Response Clustering Techniques provide a structured approach to analyzing qualitative interview data, making sense of complex responses. By grouping similar responses, researchers can identify underlying themes that might otherwise remain hidden. This method not only enhances the clarity of insights but also streamlines the analysis process, allowing for faster and more effective reporting.
Understanding the importance of Response Clustering Techniques is crucial for researchers aiming to deliver reliable insights. With the growing demand for timely analysis, these techniques help mitigate issues such as bias and inconsistency across teams. As researchers navigate through qualitative data, mastering clustering strategies will empower them to extract meaningful insights efficiently.
Analyze qualitative data. At Scale.
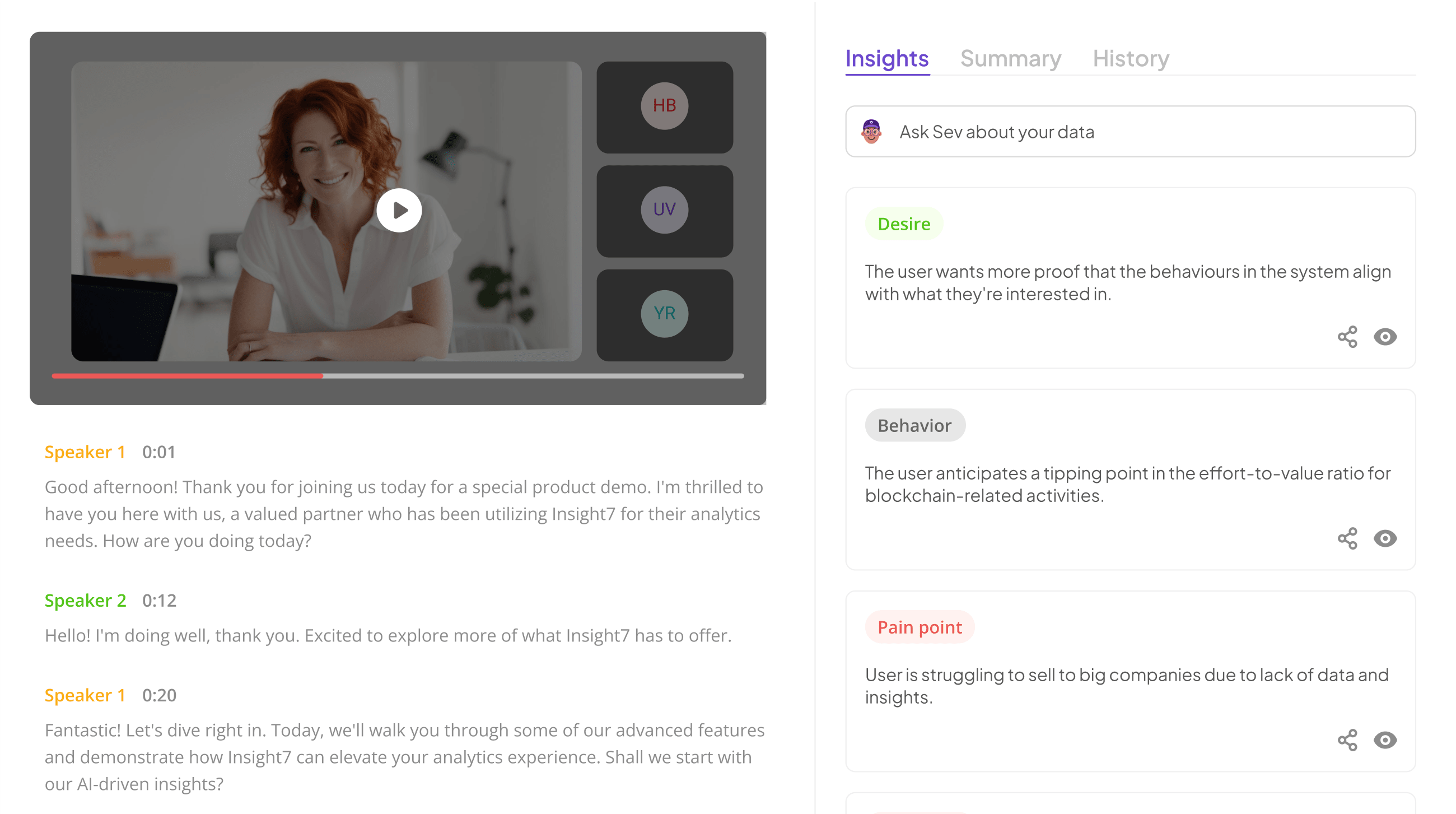
Understanding Response Clustering Techniques
Response clustering techniques play a critical role in organizing qualitative interview data. By systematically grouping responses based on similar themes or ideas, researchers can uncover patterns that may not be apparent at first glance. This process not only streamlines analysis but also enhances the interpretation of data, providing deeper insights into participant perspectives.
Understanding these techniques involves familiarizing oneself with various approaches, such as thematic analysis and content analysis. Thematic analysis focuses on identifying recurring themes, allowing for a structured understanding of complex issues. In contrast, content analysis emphasizes quantifying and categorizing responses, making it easier to compare data across different interviews. Both methods serve vital functions in response clustering, ultimately refining how qualitative data can be leveraged for meaningful conclusions. By mastering these techniques, researchers can effectively transform raw interview data into actionable insights that inform decision-making processes.
What is Response Clustering?
Response clustering is a technique used to organize qualitative interview data into meaningful groups. By clustering responses, researchers can identify overarching themes and patterns that emerge from participant feedback. This process allows for a more systematic analysis, making it easier to draw connections between various insights. As interview responses can often be diverse and complex, employing response clustering can significantly streamline the analysis.
There are several benefits to this approach. First, it enhances the interpretability of qualitative data, allowing researchers to see the bigger picture. Second, clustering methods provide a framework for organizing insights based on similar topics, making data less overwhelming. Finally, this technique aids in the identification of key trends and sentiments within the data. By understanding response clustering, researchers can ensure that they are extracting actionable insights from their qualitative data efficiently and effectively.
- Definition and importance in qualitative research
In qualitative research, the definition of response clustering techniques revolves around the systematic grouping of participant responses. This method allows researchers to identify patterns, themes, and significant insights that emerge from qualitative data. By organizing responses into clusters, researchers gain clarity on complex data, facilitating deeper analysis.
The importance of these techniques lies in their ability to enhance understanding and improve the quality of insights derived from qualitative data. Response clustering simplifies the analysis process, making it less time-consuming and more consistent across different researchers. It offers a structured approach, reducing bias and improving reliability in interpreting qualitative findings. Through response clustering, researchers can effectively address key questions, ensuring that the end results are actionable and relevant. This streamlined process is vital in meeting the increasing demand for timely insights in today's fast-paced research environment.
- Benefits of using clustering methods
Clustering methods offer significant advantages when conducting response clustering techniques in qualitative interview data. By organizing complex information into manageable clusters, researchers can identify key themes and patterns that emerge from interviews. This structured approach minimizes cognitive overload and allows for a more focused analysis. As a result, researchers can draw connections between various data points more efficiently, enhancing the overall interpretation of the findings.
Moreover, clustering supports the identification of insights that may not be immediately apparent. For instance, grouping similar responses helps illuminate broader trends and sentiments within the data. This can lead to richer, more nuanced results, enabling researchers to address specific research questions with greater clarity. In summary, utilizing clustering methods not only streamlines the analysis process but also deepens the understanding of the qualitative data collected, making it a valuable technique in qualitative research.
Types of Response Clustering Techniques
Response clustering techniques are critical for extracting meaningful insights from qualitative interview data. Several methods can be employed, with two prominent techniques being thematic analysis and content analysis. Each of these techniques serves distinct purposes and helps researchers navigate complex qualitative data.
Thematic analysis involves identifying, analyzing, and reporting patterns or themes within the data. This technique is particularly useful for highlighting overarching narratives and understanding participant perspectives. Conversely, content analysis focuses on quantifying and analyzing the presence of certain words, phrases, or concepts within the data. This method can be beneficial for revealing frequency and patterns that may not be immediately apparent.
By employing these response clustering techniques, researchers can streamline their analysis process, build consistency across their interpretations, and derive actionable insights. Such methods are vital tools for anyone looking to enhance the quality and efficiency of their qualitative research efforts.
- Thematic analysis
Thematic analysis is a fundamental approach in response clustering techniques, enabling researchers to identify and interpret patterns within qualitative interview data. This method begins with coding your data, where key phrases and ideas are tagged for easier reference. By coding, you essentially begin organizing the responses into meaningful categories, which can lead to the emergence of broader themes.
Once themes are identified, they are clustered together based on similarities and relevance to your research goals. This process allows for a deeper understanding of participant perspectives and experiences. Moreover, thematic analysis provides sentiment insights, which helps in capturing the underlying emotions associated with each theme. Ultimately, leveraging thematic analysis not only saves time but enhances the quality of your qualitative insights, ensuring they are more actionable and aligned with your research objectives.
- Content analysis
In content analysis, the focus lies on systematically examining qualitative data to identify patterns and themes. This process is essential for effectively organizing and interpreting information gathered from interviews. It allows researchers to distill complex interview data into manageable insights, which can be further analyzed using various response clustering techniques.
By defining themes and coding responses, content analysis enables researchers to categorize data into coherent groups. This categorization not only streamlines the analysis but also enhances clarity when presenting findings. Common techniques used include qualitative coding and theme mapping, which help structure the data further, ensuring that insights are easily accessible. Engaging in thorough content analysis can significantly boost the quality and reliability of research outcomes, making it a critical step in qualitative research methods.
Extract insights from interviews, calls, surveys and reviews for insights in minutes
Step-by-Step Guide to Conduct Response Clustering Techniques
To effectively conduct response clustering techniques, begin with preparing your interview data. This foundational step involves transcribing the conversations verbatim and organizing them into a manageable format. Cleaning the data helps ensure accuracy, allowing you to familiarize yourself with the content deeply. Understanding the nuances in participant responses lays the groundwork for the subsequent steps in the clustering process.
Next, focus on identifying key themes and patterns within the data. Start by coding the transcripts, which involves labeling segments of text with relevant tags or categories. Once you've established initial codes, group them into clusters that highlight overarching themes. This clustering analysis is crucial as it allows you to sift through large amounts of qualitative data, presenting insights that summarize the collective sentiments and experiences of participants. By systematically analyzing and interpreting these clusters, you can extract valuable conclusions that contribute to your research objectives.
Step 1: Preparing Your Interview Data
Preparing your interview data is a crucial first step in the response clustering techniques process. Begin by ensuring that all interview materials are transcribed accurately and organized systematically. This could involve using transcription software for efficiency and accuracy. Organizing your data by themes or participant identifiers allows for easier retrieval and analysis later on.
Once your data is organized, take the time to familiarize yourself with its contents. Read through the transcripts carefully, as this helps you grasp the nuances and context of the interview responses. Understanding the sentiments and perspectives expressed will lay a solid foundation for meaningful clustering. By properly preparing your data, you set the stage for effective analysis, enabling you to identify key themes and insights that emerge during the clustering phase.
- Data transcription and organization
Transcribing and organizing qualitative interview data is critical for effective analysis. This step involves converting audio or video recordings into written text, which ensures accurate capture of participants' insights. Using a transcription service simplifies this process, allowing for efficient bulk transcription to save time. Once transcripts are generated, they should be organized by project or themes to facilitate easy access and thoughtful review.
Subsequent to transcription, the organization of data plays a key role in response clustering techniques. Ensure you categorize the transcripts systematically, grouping similar responses together. This thematic organization helps in identifying key trends and patterns within the data. By meticulously organizing the transcripts, researchers can efficiently extract meaningful insights, paving the way for more effective analysis and interpretation. This process sets the foundation for successful coding and creates a structured approach to visualizing clusters in qualitative research.
- Familiarizing with the data
To effectively conduct response clustering techniques, it is essential to start with a thorough understanding of the data at hand. Familiarizing yourself with interview transcripts or audio files creates a solid foundation for analysis. This involves carefully reviewing the content to grasp the context and nuances of participant responses. Engaging with the data on multiple levels will enhance the accuracy of the eventual clustering process.
A few strategies can help in this familiarization stage. First, read through the transcripts thoroughly to identify initial themes and noteworthy ideas. Second, listen to audio recordings or view video recordings to capture tone and emotion, which may not be evident in text form. Lastly, organizing the data into manageable sections can make it easier to analyze. By taking these steps, researchers can prepare themselves to effectively apply clustering techniques, ensuring that they extract meaningful insights from their qualitative data.
Step 2: Identifying Key Themes and Patterns
In Step 2 of the response clustering process, the primary focus is on identifying key themes and patterns within your qualitative interview data. This stage builds upon the initial data preparation and encourages you to delve deeper into the content by coding it effectively. Start by assigning codes to segments of the data, capturing essential insights and characteristics of respondents' experiences. By categorizing these codes, you lay the groundwork for effective clustering.
Once coding is complete, group these codes into broader clusters. This grouping allows you to discern significant themes, such as challenges faced or desired improvements, that emerge from your data. The ability to draw these connections not only streamlines the analysis process but also aids in interpreting the nuances of respondents' feedback. By clearly defining these clusters, you can focus on the most relevant insights, ultimately leading to meaningful conclusions and actions.
- Coding the data
To effectively code the data in qualitative interviews, you should first familiarize yourself with the nuances of response clustering techniques. Begin by organizing your transcribed interview data into manageable segments. This might involve highlighting responses that resonate with specific themes or concepts, which will later serve as your foundational codes. Ensure that each segment captures a unique insight, as these will form the building blocks of your analysis.
Once you have organized the data, establish a coding system based on recurring themes or patterns. Identify key ideas that are significant in the context of your research objectives. As you move forward, group similar codes into clusters to reveal deeper insights within the qualitative data. This systematic approach not only simplifies the analysis process but also enhances the clarity of findings, allowing you to uncover meaningful interpretations that may inform your research conclusions.
- Grouping codes into clusters
Grouping codes into clusters involves organizing your coded data into meaningful categories that reflect underlying themes. This process is crucial when applying response clustering techniques, as it enables researchers to identify patterns and draw insights from qualitative interview data. Begin by examining your codes for similarities in content or context. As you identify these groupings, consider the nuances of the data. Clustering codes allows you to synthesize information and makes it easier to extract actionable insights.
To effectively cluster your coded data, here are several key points to consider:
Identify Common Themes: Look for codes that share commonalities, whether they relate to specific experiences or sentiments expressed by participants.
Use Visual Tools: Tools such as mind maps or thematic charts can help visualize relationships between codes, aiding in the clustering process.
Refine and Revise: As you group codes, be open to refining them. New insights may emerge that require re-evaluating your initial groupings.
Document Everything: Keep clear documentation of why codes were grouped together, ensuring transparency in your analysis process.
By clustering codes thoughtfully, you can transform scattered responses into coherent clusters that provide a richer understanding of the interview data. This structured analysis will ultimately enhance the quality and relevancy of your insights.
Step 3: Analyzing and Interpreting Clusters
In Step 3, analyzing and interpreting clusters requires a systematic approach to derive meaningful insights from your qualitative data. First, begin by carefully reviewing the indexed clusters generated from the coding process. This stage is crucial for identifying patterns and trends, which will help to illuminate the underlying themes within your data. Focus on the connections between different clusters and assess how they can address your research questions.
Next, extracting actionable insights is pivotal. Look for recurring sentiments and participant quotes that exemplify the themes. Organize these insights based on their relevance to your research objectives. By synthesizing this information, you can draw informed conclusions that reflect the perspectives of your participants. Utilizing response clustering techniques not only enhances your understanding but also equips you to present clear findings to stakeholders or in your reports. Ultimately, effective analysis transforms complex data into comprehensible and impactful narratives.
- Reviewing clustered data
When reviewing clustered data, the goal is to ensure that the themes extracted truly encapsulate the insights gleaned from interviews. This involves a systematic examination of identified clusters, where researchers not only assess the accuracy of the grouped responses but also dive deeper into their meaning. Crucially, this stage helps in discerning the subtleties that may not initially be apparent from surface-level analysis.
Researchers should employ techniques such as thematic and content analysis during this process. First, it's vital to revisit the coding framework used in the earlier steps. This helps in confirming that the codes align with their corresponding themes. Next, extracting insights involves looking at each theme's supporting quotes, enabling researchers to connect the qualitative data to specific participant testimonies. By navigating through this structured analysis, researchers can effectively synthesize data into meaningful conclusions and identify actionable insights.
- Extracting insights and conclusions
Extracting insights and conclusions from clustered data is a critical phase in qualitative research. This process begins once you have grouped responses into relevant themes, which allows for a clearer interpretation of the data. By analyzing these clusters, researchers can identify overarching trends and sentiments that may not be immediately apparent from individual responses.
In this stage, focus on summarizing key findings and correlating them with your initial research questions. Use the insights derived from response clustering techniques to draw informed conclusions. These conclusions should reflect a nuanced understanding of participant experiences and provide actionable recommendations based on the data analysis. Ultimately, this step not only enhances your understanding but also reinforces the value of qualitative research in understanding complex phenomena.
Conclusion: Harnessing the Power of Response Clustering Techniques in Research
In conclusion, harnessing the power of response clustering techniques can significantly enhance qualitative research outcomes. By effectively grouping responses, researchers can uncover key patterns and insights that may otherwise remain hidden. This method allows for a more organized analysis of data, making it easier to identify themes and trends relevant to the research objectives.
Moreover, employing response clustering techniques is not just about efficiency; it's about gaining deeper insights into participants' perspectives. As researchers dissect and categorize data, they can produce actionable recommendations that align with their findings. Ultimately, this structured approach supports informed decision-making and amplifies the voice of participants, enriching the overall research process.