How to Compare Multiple Transcribed Interviews Using AI
-
Bella Williams
- 10 min read
In today's fast-paced research environment, AI Interview Analysis has emerged as a game-changer for qualitative insights. Traditional methods of analyzing interviews can be painfully slow, often leading to inconsistent results and delayed reports. By leveraging AI, researchers can swiftly compare multiple transcribed interviews, ensuring more accurate and timely insights for their clients.
The importance of AI Interview Analysis goes beyond mere efficiency. It fosters a deeper understanding of patterns and themes within the data, allowing researchers to make informed decisions. By automating repetitive tasks, teams can focus on interpreting the findings rather than getting bogged down in manual processes. Ultimately, embracing AI tools is not just about speed; itโs about enhancing the quality of insights derived from interviews.
Analyze & Evaluate Calls. At Scale.
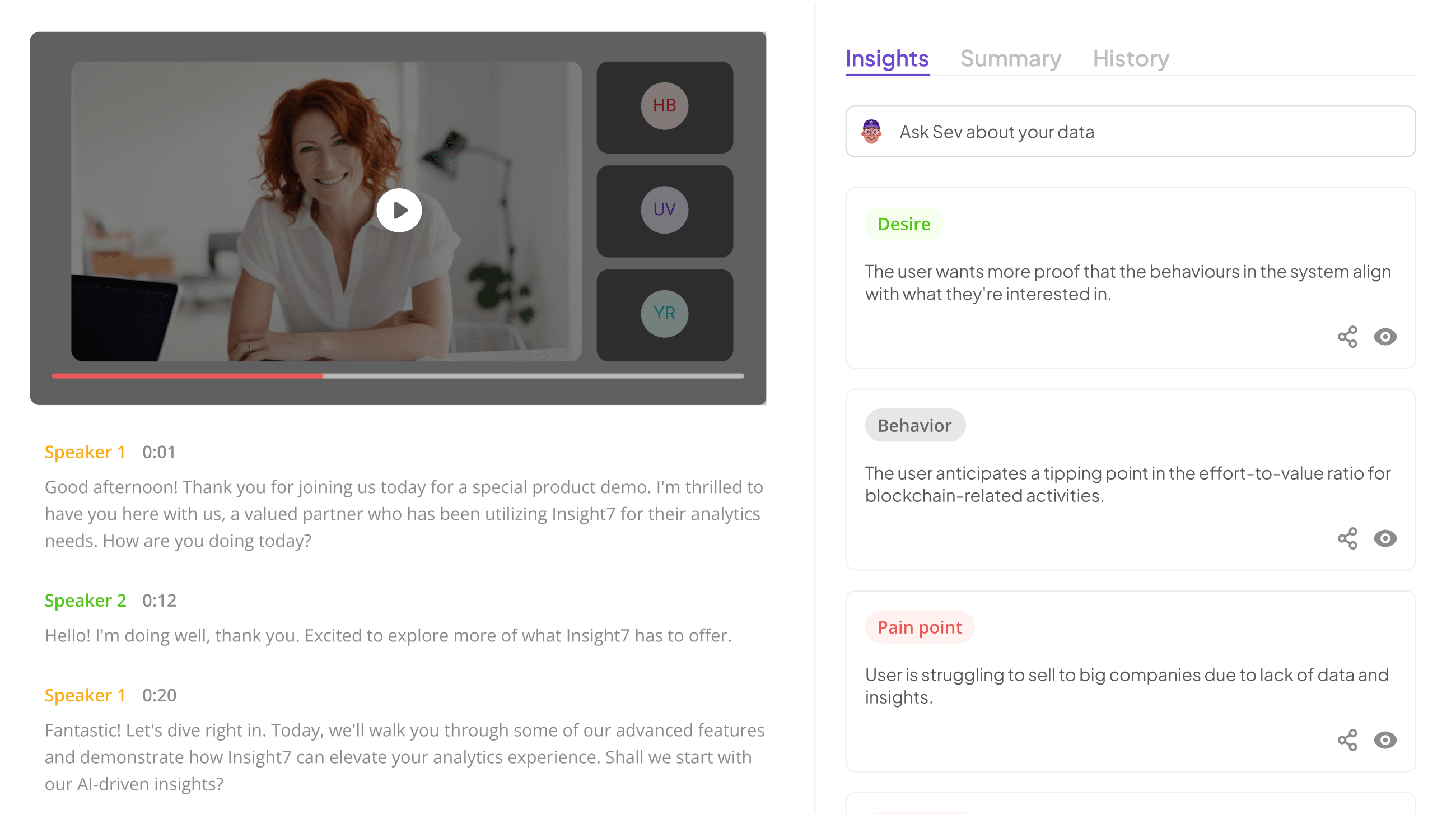
Streamlining the Process: AI Interview Analysis Techniques
Efficiently analyzing interviews is crucial for delivering timely insights. AI interview analysis techniques can significantly streamline the process by automating complex tasks that traditionally consume extensive time and effort. By utilizing advanced algorithms, AI can quickly identify common themes, allowing researchers to gain valuable insights faster than ever before. This automated text comparison helps eliminate biases that often occur when multiple analysts interpret data through different lenses.
In addition to text comparison, AI-powered tools also excel in keyword and topic extraction. These technologies capture key topics discussed in interviews, facilitating a comparative analysis that highlights both similarities and differences across multiple conversations. This dual approach not only enhances the depth of qualitative research but also improves the overall reliability of the findings. By integrating AI interview analysis techniques, research teams can focus more on strategic decision-making and less on manual data processing, ultimately leading to more insightful and balanced results.
Automated Text Comparison
Automated text comparison utilizes advanced algorithms to compare multiple transcribed interviews efficiently. This technique allows for the identification of patterns, themes, and sentiments across different interviews, making it easier to glean insights from large volumes of data. By processing text through AI, researchers can detect common threads and variations that might otherwise be overlooked during manual review.
One key advantage of AI interview analysis is its ability to perform sentiment analysis, which gauges the emotional tone behind the words. This capability can reveal how participants feel about specific topics, providing deeper context to qualitative data. Additionally, automated text comparison supports keyword and topic extraction, helping to highlight essential themes across multiple interviews. As a result, researchers can generate comprehensive reports that inform decisions with accuracy and speed, allowing for more effective presentations of findings.
- Using Algorithms for Pattern Recognition: How AI identifies common themes.
AI Interview Analysis employs various algorithms designed to identify patterns among transcribed interviews. These algorithms analyze the text for recurring themes, allowing researchers to cluster insights into meaningful categories. By employing techniques like natural language processing, AI can efficiently sift through large volumes of data, identifying similarities and differences among participant responses. As a result, researchers can gain a comprehensive view of perspectives and sentiments, enhancing their analysis process.
One effective method is sentiment analysis, where AI determines the emotional tone of responses. This enables researchers to understand participants' feelings regarding specific topics, uncovering underlying sentiments that may not be explicitly stated. Additionally, tailored keyword extraction algorithms can highlight critical topics, further refining the focus on essential areas. Together, these AI-powered tools not only expedite the analysis but also ensure that insights are derived from a nuanced understanding of the interviews, ultimately improving decision-making processes based on the gathered insights.
- Sentiment Analysis: Understanding emotional tone through AI.
Sentiment analysis is a critical aspect of AI interview analysis, enabling the identification of participants' emotional tones. By applying algorithms, AI can discern positive, negative, or neutral sentiments expressed during interviews. This technology helps researchers understand not only the content but also the feelings associated with various responses, adding depth to qualitative data analysis.
The process begins with transcribed interviews fed into sentiment analysis models, which evaluate language patterns, word choices, and context. Utilizing these insights, analysts can detect prevailing emotions that might influence the overall findings of a study. For example, if multiple interviewees express frustration, it signals areas that require immediate attention or improvement. Ultimately, grasping emotional nuances allows for a comprehensive understanding of interview data, revealing hidden insights that might otherwise remain overlooked.
Keyword and Topic Extraction
Keyword and topic extraction is a crucial step in AI interview analysis, as it helps in distilling vast amounts of qualitative data into digestible insights. These AI-powered tools analyze transcribed interviews and automatically identify key themes and keywords. This process allows researchers to uncover underlying patterns that may not be immediately apparent, facilitating deeper understanding and analysis.
The first phase involves extracting keywords that encapsulate important concepts, sentiments, and topics from the interviews. Next, comparative analysis comes into play, enabling the evaluation of similarities and differences across multiple interviews. By clustering insights, researchers can easily visualize how different participants contribute to specific themes. This organized approach not only saves time but also enhances the overall quality of analysis, providing significant value when assessing participant perspectives and responses. Thus, integrating keyword and topic extraction into AI interview analysis is essential for generating actionable insights from research data.
- AI-Powered Tools: How AI extracts key topics from interviews.
AI has transformed how we analyze interviews by providing powerful tools that extract key topics effectively. In AI interview analysis, automated systems enable researchers to quickly identify recurring themes without the extensive time commitment typically associated with manual analysis. By utilizing algorithms designed for this purpose, AI can sift through large volumes of data to pinpoint critical insights, ensuring researchers capture essential information efficiently.
Moreover, these tools enhance the consistency of findings across different interviews, mitigating biases that may arise from individual interpretation. As AI identifies key topics, it streamlines the comparative analysis process, allowing teams to draw meaningful parallels and variations among interviews. This efficiency not only expedites report generation but also enhances the overall quality of insights derived from qualitative data. In this way, AI-powered tools provide a reliable solution for those seeking to harness interview data more effectively, ultimately driving more informed decision-making.
- Comparative Analysis: Studying similarities and differences across interviews.
Comparative analysis in AI interview analysis focuses on uncovering both similarities and differences across multiple interview transcripts. This stage is essential for synthesizing findings, as it enables researchers to identify recurring themes and unique insights that emerge from diverse voices. By leveraging AI-powered tools, one can efficiently compare the emotional tones and the underlying messages conveyed in the interviews, enhancing understanding of the data at hand.
To effectively conduct a comparative analysis, consider the following steps:
Identify Key Themes: Use algorithms to detect common threads among the interviews. This allows for a comprehensive understanding of participants' perspectives.
Analyze Emotional Tone: Employ sentiment analysis to gauge the emotional nuances in each interview. Recognizing sentiment differences can reveal deeper insights beyond surface conversations.
Through these methods, researchers can achieve a more nuanced comprehension of the qualitative data. Embracing AI in this comparative analysis enhances accuracy and reduces the time taken to draw meaningful conclusions from interviews.
Extract insights from interviews, calls, surveys and reviews for insights in minutes
Top Tools for AI Interview Analysis
When it comes to AI interview analysis, several tools can significantly enhance your ability to compare and extract insights from multiple transcribed interviews. The right toolkit can streamline the entire process, saving valuable time while minimizing errors and biases often present in manual analysis. Leading the pack is Insight7, which boasts innovative features designed to simplify the extraction of key themes and sentiments from interviews. Its user-friendly interface allows researchers to efficiently navigate complex data, making it a noteworthy option for any analysis project.
Additionally, other tools like Otter.ai and Sonix provide robust transcription capabilities, making it easy to analyze large volumes of text. Otter.ai excels in live captioning, while Sonix offers advanced filtering options that help isolate specific themes. Descript stands out for its seamless editing functionalities, allowing users to refine their findings rapidly. On the other hand, Temi ensures quick turnaround times for accurate transcripts, and Trint's editing capabilities allow for in-depth analysis, making it a great choice for researchers aiming to derive actionable insights from their interviews.
๐ฌ Questions about How to Compare Multiple Transcribed Interviews Using AI?
Our team typically responds within minutes
Insight7: Leading the Way
In the realm of AI Interview Analysis, Insight7 stands out as a pioneering platform. It sets the benchmark for comparing multiple transcribed interviews effectively. With a robust suite of features designed for detailed analysis, it simplifies complex tasks. Users can effortlessly identify key themes, sentiment, and insights from diverse interviews, allowing them to make informed decisions faster.
To appreciate how Insight7 leads the way, consider its ability to execute advanced text comparisons and thematic extractions. First, it employs algorithms to detect recurring patterns and themes, making the process less time-consuming. Secondly, it utilizes sentiment analysis to gauge emotional tones across different conversations. This dual approach not only enhances the analysis but also amplifies user understanding of underlying issues, leading to better results in decision-making. Through these innovative capabilities, Insight7 ensures that users experience a seamless transition from data collection to actionable insights.
- Innovative Features: Why Insight7 tops the list for interview analysis.
Insight7 stands out in the realm of AI interview analysis with its innovative features that enhance transcription and interpretation processes. This platform excels by offering automated text comparison, which efficiently identifies patterns and themes across multiple interviews. With advanced algorithms, Insight7 not only captures sentiments but also distills complex conversations into actionable insights, making it easier for teams to understand diverse viewpoints.
Another key feature is the robust keyword and topic extraction. This ensures that researchers can quickly identify pivotal themes, allowing for easier comparative analysis. Consequently, stakeholders can readily spot similarities and discrepancies that might emerge from various interviews, significantly streamlining the analysis process. Insight7's unique combination of speed, accuracy, and user-friendliness positions it as the top choice for organizations looking to foster deeper understanding through AI interview analysis. By facilitating richer insights and reducing manual effort, it empowers teams to embrace a more efficient approach to research.
- Use Case Scenarios: Practical applications of Insight7.
Understanding the potential of AI Interview Analysis can greatly enhance your qualitative research. Imagine using a platform where interviews can be effortlessly transcribed and compared side-by-side. Insight7 enables you to import multiple interviews and cluster them into themed projects, offering a nuanced approach to data analysis. By organizing your transcripts in one location, analyzing them becomes a streamlined task, allowing you to focus on deriving meaningful insights.
In practical applications, Insight7 empowers users to extract key themes, quotes, and actionable insights from interviews. Whether you are conducting user research or exploring market trends, the AI capabilities assist in identifying common patterns and emotional tones. Users can generate specific analysis kits tailored to various objectives, providing a faster and more effective analysis process. With Insight7, researchers can turn transcribed interviews into valuable insights, making it an essential tool for understanding complex data landscapes.
Other Tools for AI Interview Analysis
In addition to popular options like Otter.ai and Descript, there are numerous other tools for AI interview analysis that can significantly enhance your workflow. For instance, Sonix offers advanced filtering capabilities that allow users to categorize and sort transcripts based on various parameters. This makes it easier to isolate specific themes or responses in large datasets, streamlining the analysis process efficiently.
Another noteworthy tool is Temi, which is recognized for its quick turnaround times and high accuracy in transcription. The rapid generation of transcripts can be invaluable, especially when time is of the essence. Trint also stands out with its robust editing functionalities, enabling teams to easily fine-tune their transcripts to match the required format.
Each of these tools can play a pivotal role in enhancing AI interview analysis, making it easier to draw insights that drive better decision-making. Selecting the right tool based on workflow needs can lead to a more effective and efficient analysis process.
- Otter.ai: Features and benefits for transcription analysis.
The utilization of advanced transcription tools significantly enhances AI interview analysis. One of the most notable features of such a tool is its ability to provide seamless transcription from various audio and video sources. Users can effortlessly upload multiple files, allowing for bulk transcription without the need for additional software. This efficiency is particularly beneficial when comparing multiple transcribed interviews, as it reduces time and effort while maintaining accuracy.
Furthermore, the transcription service offers users the capability to analyze transcripts collectively. After transcribing, users can delve into extracting themes, quotes, and relevant clips, which simplifies the comparative analysis. The analysis becomes more insightful, enabling the identification of patterns and trends across interviews. Data privacy is given utmost importance, ensuring compliance with standard regulations. Overall, these features make it an invaluable asset for researchers and analysts looking to derive meaningful insights from their interview data.
- Sonix: Advanced filtering options.
Advanced filtering options enhance the process of AI Interview Analysis, allowing users to refine their search within transcribed data. This feature enables users to quickly pinpoint specific themes or sentiments across various interviews, significantly enhancing analysis efficiency. By applying filters based on keywords, speaker identification, and date ranges, users can create tailored results that lead to deeper insights and more informed conclusions.
Furthermore, advanced filtering options facilitate comparative analyses between multiple interviews. Users can observe how different participants addressed similar topics, or identify variations in emotional tone. This capability not only streamlines the workflow but also provides a robust structure for uncovering meaningful data patterns and contrasts. In the realm of AI Interview Analysis, these advanced features empower researchers to synthesize complex information into actionable insights, optimizing the overall research experience.
- Descript: User-friendly interface and functionality.
Using an intuitive interface, this platform enables users to tackle AI Interview Analysis effortlessly. Designed with simplicity in mind, it avoids overwhelming functionalities, allowing anyone to begin analyzing transcribed interviews right away. Central to the experience are two main areas: the 'Library' and 'Projects'. The Library houses all your data, while Projects is where users cluster related files together for efficient access and analysis.
Once interview transcripts are imported, whether through direct upload or transcription from videos, the analysis process can commence seamlessly. Users can pull insights, identify themes, and generate summaries with ease. Additionally, pre-designed kits aid in focused analysis, offering a quick route to extracting meaningful insights tailored to specific research needs. Overall, the user-friendly interface promotes a smooth workflow, making AI Interview Analysis accessible even to those with minimal technical expertise.
- Temi: Quick turnaround time and accuracy.
When evaluating AI interview analysis, the emphasis on quick turnaround time and accuracy is paramount. Utilizing advanced algorithms, AI tools can process large volumes of transcribed interviews swiftly, thus enabling researchers to focus on insights rather than get bogged down by analytical delays. The speed at which AI can transcribe and analyze interviews allows for timely reporting, essential for keeping pace with client demands.
Accuracy is equally crucial as it ensures that derived insights are reliable and actionable. By employing techniques like automated text comparison and keyword extraction, AI tools minimize human error and provide consistent results across interviews. This combination of speed and precision fosters a seamless workflow, making it an ideal choice for professionals seeking to enhance their qualitative research capabilities. Ultimately, leveraging AI for interview analysis leads to quicker insights that are grounded in solid data, driving more informed decision-making in various sectors.
- Trint: Powerful editing capabilities for interview analysis.
Trint offers robust editing capabilities specifically designed for interview analysis, making it a go-to tool for researchers and analysts alike. With its user-friendly interface, you can effortlessly upload and transcribe multiple interviews, providing a streamlined workflow that saves significant time. The platform supports over 60 languages, delivering high accuracy in transcriptions, which is crucial for reliable AI interview analysis.
Once your interviews are transcribed, Trint enables you to highlight key themes, quote excerpts, and generate visualizations from your data. This facilitates an in-depth comparative analysis of different interviews, allowing you to identify patterns and draw actionable insights quickly. Additionally, the ability to ask specific questions across your data set enhances your analytical capabilities, paving the way for deeper understanding and improved decision-making processes in your projects. In an era where data privacy is paramount, Trint also ensures compliance with regulations, giving you peace of mind as you work with sensitive information.
Conclusion: Embracing AI Interview Analysis for Effective Results
In conclusion, embracing AI interview analysis opens new avenues for achieving effective results. By automating tedious tasks, organizations can significantly reduce the time spent on manual analysis. This efficiency not only streamlines workflows but also ensures a more consistent and unbiased examination of data. Using AI tools helps researchers uncover valuable insights that might otherwise be overlooked, ultimately enhancing the quality of reports.
Moreover, adopting AI interview analysis empowers teams to focus on strategic decision-making rather than getting bogged down in minutiae. By leveraging advanced technologies to compare multiple transcribed interviews, businesses can gain a comprehensive understanding of participant sentiments and themes. This approach fosters better-informed choices, ensuring that insights are both actionable and relevant in a competitive landscape.
๐ฌ Questions about How to Compare Multiple Transcribed Interviews Using AI?
Our team typically responds within minutes