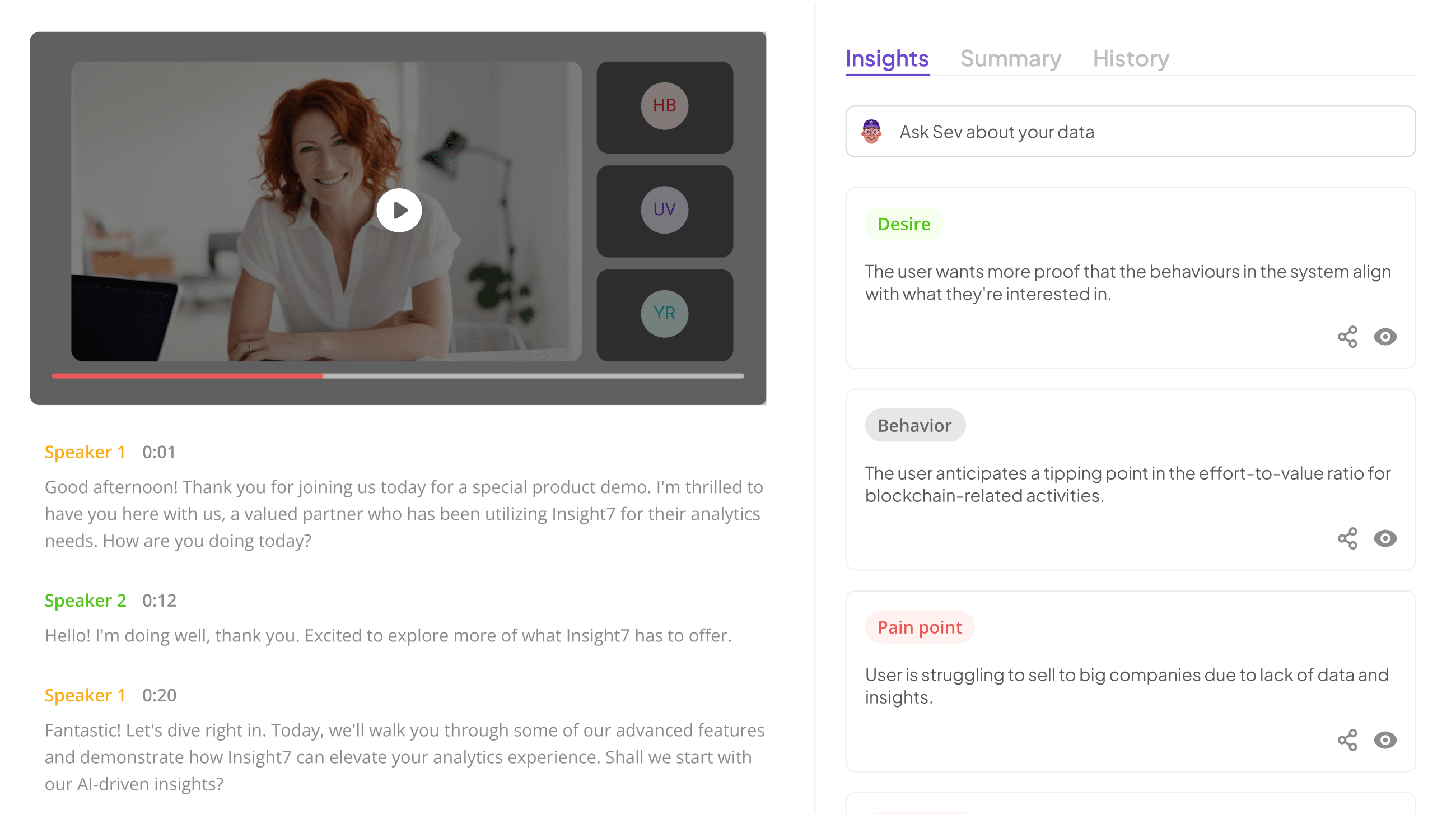
Understanding Qualitative Insights AI for Customer Feedback
Qualitative Insights AI plays a crucial role in understanding customer feedback, enabling businesses to grasp the nuances that quantitative data may overlook. By analyzing customer emotions and opinions expressed in interviews and open-ended survey responses, organizations can identify key sentiments and emerging trends. This AI-driven approach facilitates faster and more accurate insights, addressing common pain points like bias and inconsistencies in manual analysis.
To harness these insights effectively, businesses need to implement specific strategies. First, they must focus on collecting rich, unstructured data from various sources, as this forms the foundation of meaningful customer intelligence. Secondly, employing sophisticated AI algorithms allows organizations to detect patterns and sentiments efficiently, transforming raw data into actionable intelligence. Lastly, interpreting these AI-generated insights is vital for decision-making, enabling businesses to adapt their strategies based on genuine customer feedback. By embracing Qualitative Insights AI, organizations can enhance their understanding of customers and improve their offerings accordingly.
Extracting Qualitative Insights AI from Unstructured Data
Extracting qualitative insights from unstructured data is vital for understanding customer sentiments. Unstructured data, such as customer feedback from surveys, social media, and reviews, can hide valuable patterns. To effectively extract these insights, it is essential to utilize advanced AI methods that can sift through vast amounts of unorganized information, helping to highlight significant themes. This process transforms raw data into actionable insights that illuminate the voice of the customer.
Several techniques can aid in refining this process. First, natural language processing (NLP) algorithms analyze text to detect sentiments and trends. Next, machine learning models can categorize responses, ensuring that insights reflect customer experiences accurately. Finally, visual analytics tools interpret the data effectively, enabling stakeholders to spot significant patterns. By applying these methods cohesively, organizations can harness qualitative insights AI, revealing the motivations and preferences that drive customer behavior.
- Importance of Unstructured Data in Voice of Customer Analysis
Unstructured data plays a pivotal role in Voice of Customer analysis, as it embodies the rich and varied opinions, attitudes, and emotions expressed by customers. This type of data, often found in interviews and open-ended survey responses, offers invaluable qualitative insights that can reveal underlying patterns in customer sentiment. By analyzing this unstructured information, organizations can uncover trends that might not be visible through traditional quantitative data alone.
Moreover, utilizing Qualitative Insights AI enhances the processing of unstructured data, making it easier to identify customer sentiments quickly and accurately. This not only improves the speed of reporting but also mitigates bias and inconsistency, enabling teams to generate cohesive insights more efficiently. In today's fast-paced environment, harnessing the power of unstructured data is essential for any organization looking to thrive based on customer feedback and preferences.
- Methods to Identify Customer Sentiments and Patterns
To identify customer sentiments and patterns effectively, it's crucial to analyze qualitative data using various methods. First, categorization of feedback can illuminate prevailing trends. By grouping comments into themes, businesses can discern areas of satisfaction and concern among customers. This process often incorporates sentiment analysis, which utilizes AI to gauge emotional responses within customer remarks. Such insights can guide strategic decision-making and improve overall customer experience.
Another useful method involves triangulating data sources. Combining qualitative insights with quantitative metrics enables a more comprehensive understanding of customer behavior. For instance, cross-referencing survey data with customer interviews reveals a deeper narrative behind the numbers. This multi-faceted approach not only enhances the quality of insights but also strengthens the trustworthiness of the findings. Embracing these methods ensures that organizations remain responsive to customer needs, ultimately fostering loyalty and engagement.
Key Tools for Analyzing Voice of Customer Patterns
Analyzing Voice of Customer patterns requires effective tools that streamline the process and enhance interpretability. Several key tools serve this purpose by harnessing the power of Qualitative Insights AI. First, analysis kits enable users to ask targeted questions and extract themes efficiently from qualitative data. This can transform an overwhelming amount of unstructured feedback into manageable insights relevant to specific use cases. Themes categorize insights into groups, such as risks or customer desires, allowing a clearer understanding of customer sentiment and motivations.
Other significant tools include MonkeyLearn and Thematic, which utilize machine learning to recognize patterns and trends. Clarabridge specializes in text analytics, providing sentiment analysis markers that highlight customer emotions tied to feedback. Finally, Kapiche focuses on collating insights from various channels, ensuring a comprehensive analysis. Each tool brings unique strengths, providing varied avenues for interpreting qualitative data effectively and improving customer experiences.
- Insight7: Leading the Way in Qualitative Data Analysis
Qualitative data analysis plays a pivotal role in understanding customer feedback, especially as organizations strive to capture the voice of the customer. Insight7 stands at the forefront, integrating advanced AI technologies to streamline qualitative insights extraction. By processing unstructured data more efficiently, businesses can swiftly identify customer sentiments, preferences, and emerging trends.
Incorporating Qualitative Insights AI allows organizations to automate the initial stages of qualitative analysis, alleviating repetitive tasks. This technology empowers analysts to focus on deeper insights, facilitating informed decision-making. The power of automated analysis combined with human expertise creates a distinctive approach to understanding customer experiences. As companies harness these capabilities, they gain a competitive edge in their respective markets, making their strategies more responsive to customer needs. Overall, Insight7 exemplifies how AI can transform qualitative data analysis, illuminating patterns that would otherwise remain obscured.
- Additional Tools for Detecting Customer Voice Patterns
When delving into the realm of detecting customer voice patterns, several advanced tools complement the analytical capabilities of Qualitative Insights AI. These tools enhance understanding by allowing businesses to sift through vast amounts of unstructured data effectively. They help uncover crucial sentiments and themes that often elude manual analysis, providing an enriched perspective on customer feedback.
MonkeyLearn: This versatile tool focuses on text analysis and is adept at extracting insights from customer feedback, allowing companies to categorize responses effectively.
Thematic: By utilizing machine learning, Thematic identifies trends and sentiments in qualitative data, enabling organizations to understand customer perceptions and emotions more deeply.
Clarabridge: Known for its ability to analyze customer interactions across various channels, Clarabridge offers a comprehensive view of customer behavior and preferences.
Kapiche: This solution specializes in enabling companies to capture and analyze customer insights, transforming qualitative feedback into actionable recommendations.
Implementing these tools can empower businesses to capture the rich narrative woven through customer feedback, ultimately driving better decision-making.
- MonkeyLearn
The application of advanced tools enhances the analysis of voice of customer patterns through qualitative insights AI. These platforms facilitate the automation of data organization and sentiment analysis, significantly impacting decision-making processes. By transforming unstructured feedback into organized insights, they help researchers recognize underlying trends and sentiments that traditional methods may miss.
One such powerful tool offers a variety of features designed to streamline qualitative data analysis. Users can leverage its capabilities to segment data, identify topics, and visualize sentiment trends effectively. Additionally, these tools often provide excellent customer support to assist users through their analysis journey. This level of support fosters a more seamless experience, enabling teams to focus on what matters most: extracting actionable insights from valuable customer feedback. Employing such platforms can dramatically enhance understanding and responsiveness to customer needs, promoting a more customer-centric approach in business strategies.
- Thematic
Thematic analysis plays a crucial role in deciphering the Voice of Customer patterns using Qualitative Insights AI. By identifying themes within qualitative data, businesses can gain a sharper understanding of customer needs, preferences, and pain points. This process involves noticing repeated ideas, narratives, or sentiments that emerge from customer feedback, enabling organizations to tailor their strategies effectively.
To implement thematic analysis, one can follow several key steps. Firstly, collect qualitative data through interviews, surveys, or social media comments. Once gathered, utilize AI tools to analyze the data, enabling the identification of significant themes. Finally, interpret these themes to extract actionable insights. Understanding these patterns not only informs business decisions but also fosters customer-centric approaches, resulting in improved engagement and loyalty. By employing qualitative insights, organizations can translate customer feedback into impactful strategies, ultimately driving success in the market.
- Clarabridge
Qualitative Insights AI plays a significant role in analyzing customer feedback, particularly when it comes to unstructured data. One of the standout options available for this purpose is a tool known for streamlining data interpretation and uncovering customer sentiments. This platform allows organizations to efficiently collect insights from various sources, such as surveys and interviews, ensuring that voices of customers are accurately represented. The AI capabilities embedded within this tool enable businesses to recognize patterns, helping them understand customer needs more comprehensively.
When using this tool, companies can expect to see improvements in how they categorize and analyze qualitative data. By translating unstructured feedback into actionable insights, decision-makers can significantly enhance their strategies. The result is a clearer picture of customer satisfaction and preferences, ultimately leading to a more tailored approach in service delivery. Thus, incorporating Qualitative Insights AI through this tool not only strengthens data analysis processes but fosters a deeper connection with customers.
- Kapiche
To grasp how Kapiche operates, consider its ability to process qualitative data at scale. Businesses today often struggle with extracting actionable insights from extensive interview data. Kapiche simplifies this process through its innovative platform designed for voice of customer analysis. Users can seamlessly upload audio and text data, enabling the AI to identify themes, sentiments, and trends across the information gathered.
The platform's unique capabilities shine in its ability to support various file types and multiple languages, ensuring accessibility for global companies. Additionally, it facilitates quick data analysis by generating reports and visualizations from collected insights. Kapiche not only prioritizes data privacy but also significantly reduces the time spent on manual analysis. This efficiency allows organizations to focus on transforming qualitative insights AI into business strategies, ultimately leading to more informed decision-making processes.
Extract insights from interviews, calls, surveys and reviews for insights in minutes
Implementing Qualitative Insights AI to Understand Customer Feedback
Implementing Qualitative Insights AI to understand customer feedback involves a structured approach to data analysis. First, organizations need to gather and preprocess qualitative data from various sources like interviews and surveys. This foundational step ensures that the data is clean, relevant, and ready for analysis. Next, employing AI algorithms allows businesses to detect patterns in customer sentiment effectively. These algorithms can sift through vast amounts of unstructured data, highlighting key themes and insights that may not be apparent through manual analysis.
Once the insights are generated, the final step is interpretation. Understanding the AI-driven findings is crucial for making informed decisions. Team members should collaborate to assess the insights in the context of their organizational goals. By following this process, organizations can significantly enhance their understanding of customer voices and preferences, ultimately leading to improved products and services that align better with customer expectations.
Steps for Successful Qualitative Data Analysis
To achieve successful qualitative data analysis, begin with data collection and preprocessing. This involves gathering data from diverse sources, ensuring its accuracy and relevance. Clean and organize the data to eliminate inconsistencies, which sets a strong foundation for insightful analysis. Next, use AI algorithms to detect patterns within the data. These algorithms can highlight trends, sentiments, and prevailing themes, making it easier to understand the Voice of Customer patterns.
Interpreting these AI-driven insights is the final step. This involves careful examination of the identified themes and sentiments to draw meaningful conclusions. Consider the context of the data collected and relate it to your specific goals, such as improving customer experience or addressing pain points. By following these steps, companies can effectively harness Qualitative Insights AI to derive valuable actionable insights and enhance their understanding of customer needs and preferences.
- Step 1: Data Collection and Preprocessing
Data collection and preprocessing form the foundation of any successful analysis using Qualitative Insights AI. The first step is to gather diverse data from various customer interactions, including surveys, interviews, and social media feedback. This approach ensures that the dataset captures a wide range of customer sentiments and experiences.
Once the data is collected, preprocessing is essential. This includes cleaning the data by removing duplicates or irrelevant information, formatting it for analysis, and structuring it to highlight key insights. For effective analysis, consider categorizing feedback into themes, such as positive, negative, or neutral sentiments. By organizing the data, you facilitate quicker and more accurate identification of trends and patterns in customer feedback, leading to actionable insights. With thorough data collection and preprocessing, your qualitative insights will lay the groundwork for uncovering valuable customer behavior patterns.
- Step 2: Employing AI Algorithms for Pattern Detection
In the second step of detecting Voice of Customer patterns, employing AI algorithms becomes crucial. These algorithms analyze vast amounts of qualitative data to identify recurring themes and sentiments. By processing customer feedback effectively, AI allows organizations to uncover insights that may not be immediately apparent through manual analysis. As AI algorithms sift through qualitative data, they reveal underlying emotions and patterns, enabling companies to enhance their understanding of customer needs.
Various AI algorithms can be used for pattern detection, including Natural Language Processing (NLP) and machine learning techniques. NLP helps to analyze text data and extract relevant keywords, phrases, and sentiments. Machine learning models can classify and cluster customer feedback into actionable categories. These methods transform qualitative insights AI into a power tool for businesses, offering a clear perspective on customer experiences and preferences. As organizations embrace these technologies, they can foster improved customer relationships and drive better decision-making.
- Step 3: Interpreting AI-Driven Insights
Interpreting AI-driven insights is crucial in transforming the data analysis phase into valuable understanding. First, review the summarized findings generated by Qualitative Insights AI. These reports often highlight significant themes and underlying sentiments derived from customer feedback. By focusing on key insights, businesses can identify patterns in customer preferences that guide decision-making.
Next, contextualize these insights within your specific research focus. For instance, if the analysis reveals persistent concerns about process management, delve deeper into those comments to uncover actionable recommendations. This interpretative stage allows you to not only confirm patterns but also adjust strategies based on customer input. By continually refining your approach through AI-driven insights, you enhance your capability to respond effectively to customer needs and adapt to market changes.
Conclusion: Harnessing Qualitative Insights AI for Enhanced Customer Understanding
Harnessing Qualitative Insights AI paves the way for deeper understanding of customer behaviors and preferences. By integrating this technology, businesses can detect significant patterns within qualitative data that traditional methods often overlook. Gaining these insights empowers organizations to tailor their strategies, enhancing customer engagement and retention.
Moreover, the utilization of Qualitative Insights AI allows for dynamic responses to evolving customer needs. As organizations embrace these advanced analytical tools, they not only improve their decision-making processes but also foster a more personalized customer experience. In this rapidly changing market, understanding the voice of the customer becomes paramount for sustained success.