Automated Interview Analysis represents a significant shift in how qualitative research can be executed. Traditional methods often involve tedious manual coding that can consume valuable time and resources. However, this modern approach allows researchers to focus on extracting insights rather than getting bogged down in repetitive tasks. Through automation tools, you can efficiently convert interview transcripts into actionable insights without losing the nuances of the conversation.
The transformation begins with integrating advanced technologies such as Natural Language Processing (NLP) and Machine Learning. These technologies analyze text data to identify themes, sentiments, and critical points, providing deeper insights with enhanced accuracy. By employing automated interview analysis, researchers can streamline their workflow and focus on interpreting the data, ultimately improving the quality of their findings. Embracing these innovations not only saves time but also elevates the overall research experience.
Introduction to Automated Interview Analysis Without Manual Coding
Automated Interview Analysis revolutionizes how researchers handle qualitative data, particularly when analyzing interviews. Traditional manual coding can be slow and prone to biases, posing significant challenges for teams striving for consistent insights. As industries demand faster and more accurate results, the transition to automated methods becomes essential for enhancing research efficiency and reliability.
In this section, we will explore how Automated Interview Analysis streamlines the interview process, minimizing the time spent on data organization and improving the quality of insights generated. With the integration of Natural Language Processing and Machine Learning, researchers can access deeper analyses and achieve quicker turnaround times, ultimately enabling better decision-making and client satisfaction.
Analyze & Evaluate Calls. At Scale.
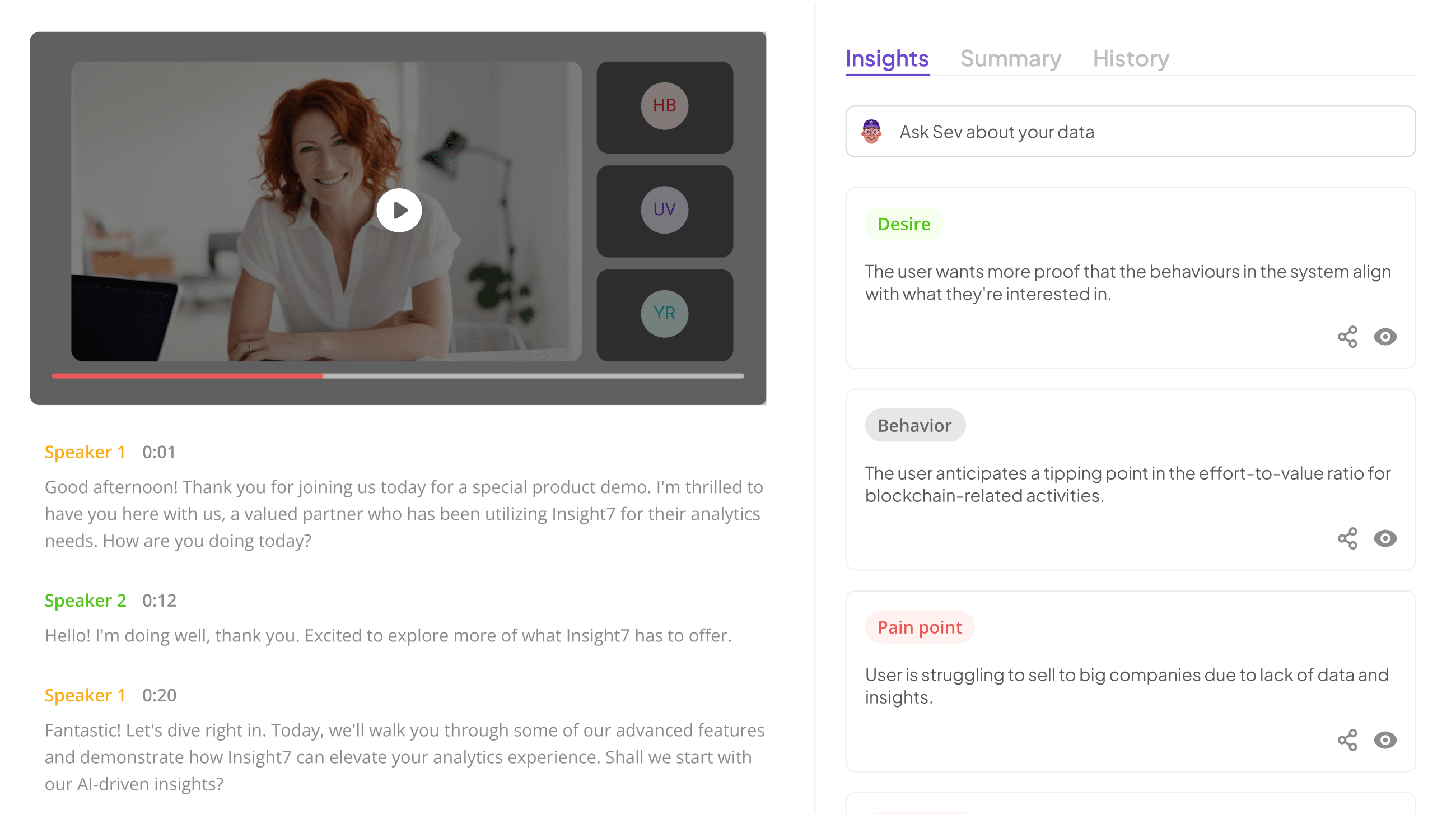
Leveraging Automated Interview Analysis Methods
Automated interview analysis plays a crucial role in enhancing the efficiency of qualitative research. Traditional methods often suffer from significant delays, as manual coding can be labor-intensive and prone to bias. By adopting automated approaches, researchers can rapidly process large volumes of interview data, ensuring timely insights for clients.
Two key components of automated interview analysis are Natural Language Processing (NLP) and Machine Learning. NLP allows software to understand and interpret human language, which significantly enhances the accuracy of data extraction. Meanwhile, machine learning algorithms can identify patterns and themes in responses, delivering deeper insights than manual analysis typically provides. These technologies not only reduce the potential for human error but also enable teams to focus on strategic decision-making rather than being bogged down by tedious coding tasks. Embracing these methods transforms the research landscape, fostering quicker and more accurate outcomes.
Understanding the Need for Automation
Conducting text-based interview analysis traditionally involves a considerable amount of manual effort, which presents various challenges. Manual coding is time-consuming and often leads to inconsistencies in how insights are generated. Different team members may interpret the same data in varied ways, creating bias and prolonging the delivery of results. Clients increasingly demand faster turnaround times for insights, prompting the need for a more efficient solution.
Automation, particularly through automated interview analysis, offers significant advantages in addressing these challenges. By streamlining the process, automation reduces the time needed to analyze text data and enhances accuracy. Automated tools can process large volumes of interview data quickly, providing insights that are consistent and reliable. As organizations pivot towards efficiency, embracing automation becomes essential to stay competitive and meet client expectations. Transitioning to automated methods not only saves time but also transforms the quality of insights delivered.
- The challenges of manual coding in interview analysis.
Manual coding in interview analysis presents several challenges that can significantly hinder the research process. One primary difficulty is the time-consuming nature of manual coding, which requires researchers to sift through vast amounts of qualitative data. This often leads to prolonged project timelines, making it hard to deliver insights to clients swiftly. Moreover, this labor-intensive approach introduces potential biases. Different researchers may interpret the same data differently, resulting in inconsistencies across insights generated by the team.
Additionally, manual coding does not scale well, especially for projects involving large data sets. As the volume of interviews grows, so does the complexity of accurately coding responses. This not only strains resources but can also degrade the quality of analysis. In contrast, automated interview analysis solutions can facilitate streamlined processing, enhance efficiency, and significantly reduce human error. By embracing automation, teams can focus on drawing deeper insights rather than getting bogged down in repetitive tasks.
- The benefits of automation in speeding up the process.
Automated Interview Analysis presents numerous benefits that significantly expedite the research process. By automating routine tasks, researchers can save valuable time that would otherwise be spent on manual coding and data entry. This increased efficiency allows for quicker turnarounds on insights, catering to the growing demand from clients for faster results in text-based analysis.
One critical advantage of automation is the ability to simultaneously analyze multiple interviews. It enables researchers to identify patterns and themes across large datasets, leading to a more comprehensive understanding of the data. Additionally, automated tools enhance the accuracy of results by minimizing human error, ensuring consistent coding and analysis. This combination of speed and precision not only aids in obtaining insights but also empowers researchers to focus on strategic decision-making, ultimately driving more effective outcomes for their projects. In this fast-paced environment, embracing automated approaches is essential for maintaining relevance and delivering quality analysis.
How Automated Tools Transform Interview Analysis
Automated Interview Analysis is reshaping the way researchers examine qualitative data. With the rise of Natural Language Processing (NLP) and Machine Learning, these tools facilitate the transformation of interviews into actionable insights rapidly. Traditional methods of manual coding often lead to biases and inconsistencies, slowing down the delivery of compelling insights. Automated tools eliminate these barriers by ensuring a consistent and objective analysis, enhancing overall accuracy.
Furthermore, such tools simplify the analysis process, making it more accessible even for small teams with limited resources. They can handle vast amounts of data, recognize patterns, and extract themes efficiently, greatly reducing the time spent on qualitative projects. As research demands grow, integrating automated solutions becomes crucial. The adoption of these technologies not only streamlines the workflow but also empowers researchers to focus on deriving meaningful conclusions rather than getting bogged down in tedious manual tasks.
- The role of Natural Language Processing (NLP) and Machine Learning.
Natural Language Processing (NLP) and Machine Learning (ML) are revolutionizing the field of Automated Interview Analysis. NLP enables machines to understand and interpret human language, transforming unstructured interview data into structured information. This technology can automatically categorize responses, identify recurring themes, and assess sentiment, allowing researchers to extract meaningful insights efficiently.
Machine Learning algorithms further enhance this process by learning from previous analyses, improving accuracy with every interaction. By employing these advanced techniques, organizations can rapidly process large volumes of text, minimizing the need for extensive manual coding. This change not only accelerates the analysis but also provides a more nuanced understanding of interview dynamics, resulting in data that is rich, comprehensive, and actionable. Overall, integrating NLP and ML streamlines the analysis process, paving the way for more informed decision-making based on direct feedback.
- Enhanced accuracy and deeper insights with automated approaches.
Automated Interview Analysis significantly elevates the accuracy of insights derived from interview data. Traditional manual coding processes often lead to inconsistencies and overlook subtle patterns embedded within the text. By employing automated approaches, organizations can achieve a higher level of precision, allowing for more reliable data interpretation. The integration of Natural Language Processing (NLP) enhances understanding by deciphering context and tone, delivering richer insights that manual methods may miss.
Moreover, automated tools can process large volumes of interviews swiftly, mitigating the time constraints often faced by analysts. With the ability to extract themes, generate visualizations, and answer specific inquiries quickly, these technologies streamline the entire analysis process. Consequently, teams can focus more on strategic interpretation rather than data encoding. Overall, leveraging automated approaches enables companies to unlock profound insights, making data-driven decisions both accurate and efficient.
Extract insights from interviews, calls, surveys and reviews for insights in minutes
Top Tools for Automated Interview Analysis
In the realm of automated interview analysis, selecting the right tools can profoundly impact the efficiency and accuracy of qualitative research. Various platforms excel in simplifying the text-based analysis process, ensuring researchers can extract meaningful insights without the burden of manual coding. One popular choice is MonkeyLearn, which harnesses the power of machine learning to categorize and analyze text data effortlessly. Its user-friendly interface allows for customizable workflows tailored to specific research needs.
Another influential tool is NVivo, renowned for its robust integration with qualitative analysis. It offers powerful features for organizing and querying interview data, making it easier for researchers to identify patterns and themes. ATLAS.ti further strengthens this landscape with its exceptional data management capabilities, supporting vast datasets and rich media formats. Finally, QDA Miner offers impressive functionalities for coding and analyzing interview data, streamlining the entire analytical process. By leveraging these tools, researchers can effectively transform their approach to automated interview analysis, yielding faster and more reliable insights.
Insight7
Automated Interview Analysis represents a remarkable shift in how we process qualitative data, particularly during text-based interviews. This technique harnesses advanced technologies to efficiently extract insights without the need for manual coding. By employing algorithms and Natural Language Processing, we can swiftly identify patterns and themes within large datasets. This leads to significant time savings and improved accuracy, essential for businesses striving to understand their market better.
Utilizing tools for Automated Interview Analysis not only enhances the analytical process but also equips organizations with actionable insights. Companies can uncover deeper meanings from customer feedback, informing strategic decisions with great precision. Furthermore, these automated methods reduce human bias and errors, ensuring a more reliable interpretation of interview data. Embracing this technology is crucial for any organization looking to advance its qualitative research efforts in today's fast-paced environment.
- Overview and key features.
Automated Interview Analysis fundamentally reshapes how researchers interpret text-based data derived from interviews. The primary strength of these tools lies in their ability to streamline the analysis process, freeing researchers from the tediousness of manual coding. By employing sophisticated algorithms, these tools not only enhance efficiency but also produce insights with impressive accuracy. This innovative approach allows for rapid identification of themes, enabling researchers to focus on deriving actionable strategies rather than getting bogged down in data processing.
Key features of an Automated Interview Analysis system include user-friendly interfaces that facilitate easy setup and customizable project goals. With just a few clicks, users can generate comprehensive reports that highlight core findings correlated with the initial objectives. Moreover, advanced analytics capabilities empower users to explore context around quotes, ensuring a reliable understanding of the gathered data. These intuitive features make it easier to derive meaningful insights without extensive coding knowledge, making interview analysis both accessible and effective.
- How Insight7 simplifies text-based interview analysis.
Text-based interview analysis can often feel overwhelming, particularly when done manually. Insight7 simplifies this process through advanced automated interview analysis methods. By employing sophisticated algorithms, it streamlines the task, allowing researchers to focus on interpreting insights rather than getting bogged down by coding efforts.
One of the standout features is its use of Natural Language Processing (NLP), which allows the system to accurately identify themes and patterns within the responses. This not only speeds up the analysis but also enhances the reliability of insights generated. Additionally, the consistency in analysis is improved, as automated processes reduce human bias and eliminate discrepancies that can arise from different team members interpreting data in varying ways. By leveraging these automation capabilities, teams can deliver faster reports and actionable insights to clients, addressing the time-sensitive needs of todayโs research environment.
Other Leading Tools
When considering other leading tools in the realm of automated interview analysis, several options stand out for their unique strengths and capabilities. MonkeyLearn is an intuitive platform that specializes in text analysis and classification, making it highly beneficial for those looking to derive insights from interview data swiftly. Its machine learning capabilities allow users to customize models according to their specific needs, simplifying the process considerably.
Nvivo, another robust tool, excels in qualitative data analysis. It accommodates a variety of data types, integrating seamlessly with other software for comprehensive research. This tool provides visual mapping features that are particularly useful for identifying themes and patterns in interview transcripts. Similarly, ATLAS.ti focuses on rich data management, offering tools for organizing and analyzing complex information effectively. Lastly, QDA Miner stands out for its capacity to process multiple interview transcripts simultaneously, making it efficient for larger datasets. By leveraging these tools, researchers can enhance their automated interview analysis, facilitating deeper insights without getting bogged down in labor-intensive coding.
- MonkeyLearn: Features and use cases.
The use of advanced tools can greatly enhance the efficiency of automated interview analysis. MonkeyLearn stands out due to its user-friendly interface, which allows users to seamlessly import and process text data. Through its intuitive design, teams can easily analyze interviews by organizing transcripts and extracting vital insights without succumbing to the complexities of manual coding.
Key features include the ability to cluster related data, enabling in-depth thematic analysis across multiple interviews. Additionally, predefined "kits" help guide users through specific analytical tasks, making it easier to capture insights relevant to marketing or product research. Such functionalities not only streamline the data analysis process but also provide a collaborative platform for teams to work together efficiently. By eliminating manual coding, users can focus on interpreting insights to make informed decisions.
- Nvivo: How it integrates with qualitative analysis.
Nvivo plays a vital role in transforming qualitative analysis by offering comprehensive features for automated interview analysis. This tool allows researchers to seamlessly organize and analyze text-based interviews, reducing the burden of manual coding and enhancing productivity. By utilizing advanced algorithms, it can rapidly categorize and synthesize large volumes of qualitative data, allowing researchers to focus on deriving insightful conclusions rather than getting bogged down in tedious tasks.
Integration with Natural Language Processing (NLP) further enhances its capabilities, enabling the identification of patterns and themes within qualitative data. As a result, users can uncover deeper insights and make more informed decisions. Even those who have traditionally relied on manual methods for coding can benefit from its user-friendly interface. Ultimately, the integration of automated interview analysis not only streamlines the research process but also elevates the overall quality of qualitative research outcomes.
- ATLAS.ti: Strengths in data management and analysis.
Automated Interview Analysis can greatly benefit from powerful tools designed for effective data management and analysis. One such tool excels by making data handling efficient and intuitive. Its capabilities include immediate analysis kits, which allow users to query specific themes and codes with just a click. This intuitive approach enables researchers to gain relevant insights quickly while also aligning them with specific use cases, such as identifying risks or improving member experiences.
Additionally, the tool provides sentiment analysis and theme clustering, streamlining the data interpretation process. Users can define goals for their analysis, helping to focus on necessary insights. This capability not only saves significant time but also allows for a more structured understanding of participant responses. By automating these processes, researchers can unlock deeper insights without the cumbersome manual coding typically associated with interview analysis. Ultimately, these strengths position it as a valuable asset in conducting efficient and insightful text-based interviews.
- QDA Miner: Capabilities in processing interview data.
QDA Miner offers powerful capabilities for processing interview data, making it an essential tool for automated interview analysis. Its unique features allow analysts to efficiently transcribe, categorize, and analyze interview content without the burden of manual coding. Users can upload their data in bulk, enabling quick access to insights gathered across multiple interviews, which streamlines the analysis process significantly.
The software employs advanced techniques such as theme extraction and sentiment analysis to provide detailed perspectives on qualitative data. Additionally, QDA Miner allows users to generate visualizations and reports, helping to translate complex data into actionable insights. The platformโs native transcription service supports over 60 languages and boasts a high accuracy rate. This feature enhances the automation process further, ensuring that the data can be analyzed swiftly and accurately. Overall, QDA Miner stands as a vital resource for those looking to enhance their interview analysis practices through automation.
Conclusion: Advancing Through Automated Interview Analysis
Automated Interview Analysis marks a significant step forward in the landscape of qualitative research. By eliminating the cumbersome process of manual coding, researchers can now focus more on deriving actionable insights from their data. This innovation not only enhances efficiency but also reduces the risk of bias that often accompanies manual interpretations.
The implementation of automated tools empowers teams to deliver faster, more reliable outcomes. As organizations strive for quick turnarounds, embracing automated methodologies becomes crucial for maintaining relevance in an increasingly data-driven world. Ultimately, advancing through automated interview analysis transforms how insights are generated, ensuring that the focus remains on understanding the human experiences underlying the data.
### Leveraging Automated Interview Analysis Methods
To fully grasp how automated interview analysis can streamline research, it's crucial to first understand the limitations of manual coding. Manual coding is often time-consuming, requiring extensive resources which can hinder timely insights. By shifting to automated analysis, researchers can expedite the processing of interview data, thus allowing for a more efficient workflow.
Automated tools leverage Natural Language Processing (NLP) and Machine Learning to analyze vast amounts of text. These technologies enhance accuracy, eliminating human error while identifying patterns and themes within the data. By utilizing these advanced methods, researchers gain deeper insights and can focus on strategic analysis rather than getting bogged down with tedious coding tasks. The transition to automated tools not only facilitates data management but also propels the quality of insights generated. As researchers embrace these technologies, they position themselves for more impactful outcomes in their studies.