How to Use AI to Build Feedback Loops from Interview Analysis
-
Hello Insight
- 10 min read
AI-Powered Feedback Loops are transforming how organizations analyze interview data and glean insights. As traditional methods can be time-consuming and often inconsistent, integrating AI-driven solutions offers a more streamlined approach to understanding responses. With AI's ability to automate calculations and minimize human bias, teams can focus on what truly matters: deriving actionable insights from interviews.
Incorporating these feedback loops into interview analysis not only accelerates the process but also enhances accuracy. By leveraging AI technologies, organizations can ensure they capture essential themes and trends effectively. The next steps involve implementing these tools, which will lead to more efficient reporting and ultimately better decisions informed by reliable data.
Analyze & Evaluate Calls. At Scale.
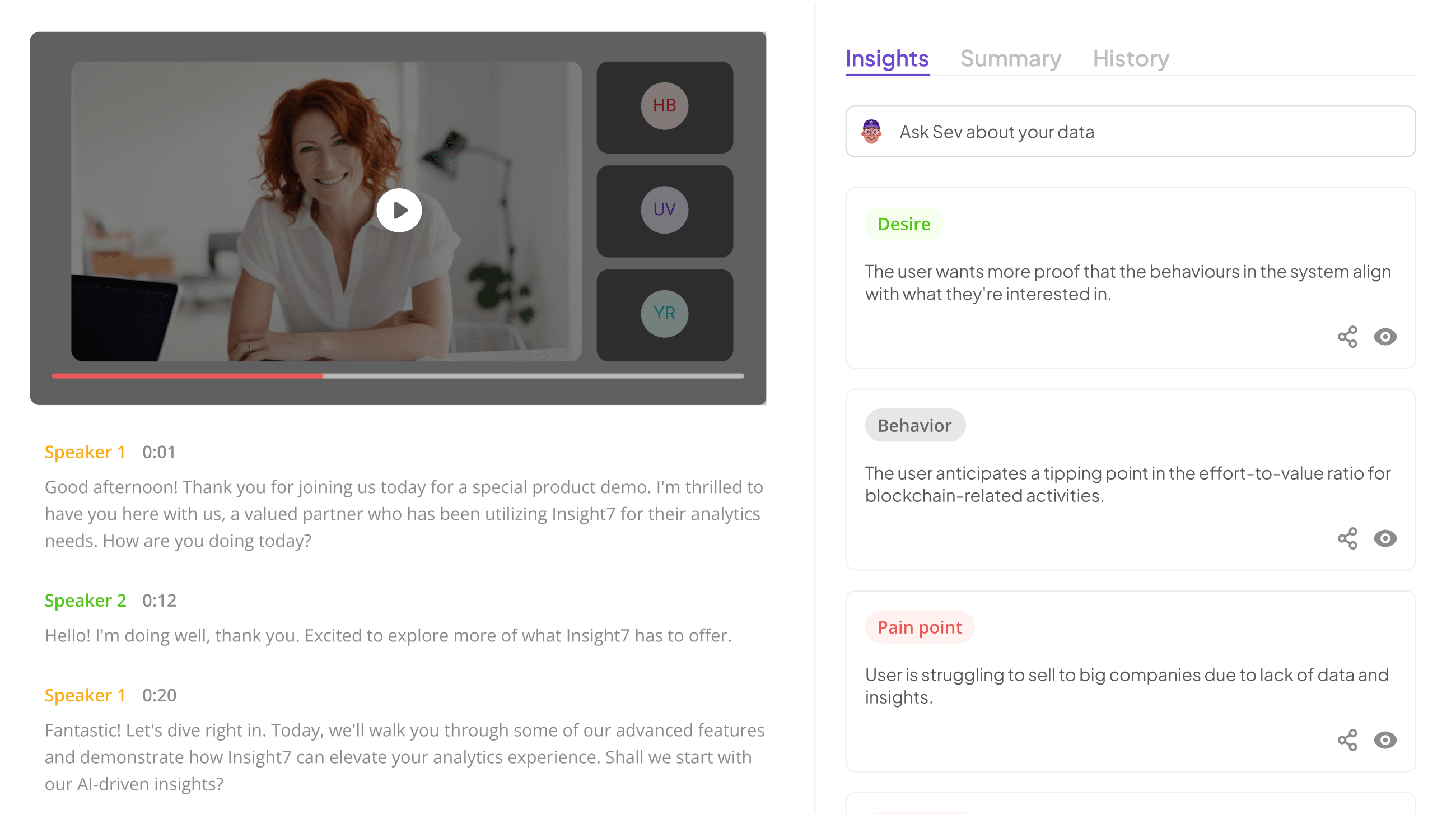
The Mechanics of AI-Powered Feedback Loops
AI-Powered Feedback Loops effectively integrate automated processes to enhance the analysis of interview data. By utilizing advanced algorithms, these systems can continuously learn from input data, ensuring that feedback remains relevant and actionable. The mechanics behind these loops involve several key elements: data collection, preprocessing, sentiment analysis, and automated feedback generation. Through each of these stages, AI identifies patterns, gauges sentiment, and evaluates responses to provide deeper insights.
The continuous nature of AI-Powered Feedback Loops means that organizations can adapt and refine their processes swiftly. As new interview data accumulates, AI analyzes it in real time, adjusting recommendations based on evolving trends. This dynamic feedback mechanism not only enhances understanding but also empowers teams to act swiftly on insights, ultimately leading to improved decision-making and stronger connections with audiences. The seamless integration of AI into feedback loops represents a significant advancement, making analysis more efficient and insightful than traditional methods.
Understanding the Role of AI in Feedback Loops
Artificial intelligence plays a pivotal role in establishing AI-Powered Feedback Loops, particularly from the analysis of interview data. This technology processes large volumes of qualitative information, transforming it into actionable insights. By automating the transcription of interviews and synthesizing key themes, AI allows organizations to respond swiftly to the evolving needs of their clients. Speed and agility are increasingly vital in today’s competitive landscape, making AI an essential tool for maintaining relevance.
Additionally, the integration of AI into feedback loops enhances both the accuracy and objectivity of insights captured during interviews. Advanced sentiment analysis enables organizations to recognize emotional tones, which can significantly impact decision-making processes. By clustering related responses, teams can identify trends and address client concerns more effectively. This streamlined approach fosters a continuous cycle of improvement, ultimately leading to better service offerings and heightened client satisfaction. Understanding these dynamics is crucial for businesses aiming to harness the power of AI in their feedback mechanisms.
Key Steps in Building Feedback Loops from Interview Data
To establish effective AI-Powered Feedback Loops from interview data, it's essential to follow structured steps. The first step involves thorough data collection and preprocessing. Ensure that all relevant interviews are documented clearly and organized effectively to facilitate analysis. This step sets the stage for subsequent actions by ensuring high-quality data is available for model training and evaluation.
The second step focuses on sentiment analysis and recognition. Utilize advanced AI algorithms to detect emotions and sentiments from the recorded interviews. Accurate sentiment detection helps identify key themes and concerns among participants. The next step includes categorization and clustering of responses to reveal patterns in feedback. By grouping similar responses, organizations can distill insights that improve decision-making processes.
Finally, automate feedback systems to ensure continuous engagement with participants. These systems should not only provide insights but also invite further feedback, creating a dynamic loop of interaction. When executed diligently, these steps will cultivate a robust AI-Powered Feedback Loop that enhances overall project outcomes.
- Step 1: Data Collection and Preprocessing
Effective data collection and preprocessing are foundational steps in establishing AI-Powered Feedback Loops from interview analysis. Begin by gathering relevant data, which may include audio recordings, transcripts, and notes from interview sessions. Organize this information efficiently to enable easy access and processing. Proper categorization at this stage is vital, as it directly influences the insights extracted later.
Once the data is collected, proceed to preprocess it, which involves transcribing audio to text if needed, cleaning the text by removing irrelevant content, and identifying themes or key topics. This helps create a structured dataset that enhances analysis accuracy. By defining themes such as employee engagement or performance management, you’ll generate targeted insights from the interviews. Ultimately, meticulous data collection and preprocessing pave the way for effective sentiment analysis and automated feedback mechanisms, driving improvements in your processes.
- Step 2: Sentiment Analysis and Recognition
In this stage, the focus is on sentiment analysis and recognition, which are critical to understanding interview feedback. Sentiment analysis utilizes algorithms to detect emotional tone in responses, providing insights into how candidates genuinely feel about specific topics. By identifying sentiments such as positive, negative, or neutral, organizations can better assess interview outcomes and candidate experiences. This step transforms qualitative data into actionable insights, highlighting areas needing improvement or further exploration.
Recognizing sentiment is vital for creating effective AI-powered feedback loops. It helps frame narratives within the data, allowing stakeholders to understand sentiments driving feedback. Moreover, this information aids in refining questions for future interviews, ensuring they elicit more targeted responses. By incorporating sentiment analysis into the feedback loop, companies can continuously enhance their interviewing processes, leading to more meaningful interactions and better recruitment outcomes. Embracing such technologies will pave the way for more insightful, data-driven decision-making.
- Step 3: Categorization and Clustering of Interview Responses
In this critical step, the focus shifts to categorizing and clustering interview responses to gain meaningful insights. By systematically organizing feedback, AI can identify patterns and trends within qualitative data. This approach allows researchers to make sense of varied opinions, showcasing common themes that emerge across different interviews. It is important to establish clear categories that reflect the main ideas expressed by participants, ensuring alignment with the research objectives.
Once responses are categorized, clustering tools can group similar insights, enabling faster identification of overarching themes. This process not only streamlines analysis but also aids in mitigating bias that may arise from individual interpretations. By utilizing these AI-powered techniques, organizations can create robust feedback loops that enhance decision-making and drive action. Ultimately, this categorization and clustering process transforms raw interview data into actionable insights, leading to improved strategies and outcomes.
- Step 4: Implementation of Automated Feedback Systems
Implementing automated feedback systems is a critical step in enhancing interview analysis through AI-powered feedback loops. These systems facilitate the timely collection and evaluation of feedback from various interviews, allowing for real-time adjustments and improvements. To begin, it is essential to establish the necessary technological infrastructure that supports automated feedback mechanisms. This ensures that valuable insights are not lost but analyzed comprehensively and efficiently.
Next, integrate AI tools that can collect, analyze, and report feedback seamlessly. These AI-driven solutions can transform raw interview data into actionable insights by identifying trends and patterns within responses. Additionally, ongoing monitoring and evaluation of these systems are essential to refine processes and address any emerging issues. Ultimately, the successful implementation of automated feedback systems not only strengthens the feedback loop but also empowers organizations to adapt and evolve based on insights drawn from interviews.
Extract insights from interviews, calls, surveys and reviews for insights in minutes
Tools and Technologies for AI-Powered Feedback Loops
In the realm of AI-Powered Feedback Loops, selecting the right tools is essential for maximizing the efficiency and effectiveness of your feedback systems. Various AI technologies are available that can significantly streamline the entire process. Firstly, tools like Otter.ai and Fireflies.ai can assist in transcribing interviews automatically, ensuring accurate data capture. This functionality allows teams to focus on analysis rather than manual transcription. Additionally, platforms such as Gong.io provide valuable insights into conversation dynamics, revealing customer sentiments and highlighting key trends.
Next, employing IBM Watson Tone Analyzer helps in assessing emotional undertones in responses, giving you a deeper understanding of customer perspectives. It allows for the categorization of feedback based on emotional context, elevating the quality of insights generated. By integrating these technologies, businesses can construct iterative feedback loops that evolve over time, ensuring that they remain adaptable and responsive to changing market demands. In conclusion, when building AI-Powered Feedback Loops, consider how these tools can work synergistically to produce actionable insights from interview analysis.
Leveraging AI Tools for Effective Feedback Loops
AI-Powered Feedback Loops transform the way organizations analyze interview data by integrating advanced tools and technologies. By using automated transcription and analysis software, teams can swiftly convert interviews into structured feedback. This streamlined process allows for faster identification of trends and issues, enabling organizations to respond efficiently. AI tools can analyze sentiment, categorize responses, and provide meaningful insights, significantly reducing the manual effort involved in traditional methods.
Implementing AI-powered tools enhances the effectiveness of feedback loops in several ways. Firstly, they facilitate real-time data collection and processing, which promotes an agile response to user insights. Secondly, these tools often include predictive analytics features, helping organizations anticipate needs and preferences. Lastly, utilizing a range of AI technologies fosters a data-driven culture, reinforcing continuous improvement in communication strategies. By embracing these solutions, organizations not only enhance their feedback mechanisms but also build more robust relationships with their stakeholders.
- insight7
Insight7 highlights the importance of integrating AI-Powered Feedback Loops into interview analysis, allowing organizations to turn insights into actionable strategies. The first step involves effectively collecting and preprocessing interview data to ensure it is comprehensive and structured. Accurate data collection lays the groundwork for a successful analytical process, which is key for generating useful feedback loops.
Next, AI can be utilized for sentiment analysis and recognition, identifying emotional tones and key themes within responses. This phase enables deeper understanding and prioritization of feedback based on the expressed sentiments of interviewees. Following this, categorization and clustering of responses are crucial for extracting patterns and trends, which can significantly enhance decision-making. Lastly, implementing automated feedback systems streamlines the flow of information, ensuring that insights gained from interviews translate into real-time adjustments and improvements in practices. Embracing these steps transforms raw data into valuable, actionable insights that foster a continuous feedback cycle.
- Otter.ai
In the realm of AI-powered feedback loops, some tools stand out for their innovative capabilities. One such tool excels at transforming spoken dialogue into accurate transcripts, making the analysis of interview data more efficient and reliable. This tool provides seamless integration with various video conferencing platforms and ensures that valuable insights are retained even when conversations become complex.
By harnessing AI, this tool enables teams to focus on extracting meaningful feedback rather than getting bogged down in manual transcription. The ability to store and organize data effectively fosters an environment where insights can be quickly reviewed and acted upon. When used strategically, the tool can significantly enhance the process of building feedback loops from interviews, offering a systematic approach to understanding user needs and concerns. This leads to actionable decisions based on comprehensive analysis, ultimately enriching user experiences.
- Fireflies.ai
AI-powered feedback loops play a vital role in transforming how we analyze interview data. One of the essential tools available can assist in optimizing this process efficiently. This tool is designed to seamlessly capture and analyze various data points from interviews, allowing for a more streamlined approach to understanding feedback and insights.
Through automated transcription and intelligent summarization features, it helps teams focus on key themes and sentiments expressed during interviews. This not only enhances clarity but also fosters improved decision-making within organizations. By utilizing real-time analytics, teams can adapt their strategies based on ongoing feedback. Ultimately, this integration contributes to a more effective feedback loop, bridging the gap between insight generation and actionable outcomes in a dynamic environment.
- Gong.io
In the realm of AI-powered feedback loops, one vital tool stands out for its ability to enhance insights from interview analysis. By capturing and analyzing conversations, this platform offers organizations a unique way to glean actionable insights. What sets this tool apart is its ability to provide a comprehensive overview of the discussions and identify patterns that may otherwise go unnoticed.
To effectively utilize the capabilities of this tool, organizations should focus on integrating it with existing workflows. This integration allows for seamless data collection and analysis, ensuring that valuable feedback is readily available. Additionally, the platform's strength lies in its data visualization capabilities, which can transform complex information into clear, understandable insights. By employing this tool in interview analyses, companies can create rich feedback loops that promote continuous improvement and informed decision-making.
- IBM Watson Tone Analyzer
The IBM Watson Tone Analyzer is a powerful tool that provides insights into the emotional tone of text data. By analyzing the transcription of interviews, it identifies various emotional cues, such as joy, sadness, anger, and fear, enhancing the understanding of user experiences. This capability is crucial for AI-powered feedback loops, as it allows organizations to gauge the emotional responses of interviewees.
When building AI-powered feedback loops using this technology, consider the following steps:
- Input Transcriptions: Begin by feeding transcribed content into the analyzer to assess the emotional tone displayed in responses.
- Analyze Emotional Tone: The tool assesses the tone, providing a breakdown of emotions connected to specific comments made during interviews.
- Collect Insights for Action: Use these insights to inform decision-making and develop strategies tailored to the emotional needs of customers or clients.
- Refine Feedback Mechanisms: Continually adjust feedback systems based on tone analysis findings to enhance communication and connection with your audience.
By integrating the Tone Analyzer into your workflow, you can create richer, more meaningful interactions with feedback, ultimately fostering deeper understanding and improved outcomes.
Evaluating and Selecting the Right Tools
When evaluating and selecting the right tools for creating AI-Powered Feedback Loops, several factors come into play. First, consider the specific needs of your project and the types of data you will be analyzing. Selecting tools that align with your objectives is crucial. Tools must efficiently handle data collection, analysis, and reporting while allowing for easy integration into your existing systems.
Next, assess the user interface and ease of use of potential tools. A user-friendly interface can significantly enhance your team’s productivity and ensure smoother collaboration. Look for tools with robust features such as sentiment analysis and automatic transcription. Finally, consider scalability and support options available. Choosing a solution that easily adapts to your growing needs will save effort and resources in the long run. Testing multiple tools allows you to identify which ones best suit your workflows and enhance your AI-Powered Feedback Loops efficiently.
Conclusion: Enhancing Interview Analysis with AI-Powered Feedback Loops
Integrating AI-Powered Feedback Loops into interview analysis can significantly improve the efficiency and reliability of insights gathered. By automating the analysis process, organizations can minimize bias and reduce manual workloads, enabling teams to focus on higher-value tasks. This leads to quicker turnaround times for reporting, which is essential in today's fast-paced environment where clients demand timely insights.
Moreover, these feedback loops create a continuous cycle of learning. As AI tools process more interviews, they can adapt and refine their analysis, providing deeper insights over time. By fostering a data-driven culture, organizations can enhance their decision-making processes and ultimately achieve better outcomes from their research initiatives.
Analyze & Evaluate Calls. At Scale.
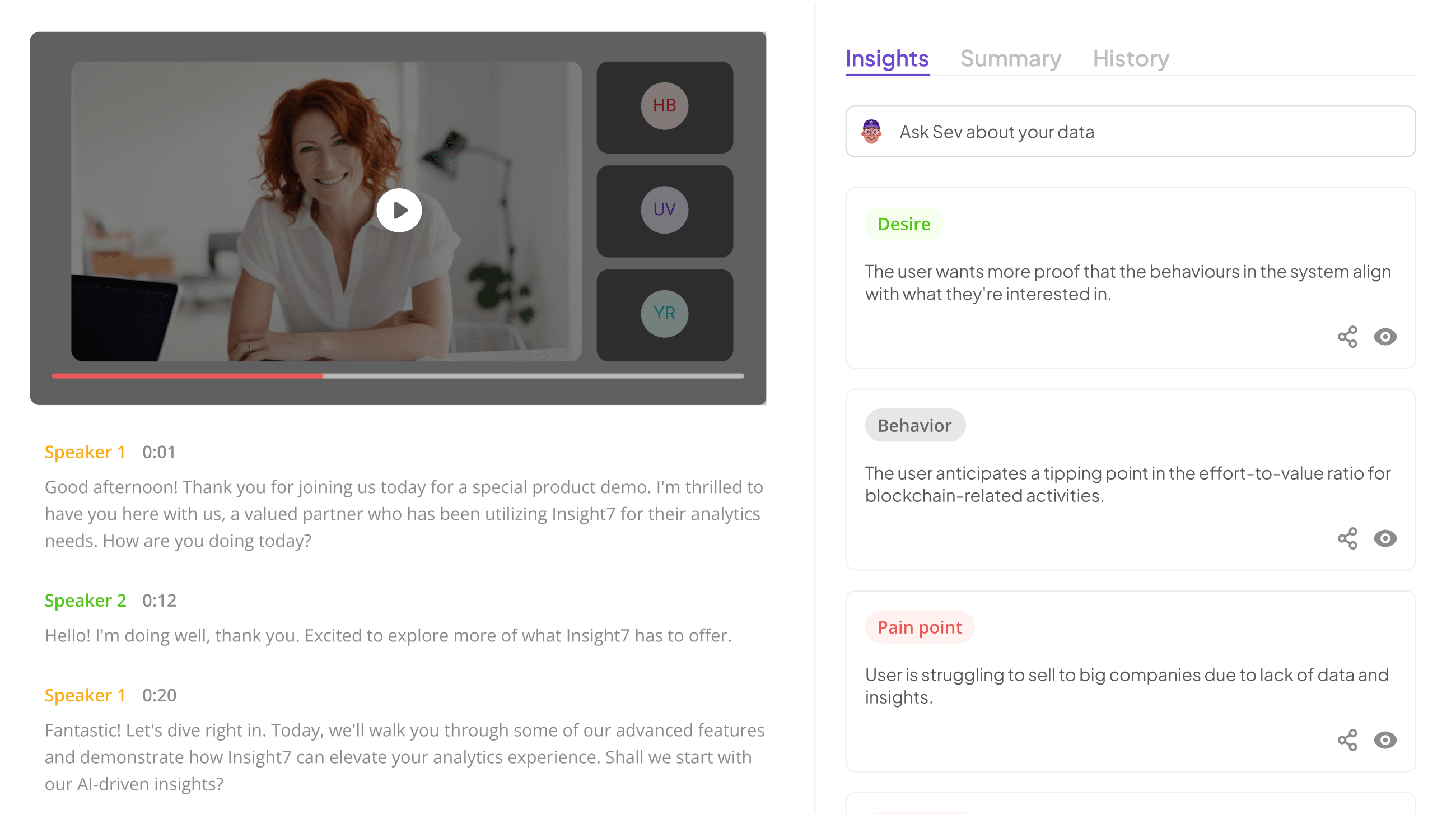