AI-Driven Interview Analysis marks a transformative approach to understand customer sentiments and experiences. As businesses increasingly rely on qualitative data from interviews, traditional manual analysis methods can be both time-consuming and error-prone. This often leads to biases and discrepancies in insights when multiple researchers interpret the same data differently.
By integrating AI tools into the interview analysis process, teams can streamline data processing and enhance consistency. AI can efficiently sift through vast amounts of qualitative information, identifying patterns and themes that might otherwise be overlooked. Ultimately, embracing AI-driven solutions not only accelerates analysis timelines but also empowers organizations to derive actionable insights from customer interviews.
Analyze & Evaluate Calls. At Scale.
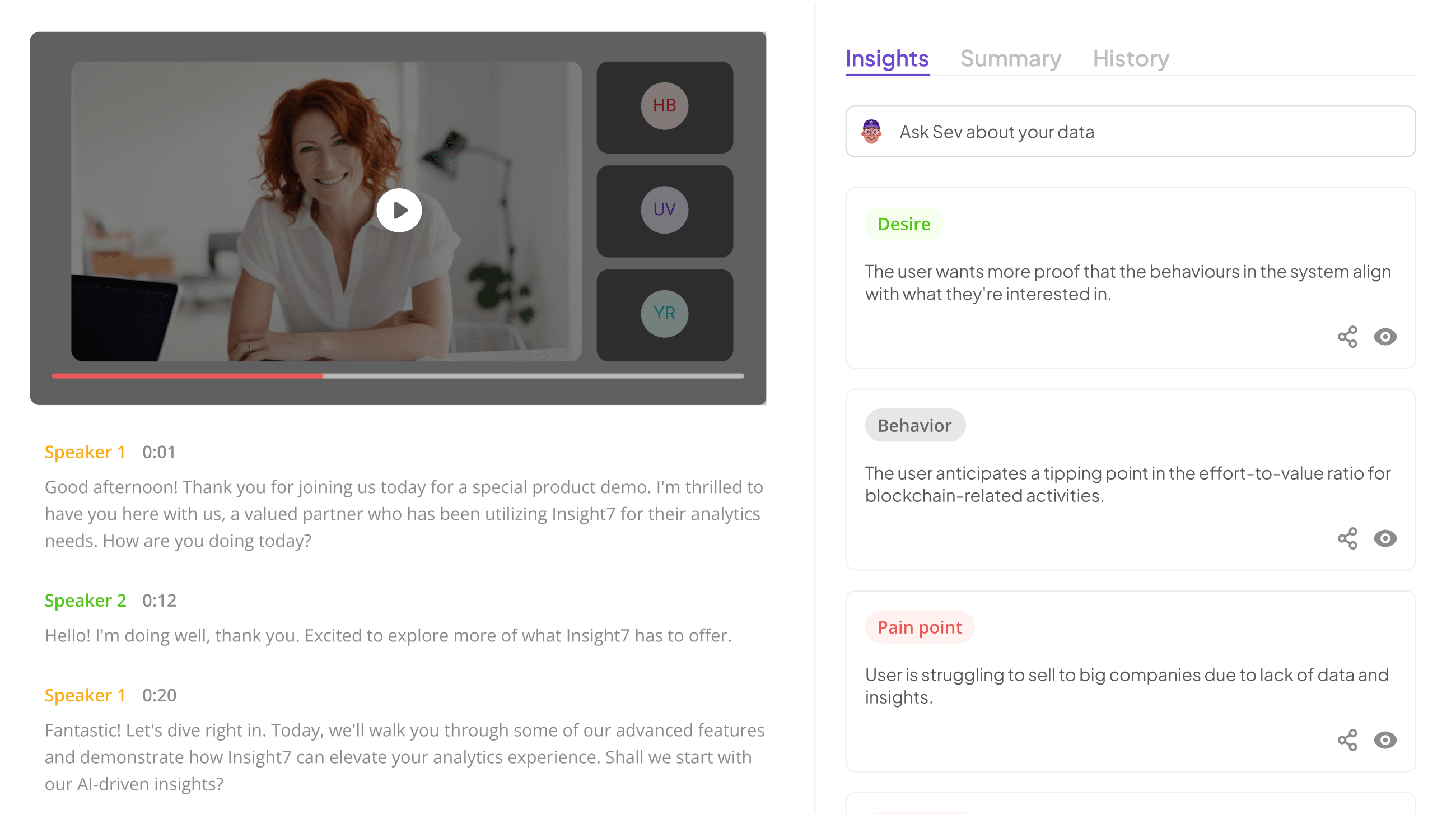
The Power of AI-Driven Interview Analysis
AI-Driven Interview Analysis is revolutionizing how we interpret customer research interviews. Traditional methods can be tedious and prone to bias, often leading to inconsistent insights across a team. With AI tools, the process becomes streamlined, allowing for faster, more accurate analysis of interview data. This technology can identify themes and sentiments that may not be immediately visible to human analysts, revealing deeper customer insights.
The power of AI tools extends beyond mere efficiency; they enhance the quality of analysis by using algorithms to minimize human error. For instance, AI can sift through large volumes of interview transcripts, extracting critical patterns and nuances. This not only saves time but also helps teams focus on strategic decision-making. Ultimately, embracing AI-Driven Interview Analysis equips organizations to make informed, data-backed decisions that drive customer satisfaction and business growth. By transforming how we analyze interviews, AI tools position us to better understand our customers and their needs.
Understanding the Importance of Customer Research
Customer research is essential for understanding the needs and preferences of your target audience. Gathering insights directly from customers helps businesses tailor their products and services to meet market demands. This is where AI-Driven Interview Analysis comes into play, enhancing data interpretation by identifying key themes and sentiments from qualitative feedback.
Effective customer research not only improves product offerings but also informs strategic decision-making. By utilizing AI tools to analyze interview data, organizations can uncover actionable insights that may have gone unnoticed. This analysis makes it easier to detect patterns, allowing businesses to adapt quickly to shifts in customer expectations. Ultimately, a strong commitment to understanding customer perspectives fosters loyalty and drives long-term growth. Embracing AI in this process equips businesses with the ability to understand their customers on a deeper level, paving the way for improved engagement and satisfaction.
Benefits of Utilizing AI Tools for Interview Analysis
Utilizing AI tools for interview analysis brings several advantages that significantly enhance the efficiency and accuracy of understanding customer insights. Primarily, AI-driven interview analysis can process a large volume of interview data quickly and effectively, which reduces the time researchers spend sifting through information. As a result, teams are able to generate insights more rapidly, meeting the increasing demand for timely reports.
Moreover, AI tools can minimize bias and inconsistency, which are common challenges in manual data interpretation. By standardizing the analysis process, these tools ensure that every researcher pulls insights from the same framework, leading to more reliable conclusions. With the integration of AI, companies can empower their teams to focus on deep analysis and actionable recommendations, rather than getting bogged down in data processing. This transformative approach not only streamlines workflows but also elevates the quality of insights derived from customer interviews, making it a game-changer for research efficiency.
Tools for AI-Driven Interview Analysis
AI-Driven Interview Analysis offers a transformative approach to understanding customer insights. By utilizing advanced technologies, teams can efficiently analyze interviews, reducing manual efforts while uncovering meaningful patterns. This shift not only enhances the accuracy of insights but also streamlines the reporting process, allowing researchers to focus on strategic decision-making.
Several AI tools can optimize your analysis workflow. For instance, Sonar excels at sentiment analysis, helping to gauge customer emotions effectively. Clarabridge specializes in text analytics, providing actionable insights regarding customer experiences. Otter.ai simplifies transcription and can identify key themes in your spoken data, making the analysis process easier. Lastly, MonkeyLearn uses machine learning for flexible text analysis, adapting to your specific research needs. Leveraging these tools leads to faster insights and greater consistency across research teams, ultimately enhancing the quality of customer understanding.
Extract insights from interviews, calls, surveys and reviews for insights in minutes
Insight7: Transforming Customer Insights with AI
AI-Driven Interview Analysis offers a transformative approach to extracting deep insights from customer research interviews. Traditional methods, often reliant on manual processes, can lead to biases and oversight of critical data. By integrating AI tools, organizations can streamline this process, uncovering patterns and trends that might otherwise go unnoticed. The enhanced accuracy of AI enables businesses to gain a clearer understanding of customer sentiments and preferences.
One of the key advantages of AI-Driven Interview Analysis is its ability to process large volumes of qualitative data rapidly. This efficiency not only speeds up the analysis phase but also allows teams to focus on strategy and decision-making. AI tools can identify recurring themes in customer feedback, facilitating data-driven decisions that enhance business strategies. By implementing these innovative technologies, businesses can effectively respond to customer needs, driving growth and satisfaction.
Other Noteworthy Tools
When exploring AI-driven interview analysis, several noteworthy tools stand out. Each tool provides unique features that enhance the interviewing process and deliver actionable insights. First, consider Sonar, which specializes in sentiment analysis. This tool effectively captures emotional tones and helps decode participant reactions, allowing researchers to gauge feelings behind statements. Then there's Clarabridge, known for its robust text analytics capabilities. It not only organizes data but also extracts significant insights regarding customer experiences.
Another essential tool is Otter.ai, which simplifies the transcription process while also identifying keywords. Its ability to provide discussive keywords can open new avenues for understanding customer needs. Lastly, MonkeyLearn offers customizable text analysis through machine learning. Users can train the tool to recognize specific patterns related to their research goals. Together, these tools illustrate the potential of AI-driven interview analysis to transform raw data into meaningful insights effortlessly.
- Sonar: A comprehensive tool for sentiment analysis.
In the realm of sentiment analysis, Sonar stands out as a comprehensive tool tailored to enhance understanding of customer feedback. Utilizing advanced algorithms, Sonar effectively decodes the emotional nuances embedded in customer research interviews. This tool empowers businesses by transforming subjective opinions into quantifiable insights, providing clarity amid the chaos of data.
With its intuitive interface, Sonar allows users to upload interview transcripts directly into the platform. The tool employs sophisticated AI-driven analysis techniques to categorize sentiments as positive, negative, or neutral. By offering a clear visualization of the emotional landscape, Sonar supports informed decision-making, enabling teams to strategize effectively based on customer feelings. For anyone looking to harness AI-driven interview analysis, Sonar serves as an invaluable resource in distilling rich qualitative data into actionable insights.
- Clarabridge: Specializes in text analytics and customer experience insights.
In todayโs competitive landscape, understanding customer sentiment is crucial for businesses aiming to enhance their customer experience. Text analytics has emerged as an essential tool that leverages AI capabilities to sift through vast amounts of qualitative data derived from customer interviews. By transforming unstructured data into coherent insights, companies can better grasp customer feelings, preferences, and pain points.
The value of analyzing customer research interviews lies in identifying patterns and trends that can help organizations make informed decisions. With advanced text analytics, businesses can automate the process of synthesizing interview data, leading to faster and more precise insights. Implementing these tools enables companies to adapt and innovate in response to customer needs, thereby fostering a more engaging and user-centric experience. As organizations embrace AI-driven interview analysis, they unlock the potential to drive strategic growth.
- Otter.ai: Facilitates transcription and keyword identification.
Transcription accuracy is crucial in the realm of customer research interviews, as it directly impacts the insights derived from the data. An AI-driven tool excels in this area by transforming spoken dialogue into text with impressive precision. This feature not only saves time but also ensures that every detail is captured without the manual effort of traditional transcription methods.
Once the interviews are transcribed, the tool enhances the user experience through keyword identification. By extracting significant phrases and concepts, researchers can focus on the core themes without getting lost in the details. This dual functionality aids in synthesizing the information effectively, paving the way for deeper analysis. As a result, the process of conducting customer research interviews becomes not just simpler but also far more insightful, enabling teams to make informed decisions based on robust data.
- MonkeyLearn: Offers flexible text analysis using machine learning.
AI-Driven Interview Analysis enhances the examination of customer research interviews through powerful machine learning techniques. In a world where understanding customer sentiment is paramount, this technology offers a flexible text analysis solution. By ingesting varied data formats, it efficiently converts audio and video interviews into transcribed text, simplifying the review process.
Once the data is transcribed, users can analyze individual interviews or compare multiple sessions within a project. This capability allows for thematic extractions, quote collections, and comprehensive reporting. The AI analyzes these insights to inform key questions, thus facilitating a deeper understanding of customer perceptions. Additionally, the emphasis on data privacy and compliance ensures that organizations can harness these insights securely and confidently. As you develop your customer research strategy, integrating this innovative approach can lead to clearer, actionable insights that drive business success.
Steps to Conduct AI-Driven Interview Analysis
Preparing for AI-Driven Interview Analysis begins with gathering and organizing your interview data. Ensure that all transcripts are accurately captured, whether through manual transcription or AI-based tools. Properly structured data is vital as it minimizes errors in subsequent steps. Clean up the data by removing irrelevant content, which helps focus the analysis on valuable insights.
Next, apply suitable AI tools to analyze the prepared data. These tools can provide deeper insights by identifying patterns, sentiments, and themes that may not be immediately apparent. After the analysis, interpreting the AI-generated insights is crucial. Look for actionable takeaways that can inform business decisions or strategy adjustments. By following these steps, you can streamline your interview analysis, reduce bias, and enhance the consistency of the insights gathered. Embracing these methodologies positions you to derive more detailed customer insights effectively.
Step 1: Preparing Your Interview Data
To begin your AI-Driven Interview Analysis, thorough preparation of your interview data is essential. This initial step involves organizing and cleaning your data to ensure accurate analysis. Start by transcribing your interview recordings, which can be simplified using specialized transcription services. Accurate transcription is crucial as it forms the foundation for deriving insights later on.
Next, categorize your data meaningfully based on themes or key topics discussed during interviews. This organization aids AI tools in effectively recognizing patterns and extracting relevant insights. When your data is well-structured, AI algorithms can work more efficiently, offering deeper analysis. Finally, ensure that your data complies with privacy regulations, maintaining security and confidentiality. By meticulously preparing your interview data, you set the stage for a streamlined AI analysis process that leads to actionable insights and informed decision-making.
Step 2: Applying AI Tools for Deep Analysis
Applying AI tools for deep analysis is crucial in extracting meaningful insights from customer research interviews. With AI-driven interview analysis, companies can streamline their processes and reduce the time spent on manual review. Utilizing AI allows teams to automatically aggregate common themes, leading to more consistent and unbiased conclusions across their research.
To effectively implement AI tools, consider the following steps:
- Data Preparation: Ensure your interview transcripts are clean and well-organized, making them suitable for analysis.
- Tool Selection: Choose appropriate AI tools tailored to your specific needs, such as keyword extraction or sentiment analysis.
- Implementation: Integrate the AI tools into your analysis workflow to enable efficient processing of large data sets.
- Insight Generation: Focus on interpreting the findings generated by the AI tools to distill actionable insights for your business.
By harnessing AI tools, businesses can enhance their understanding of customer sentiment and refine their strategies more effectively.
Step 3: Interpreting AI-Generated Insights
Interpreting AI-generated insights requires a thoughtful approach to extract the most relevant information for decision-making. After utilizing AI tools in your interview analysis, it's essential to focus on the generated insights, identifying key themes and quotes that resonate with your research objectives. These insights, derived from AI-driven analysis, provide a foundation upon which you can build actionable strategies.
Begin by reviewing the AI-generated reports and summaries, which often highlight crucial findings. Pay attention to patterns and recurring themes that emerge from your interviews. This process not only helps in confirming initial hypotheses but also unveils unexpected insights that may inform your strategies. The beauty of AI-driven interview analysis lies in its ability to process vast data quickly, transforming raw information into valuable insights that can significantly advance your understanding of customer needs and preferences.
Conclusion: Embracing AI-Driven Interview Analysis for Customer Research Success
In today's fast-paced research environment, embracing AI-driven interview analysis is essential for gaining a competitive edge. By leveraging advanced AI tools, businesses can process interview data more efficiently, ensuring insights are generated quickly and accurately. This approach helps reduce the biases and inconsistencies that often arise when multiple researchers analyze interviews manually.
Furthermore, AI-driven analysis allows teams to focus on strategic decision-making rather than getting bogged down in time-consuming data processing. Ultimately, integrating these tools not only enhances the quality of insights derived from customer research but also paves the way for more meaningful interactions with clients. Adopting this methodology will undoubtedly lead to greater success in understanding customer needs and expectations.