Qualitative Data Clustering is an essential process in the realm of data synthesis, particularly for qualitative research. When researchers conduct interviews, they gather rich narratives that reveal perspectives and experiences, but these insights can be overwhelming in raw form. Clustering allows for the organization of this data, transforming scattered quotes and anecdotes into coherent themes that highlight significant findings.
Understanding the principles behind qualitative data clustering is crucial for effective analysis. It not only enhances the clarity of insights but also aids researchers in navigating complex information. By grouping related concepts and identifying overarching themes, you can ensure that critical nuances emerge, ultimately leading to a more profound synthesis of the interview data. This foundational understanding sets the stage for a deeper exploration into clustering methods and techniques.
Analyze qualitative data. At Scale.
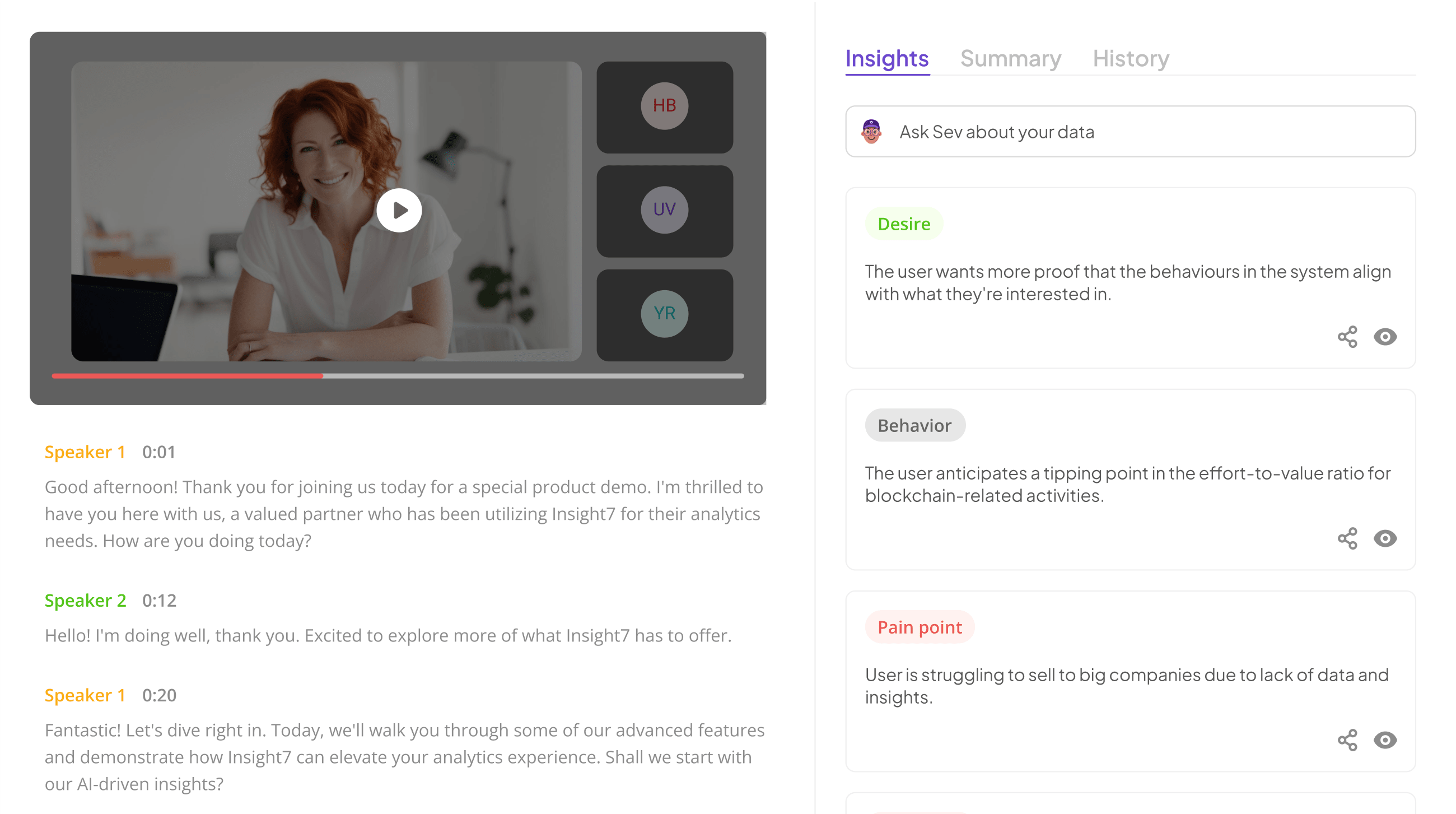
Why Cluster Qualitative Interview Data for Synthesis?
Clustering qualitative interview data for synthesis is essential for enhancing analytical effectiveness. This process allows researchers to organize diverse insights into coherent themes. When qualitative data is grouped meaningfully, it uncovers deeper patterns and connections that may otherwise remain hidden when analyzing responses individually.
Improved insights gained from clustering can streamline decision-making processes. With organized data, researchers can identify prevalent themes that resonate with stakeholder interests and needs. Additionally, qualitative data clustering saves time, enabling quicker synthesis and reporting. By mitigating biases and inconsistencies that may arise from individual interpretations, researchers can deliver more reliable and valid insights. Ultimately, clustering transforms raw qualitative data into actionable knowledge, paving the way for informed strategic decisions and fostering a deeper understanding of the research topic.
Benefits of Qualitative Data Clustering
Qualitative Data Clustering offers numerous advantages that enhance the analysis process and lead to more actionable insights. One primary benefit is improved insights derived from diverse participant responses. By clustering related data points, researchers can better identify recurring themes and significant patterns, helping them to understand participant sentiments and experiences on a deeper level.
Another notable benefit is the efficiency gained in data analysis. Clustering simplifies large volumes of qualitative data into manageable segments, allowing for quicker identification of critical insights. This organized approach not only saves time but also reduces the complexity of interpretation, enabling researchers to focus on synthesizing findings effectively. Overall, qualitative data clustering streamlines the research process, leading to more refined and impactful conclusions. By transforming raw data into structured insights, this methodology supports improved decision-making and strategy development.
- Improved Insights
Improving insights is a vital outcome of effectively clustering qualitative interview data. By organizing and categorizing responses, researchers can uncover patterns that might otherwise go unnoticed. This process illuminates key themes, allowing for a clearer understanding of participant experiences and perspectives. Improved insights can lead to actionable recommendations that inform strategy and decision-making.
The journey toward enhanced understanding begins with careful data organization. By transcribing interviews and developing a coding scheme, researchers set the stage for successful qualitative data clustering. Next, by identifying patterns and grouping related themes, the data transforms from a scattered collection of responses into a cohesive narrative. This synthesis not only reveals the deeper meanings within the data but also facilitates communication of findings to stakeholders, ensuring the insights are both accessible and impactful. In doing so, organizations can harness the full potential of qualitative data, paving the way for informed actions and strategic growth.
- Efficient Data Analysis
Efficient data analysis plays a pivotal role in the clustering of qualitative interview data. By systematically organizing and evaluating your findings, you enhance the clarity of insights derived from interviews. This process involves identifying themes and patterns that emerge from the raw data. As a result, analysts save significant time and energy while refining their understanding of complex narratives.
To achieve efficient qualitative data clustering, consider the following steps:
Organizing Your Data: Carefully transcribing interviews ensures that essential details are captured. Developing a coding scheme also aids in categorizing themes effectively.
Applying Clustering Techniques: This involves identifying recurring patterns and grouping related themes to simplify analysis. Such organization allows for a clearer interpretation of varied insights.
Synthesizing Clustered Data: Finally, integrate thematic clusters into a cohesive narrative. This creates a comprehensive understanding of participant experiences and drives meaningful conclusions from your qualitative analysis.
Common Challenges in Qualitative Interview Data Clustering
Clustering qualitative interview data presents several common challenges that analysts need to navigate. One major issue is data diversity. Interview responses can be rich and varied, covering a wide spectrum of perspectives and experiences. This diversity is valuable but can also complicate the clustering process, as it becomes essential to identify common themes amidst the noise.
Another significant challenge is the subjectivity in interpretation. Different researchers may have varying biases or viewpoints that affect how they perceive and categorize data. This inconsistency can lead to fragmented insights or misaligned clusters, which may cloud the analysis. Addressing these common challenges in qualitative data clustering is crucial for achieving accurate and actionable results, ultimately improving the overall quality of synthesizing insights for decision-making. By acknowledging these hurdles, teams can take proactive steps to enhance their clustering strategies and streamline their analysis process.
- Data Diversity
Data diversity plays a crucial role in qualitative research, particularly when clustering qualitative interview data for synthesis. Each participant brings unique perspectives, experiences, and backgrounds that contribute to a rich pool of information. This variety can enrich the analysis but also complicates the clustering process. Researchers must navigate the broad spectrum of opinions and themes to derive coherent insights.
When clustering qualitative data, it's important to recognize the distinct categories of diversity present, such as demographic variances, cultural influences, and individual experiences. A thoughtful approach to qualitative data clustering enables researchers to identify meaningful patterns while respecting the individuality of each data source. By embracing data diversity, researchers can unveil deeper narratives, leading to more robust conclusions and actionable insights. Ultimately, recognizing and honoring this diversity enhances the synthesis process, transforming a collection of interviews into a comprehensive understanding of the subject matter.
- Subjectivity in Interpretation
Subjectivity in interpretation plays a pivotal role in the clustering of qualitative interview data. Each researcher carries their own biases and perspectives, influencing how they perceive and categorize insights. This subjectivity can lead to varied interpretations of similar data, impacting the overall analysis. In qualitative data clustering, understanding these subjective influences is crucial for achieving consistency and reliability in findings.
One way to navigate subjectivity is through collaborative analysis, where multiple perspectives are gathered to reach a consensus. Team discussions can help uncover biases and establish a common framework for interpreting data. Furthermore, employing coding schemes that are thoroughly defined can minimize personal biases, enabling a more objective approach to clustering. It is essential to remain aware of how subjectivity can shape the analysis of qualitative data, as it directly affects the synthesis and validity of insights derived from interviews.
Extract insights from interviews, calls, surveys and reviews for insights in minutes
Steps to Effectively Cluster Qualitative Interview Data
To effectively cluster qualitative interview data, begin by organizing your raw information systematically. Start by transcribing interviews accurately, ensuring that every detail is captured. Once transcriptions are ready, develop a coding scheme that categorizes responses based on prevalent themes or topics. This foundational step lays the groundwork for efficient qualitative data clustering, aiding in the smooth identification of patterns later.
Following proper organization, apply qualitative data clustering techniques. Focus on identifying recurring patterns within your data that signify significant themes. Group these themes to uncover relationships and connections in participant responses. After clustering, synthesize the data by integrating these thematic clusters into a cohesive narrative. This approach not only enhances understanding but also facilitates actionable insights drawn from qualitative research. Ultimately, these steps ensure a structured pathway for analyzing qualitative data, leading to deeper insights and informed decision-making.
Step 1: Organizing Your Data
To effectively cluster qualitative interview data, the first crucial step involves organizing your data systematically. Start by transcribing interviews accurately; this creates a text-based representation of your discussions. Clear transcription ensures that you capture intricate details, emotions, and nuances, which are essential for meaningful analysis. Make sure to review the transcripts for any inaccuracies that could lead to misinterpretations later on.
Next, develop a coding scheme to categorize the data effectively. This involves identifying key themes and concepts, assigning codes that resonate with your findings. Implementing a coherent coding system allows for easier tracking of insights as you progress in your qualitative data clustering. Remember, well-organized data forms the foundation for insightful analysis and synthesis later on in your project. Having strong organizational strategies in place is vital for unlocking the rich potential of qualitative insights hidden within your data.
- Transcribing Interviews
Transcribing interviews is a critical first step in the qualitative data clustering process. It transforms spoken dialogue into written text, facilitating easier analysis and synthesis of information. Accurate transcription allows researchers to capture the nuances of participants' experiences and insights, which can be pivotal for understanding the themes that emerge from the data.
To ensure high-quality transcriptions, consider using reliable transcription tools that can handle bulk uploads and support multiple languages. Quality matters here; an accuracy rate of 95% is ideal for retaining the essence of conversations. Once transcribed, the text serves as the foundation for developing coding schemes and clustering qualitative data effectively. As researchers delve into the transcription, they can begin to identify patterns and themes, paving the way for a nuanced understanding of the qualitative insights gathered through interviews. This structured approach ultimately enhances the efficiency of analysis and the power of the resulting synthesis.
- Developing a Coding Scheme
A coding scheme is essential in the qualitative data clustering process, as it provides a structure for organizing your insights. Begin by identifying key themes and concepts that emerge from your interviews. This initial step involves carefully reading through the interview transcripts to pinpoint recurring ideas or sentiments. Once these themes are recognized, you can develop specific codes that represent them, creating a clear framework to categorize the data.
Next, it is crucial to apply these codes consistently throughout all interview transcripts. This consistency will help you maintain an organized approach when analyzing the data. For effective qualitative data clustering, ensure each code has a clear definition and examples from the transcripts to support it. Not only does this make your analysis more reliable, but it also allows for easier integration of insights later. Ultimately, this coding framework serves as a foundational tool, guiding the synthesis of clustered qualitative data into meaningful narratives.
Step 2: Applying Qualitative Data Clustering Techniques
To effectively apply qualitative data clustering techniques, it is essential to first identify patterns within the qualitative interview data. This involves thoroughly reviewing transcripts and coding responses to uncover recurring themes or categories. By taking a systematic approach, you can classify your data into meaningful groups that reflect the underlying sentiments and perspectives shared by participants.
Once patterns are recognized, the next step is to group these themes logically. This clustering not only simplifies the data but also facilitates deeper analysis. Each cluster should encapsulate a distinct aspect of the qualitative insights, and it can significantly enhance your understanding of the data. With a clear organization of clustered themes, you can create a comprehensive narrative that highlights key insights and supports your objectives for data synthesis. By following this structured approach, qualitative data clustering can lead to more effective synthesis and actionable outcomes.
- Identifying Patterns
Identifying patterns in qualitative data is a critical step in the clustering process. This stage involves recognizing recurring themes and ideas that emerge from interview responses. By closely analyzing the narratives shared during interviews, researchers can pinpoint significant insights that reflect participants' experiences. These patterns help to distill complex data into more manageable, meaningful clusters.
To effectively identify patterns, consider a few strategies. First, review your transcriptions or notes multiple times to uncover any latent themes. Second, employ coding techniques to categorize similar responses, which can illuminate overarching trends. Lastly, use visual tools, like mind maps, to connect these themes and highlight relationships among them. This structured approach not only clarifies the data but also facilitates the synthesis of insights, ultimately enhancing the overall understanding of the qualitative research.
- Grouping Themes
Effective qualitative data clustering hinges on grouping themes that emerge from your interviews. This process is essential for transforming raw data into coherent insights. Begin by reviewing your transcriptions and identifying recurring patterns or concepts that stand out. Grouping these themes involves categorizing similar ideas or sentiments expressed by participants, allowing you to streamline your analysis.
Once the themes are laid out, examine the relationships between them. This interaction can reveal deeper insights into the underlying motivations or challenges within your data. For instance, themes related to customer engagement may link closely to issues of satisfaction and pain points. By systematically organizing these thematic clusters, you not only enhance the clarity of your findings but also strengthen the basis for actionable recommendations. Ultimately, grouping themes is vital for developing a comprehensive understanding of your qualitative data and its implications.
Step 3: Synthesizing the Clustered Data
To synthesize clustered qualitative data effectively, focus on integrating thematic clusters that emerge from your analysis. Begin by reviewing the identified themes and insights derived from your qualitative data clustering process. This step is crucial, as it enables you to pinpoint common patterns or unique perspectives among the clustered data. By establishing connections between these themes, you will find that your analysis provides a more rounded understanding of the subjects at hand.
Next, craft a cohesive narrative that weaves together these insights. This narrative should clearly articulate the relationships among themes, showcasing the overall context and meaning. Consider incorporating quotes or specific examples from your interviews to reinforce your findings. By synthesizing the clustered data, you not only highlight the key insights but also enhance the clarity and relevance of your conclusions. In doing so, the synthesized data can guide future actions and inform decision-making processes effectively.
- Integrating Thematic Clusters
Integrating thematic clusters is a critical step in the qualitative data clustering process. Once you have grouped your qualitative data into distinct themes, the next logical step is to synthesize these clusters into a coherent narrative. This ensures that the insights derived from the interviews are not only organized but also presented in a manner that tells a compelling story. Each thematic cluster will highlight key findings, allowing for easier identification of trends, challenges, and potential solutions.
To effectively integrate these thematic clusters, you should follow a few key approaches. First, ensure that you relate each cluster to your research questions or objectives. Next, examine the relationships between clusters, noting how they may influence or complement each other. Finally, highlight representative quotes or examples from the interviews to enrich your findings. This integration process enhances the visibility of insights while fostering a deeper understanding of the qualitative data gathered.
- Crafting a Cohesive Narrative
A compelling narrative emerges from effectively synthesizing qualitative interview data. This process begins with identifying and clustering relevant themes that reflect participants' insights and experiences. By interconnectedly linking these themes, researchers create a rich tapestry of understanding that drives actionable conclusions. This cohesive narrative not only highlights key findings but also narrates a holistic story, allowing stakeholders to grasp the essence of the data collected.
Crafting such a narrative involves careful integration of clustered data, ensuring that each theme speaks to the overall objective of the research. It is crucial to contextualize quotes and insights, providing clarity and depth to the narrative. Ultimately, this approach enriches the presentation of qualitative insights by making them more engaging and easily digestible for varied audiences. A well-crafted narrative transforms raw data into strategic recommendations, fostering informed decisions and effective implementation.
Conclusion: Mastering Qualitative Data Clustering for Enhanced Insights
Mastering qualitative data clustering enables researchers to draw meaningful insights from complex interview data. This approach transforms vast and varied responses into organized clusters, making it easier to identify themes and patterns. By grouping similar ideas, one can uncover underlying trends that may not be immediately apparent, thereby enriching the analysis.
Moreover, effective clustering enhances the synthesis process, allowing for a clearer narrative that highlights key findings. By implementing structured techniques, researchers can efficiently navigate through qualitative data, ensuring that their insights are both actionable and relevant. Ultimately, mastering qualitative data clustering not only streamlines the analysis but also fosters a deeper understanding of the researched phenomena.
Analyze qualitative data. At Scale.
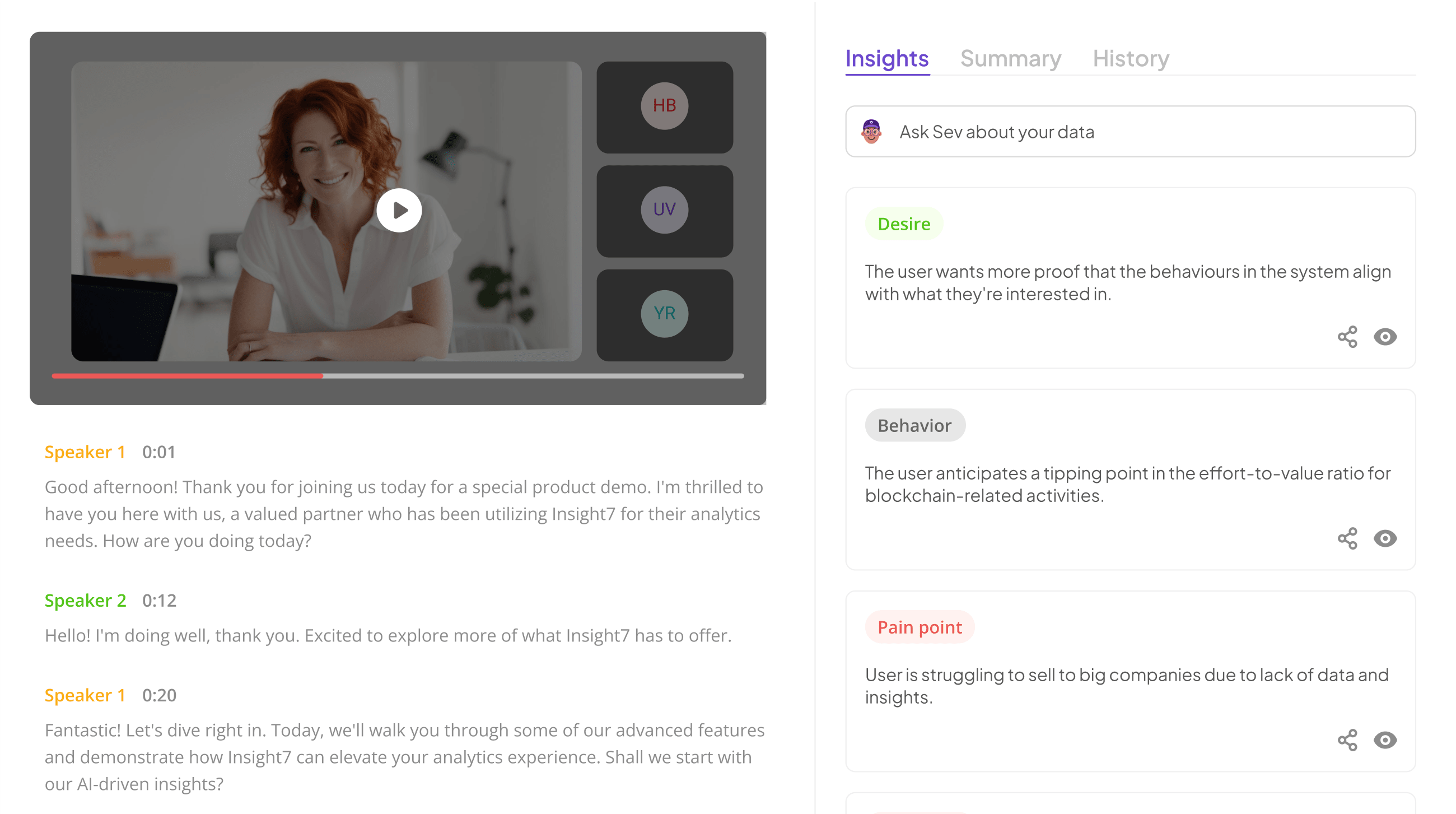